BACKGROUND
Severe acute respiratory syndrome coronavirus 2 (SARS-CoV-2) is the highly contagious and virulent coronavirus responsible for the global outbreak of coronavirus disease 2019 (Covid-19). Between 2020 and 2023, Covid-19 imposed an unprecedented burden on global public health systems, and by December 2023, it had been responsible for at least 770 million reported cases and close to 7 million reported deaths. 1
The pandemic’s containment and the restoration of societal normalcy have been achieved through two key routes: (i) the development and widespread use of SARS-CoV-2 vaccines and (ii) the gradual increase in natural infection-acquired immunity. While progress has been made, critical hurdles remain, notably the need for equitable vaccine distribution and effective treatment options for those unvaccinated or immunocompromised. 2 Furthermore, there are also some concerns surrounding vaccine efficacy against a backdrop of waning immunity 2, 3 and the emergence of immunoevasive viral strains. 4, 5 It is now a widely held view that Covid-19 is likely to transform into another endemic human coronavirus, possibly with seasonal epidemic waves. 6 Irrespective of the number of infections that occur or the intensiveness with which vaccines are used, it remains uncertain whether true herd immunity of sorts achieved with measles and rubella will ever be achieved for Covid-19.
While data are currently being collected and analyzed to understand how newly evolved SARS-CoV-2 variants might impact the effectiveness of vaccines and the severity of future Covid-19 infection waves, there remains a demand for both host-directed and pathogen-directed drugs that could be utilized to treat the mild, moderate, and severe manifestations of the disease.
In light of this, multiple existing drugs have been sought to treat or control SARS-CoV-2 infection. 7 An example is ritonavir-boosted nirmatrelvir ( Paxlovid) (DrugBank: DB16691), a protease inhibitor used for the treatment of mild to moderate Covid-19 in adults who are at high risk of developing severe symptoms. 8 Additionally, remdesivir (Veklury) (DrugBank: DB14761), an adenosine triphosphate (ATP) analog targeting the conserved viral RNA-dependent RNA polymerase, shortens the recovery time for adults hospitalized with Covid-19 infection and pneumonia, while also mitigating disease severity and associated mortality. 9– 11 Other virus-directed antiviral drugs like favipiravir (DrugBank: DB12466), molnupiravir (DrugBank: DB15661), and ritonavir (DrugBank: DB00503) may also potentially improve the health outcomes of Covid-19 patients. 12 Furthermore, a host-directed drug such as dexamethasone (DrugBank: DB01234), an anti-inflammatory corticosteroid, has demonstrated its effectiveness in reducing mortality among severely infected patients. It achieves this by modulating inflammation-mediated lung injury, preventing in some cases progression to respiratory failure and death. 13 Another host-directed drug, aspirin (DrugBank: DB00945), 14 decreases the risk of complications and mortality in hospitalized Covid-19-infected patients. 14– 16
While these drugs are mainly utilized for severe Covid-19 cases, some virus-directed monoclonal antibodies (e.g., bebtelovimab, casirivimab) received emergency use authorization at various stages of the pandemic for managing mild to moderate Covid-19. 17 Additionally, other monoclonal antibodies including the combination of bebtelovimab, casirivimab, and imdevimab and the combination of bamlanivimab and etesevimab have been useful for managing mild to moderate Covid-19 in adults. 17 These monoclonal antibodies inhibit viral entry into host cells by preventing viral attachment to human ACE2 receptors.
Host-targeted monoclonal antibodies such as tocilizumab (DrugBank: DB06273) and sarilumab (DrugBank: DB11767), 18– 21 which modulate aberrant immune responses to infection by binding to the host interleukin 6 (IL6) receptor (IL6R), have also been granted emergency use authorization. Specifically, these IL6R-binding monoclonals are used for treating hospitalized adults and pediatric patients (2 years of age and older) who are receiving systemic corticosteroids and require supplemental oxygen, non-invasive or invasive mechanical ventilation, or extracorporeal membrane oxygenation. 21
Although existing drugs have been recommended for managing Covid-19, concerns have arisen about post-hospitalization effects and the appropriateness of using these drugs in different Covid-19 disease phases. 22– 24 Covid-19 exhibits a wide spectrum of symptoms and severities, necessitating a nuanced approach to treatment. Personalized medicine, where the right drug is administered to the right patient group at the right disease phase, could revolutionize Covid-19 treatment strategies. Hence, it would be useful to identify effective drugs that are specific to different phases of the disease, and which could also be potentially applicable to combating any future emergence of other coronaviruses.
Given that the development of new medications is a time-consuming process, the repurposing of existing medications for other indications may prove to be a viable alternative. However, most studies that have implemented computational methods to identify drug repurposing candidates for Covid-19 have so far leveraged disease–gene associations, protein–protein interaction, and drug–target data, but less consideration is given to the interactions between other biomedical and molecular features specific to different Covid-19 disease phases, such as those recorded in large-scale multi-omics profiling efforts. 25– 28 Applying computational multi-omics data-driven analysis to the repurposing of existing medications is, in fact, a potentially and highly efficient means of drug discovery since the pharmacological properties, formulations, and toxicities of such agents are already known. 25– 31 In this study, we explore the utility of incorporating disease state-specific omics graphs (DSOGs) along with drug-related data to identify drug repurposing candidates for mild, moderate, or severe Covid-19 disease states. We employ MultiXrank, 32 a random walk with restart (RWR) algorithm that can combine multiple heterogeneous networks and allows for universal multi-layer network exploration. We demonstrate that this integrative multi-omics network-based approach with drug data has the potential to repurpose drugs in different disease states and can be applied to other diseases.
MATERIALS AND METHODS
Study Design and Procedures
The methodology employed in this study ( Figure 1 ) encompasses five main steps: (i) curation and pre-processing of data related to the action of drugs and to the molecular omics profiles associated with the different phases of the disease; (ii) multi-layer network-based random walk analysis; (iii) predicting drug repurposing candidates; (iv) drug prediction robustness analysis; and (v) validation of predicted candidate drugs.
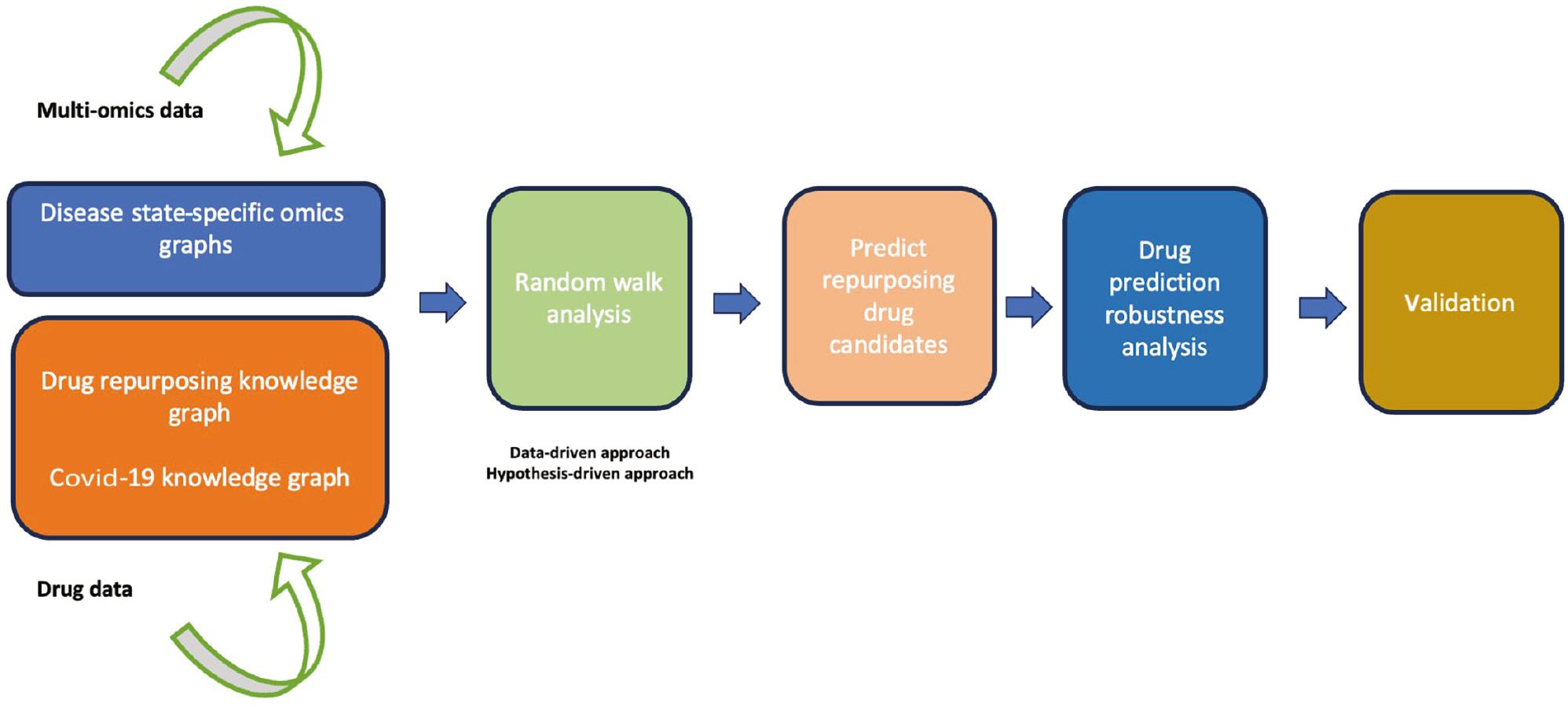
Diagram illustrating the workflow implemented in this study. The workflow begins with curating multi-omics data and drug data, followed by a random walk with restart network analysis using both data-driven and hypothesis-driven approaches. Next, we prioritized and characterized candidate drugs, followed by drug prediction robustness analysis. Finally, we concluded the analysis by validating the predicted drug candidates.
Drug Repurposing Knowledge Graph, Covid-19 Knowledge Graph, and DSOGs
We utilized an existing drug repurposing knowledge graph (DRKG) constructed by Ioannidis et al. 31 The DRKG is a biological knowledge graph relating genes, compounds, diseases, biological processes, side effects, and symptoms as of 2020 when it was constructed. The DRKG includes information from six existing databases: (i) Global Network of Biomedical Relationships, 33 (ii) STRING, 34 (iii) IntAct, 35 (iv) Hetionet, 36 (v) DrugBank, 37 and (vi) Drug–Gene Interaction Database. 38 The DRKG includes 97,238 entities classified into 13 different node types ( Table 1 ) and consists of 5,874,261 triplets belonging to 107 edge types ( Supplementary Table 1 ). We leveraged the gene pathway and gene biological process edge types ( Supplementary Table 1 ) in the DRKG to construct pairwise interaction between biological processes and pathways based on the semantic relation that biological processes and pathways that share similar disease-related genes are indirectly associated. This was achieved by exploring the associations between genes, biological processes, and pathways to investigate the biological processes and pathways enriched among the disease-associated genes. We then paired pathways and biological processes sharing common genes.
