INTRODUCTION
Precision diagnostics is a prerequisite for precision medicine by enabling a characterization of diseases on a molecular level leading to a mechanism-based treatment. Giving the right drug to the right patient at the right dose and at the right time requires novel biomarker signatures to stratify patients accordingly.
Diagnostics play an essential role in the context of drug repurposing, where existing drugs are investigated and repurposed for a new therapeutic use. They are important to identify the eligible patient population for a repurposed drug. Diagnostics are extremely valuable in determining which patients are most likely to benefit from a specific treatment. This way the effectiveness of a drug or combination of drugs is significantly increased and the chance of potential adverse effects is reduced. The validation of a diagnostic test is essential to demonstrate that it is suitable for its intended use. Validation data must be provided along the possible physiological concentrations, particularly close to the limit of detection as well as on specimen type and stability. The use will be limited to the described patient populations (e.g., age, sex, ethnicities) and clinical indications (e.g., subtype of disease, symptomatic vs asymptomatic). In parallel testing, if two or more tests are applied to a patient at the same time, it is important to predefine under which conditions the patient is considered to be positive and eligible for a specific therapeutic intervention. In addition to precise diagnostics in the wet lab, both the patient recruitment and avoidance of adverse events can currently be supported by artificial intelligence (AI) based on empirical datasets. 1, 2 The validation of AI algorithms in case of clinical decision-making is important as they can potentially impact patient selection. Here, EU laws and regulation that are currently constructed (in 2024) will give further guidance with emphasis of human intervention and data interpretation. Adequate standards are still in preparation, so using cohorts from public databases is the current option for validation.
Different kinds of omics technologies are used to identify new diagnostic, predictive, prognostic, and monitoring biomarkers to support innovative clinical trials as well as precision medicine. The convergence of multi-omics and AI-based technologies are currently supporting methodological innovations in drug repurposing. 3 The identification of the underlying disease mechanism will lead us to new disease definitions and options for causal treatments. Mechanism-based drug repurposing will revolutionize the way we approach drugs. It will help us find new uses for already registered drugs, increase precision, and radically cut down on costs and time in the drug development process ( https://repo4.eu/).
METHODS
In the past decades we have seen that oncology as a field is no longer solely focusing on the origin of each individual cancer by organ. In cases like breast cancer categorization into luminal A, luminal B, HER2-enriched, and triple-negative breast cancer (TNBC), a change of standard in treatment was tangible 4 and in colorectal cancer (CRC) the initial subtyping led to new therapy options for a subset of patients. More and more genomics and transcriptomics are becoming an integral part of initial patient stratification. For CRC, the consensus molecular subtypes originated from gene expression data and led to subtypes that each have different options to tackle their unique molecular pattern as shown in Table 1 . 5
Consensus Molecular Subtypes (CMS) in CRC According to Guinney et al. 5 .
CMS1 MSI Immune | CMS2 Canonical | CMS3 Metabolic | CMS4 Mesenchymal |
---|---|---|---|
14% | 37% | 13% | 23% |
MSI, CIMP high, hypermutation | SCNA high | Mixed MSI status, SCNA low, CIMP low | SCNA high |
BRAF mutations | KRAS mutations | ||
Immune infiltration and activation | WNT and MYC activation | Metabolic deregulation | Stromal infiltration, TGFß activation, angiogenesis |
Worse survival after relapse | Worse relapse-free and overall survival |
CRC, colorectal cancer.
Mixing mutational data with gene expression, the different signatures allowed the more targeted treatment of patients. That said, each of the subtypes still represents a large variability of molecular patterns as not all criteria have to be met to fall under a given category. This still allows some patients to be treated with a medication that is not in line with their molecular makeup. Therefore, the trend of going even further into subtyping has gotten a lot of traction as seen, for example, in the quite diverse subgroup of TNBC. 6 Here, the standard treatments as in other breast cancer types seem to fail. The FUTURE trial (ClinicalTrials.gov identifier: NCT03805399) used four subtypes of TNBC to find treatment options for those women in dire need of new medications. While the drugs used in the study were for the most part classical oncological drugs, their combination and usage were different from the initial setting in breast cancer. The usage of immunohistochemistry and a focused panel for genetic sequencing via next-generation sequencing (NGS) led to an efficient patient stratification and improved the overall outcome.
All techniques while being used in a clinical setting have to undergo standard procedures to ensure validity in each case. This means that all assays have to be properly validated with batch-to-batch control (minimum of three different batches) and ensuring analyte stability in terms of chemical (especially in case of e.g., RNA) and populational (is the biomarker still valid in a different ethnic background). Additionally, single-patient testing might be feasible, but scalability is vital to ensure usage of these methods in a larger population. As drug repurposing might be only one question for the treating physician, using other tests in parallel has to be possible. This will allow to find the suitable options for patient treatment and impact greatly on their health.