Description of the Node Types in the Drug Repurposing Knowledge Graph.
Node Type | Number of Features |
---|---|
Anatomy | 400 |
Anatomical Therapeutic Chemical | 4048 |
Biological process | 11,381 |
Cellular component | 1391 |
Compound | 24,313 |
Disease | 5103 |
Gene | 39,220 |
Molecular function | 2884 |
Pathway | 1822 |
Pharmacologic class | 345 |
Side effect | 5701 |
Symptom | 415 |
Taxonomy | 215 |
We additionally considered the Covid-19 knowledge graph (Covid-19 KG) built by Hsieh et al. in 2021. 29 This Covid-19 KG is notable for its integration of drug data from the Comparative Toxicogenomics Database, specifically data available as of 2021, 39 along with protein–protein interactions involving SARS-CoV-2 and host proteins from a study by Gordon et al. 40 In addition to the virus–host interaction data, we also included SARS-CoV-2 and host protein interactions from IntAct database. 35 The SARS-CoV-2 and host protein interaction data extracted from IntAct were derived from several studies that examined protein–protein interactions between SARS-CoV-2 and humans. Following the merge of the Covid-19 KG and virus–human protein interaction data, the resulting graph represents the interactions between entities belonging to five different node types ( Table 2 ) and consists of 33,621 triplets belonging to five edge types ( Supplementary Table 2 ).
Description of the Node Types in the Covid-19 Knowledge Graph.
Node Type | Number of Features |
---|---|
SARS-CoV-2 baits | 23 |
Host genes and drug targets | 10,959 |
Pathways | 274 |
Drugs (chemical/compound) | 4266 |
Biological process (phenotypes) | 1893 |
Covid-19, coronavirus disease 2019; SARS-CoV-2, severe acute respiratory syndrome coronavirus 2.
Furthermore, we considered DSOGs constructed from our previous study 41 for downstream analysis. The DSOGs were constructed by integrating harmonized proteomics, transcriptomics, metabolomics, and lipidomics datasets retrieved from the studies by Overmyer et al. 42 and Su et al., 43 together with a unified knowledge graph, assembled by merging protein–protein interactome, metabolite–metabolite interactome, transcript–transcript interactome, and lipid–lipid interactome curated from literature and databases. 41 The DSOG consisted of four node types (i.e., protein, transcript, metabolite, and lipid) and nine edge types (i.e., protein–protein, transcript–transcript, metabolite–metabolite, lipid–lipid, protein–transcript, protein–metabolite, transcript–metabolite, protein–lipid, and transcript–lipid).
The process used to construct the graphs is described in Agamah et al. 41 In summary, the World Health Organization Ordinal Scale was used as a disease severity reference to harmonize Covid-19 patient metadata across the studies by Overmyer et al. 42 and Su et al. 43 These harmonized metadata were then used to categorize the multi-omics data into mild, moderate, and severe Covid-19 disease phases. Subsequently, a correlation network approach was implemented to construct co-expression networks for proteomics, transcriptomics, metabolomics, and lipidomics data for each disease state. The co-expression networks generated were integrated/merged based on the disease state and omics data type to construct DSOGs.
Overall, these above data sources were used to construct a unified and integrated knowledge graph comprising three subgraphs including the Covid-19 KG, the DRKG, and the DSOG from which we conducted an in-depth quality check (see the section Data Pre-processing, Quality Control, and Filtering ), prioritize, characterize, and repurpose specific drugs for the mild, moderate, and severe state of Covid-19.
Data Pre-processing, Quality Control, and Filtering
We observed differences in the gene and drug identifiers across the DRKG and Covid-19 KG. To achieve consistency in the identifiers across the datasets, we mapped gene identifiers onto gene symbols using the UniProt database resource 44 and drug identifiers onto drug names using the DrugBank database. 37 To identify clinically approved drugs with known safety profiles and pharmacokinetic properties, we filtered/cleaned the drug-related data by maintaining (i) drug–drug interactions between US Food and Drug Administration (FDA)-approved drug candidates, (ii) drug–protein/gene interactions between FDA-approved drug candidates and proteins/genes, and (iii) biological process/pathway–chemical interactions between biological processes/pathways and FDA-approved chemicals. As our investigation is centered around identifying potential repurposable drugs for Covid-19, we took additional measures to refine our analysis. To prioritize approved drugs with known safety profiles for Covid-19, we specifically removed interactions involving drugs that have been studied (based on literature evidence) and found to lack therapeutic effectiveness in treating Covid-19, as is the case with quinolones like chloroquine and hydroxychloroquine. 45, 46 Moreover, we omitted certain endogenous substances including hormones such as progesterone, testosterone, and melatonin, as well as alcohol (ethanol), various compounds like cholesterol, and cocaine, and gases such as oxygen and hydrogen from our analysis. Overall, we utilized about 2782 FDA-approved drugs for downstream analysis.
RWR Network Analysis
The random walk method is a technique for detecting the spread of biological information through networks. The concept behind the random walk method is such that a hypothetical particle exploring the network structure takes discrete steps (walks) in some direction from a seed node. 47 The walk explores different layers based on the premise that related nodes lie close to each other in the network. 47 To perform RWR network analyses, we utilized MultiXrank, 32 an RWR on a multi-layer network algorithm, to explore DSOGs, Covid-19 KG, and DRKG. Whereas the MultiXrank algorithm enables random walk analysis on multiple large multidimensional datasets in a multi-layer network framework, other methods are limited in the combination and heterogeneity of networks that they can handle. 48 In brief, the first step of the algorithm is to create adjacency matrices for the input graphs, followed by computing different transition probabilities of the RWR on the graphs. The probabilities are estimated based on the concept that an imaginary particle starts a random walk from the seed node to other nodes in the network. These different transition probabilities describe the walks within a graph and the jumps between graphs. A higher probability score (close to 1) suggests a higher likelihood of walking or jumping between graphs. We made specific adjustments to the algorithm’s configuration script based on the input datasets. While the global restart probability was set at 0.7, the intra-layer jump probability and the probability to restart in a specific layer were set to 0.5 and 1, respectively. Other parameters such as inter-layer jump probability were fine-tuned to align with the number of input graph layers. We set parameters in the algorithm to control the behavior of the algorithm to achieve desired outcomes.
As a result of this analysis, we obtained multi-layered graphs that detailed the exploration of seed nodes across various omics layers and drug data, along with a ranked list of features in each graph layer.
RWR analysis on the DRKG, Covid-19 KG, and DSOG
We performed an initial analysis focused on identifying drugs that can be repurposed for Covid-19 without accounting for omics profiles. Thus, we employed the DRKG and Covid-19 KG as the input data sources in the RWR algorithm to predict candidate drugs for Covid-19. The algorithm accepts as layers, monoplex graphs, and/or a combination of monoplex graphs (multiplex). Specifically, in our analysis, edge types with the same node entities including the drug–drug and gene–gene interactions each served as a monoplex and were interconnected by edge types with different node entities including the gene–drug, pathway–gene, phenotype (biological process)–gene, phenotype (biological process)–drug, and SARS-CoV-2–host gene interactions ( Supplementary Tables 1 and 2 ).
In subsequent analysis to predict potential drugs for different Covid-19 disease states, we utilized the DRKG, Covid-19 KG, and DSOG as input data. Herein, the input data included the proteomics, transcriptomics, metabolomics, and lipidomics disease state graphs, which also served as individual monoplex layers. Similar to the analysis on the DRKG and Covid-19 KG, graphs with edge types of the same node entities were interconnected by graphs with edge types of different node entities including the protein–transcript, protein–metabolite, transcript–metabolite, protein–lipid, and transcript–lipid interactions from the DSOG which were not utilized in the previous analysis.
Prediction of drugs using the RWR algorithm is based on a network exploration process where simulated particles walk iteratively from one node to one of its neighbors with some probability. In this process, the walk is restricted to restart from seed nodes to prevent the random walker from being trapped in dead ends. 47
Selection of seeds for RWR based on a hypothesis- and data-driven approach
To select seed nodes for the analysis, we implemented two approaches: (i) a hypothesis-driven approach where we selected seeds based on their impact on disease severity to test the hypothesis of their differential associations with mild, moderate, and severe Covid-19 disease states and (ii) a data-driven approach where we selected, after merging the different co-expression networks, the features with the highest node integrated centrality score in each omics layer as seeds. The hypothesis-driven approach has the advantage of bringing the question being investigated into focus by designing the model with a specific biological hypothesis in mind and exploring variations across disease phases, whereas the data-driven approach enables a more unbiased and informed model. 49, 50 Although hypothesis- and data-driven modeling approaches are not mutually exclusive, it is worth noting that this diversity is beneficial: Most model-building tools and models have a specific and clear role; however, at the same time, combining hypothesis- and data-driven approaches in an interoperable way provides an immense impact on our understanding of the disease phases as modeling and integrating data at different biological scales. 49, 50
IL6 and IL6R features were used as hypothesis-driven seeds for the random walk analysis because of the evidence for their significant role in the pathology of SARS-CoV-2 and Covid-19. 51– 53 IL6 is a cytokine, a type of signaling molecule involved in various inflammatory and immune responses. The inflammatory response plays a critical role in Covid-19, with an excessive inflammatory response leading to a “cytokine storm” increasing the severity of Covid-19. Since IL6 interacts with cells via IL6R, it has been hypothesized that inhibition of IL6R might reduce the likelihood of cytokine storms developing, ameliorate the symptoms of severe Covid-19, and reduce mortality. 48 In this context, we, therefore, used IL6 and IL6R as hypothesis-driven seeds in an RWR analysis that we refer to as our “hypothesis-driven approach.”
For an RWR using data-driven seeds, we selected seeds following the approach described in our previous study. 41 Specifically, we selected, after merging the different co-expression networks, the features with the highest integrated centrality score in each omics layer. 41 The features were ranked by leveraging the node degree, closeness, betweenness, and eigenvector centrality metrics to compute an integrated score (see Supplementary data, Equation 1 ). These centrality metrics provide insight into the importance of a node. For instance, the closeness metric measures how close a node is to all other nodes in the network. A lower closeness centrality score indicates the node is on average closer to other nodes, potentially making it a faster “information hub.” The degree metric measures the number of connections (edges) a node has with other nodes. A higher degree signifies the node has more direct connections, suggesting it might be more influential or receive more information flow. The betweenness metric captures how often a node lies on the shortest path between other pairs of nodes in the network. A higher betweenness score suggests the node acts as a crucial bridge for information flow within the network. The eigenvector metric considered goes beyond the number of connections and considers the “importance” of a node’s neighbors. Nodes with high eigenvector centrality are considered influential due to their connections to other influential nodes. By integrating these diverse perspectives, the calculated score provides a comprehensive understanding of a node’s relative importance within the network structure and its potential role in information flow and communication. Herein, the RWR analysis using the data-driven seeds is referred to as our “data-driven approach.”