Having all of these in mind, the next logical step is to look past the standard oncological options and enrich the therapy option for each subtype by employing drug repurposing. This allows not only the use of drugs with a known and for the most part lower adverse effects spectrum, but also, by thinking outside the organ subtype confined space, the envisioning of repurposing for multiple different subtypes originating from anywhere in the body that share to some part the molecular pattern addressed by these compounds. The combination of mutational analysis and gene expression has been proven efficient to enable mechanistic assumptions. Whereas in the past a subtype was characterized by a few features, the use of NGS provides more options by its sheer scale. Tools like DNAS 7 allow pan-cancer subtyping while others like NeDRex 8 help to find mechanistically solid drug repurposing options.
In addition, molecular patterns can be used to identify novel compound effect relations like via MNBDR 9 that are based more on modules and the mode of action regardless of being a primary or even tertiary target. The efficacy is more likely to rise by the combination of drugs that might not have been in clinical routine due to the current indication for the individual drug. 10
The discovery of novel protein biomarkers requires several components such as highly sensitive analysis platforms, advanced data analysis, machine learning (ML) approaches to identify the best signatures, and a scientifically driven team to enable a sound analysis while generating data about the disease and its potential mechanism.
New biomarker signatures can be derived from various sample sources such as plasma/serum, spinal fluid, or cells such as peripheral blood mononuclear cell (PBMCs) or other immune cells. High-throughput analyses of proteins and post-translational modifications using immuno-based methods can speed up the discovery and validation of potential biomarker candidates while limiting the attrition rate of candidates and enabling fast translation of findings into other immunoassays.
The scioDiscover platform is an ideal example for such a biomarker discovery platform as it combines antibody-based profiling with high-sensitivity, high-throughput, very low sample requirements: e.g., 5 μl of plasma/serum or spinal fluid per sample and high reproducibility. This platform was used in two of Sciomics’ biomarker projects, one for prediction and early diagnosis of acute kidney injury (AKI) and the other for predicting a severe Covid-19 disease trajectory showing successfully that biomarker discovery and knowledge generation can be done at the same time.
The workflow established at Sciomics enables very fast discovery timelines e.g., less than 8 weeks from start of discovery to the patent filing for the Covid-19 project. 11 ML-assisted biomarker development and the subsequent validation of protein biomarker candidates using e.g., enzyme-linked immunosorbent assay (ELISA) assays are the next steps in the development process. Validated markers such as S100A8/A9 and C-reactive protein (CRP) show a very good correlation between scioDiscover-derived data and commercially available ELISA assays as well as clinical assays highlighting the ease of translation of results obtained with our discovery platform. The generalized procedure is depicted in Figure 1 .