Ranking candidate drugs for Covid-19 disease states
The RWR approach has the benefit of capturing the global topology of a graph and representing a measure of proximity from all the nodes to the seed(s) based on the graph topology. 54 The measure of proximity between nodes is a relevant measure quantifying how closely connected a node is with the seeds and can be used to rank nodes. 47, 54, 55 Overall, the network’s structure and connectivity patterns impact how random walks propagate through the nodes and influence the ranking of nodes based on their importance and relevance. Highly connected nodes or hubs may attract more random walkers and have a greater influence on the ranking of nodes. In this study, nodes within each layer were ranked based on their measure of proximity to the seed nodes, representing the relatedness of nodes to the seeds. The measure of proximity was the geometric mean of the node’s proximity to the seeds. 47
RESULTS
Predicting Candidate Drugs Using Existing Knowledge Graphs and Hypothesis-driven Seeds
To predict potential Covid-19 drugs, we systematically analyzed the DRKG and Covid-19 KG excluding DSOGs by implementing an RWR analysis (see Materials and Methods ). The RWR is an approach that allows for the exploration of the DSOGs, Covid-19 KG, and DRKG to identify patterns and prioritize features within the network. The algorithm, MultiXrank, conducts multiple random walks over the graphs, each originating from the seed nodes. These walks iteratively traverse from one node to a neighboring node at random, thus simulating a pattern that results in a multi-layered graph. In our hypothesis-driven approach, we selected IL6 and IL6R as seeds given their established roles as aggravators of the disease. The random walk process restricts the restarts from seed nodes ( IL6 and IL6R) during network exploration. The RWR analysis revealed a multi-layered graph describing the random walks from the seed nodes ( Figure 2 ) and a set of potential therapeutics ( Table 3 ) for the treatment of Covid-19. These included immunosuppressants, vital minerals, anticancer agents, antivirals, antibiotics, angiotensin receptor blockers, and corticosteroids (as detailed in Table 3 ). Notable among these are presently recommended drugs for treating the disease such as tocilizumab, 21, 56, 57 dexamethasone, 13 and losartan. 58, 59 Furthermore, our analysis pinpointed additional potential pharmaceutical options, such as cannabidiol and doxorubicin. 60– 62 While these have yet to be definitively endorsed for Covid-19 treatment, prior research indicates their potential candidacy based on their efficacy and merits for further experimental validations. 60, 63 Figure 2 shows a multi-layered graph generated from the RWR analysis highlighting interactions involving highly ranked drug candidates and other features such as IL2, IL1B, HCK, and TYK2.
Top 20 Potential Covid-19 Drugs, Ranked According to Their Measure of Proximity to IL6 and IL6R Seed Nodes as Determined Through the RWR Analysis of the DRKG and Covid-19 KG.
Drug Name | Drug Category | Mechanism of Action Potentially Linked with Covid-19 | Measure of Proximity | Reference a |
---|---|---|---|---|
Tocilizumab b | IL6R antagonist | Suppresses immune response by blocking IL6 signaling | 0.0353861 | 21 |
Zinc | Essential mineral/nutrient | Interferes with viral RNA synthesis to inhibit replication | 0.0007941 | 123 |
Sirolimus | Immunosuppressive drug | Expresses immunomodulatory and anti-inflammatory properties and inhibits the expression of pro-inflammatory cytokines | 0.0003784 | 124, 125 |
Choline | Essential nutrient | Supports cell membrane integrity and neurotransmitter function | 0.0003440 | 126 |
Ivermectin | Antiparasitic drug | Inhibits viral replication and modulates the host immune response | 0.0003056 | 127 |
Dactinomycin | Anticancer | Expresses immunomodulatory properties and inhibits viral cellular transcription | 0.0002734 | 79, 80 |
Losartan | Angiotensin receptor blocker | Reduces the activity of the renin–angiotensin system | 0.0002327 | 128 |
Ribavirin | Antiviral | Interferes with viral RNA synthesis and replication | 0.0002168 | 129, 130 |
Azithromycin | Antibiotic | Expresses antiviral and anti-inflammatory properties | 0.0002000 | 131 |
Tenofovir | Antiviral | Interferes with viral RNA synthesis to inhibit replication | 0.0001930 | 132 |
Acetaminophen | Analgesic | Expresses antipyretic and analgesic effects and inhibits the cyclooxygenase pathways | 0.0001665 | 133, 134 |
Dexamethasone | Corticosteroid | Suppresses immune response | 0.0001633 | 98 |
Methotrexate | Immunosuppressive drug | Suppresses immune response | 0.0001624 | |
Cyclosporine | Immunosuppressive drug | Express anti-inflammatory and antiviral properties | 0.0001614 | 135, 136 |
Cisplatin | Anticancer | 0.0001555 | ||
Tacrolimus | Immunosuppressive drug | Mitigates the hyperinflammatory response | 0.0001532 | 137 |
Indomethacin | Non-steroidal anti-inflammatory drug | Expresses anti-inflammatory properties and reduces pain and fever | 0.0001505 | 63, 138 |
Cannabidiol | Cannabinoid | Inhibits viral replication by upregulating the host inositol-requiring enzyme 1α ribonuclease endoplasmic reticulum stress response and interferon signaling pathways | 0.0001488 | 60 |
Doxorubicin | Anticancer | Expresses antiviral and immunomodulatory properties | 0.0001486 | 61 |
Diclofenac | Non-steroidal anti-inflammatory drug | Expresses anti-inflammatory properties and reduces pain and fever | 0.0001481 | 138 |
aThe references point to publications that have reported the drugs’ mechanism of action potentially linked with Covid-19.
bDrugs with direct contact to seed nodes as observed in first neighbors.
Covid-19, coronavirus disease 2019; Covid-19 KG, Covid-19 knowledge graph; DRKG, drug repurposing knowledge graph; IL6, interleukin 6; IL6R, interleukin 6 receptor; RWR, random walk with restart.

Graph representation of interactions between drugs and other features as observed from predicting candidate drugs using existing knowledge graphs and hypothesis-driven seeds. Blue edges represent interactions between drugs (green nodes). Cyan edges represent interactions between biological processes (pink nodes) and drugs. Red edges represent biological process–proteins (gray nodes) interactions and biological process–transcript (yellow nodes) interactions. Black edges represent drug–protein, drug–transcript, protein–transcript, and transcript–transcript interactions. The graphs were generated by defining filtering criteria based on the node degree between 4 and 1633 in Cytoscape.
Predicting Candidate Drugs Using Existing Knowledge Graphs, DSOGs, and Hypothesis-driven Seeds
To discover potential candidate drugs that could be used to specifically treat the disease in either its mild, moderate, or severe phases, we employed the RWR method in a network-based approach utilizing the knowledge graphs (DRKG and Covid-19 KG), alongside the DSOGs encompassing transcriptomics, proteomics, metabolomics, and lipidomics data (see Materials and Methods ). The outcome of the RWR analysis is a ranked list of potential drugs and a multi-layered graph describing the random walks from the seed nodes ( IL6 and IL6R). Across different analyses of the mild, moderate, and severe disease states, we consistently identified the same sets of drugs ( Table 4 ) that could potentially be impactful during Covid-19 treatment. Notably, our analysis revealed that drugs known to suppress the immune response and reduce inflammation, including those promoting IL6 production, consistently ranked high across all investigated disease states ( Table 4 ). This observation aligns with expectations, considering our choice of IL6 and IL6R as seeds: Both are pivotal biomarkers identified in multiple studies as displaying expression levels that are positively associated with severe disease states. 64, 65 These biomarkers are prominently expressed during cytokine storms that are characteristic of severe Covid-19 cases. 65
Top 20 Potential Drugs for Mild, Moderate, and Severe Covid-19, Ranked According to Their Measure of Proximity to IL6 and IL6R Seed Nodes as Determined Through the RWR Analysis of the Covid-19 KG, DRKG, and DSOG.
Drug | Drug Category | Mechanism of Action Potentially Linked with Covid-19 | Measure of Proximity in the Mild State | Measure of Proximity in the Moderate State | Measure of Proximity in the Severe State | Reference a |
---|---|---|---|---|---|---|
Dexamethasone | Corticosteroid | Suppresses immune response | 0.0033655 | 0.0033691 | 0.0033697 | 98 |
Sarilumab b | Anti-IL6R monoclonal antibody | Suppresses immune response by blocking IL6 signaling | 0.0031924 | 0.0031908 | 0.0031911 | 19 |
Tocilizumab b | Anti-IL6R monoclonal antibody | Suppresses immune response by blocking IL6 signaling | 0.0031908 | 0.0031894 | 0.0031897 | 21 |
Zinc | Essential mineral/nutrient | Expresses antiviral properties and interferes with viral RNA synthesis to inhibit replication | 0.0002730 | 0.0002681 | 0.0002692 | 123, 139 |
Sirolimus | Immunosuppressive drug | Expresses immunomodulatory and anti-inflammatory properties and inhibits the expression of pro-inflammatory cytokines | 0.0001524 | 0.0001526 | 0.0001530 | 124, 125 |
Histamine | Depressor amine | Expresses immunomodulatory and anti-inflammatory properties | 0.0001420 | 0.0001413 | 0.0001416 | 140, 141 |
Curcumin | Natural compound | Expresses immunomodulatory and anti-inflammatory properties that inhibit severe inflammation and cytokine storm | 0.0001366 | 0.0001358 | 0.0001363 | 142, 143 |
Cyclosporine | Immunosuppressive drug | Expresses anti-inflammatory and antiviral properties | 0.0001352 | 0.0001370 | 0.0001369 | 135, 136 |
Doxorubicin | Anticancer | Inhibits the protease-mediated viral entry to the host cell | 0.0001314 | 0.0001316 | 0.0001318 | 61 |
Morphine | Opioid pain medication | Contributes to improving respiratory failure | 0.0001301 | 0.0001281 | 0.0001284 | 144 |
Dactinomycin | Anticancer | Expresses immunomodulatory properties and inhibits viral cellular transcription | 0.0001292 | 0.0001290 | 0.0001288 | 79, 80 |
Simvastatin | Lipid-lowering drug | Expresses anti-inflammatory, immunomodulatory properties and reduces viral replication | 0.0001290 | 0.0001289 | 0.0001287 | 145, 146 |
Hydrocortisone | Corticosteroid | Expresses anti-inflammatory and immunomodulatory properties | 0.0001274 | 0.0001279 | 0.0001276 | 98 |
Vitamin C | Essential mineral/nutrient | Express antioxidant properties and improves immune function | 0.0001268 | 0.0001269 | 0.0001276 | 105 |
Mycophenolic acid | Immunosuppressive drug | Expresses immunomodulatory properties | 0.0001236 | 0.0001236 | 0.0001239 | 99 |
Methotrexate | Immunosuppressive drug | Expresses immunomodulatory properties | 0.0001182 | 0.0001195 | 0.0001194 | |
Dopamine | Catecholamine neurotransmitter | Influences the expression of ACE2 | 0.0001178 | 0.0001187 | 0.0001197 | 147 |
Indomethacin | Non-steroidal anti-inflammatory drug | Expresses anti-inflammatory properties and reduces pain and fever | 0.0001170 | 0.0001165 | 0.0001162 | 63 |
Paclitaxel | Anticancer | Express anti-inflammatory and antiviral properties | 0.0001159 | 0.0001159 | 0.0001161 | |
Metformin | Biguanide antihyperglycemic | Inhibits viral replication | 0.0001158 | 0.0001158 | 0.0001164 | 100 |
aThe references point to publications that have reported the drugs’ mechanism of action potentially linked with Covid-19.
bDrugs with direct contact to seed nodes as observed in first neighbors.
Covid-19, coronavirus disease 2019; Covid-19 KG, Covid-19 knowledge graph; DRKG, drug repurposing knowledge graph; DSOG, disease state-specific omics graph; IL6, interleukin 6; IL6R, interleukin 6 receptor; RWR, random walk with restart.
We observed differences between the multi-layered graphs generated by the RWR analysis on the mild, moderate, and severe disease states. Specifically, these differences were observed in the levels of connectivity between molecular features and the drug repurposing candidates. We therefore performed network topology analysis on the multi-layered graphs generated from the RWR analysis to explore the differences between these graphs and how that can provide more insights into the use of predicted drugs across mild, moderate, and severe disease states. First, we evaluated the degree, betweenness, and closeness measures of the drug repurposing candidates and observed relatively similar scores ( Table 5 ). The node degree indicates the number of connections a drug has, revealing its involvement in broader disease processes. Higher degrees might suggest broader applicability across multiple diseases. The betweenness centrality measures a drug’s “traffic control” role, indicating how often it lies on the shortest paths between other nodes. High betweenness suggests a potential “bridge” molecule connecting different disease pathways. The closeness centrality reflects how quickly information can reach other disease elements or nodes from a candidate drug. High closeness suggests a central position within the disease network, potentially making it a good starting point for treatment. For instance, cyclosporine had a higher degree of 6 in the multi-layered graph generated by the RWR analysis on the moderate disease state as compared to a degree of 5 in the multi-layered graph generated by the RWR analysis on the mild disease state and a degree of 4 in the multi-layered graph generated by the RWR analysis on the severe disease state with a corresponding high betweenness score of 0.0014 and a closeness score of 0.4832, suggesting it might have potential utility for the moderate disease state.
Node Degree, Betweenness, and Closeness Centrality Measures for the Drug Repurposing Candidates Predicted Using the Hypothesis-driven Approach.
Drug Name | Degree, Mild State | Degree, Moderate State | Degree, Severe State | Betweenness, Mild State | Betweenness, Moderate State | Betweenness, Severe State | Closeness, Mild State | Closeness, Moderate State | Closeness, Severe State |
---|---|---|---|---|---|---|---|---|---|
Dexamethasone | 1633 | 1632 | 1633 | 0.6282 | 0.5881 | 0.6293 | 0.6355 | 0.6233 | 0.6228 |
Tocilizumab | 830 | 829 | 830 | 0.11436 | 0.1008 | 0.1103 | 0.4643 | 0.4482 | 0.4571 |
Sarilumab | 469 | 469 | 469 | 0.0286 | 0.0246 | 0.0283 | 0.4432 | 0.4289 | 0.4373 |
Sirolimus | 7 | 8 | 6 | 0.0063 | 0.0068 | 0.0054 | 0.4831 | 0.4889 | 0.4512 |
Vitamin C | 6 | 6 | 4 | 0.0047 | 0.0034 | 0.0004 | 0.4860 | 0.4525 | 0.4460 |
Cyclosporine | 5 | 6 | 4 | 0.0007 | 0.0014 | 0.0004 | 0.4769 | 0.4832 | 0.4460 |
Dactinomycin | 5 | 4 | 4 | 0.0007 | 0.0003 | 0.0004 | 0.4769 | 0.4367 | 0.4460 |
Paclitaxel | 5 | 5 | 4 | 0.0007 | 0.0009 | 0.0004 | 0.4769 | 0.4739 | 0.4460 |
Simvastatin | 5 | 5 | 4 | 0.0007 | 0.0009 | 0.0004 | 0.4769 | 0.4739 | 0.4460 |
Hydrocortisone | 4 | 5 | 4 | 0.0004 | 0.0007 | 0.0004 | 0.4528 | 0.4454 | 0.4460 |
Zinc | 4 | 5 | 4 | 0.0005 | 0.0011 | 0.0005 | 0.3652 | 0.3903 | 0.3637 |
Indomethacin | 4 | 5 | 4 | 0.0004 | 0.0009 | 0.0004 | 0.4528 | 0.4739 | 0.4460 |
Mycophenolic acid | 4 | 5 | 4 | 0.0004 | 0.0009 | 0.0004 | 0.4528 | 0.4739 | 0.4460 |
Doxorubicin | 4 | 5 | 4 | 0.0004 | 0.0009 | 0.0004 | 0.4528 | 0.4739 | 0.4460 |
Methotrexate | 4 | 5 | 4 | 0.0004 | 0.0007 | 0.0004 | 0.4528 | 0.4454 | 0.4460 |
Morphine | 4 | 4 | 3 | 0.0005 | 0.0007 | 0.0003 | 0.4768 | 0.4738 | 0.4459 |
Curcumin | 3 | 4 | 3 | 0.0 | 0.0001 | 0.0004 | 0.4644 | 0.4716 | 0.4483 |
Metformin | 3 | 3 | 2 | 0.0 | 0.0 | 0.0 | 0.4644 | 0.4355 | 0.4354 |
Histamine | 3 | 3 | 1 | 0.0002 | 0.0 | 0.0 | 0.3866 | 0.3705 | 0.3601 |
Dopamine | 1 | 1 | 1 | 0.0 | 0.0 | 0.0 | 0.3615 | 0.3584 | 0.3601 |
Similarly, mycophenolic acid had a higher degree of 5 in the multi-layered graph generated by RWR for the moderate disease state as compared to a degree of 4 in both the multi-layered graph generated by the RWR analysis on the mild disease state and the multi-layered graph generated by the RWR analysis on the severe disease state with a corresponding high betweenness score of 0.009 and a closeness score of 0.4739, also suggesting it might have potential utility for the moderate disease state.
In Table 5 , the drugs were ranked according to the node degree (starting from the mild disease state) with the corresponding betweenness and closeness scores. There are variations in the betweenness and closeness scores. For instance, drugs with higher degree scores for specific disease states have high betweenness and closeness scores for that disease state. As shown in Table 5 , three drugs (dexamethasone, tocilizumab, and sarilumab) had betweenness scores between 0.024 and 0.64 in at least one disease state. The remaining had a betweenness score between 0.0 and 0.007 across all disease states. Additionally, all drugs in Table 5 had a closeness score between 0.3 and 0.63.
Top 20 Potential Drugs for Mild, Moderate, and Severe Covid-19, Ranked According to Their Measure of Proximity to STAT1, SOD2, 3-Hydroxyoctanoate, and Unknown_mz_815.61548_+_RT_27.063 Seed Nodes as Determined Through the RWR Analysis of the Covid-19 KG, DRKG, and DSOG.
Drug | Drug Category | Mechanism of Action Potentially Linked with Covid-19 | Measure of Proximity in the Mild State | Measure of Proximity in the Moderate State | Measure of Proximity in the Severe State | Reference a |
---|---|---|---|---|---|---|
Glutathione | Antioxidant | Protects cells from damage caused by oxidative stress | 0.0007061 | 0.0007044 | 0.0007044 | 104, 105 |
Crizotinib | Tyrosine kinase inhibitor | Expresses immunomodulatory properties and could inhibit receptor tyrosine kinases and affect cellular processes relevant to viral replication | 0.0007022 | 0.0007005 | 0.0007002 | 111 |
Curcumin | Natural compound | Expresses antiviral, immunomodulatory, and antioxidant properties that may contribute to inhibit inflammation and oxidative stress and reduce lung injury | 0.0006966 | 0.0006948 | 0.0006951 | 142 |
Vorinostat | Histone deacetylase inhibitor | Expresses anti-inflammatory and antiviral properties | 0.0006943 | 0.0006952 | 0.0006954 | 112 |
Vinblastine | Anticancer | Disrupts microtubule dynamics, leading to mitotic spindle dysfunction and cell cycle arrest | 0.0006920 | 0.0007029 | 0.0007022 | 148 |
Iron | Essential mineral/nutrient | Expresses anti-inflammatory and immunomodulatory properties | 0.0006917 | 0.0006899 | 0.0006944 | 149 |
Mebendazole | Antihelminthic | Expresses antiviral and immunomodulatory properties and may interfere with viral replication | 0.0006913 | 0.0006913 | 0.0006910 | 101 |
Podofilox | Topical agent | Stimulates the production of interferon-γ, a cytokine that plays a role in the immune response | 0.0006900 | 0.0007003 | 0.0007002 | 150 |
Valine | Essential amino acid | Protein synthesis | 0.0006893 | 0.0006891 | 0.0006891 | 151, 152 |
Acetylcysteine | Antioxidant and glutathione inducer | Expresses antioxidant, immunomodulatory, and mucolytic properties | 0.0006878 | 0.0006877 | 0.0006878 | 153 |
Thimerosal | Methyltransferase inhibitor | Induces Th2-type cytokines via influencing cytokine secretion by human dendritic cells | 0.0006826 | 0.0006824 | 0.0006824 | |
Fludarabine | Chemotherapy drug | Inhibits type I interferon-induced expression of ACE2 | 0.0006813 | 0.0006811 | 0.0006812 | 92 |
Calcipotriol | Anti-psoriatic | Enhances cell differentiation | 0.0006800 | 0.0006800 | 0.0006800 | |
Teniposide | Cytotoxic drug | Inhibits SARS-CoV-2 3-chymotrypsin-like cysteine protease | 0.0001087 | 0.0001091 | 0.0001096 | 154 |
Omacetaxine | Cephalotaxine ester and protein synthesis inhibitor | Inhibits protein translation and interferes with viral replication | 0.0001015 | 0.0000955 | 0.0001009 | 155 |
Etoposide | Topoisomerase II inhibitor | Express anti-inflammatory properties and suppresses cytokine production | 0.0001010 | 0.0000973 | 0.0001022 | 156, 157 |
L-glutamine | Amino acid | Expresses immunomodulatory, anti-inflammatory, and antioxidant properties | 0.0000945 | 0.0000943 | 0.0000942 | 158, 159 |
Carglumic acid | Analog of N-acetylglutamate | Expresses enzyme properties for processing excess nitrogen produced when the body metabolizes proteins | 0.0000942 | 0.0000939 | 0.0000939 | |
Pregabalin | Anticonvulsant drug | Reduces Covid-19-related pain and cough | 0.0000591 | 0.0000591 | 0.0000591 | 160 |
Threonine | Essential amino acid | Expresses immunomodulatory, anti-inflammatory, and antioxidant properties | 0.0000579 | 0.0000578 | 0.0000579 | 152 |
aThe references point to publications that have reported the drugs’ mechanism of action potentially linked with Covid-19.