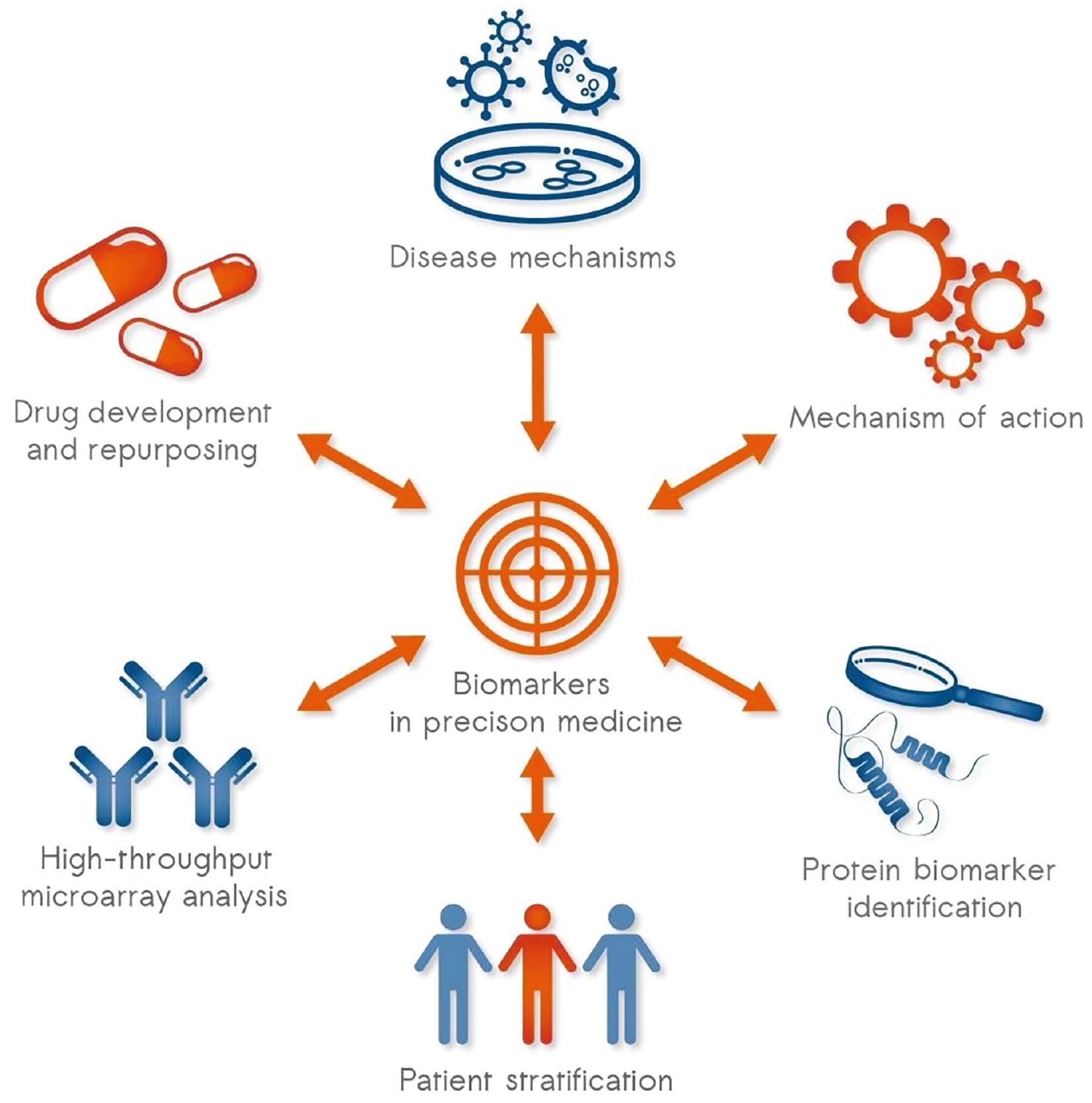
Biomarkers are a core element for precision medicine as these enable stratification, early diagnosis, and treatment response prediction; at the same time, protein analysis sheds light on disease mechanisms as well as drug mode-of-action mechanisms.
The AKI project is now the lead project for commercialization at Sciomics and a great example that demonstrates the power of protein analysis combined with ML-based data analysis. Two biomarker signatures were sought after, one for predicting the risk for an AKI after a severe surgery and the second for an early and time-point-independent diagnostic assay. Both signatures can improve the outcomes for patients as a timelier intervention is possible, can help to select patients for clinical trials using drugs that stabilize the kidney, 12 and can help to identify potential drugs for repurposing through identifying direct targets or yielding a deeper insight into the mechanism of the AKI development. This project is a great example to show the potential of biomarker signatures and drug development.
Next to yielding biomarker candidates, all discovery studies also add insights into the respective disease and the underlying mechanisms, and provide further information about the patient collective as more than 1400 proteins are profiled in a single assay. This approach is ideal to further precision medicine as novel biomarkers in combination with mechanistic knowledge and patient cohort characterization from a single analysis speed up research and knowledge generation. Furthermore, the discovery to the commercialization process of these biomarkers is further explored and optimized during the Repo4EU project to streamline the process for all future projects to bring novel precision diagnostic means to patients.
CHALLENGES AND OPPORTUNITIES WITH MULTI-OMICS INTEGRATION IN PRECISION MEDICINE
The potential of multi-omics data integration and analytics in precision medicine is far reaching, offering significant advancements such as improved diagnostic accuracy, minimized adverse effects, enhanced drug efficacy, and notable opportunities in drug repurposing. 13 The increasing availability of public data resources coupled with advancements in AI are pivotal in harnessing our collective knowledge and maximizing the utility of our data, heralding a new frontier in drug repurposing. However, the analysis of biological data often relies on proxy models that approximate true biological systems, introducing varying levels of bias and complicating the analysis process. Despite these challenges, each omics layer offers a unique view of biological processes. By integrating these diverse perspectives and gaining a deeper understanding of biomolecular mechanisms, alongside leveraging advanced analytic tools such as AI, we can significantly enhance the likelihood of successful drug repurposing.