Covid-19, coronavirus disease 2019; Covid-19 KG, Covid-19 knowledge graph; DRKG, drug repurposing knowledge graph; DSOG, disease state-specific omics graph; RWR, random walk with restart; SARS-CoV-2, severe acute respiratory syndrome coronavirus 2; SOD2, superoxide dismutase 2; STAT1, signal transducer and activator of transcription 1.
Next, we evaluated the contribution of other molecular features in the networks. To begin with, beyond the central influence of top-ranked drugs ( dexamethasone, tocilizumab, and sarilumab) in the multi-layered graph generated from the RWR analysis on the mild disease state ( Figure 3A ), three key inflammatory-related features, C-C motif chemokine ligand 2 ( CCL2), C-C motif chemokine ligand 4 ( CCL4), and negative elongation factor complex member C/D ( NELFCD), formed distinct subnetworks, acting as crucial hubs that connected seed nodes and promising candidates for drug repurposing. In mild Covid-19 cases, CCL2 helps recruit monocytes and macrophages, which are essential for fighting the virus as compared to excessive immune cell recruitment in severe disease states. 66, 67 Also, CCL4 levels in mild disease states help recruit necessary immune cells to fight the virus. 68 Thus, using drug repurposing candidates that could influence the recruitment of immune cells in mild disease states could be more appropriate, acknowledging the fact that the development of clinical Covid-19 involves cell activation such as dysfunctional mast cell activation. 69 From the predicted drugs, histamine is a biogenic amine known to attract and activate immune cells, particularly mast cells, basophils, neutrophils, and certain T cells, through specific histamine receptors. 70 Histamine can stimulate mast cell degranulation, leading to the release of CCL4 and CCL2 among other inflammatory mediators. 71, 72 This suggests a potential indirect link between histamine and these chemokines in inflammatory processes. Paclitaxel is known to modulate the immune system in various ways, including (i) promoting the migration of T cells and other immune cells into tumors, (ii) enhancing the activity of antigen-presenting cells, vital for activating T cells, and (iii) modulating the expression of immune-related genes that influence inflammation and immune responses. Paclitaxel induces the release of cytokines like tumor necrosis factor ( TNF) and IL6 and chemokines like CCL2 and might, therefore, help control viral infection by stimulating immune cell recruitment and boosting immune responses. 73 Metformin might enhance the activity of certain immune cells like macrophages and natural killer cells, potentially aiding in viral clearance. 74, 75 Metformin activates 5’ adenosine monophosphate-activated protein kinase (AMPK), a cellular energy sensor that regulates various metabolic and inflammatory processes. 76 AMPK activation can downregulate pro-inflammatory signaling pathways and reduce the production of inflammatory mediators like TNF and IL6. 77
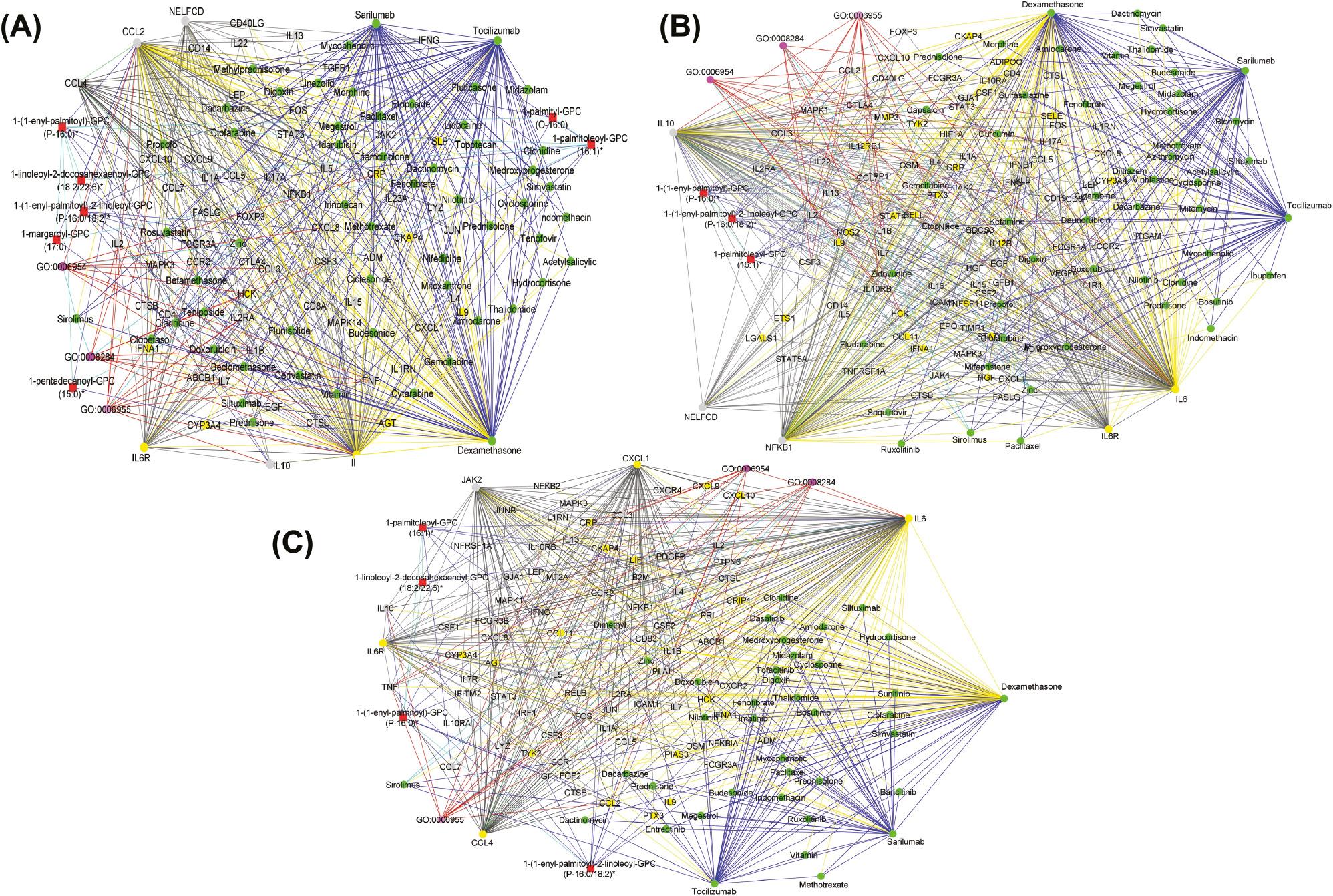
(A) Graph representation of the interaction between drugs (green nodes), proteins (yellow nodes), transcripts (gray nodes), metabolites (red nodes), and biological process (pink nodes) as observed from predicting candidate drugs using existing knowledge graphs, mild disease state-specific omics graphs (DSOGs), and hypothesis-driven seeds. The graph reveals distinct subnetworks formed by hubs CCL2, CCL4, and NELFCD demonstrating extensive interactions with drug candidates, seed nodes (IL6 and IL6R), and other molecular features. (B) Graph representation of the interaction between drugs (green nodes), proteins (yellow nodes), transcripts (gray nodes), metabolites (red nodes), and biological process (pink nodes) as observed from predicting candidate drugs using existing knowledge graphs, moderate DSOGs, and hypothesis-driven seeds. The graph reveals distinct subnetworks formed by hubs NFKB1, IL10, and NELFCD demonstrating extensive interactions with drug candidates, seed nodes (IL6 and IL6R), and other molecular features. (C) Graph representation of the interaction between drugs (green nodes), proteins (yellow nodes), transcripts (gray nodes), metabolites (red nodes), and biological process (pink nodes) as observed from predicting candidate drugs using existing knowledge graphs, severe DSOGs, and hypothesis-driven seeds. The graph reveals distinct subnetworks formed by hubs CXCL1, CCL4, and JAK2 demonstrating extensive interactions with drug candidates, seed nodes (IL6 and IL6R), and other molecular features. Yellow edges represent drug–protein and drug–transcript pairwise interactions. Red edges represent biological process–protein interactions and biological process–transcript interactions. Green edges represent protein–protein interactions. Black edges represent transcript–transcript interactions and protein–transcript interactions. Blue edges represent drug–drug interactions. Light blue edges represent biological process–biological process interactions and biological process–pathway interactions. The graphs were generated by defining filtering criteria based on the node degree between 4 and 1633 in Cytoscape. CCL2, C-C motif chemokine ligand 2; CXCL1, C-X-C motif chemokine ligand 1; IL6, interleukin 6; IL6R, interleukin 6 receptor; JAK2, Janus kinase 2; NFKB1, nuclear factor kappa B subunit 1; NELFCD, negative elongation factor complex member C/D.
The NELFCD, as part of NELF, regulates RNA polymerase II pausing, potentially influencing viral RNA synthesis during viral genome replication. 78 This suggests that drugs with the potential to inhibit SARS-CoV-2 replication could be appropriate for repurposing. Such drugs would include, for example, dactinomycin which, besides having immune-modulatory properties, also inhibits viral genome replication. 79, 80
Analysis of the multi-layered graph generated by the RWR analysis during predictions for the moderate disease state identified NELFCD, nuclear factor kappa B subunit 1 ( NFKB1), and IL10 as hubs influencing the network based on their high connectivity, forming both direct and indirect pathways between the seed nodes and the top candidates for drug repurposing ( Figure 3B ). NFKB1 activates genes encoding pro-inflammatory cytokines, chemokines, and adhesion molecules, orchestrating the body’s initial response to viral infection and enhancing the severity of Covid-19 symptoms. 81, 82 Thus, moderating NFKB1 activity could mitigate cytokine storms and improve outcomes. Corticosteroids like dexamethasone can be used in severe Covid-19 to suppress NFKB1 activity and reduce inflammation. It is, however, important to note that while dampening NFKB1 can be beneficial, completely suppressing it could impair the body’s ability to fight the virus. Thus, finding the right balance remains crucial. IL10 is a natural anti-inflammatory cytokine, acting as a brake on the immune response. It helps control excessive inflammation, as disease severity progresses, particularly in moderate disease states, to prevent tissue damage. However, overactive IL10 production in moderate Covid-19 cases can dampen the immune system’s ability to fight the virus, potentially prolonging the infection and allowing persistent viral replication. From our results ( Figure 3B ), drugs with the potential to modulate IL10 activity could be beneficial during moderate disease states to balance the suppression of excessive inflammation with optimal immune functioning. For instance, sirolimus inhibits the mammalian target of the rapamycin (mTOR) pathway, which can indirectly suppress IL10 production by limiting signal transducer and activator of transcription 3 ( STAT3) signaling. 83, 84 Also, it can dampen the activation of certain immune cells like T cells, which may indirectly decrease IL10 production. Additionally, sirolimus promotes the differentiation and expansion of regulatory T cells, a subset of T cells that naturally suppress inflammation and can promote IL10 production as part of their suppressive function. 85
In the multi-layered graph generated by the RWR analysis during predictions for the severe disease state ( Figure 3C ), we observed key inflammation-related features like C-X-C motif chemokine ligand 1 ( CXCL1), CCL4, and Janus kinase 2 ( JAK2) to establish subnetworks which included both direct and indirect interactions with the seed nodes and top-ranked drug candidates ( Figure 3C ). JAK2, a signaling molecule inside immune cells, expresses both inflammatory and anti-inflammatory effects during Covid-19. For its inflammatory role, JAK2 activates certain signaling pathways, including the JAK2/STAT3 pathway, that trigger inflammatory responses in the lungs, which may help fight viral infections. 86 For its anti-inflammatory role, JAK2 also activates pathways promoting tissue repair and regeneration. Thus, drug repurposing candidates with the potential to influence JAK2 signaling may represent an effective therapeutic strategy for controlling the disease. 87 For instance, IL6 binds to soluble and transmembrane IL6R and the resultant complex induces homodimerization of gp130, leading to activation of JAK2. 88 This suggests that sarilumab and tocilizumab targeting the IL6R indirectly activate JAK2 downstream by limiting the IL6-mediated signaling pathway. 88, 89 Sirolimus targeting an mTOR pathway connected to JAK2 can be appropriate. Increased levels of CXCL1 and CCL4 have been associated with severe disease and hyperinflammatory states, suggesting a potential role in Covid-19 disease progression. 67, 90 In general, the drug repurposing candidates ( Table 4 ) with anti-inflammatory activities, immunomodulatory activities, and viral replication inhibitory activities have the best potential to manage excessive inflammation and limit viral persistence during the severe disease state. We further observed from the shortest path analysis how these molecular features act as mediators connecting the drug repurposing candidates to the seed nodes across the mild, moderate, and severe disease states ( Supplementary File 4 ).
Predicting Candidate Drugs Using Existing Knowledge Graphs, DSOGs, and Data-driven Seeds
To investigate the prediction of drugs that might be differentially applicable to treating different Covid-19 disease states, we used a data-driven approach to identify seeds by computing an integrated node centrality metric score leveraging the node degree, closeness, betweenness, and eigenvector centrality metrics (see Materials and Methods and Supplementary Table 3 ).
In the transcriptomics layer, we identified STAT1 as a seed node. STAT1 is known to be involved in immune responses and antiviral activity 67 and is reported to be upregulated in mild and severe Covid-19 cases, with the phosphorylation of the gene associated with both the upregulation of ACE2 expression and the development of severe disease states. 91, 92 In the proteomic layer, superoxide dismutase 2 ( SOD2) was identified as a seed node. SOD2 is an essential antioxidant enzyme that protects cells from superoxide radical anions which are known to be significantly under-expressed in the plasma 93 and lung cells of severe Covid-19 patients. 94 In the metabolomics layer, 3-hydroxyoctanoate was identified as a seed node. This metabolite is generated during medium-chain fatty acid oxidation and serves as a marker for primary defects in beta-hydroxy fatty acid metabolism. It is also affiliated with essential pathways such as those responsible for macrophage activation and platelet aggregation, with increases in 3-hydroxyoctanoate concentrations being associated with asymptomatic Covid-19 infections. 95 In the lipidomics layer, we identified “unknown_mz_815.61548_+_RT_27.063,” an uncharacterized lipid associated with disease severity, as a seed node.
Using these seed nodes for the RWR analysis across the various disease states, we identified several potential drug repurposing candidates ( Table 6 ) including “natural compounds” (such as glutathione and curcumin) and inhibitors of signal transduction pathways of protein kinases and cell proliferation (tyrosine, histone deacetylase, and methyltransferase). The results revealed the same drug repurposing candidates across the various disease states ( Table 6 ). However, network topology analysis revealed differences between the multi-layered graphs generated by the RWR analysis ( Figure 4A–C ). Specifically, these differences were observed in the connectivity of the drug repurposing candidates with the corresponding node degree, betweenness centrality, and closeness centrality scores ( Table 7 ). This provides more insights into the most appropriate drug repurposing candidates for the different disease states. For instance, curcumin had a higher degree of 1076 in the multi-layered graph generated by the RWR analysis on the mild disease state as compared to a degree of 4 in the multi-layered graph generated by the RWR analysis on the moderate disease state and a degree of 2 in the multi-layered graphs generated by the RWR analysis on the severe disease state. Podofilox had a higher degree of 478 in the multi-layered graph generated by the RWR analysis on the severe disease state as compared to a degree of 2 for both multi-layered graphs generated by the RWR analysis on the mild and moderate disease states. Therefore, whereas curcumin is an appropriate drug repurposing candidate with potential utility during mild Covid-19, podofilox is an appropriate drug repurposing candidate with potential utility during severe Covid-19. Furthermore, vinblastine showed a high degree of 1905 in the multi-layered graph generated by the RWR analysis on the moderate disease state and a degree of 1378 in the multi-layered graph generated by the RWR analysis on the severe disease state as compared to a degree of 4 in the multi-layered graph from the RWR analysis on the mild disease state. This suggests that vinblastine might be most effective in tackling advanced disease stages. Also, crizotinib showed a high degree of 1919 in the multi-layered graph generated by the RWR analysis on the mild disease state and a degree of 1947 in the multi-layered graph generated by the RWR analysis on the moderate state as compared to a degree of 2 in the multi-layered graph from the RWR analysis on the severe disease state; this indicates that it might be most appropriate for treatment of the mild and moderate stages of Covid-19 ( Table 7 ). Similarly, glutathione showed a high degree of 212 in the multi-layered graph generated by the RWR analysis on the mild disease state and a degree of 234 in the multi-layered graph generated by the RWR analysis on the moderate disease state as compared to a degree of 118 in the multi-layered graph from the RWR analysis on the severe disease state; this suggests that it can be a promising drug repurposing candidate for the mild and moderate disease states. Noticeably, nodes with higher degree scores ( Table 7 ) have higher betweenness and closeness scores revealing how often these nodes lie on the shortest paths between other nodes in the network and mediate how quickly information can reach other disease-related features from a candidate drug ( Supplementary File 5 ). Such nodes have high relevance within biological systems and, besides their specific biological activities, might also facilitate communication and synergy between biological pathways, making them key targets for the management of the disease.
Node Degree, Betweenness, and Closeness Centrality Measures for the Drug Repurposing Candidates Predicted Using the Data-driven Approach.
Drug Name | Degree, Mild State | Degree, Moderate State | Degree, Severe State | Betweenness, Mild State | Betweenness, Moderate State | Betweenness, Severe State | Closeness, Mild State | Closeness, Moderate State | Closeness, Severe State |
---|---|---|---|---|---|---|---|---|---|
Crizotinib | 1919 | 1947 | 2 | 0.5147 | 0.4171 | 0.0025 | 0.5478 | 0.5297 | 0.3987 |
Curcumin | 1076 | 4 | 2 | 0.3281 | 0.0005 | 0.0 | 0.5389 | 0.4317 | 0.3983 |
Glutathione | 212 | 234 | 118 | 0.0397 | 0.0350 | 0.0439 | 0.3532 | 0.3611 | 0.3657 |
Vinblastine | 4 | 1905 | 1378 | 0.0009 | 0.4787 | 0.6440 | 0.4364 | 0.5639 | 0.5556 |
Acetylcysteine | 2 | 2 | 1 | 0.0 | 0.0 | 0.0 | 0.3106 | 0.3028 | 0.2743 |
Calcipotriol | 2 | 2 | 1 | 0.0 | 0.0 | 0.0 | 0.3178 | 0.3186 | 0.3370 |
Etoposide | 2 | 2 | 2 | 0.0 | 0.0 | 0.0001 | 0.3987 | 0.3993 | 0.3715 |
Fludarabine | 2 | 3 | 2 | 0.0 | 0.0 | 0.0 | 0.3178 | 0.3902 | 0.3983 |
Iron | 2 | 2 | 1 | 0.0 | 0.0 | 0.0 | 0.3106 | 0.3028 | 0.2743 |
Mebendazole | 2 | 2 | 1 | 0.0 | 0.0 | 0.0 | 0.3178 | 0.3186 | 0.3370 |
Omacetaxine | 2 | 2 | 1 | 0.0 | 0.0 | 0.0 | 0.2438 | 0.2466 | 0.2460 |
Podofilox | 2 | 2 | 478 | 0.0 | 0.0 | 0.1438 | 0.3178 | 0.31863 | 0.4092 |
Teniposide | 2 | 2 | 2 | 0.0 | 0.0 | 0.0001 | 0.3987 | 0.3993 | 0.3715 |
Thimerosal | 2 | 2 | 1 | 0.0 | 0.0 | 0.0 | 0.3106 | 0.3028 | 0.2743 |
Valine | 2 | 2 | 1 | 0.0 | 0.0 | 0.0 | 0.3106 | 0.3028 | 0.2743 |
Vorinostat | 2 | 4 | 2 | 0.0009 | 0.0005 | 0.0 | 0.4199 | 0.4317 | 0.3983 |
Carglumic acid | 1 | 1 | 1 | 0.0 | 0.0 | 0.0 | 0.2610 | 0.2653 | 0.2678 |
L-glutamine | 1 | 1 | 1 | 0.0 | 0.0 | 0.0 | 0.2610 | 0.2653 | 0.2678 |
Threonine | 1 | 2 | 1 | 0.0 | 0.0 | 0.0 | 0.3106 | 0.3028 | 0.2743 |
Pregabalin | 1 | 1 | 1 | 0.0 | 0.0 | 0.0 | 0.3539 | 0.3463 | 0.3463 |
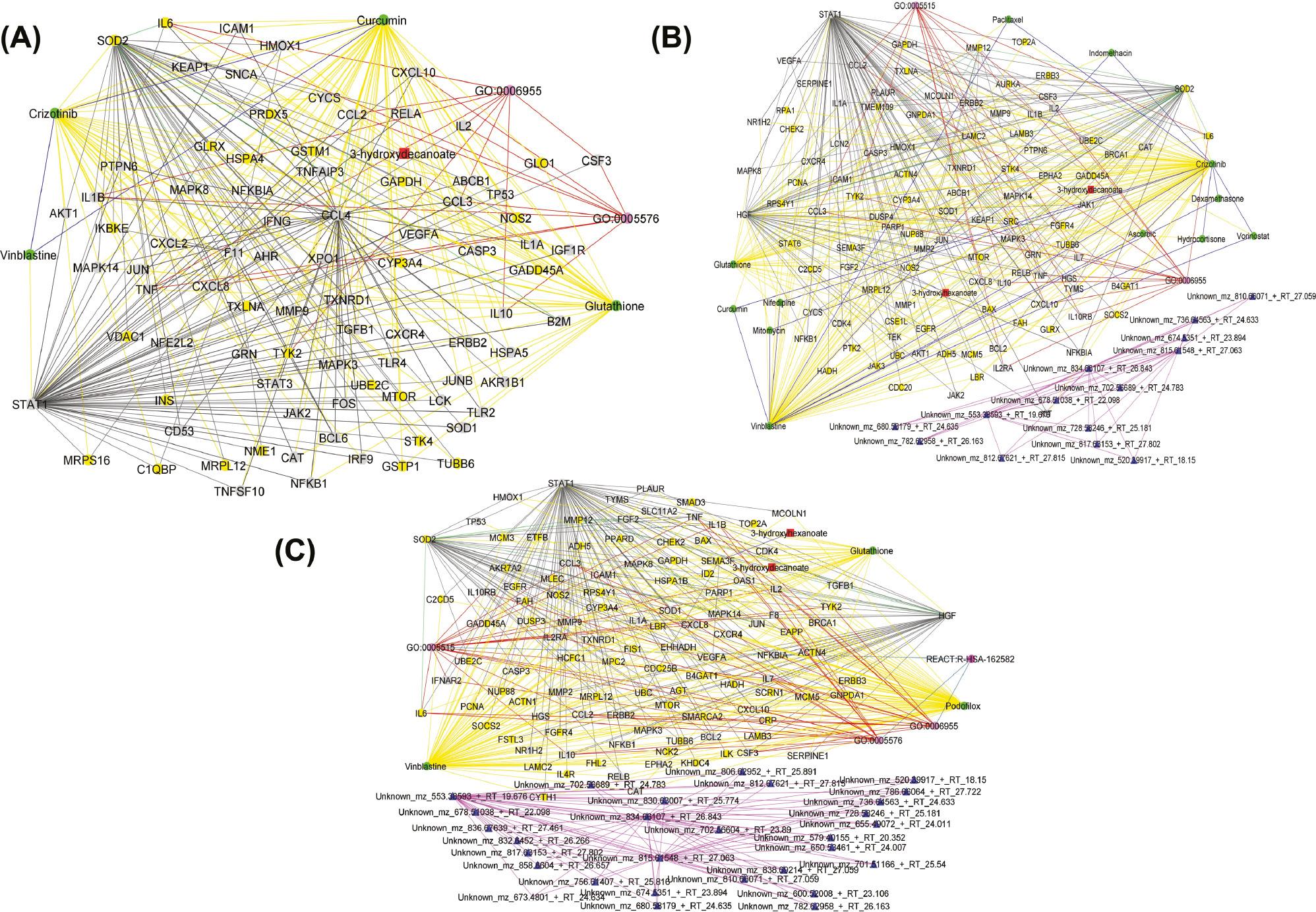
(A) Graph representation of the interaction between drugs (green nodes), proteins (yellow nodes), transcripts (gray nodes), metabolites (red nodes), lipids (blue nodes), and biological processes and pathways (pink nodes) as observed from predicting candidate drugs using existing knowledge graphs, mild disease state-specific omics graphs (DSOGs), and data-driven seeds. The graph reveals distinct subnetworks formed by hub CCL4, demonstrating extensive interactions with drug candidates and other molecular features including seed nodes (STAT1 and SOD2). (B) Graph representation of the interaction between drugs (green nodes), proteins (yellow nodes), transcripts (gray nodes), metabolites (red nodes), lipids (blue nodes), and biological processes and pathways (pink nodes) as observed from predicting candidate drugs using existing knowledge graphs, moderate DSOGs, and data-driven seeds. The graph reveals distinct subnetworks formed by the hub HGF, demonstrating extensive interactions with drug candidates and other molecular features including seed nodes (STAT1 and SOD2), as well as a subnetwork formed among lipids. (C) Graph representation of the interaction between drugs (green nodes), proteins (yellow nodes), transcripts (gray nodes), metabolites (red nodes), lipids (blue nodes), and biological processes and pathways (pink nodes) as observed from predicting candidate drugs using existing knowledge graphs, severe DSOGs, and data-driven seeds. The graph reveals distinct subnetworks formed by the hub HGF, demonstrating extensive interactions with drug candidates and other molecular features including seed nodes (STAT1 and SOD2), as well as a subnetwork formed among lipids. Yellow edges represent drug–protein and drug–transcript pairwise interactions. Red edges represent biological process–protein interactions and biological process–transcript interactions. Pink edges represent pairwise interactions between lipids. Green edges represent protein–protein interactions. Black edges represent transcript–transcript interactions and protein–transcript interactions. Dark blue edges represent drug–drug interactions. Light blue edges represent biological process–biological process interactions and biological process–pathway interactions. The graphs were generated by defining filtering criteria based on the node degree between 4 and 1633 in Cytoscape. CCL4, C-C motif chemokine ligand 4; HGF, hepatocyte growth factor; SOD2, superoxide dismutase 2; STAT1, signal transducer and activator of transcription 1.