The synergistic potential of AI and multi-omics lies chiefly in enhancing decision-making. This integration maximizes our existing knowledge, supported by data, to inform decisions with the highest probability of success. AI-driven patient stratification and identification of timely therapeutic opportunities enable more precise targeting. Multi-omics data/analytics, with its comprehensive biological insights, plays a crucial role in this process. By facilitating better early-stage evaluation and pinpointing potential points of failure, multi-omics contributes to the identification of novel, repurposed drugs, thereby potentially improving patient outcomes and enabling precision medicine. 14, 15
One of the primary challenges in multi-omics and (public) data analysis is the integration of diverse and complex datasets. The variability, complexity, and often incomplete nature of biological and molecular data present significant hurdles in applying AI effectively. In particular, issues like biased population samples in clinical cohorts and the impact of small changes in experimental procedures on molecular readings can greatly affect the results derived from AI applications. 16 Moreover, public data, while invaluable, are not uniform. Differences in experimental procedures, data annotations, and overall data quality across studies complicate effective data integration. The inconsistency in metadata annotations, ranging from experimental procedures to disease classifications, poses another major challenge. Therefore, thorough quality control and significant effort in data integration are essential to fully leverage AI in advancing drug discovery and research. 17
In order to drive forward progress in precision medicine in a way that is both data-driven and clinically relevant, we advocate for a synergistic approach that integrates both public and proprietary multi-omics data, AI-enabled analytics, and human-centered data exploration. User-friendly, interactive tools are essential in order to facilitate such a synergistic approach, and to include subject matter experts such as biologists or physicians, who may lack the data science skills necessary to extract insights from vast stores of multi-omics data. To address this challenge, BioLizard developed BioMx, a key component of the BioVerse data analytics and exploration ecosystem, which is designed to automate computational aspects of multi-omics data analysis while keeping experts in the loop for critical decision-making. BioMx offers a wide range of state-of-the-art methodologies for data analysis and exploration. Critically, this platform is also fully customizable, in recognition of the fact that different biological use cases will require tailored analytical solutions in order to best extract insights from the data. Furthermore, the BioVerse ecosystem ensures complete data transparency, traceability, management, and visualization, providing essential tools for informed, data-driven, and AI-supported decision-making.
In summary, AI presents significant new opportunities in extracting insights from complex multi-omics data to drive forward progress in patient stratification and personalized medicine. However, data visualization and exploration technologies must co-evolve to ensure that key stakeholders can effectively leverage these technological advancements to the ultimate benefit of patients. The greatest benefits will arise from the seamless intertwining of AI-driven data analytics with human-driven data interpretation, prioritization, and decision-making.
CONCLUSION
We have highlighted the need for stratifying patients into distinct mechanistic subgroups, or endotypes, for precision medicine and to improve the benefit of current pharmacological interventions. Endotypes are subsets within the patient population that share the same underlying disease mechanism that led to the condition. Particularly in complex diseases, this underlying mechanism manifests as a network with several compromised elements, which are more effectively addressed through a network pharmacology approach. 18 There is currently, however, a lack of precision biomarkers capable of accurately predicting mechanistic disease endotypes. This knowledge gap is a pivotal roadblock in precision medicine, leading to low precision pharmacological therapies and high frequency of failures in clinical trials.
Proteins, particularly those that are secreted, constitute a valuable reservoir of biomarkers easily accessible and quantifiable through blood that can provide precise information regarding the causal disease mechanism. 19 Blood and plasma protein biomarkers are considerably stable and can be quantified through mass spectrometry or antibody-based immunoassays, even in biobanked samples. The latter is easily translated into point-of-care settings and relatively inexpensive. 20 Moreover, recent developments in recombinant antibody technology facilitate the quick development of new antibody biomarkers for new protein targets or specific protein modifications. However, an exception is phosphorylation targets used as signaling read-outs, which typically require methods centered around cells, platelets, or exosomes. 21
Exploring and developing existing drugs for new indications require the development of diagnostic tests to identify patient populations that will benefit from the drug in its new therapeutic context. These diagnostics are instrumental in designing clinical trials for repurposed drugs. They help stratify patient populations for new innovative trials, ensuring that trial participants are more likely to benefit from the repurposed drug, as the underlying disease mechanism will be addressed. 22 Overall, this will significantly reduce time and costs within the drug development process.
The identification of diagnostic and/or predictive biomarkers reflecting the disease mechanism and the respective development of diagnostics with clinical utility for new indications of known drugs is a complex and interdisciplinary process. This process requires the collaboration between preclinical researchers, clinicians, bioinformaticians, statisticians, and regulatory experts. With genetics, transcriptomics, epigenomics, and multiplex proteomics being already powerful tools on their own, the integration of these diverse datasets and the knowledge of already stored patient data, for example, from electronic health records and information on comorbidities will lead to the next level in precise diagnostics enabling the best personalized treatments. Diagnostics and their associated biomarkers ensure that repurposed drugs are used effectively and safely for new indications, ultimately improving patient care and advancing medical science.