Analysis of the multi-layered graphs generated by the RWR analysis revealed several features that establish subnetworks ( Figure 4 ). Specifically, we observed the CCL4 to establish subnetwork in the mild disease state and hepatocyte growth factor ( HGF) to establish subnetworks in both the moderate and severe disease states. HGF expresses anti-inflammatory properties and plays a complex and multifaceted role in the battle against Covid-19. 96 While it initially acts as a crucial player in lung tissue repair following viral damage, its activity can also contribute to excessive inflammation if not properly regulated. 96 HGF can activate certain signaling pathways that promote inflammation in moderate to severe cases. On the other hand, upregulation of HGF represents a robust counter-regulatory mechanism employed by the host immune response to counteract pro-inflammatory cytokines.
Drug Prediction Robustness Analysis
The three highest-ranked candidate drugs yielded by both the hypothesis-driven approach ( dexamethasone, sarilumab, and tocilizumab) and the data-driven approach ( glutathione, crizotinib, and curcumin) are all known to be efficacious in controlling moderate to severe Covid-19. However, the high rankings of these drugs (based on the measures of proximity) are expected simply because their efficacy during moderate to severe Covid-19 treatment has been comprehensively reported in the literature up to 2021. These reports are reflected in the Covid-19 KG layers of our networks.
In this section, we removed direct interactions between the top three predicted potential drug candidates identified using the hypothesis-driven and data-driven approaches and other features (such as drugs, proteins, and transcripts) to assess the influence of these interactions and features on the drug predictions. We assessed the robustness of the drug predictions in both the hypothesis-driven and data-driven approaches by repeating the RWR analysis (as described in the Materials and Methods section) after individually and collectively removing direct interactions associated with the three highest-ranked candidate drugs and examining changes in the drug rankings.
These analyses yielded a relatively consistent trend ( Supplementary Files 1 and 2 ): Drugs such as sirolimus, histamine, cyclosporine, and vorinostat that initially ranked below the drugs that were removed tended to achieve higher rankings following the removal of the initial top-ranked drug candidates. The measure of proximity of the drugs that attained elevated rankings varied from one drug removal experiment to the next, but generally increased relative to the measure of proximity that the drugs obtained in the absence of any exclusions ( Supplementary Files 1 and 2 ).
Furthermore, the drug removal analyses revealed additional candidate drugs that were not apparent in the absence of drug removal. For instance, with the hypothesis-driven approach, when we removed dexamethasone and tocilizumab simultaneously for each disease state, drugs like dinoprostone and perhexiline emerged among the top drug candidates across the mild, moderate, and severe disease states ( Supplementary File 1 ). Similarly, when we removed sarilumab, we observed ketamine, acetylsalicylic acid (aspirin), and menadione in the top 20. Concurring with the individual drug removal, when dexamethasone, tocilizumab, and sarilumab were collectively removed, we observed that dinoprostone, perhexiline, menadione, iron, and ketamine all entered the top 20 for the disease states.
When we excluded the top-ranked drug candidates that were revealed by the data-driven approach ( glutathione, crizotinib, and curcumin), we observed similar ranking score changes to those seen with the drug candidates identified by the hypothesis-driven approach. For example, when glutathione was removed, the measure of proximity of both L-glutamine and carglumic acid dropped substantially (from 0.0000945 and 0.0000942 to 0.0000022 and 0.0000594, respectively) resulting in lower rankings, whereas thimerosal disappeared completely ( Supplementary File 2 ). This observation could be partly because (i) thimerosal interacted with nodes that established connections with glutathione and crizotinib and (2) thimerosal established direct interactions with glutathione and crizotinib.
When glutathione, crizotinib, and curcumin were collectively removed, several notable drug candidates surfaced among the top hits, including penicillamine, pregabalin, dexamethasone, midostaurin, and treprostinil ( Supplementary File 2 ). Similar to when crizotinib and curcumin were individually removed, the measure of proximity of L-glutamine and carglumic acid increased from 0.0000945 and 0.0000942 to 0.0001621 and 0.0001629 for crizotinib removal and from 0.0000945 and 0.0000942 to 0.0001306 and 0.0001308 for curcumin removal, respectively ( Supplementary File 2 ).
In Silico Validation of Top Hit Candidate Drugs
To validate the Covid-19 drug predictions, we aimed to investigate how enriched the potential candidate drugs are as anti-Covid drugs in other databases. Specifically, we conducted RWR analyses using hypothesis-driven seeds on drug data extracted from DrugCombDB (version 2.0), a drug resource database. 97 We implemented these analyses to investigate whether we were able to predict known efficacious Covid-19 drugs ( Table 4 ). Among the top-ranked drugs ( Supplementary File 3 ) revealed by these analyses were dexamethasone (rank 1), simvastatin (rank 7), cyclosporine (rank 8), hydrocortisone (rank 9), paclitaxel (rank 11), indomethacin (rank 15), and methotrexate (rank 16).
DISCUSSION
In this work, we employed computational analyses for the prediction of drug repurposing candidates tailored for disease state-specific Covid-19 treatment. Leveraging a combination of knowledge graphs (DRKG and Covid-19 KG), along with Covid-19 DSOGs generated from experimental proteomics, transcriptomics, metabolomics, and lipidomics data, enabled the identification of various drug repurposing candidates that could potentially be usable as treatments for specific Covid-19 disease states. In our study, we recognize the importance of edge information in characterizing the interactions between nodes as described in Supplementary Tables 1 and 2 . Within the framework of the RWR algorithm, each layer is made up of interactions of specific edge types, such as drug–drug, drug–protein, and drug–transcript interactions, which can offer unique insights into the connectivity and relationships within the network, including the potential indirect associations between nodes and their impact on network dynamics. We implemented MultiXrank, a random walk algorithm capable of handling multiple multi-layered graphs and integrated drug data to predict candidate drugs for the mild, moderate, and severe Covid-19 disease states. The analysis resulted in the generation of multi-layered graphs that described the exploration of seed nodes across different DSOGs. One of the key advantages of the RWR analysis compared to the naïve first neighbor method is its ability to explore distant nodes in multi-layered graphs beyond just the immediate neighbors of a given seed node. The RWR analysis enables us to “restart” random walks from the seed nodes, traverse the network iteratively, and assign restart probabilities to nodes based on their likelihood of being visited. By incorporating restart probabilities and iteratively exploring the network based on random walks, RWR can uncover connections to nodes that may not be directly adjacent to the seed but are still given network connectivity and influence.
Although the hypothesis-driven and data-driven methodologies differed, the findings from both approaches have contributed to prioritizing potential drug repurposing candidates for mild, moderate, and severe Covid-19. We recommend considering both data- and hypothesis-driven approaches in studies utilizing multiple-source omics datasets. The hypothesis-driven approach revealed mostly drugs known to suppress the immune response and reduce inflammation, including those promoting IL6 production. In contrast, the data-driven approach revealed more diverse drugs including “natural compounds” (such as glutathione and curcumin) and inhibitors of signal transduction pathways of protein kinases and cell proliferation (tyrosine, histone deacetylase, and methyltransferase). The random walk analysis using both the hypothesis-driven and data-driven approaches yielded distinct multi-layered graphs ( Figures 3 and 4 ) characterized by different hubs and interactions with the candidate drugs, highlighting the unique perspectives offered by each method. With these differences, a consistent finding emerged from both approaches: Cross-layer interactions between omics features and drug repurposing candidates play a role in the dynamics of the drugs at the different disease states.
Some of the drugs identified through both hypothesis-driven and data-driven approaches are being, or have already been, tested in various clinical trials to assess their efficacy and effectiveness in the treatment of Covid-19. For instance, from the hypothesis-driven approach, corticosteroids such as dexamethasone and hydrocortisone have demonstrated an association with lower 28-day all-cause mortality in critically ill patients with Covid-19, 98 including mycophenolic acid, which has been investigated and validated to reduce mortality and hospital stays in patients with moderate to severe Covid-19, 99 indomethacin, which has been found in clinical trials to be safe and effective for treating mild and moderate Covid-19 cases, 63 and the diabetes medication metformin, which exhibits potential in reducing prolonged illness by inhibiting virus replication when administered during the acute phase of Covid-19. 100 From the data-driven approach, the antihelminthic drug mebendazole enhanced innate immune responses and restored inflammation to normal levels in symptomatic non-hospitalized Covid-19 patients during a recent clinical trial. 101 Also, etoposide has been investigated for its potential to treat severe disease, albeit with observed adverse events that warrant further investigation. 102
In general, immunosuppressive drugs might have a beneficial effect in the moderate to severe phase of Covid-19 because it is in this phase dysregulated pro-inflammatory immune responses can precipitate tissue damage and result in acute respiratory distress syndrome, organ failure, and mortality. 103 On the other hand, drugs predicted using the data-driven approach either had antioxidant properties (such as glutathione, and curcumin) or were inhibitors of tyrosine kinase, histone deacetylase, methyltransferase, and protein synthesis ( Table 7 ). The antioxidants can protect immune system cells and those directly targeted by SARS-CoV-2 from oxidative stress. For example, glutathione is an antioxidant assumed to have a vital role in maintaining the balance of reactive oxygen species (ROS) and aids in diverse cellular processes including immune responses. 104, 105 Notably, oxidative stress reflects an imbalance between ROS generation and antioxidation mechanisms 106 and plays an important role in Covid-19 onset, progression, and severity, 107– 109 possibly by exacerbating inflammation and tissue damage. 110 This, therefore, suggests that glutathione’s capability to counteract ROS and diminish oxidative stress holds promise for mitigating some of the adverse effects inflicted by the virus. 104 Glutathione and SOD2 bring unique strengths such that their combined efforts provide a multi-layered defense against oxidative stress and its harmful consequences. By neutralizing superoxide radicals, SOD2 sets the stage for glutathione to efficiently handle other free radicals and detoxify the cell. Also, given the aggressive inflammatory response and the production of cytokines occurring during severe Covid-19 disease states, some known inhibitors of receptor tyrosine kinases and cell proliferation, such as crizotinib and vorinostat, have been investigated as Covid-19 treatments. 111, 112 For instance, a recent study has shown that histone deacetylase inhibitors modulate immune responses in stimulated monocytes, 112 whereas tyrosine kinase inhibitors have the potential to reverse pulmonary insufficiency because of their anti-inflammatory activities, cytokine suppression activities, or antifibrotic activities. 111
Overall, the prioritized drug repurposing candidates ( Tables 3, 4, and 7 ) exhibit the potential to target a multitude of specific biological pathways and gene ontology processes that are associated with Covid-19 outcomes. Among these candidates are those that have shown promise in treating other diseases or conditions such as cancer, malaria, viral infections, and obstructive pulmonary disease. For instance, glutathione shows activity against HIV, influenza A, and hepatitis C by inhibiting viral replication and modulating immune response. 113 Curcumin shows activity against HIV, influenza A, hepatitis C, and dengue virus by inhibiting viral entry and replication. 114 Vorinostat shows activity against HIV by inhibiting viral replication and disrupting HIV-1 latency in patients on antiretroviral therapy. 115 Consequently, there is the possibility of repurposing these drugs to combat Covid-19 and other virus-induced conditions.
The approach implemented in this study is relevant to identifying drugs that warrant further exploration. Our methodology leading to the findings has been controlled through leveraging a data-driven approach complemented by a hypothesis-driven approach to minimize bias and ensure the robustness of findings effectively. However, validation procedures on interactome datasets such as (i) training a model to predict with which targets a previously unseen drug will interact and (ii) finding new putative interactions between drugs and other omics data types already in the datasets, can be implemented to reduce bias. 116 It is important to mention that some of the drugs that were highly ranked in our hypothesis-driven and data-driven analyses as potential Covid-19 treatments have not, to our knowledge, been tested before in the context of Covid-19 treatment. These included podofilox, calcipotriol, vinblastine, etoposide, and carglumic acid identified from the data-driven approach and paclitaxel identified from the hypothesis-driven approach. The shortest path analysis revealed molecular features that were close to the drugs ( Supplementary Files 4 and 5 ). Considering the drugs generated from the data-driven approach, podofilox, for example, inhibits topoisomerase I by stabilizing the covalent complex formed between the enzyme and a broken DNA strand. 117 This prevents religation, causing DNA damage and eventually cell death. 117 Podofilox is known to downregulate SOD2 expression in cancer cells and indirectly modulate SOD2 activity, impacting ROS levels and influencing cell survival and death. The ROS impact on Covid-19 progression 107– 109 suggests that podofilox may have a potential role in Covid-19 treatment. Also, etoposide possesses an immunosuppressive effect. While suppressing certain immune cells, etoposide may also selectively eliminate abnormal or activated T cells involved in the inflammatory process. 118 This can be beneficial in some inflammatory conditions, potentially mitigating immune-mediated damage. Additionally, etoposide has the potential to influence the production of certain cytokines and signaling molecules involved in immune communication. 119 In the context of Covid-19, this could have both pro-inflammatory and anti-inflammatory effects. Vinblastine can modulate the production of certain cytokines, signaling molecules that orchestrate immune responses. 120
Furthermore, the analysis conducted indicated that ritonavir, an HIV protease inhibitor utilized in combination with nirmatrelvir in the potent COVID-19 treatment paxlovid, was assigned a lower ranking in our data-driven analysis. This may be attributed to the characteristics of the exploited knowledge graphs that contained limited information about the impacts of ritonavir on the transcriptomics, proteomics, lipidomics, and metabolomics of human cells. As a result, the topology of the networks that we used was biased in favor of ranking better-researched compounds like dexamethasone and tocilizumab. In our analysis, we did not identify nirmatrelvir among the ranked drug candidates either. This observation is partly attributed to the choice of seeds for the RWR analyses and also to the fact we focus here on the omics networks from the host because nirmatrelvir targets the viral genome (polyprotein 1ab) and could, therefore, not be captured by the network exploration.
This analysis, drawing on diverse datasets, has provided valuable insights that contribute to ongoing efforts to combat endemic Covid-19 and the long-term health consequences of repeated SARS-CoV-2 infections. While some of the identified drugs have been implemented in disease management, several promising candidates are yet to be investigated for Covid-19 disease treatment and require experimental validation which is beyond the scope of this study. However, the predictions provide a starting point for further experimental validation and clinical investigations. Ensuring the safety and efficacy of new Covid-19 drugs requires rigorous experimental and clinical testing and validation. In vitro analyses and clinical trials must be conducted to determine the cytotoxicity, optimal dosages, administration protocols, and potential interactions with other medications. These experiments would ultimately be needed to provide actual proof that many of the less well-studied drug repurposing candidates that we have identified could indeed be used to effectively treat Covid-19. Importantly, the algorithmic framework implemented in this study can be translated to other diseases to investigate relevant drug repurposing candidates and to explore the dynamics among drugs and multi-omics features in a multi-layered network. We recommend considering both data- and hypothesis-driven approaches in studies utilizing multiple-source omics datasets.
LIMITATIONS
Considering the limitations of the DRKG and Covid-19 KG data, which predate large-scale drug evaluations, incorporating more recent drug information is crucial for future studies. While this study identified potential drugs for acute Covid-19 treatment, it did not address long Covid or the impact of comorbidities and disease severity. Thus, future investigations should explicitly explore treatment options for long Covid Furthermore, our drug prediction analysis did not account for Covid-19 comorbidities and recommends further studies to refine drug prediction analysis specific for mild, moderate, or severe Covid-19-infected patients experiencing other infections. Additionally, we acknowledge that incorporating information about pharmacokinetics, pharmacodynamics, and drug effects such as activator, or inhibitor, status or details on drug synergy could provide a more comprehensive understanding of the therapeutic implications. To maximize the potential of our approach, future work should incorporate pharmacokinetics, pharmacodynamics, drug effect information, and drug synergy analysis. By systematically evaluating how the therapeutic activities of different drugs might combine, we can identify and prioritize the most promising combination therapies for further testing and development in the fight against Covid-19. While we have not used dynamic networks in this study, we compared static networks in the mild, moderate, and severe phases of Covid-19 and drew conclusions on the drugs that may be effective in each of these phases. We did not account for temporal changes such as the dynamic nature of biological interactions in the network. We acknowledge this limitation and recognize the implications it may have on the relevance of the results, especially in scenarios where temporal dynamics may play a critical role. We recommend future studies to incorporate temporal changes and time-dependent processes, as well as methods to explore time series data and time-dependent processes. 121, 122
CONCLUSION
This study explored an integrative multi-layered network approach to identify drugs for repurposing against Covid-19 disease phases. We analyzed multi-omics data (proteomics, transcriptomics, metabolomics, and lipidomics) and drug-related data (DRKG and Covid-19 KG) using the RWR technique. Notably, we conducted RWR analyses in both hypothesis-driven and data-driven manners, incorporating information specific to disease severity levels (mild, moderate, severe) via dedicated DSOGs. Our multi-layered network approach successfully identified potential drug candidates for repurposing against mild, moderate, and severe Covid-19. The incorporation of disease state-specific omics data significantly influenced the predicted drug candidates. Both immunosuppressive and pathway-targeting mechanisms emerged as potential approaches for Covid-19 treatment.