Introduction
The success of a new product or service depends on the existence of market demand. As a result, innovators put a great deal of effort into predicting and assessing customer needs and desires (e.g., Adams et al., 1998). Academics have long been interested in how innovators might best make such assessments, which requires navigating inherent uncertainties and ambiguities about what customers will want and pay for (Daft and Lengel, 1986). A variety of practitioner-developed methodologies that claim to help innovators address these challenges have also emerged (e.g., Blank and Dorf, 2012; Ries, 2011; Brown, 2008). Yet, despite all this attention, new innovation failure rates remain persistently high, raising questions about the effectiveness of extant theories and methods in general, and across innovation contexts (Fragkandreas, 2017; Storvang et al., 2020).
This study builds on grounded theory about potential sources of, and solutions to, innovation failure through an investigation of five theoretically sampled innovation projects in a US-based educational technology company. The study was guided by one overarching question: How might would-be innovators best combine theory building and theory testing approaches to navigating market uncertainty? The findings illustrate the challenges and opportunities of effectively combining these approaches. The paper theorizes that the appropriate balance of inductive theory building and deductive theory testing – as well as the required methodological rigor with which innovators must approach their work – is heavily influenced by the multiplicity of the innovation context. Building on use of the term in stakeholder theory (e.g., Neville and Menguc, 2006; Hillebrand et al., 2015), multiplicity is defined as the overlapping of stakeholder types and interests in a given innovation context. As multiplicity increases, so does the possibility that some of the associated interests are in tension or conflict. The data presented suggest that multiplicity and the potential tensions it can create increase the value of rigorous, inductive theory building, while simultaneously increasing the potential risks and costs of poorly grounded theory testing.
The study makes three key contributions to our understanding of innovation. First, it extends our knowledge about the benefits of engaging with potential customers during innovation projects. The study offers new insights about what such engagement should look like. It also develops new theory about how the nature and extent of engagement should vary across innovation contexts and throughout the course of a given innovation project. Second, the study adds empirical credence to nascent concerns about the strong bias towards action – particularly in the form of testing and experimentation – emphasized by popular, practitioner-developed innovation methods and related academic theories (Felin et al., 2019; Gans et al., 2019). Although this study does not doubt that experimentation is highly valuable, it extends recent arguments that not all theories and hypotheses are created equal (Felin et al., 2019). My findings demonstrate that the origins of theories and hypotheses matter, particularly in contexts where multiplicity is high, and inadequate attention to theory development – and poorly grounded hypotheses – can lead to costly blind spots and path dependence. In such contexts, a bias towards testing-oriented action may increase the chances of a failed product launch. Rigorous, grounded theory building may help protect against such risks.
Third, the findings have potentially profound implications for the teaching and practice of innovation. The analysis suggests misunderstandings about, or misapplications of, social science research methodologies that (supposedly) underpin popular innovation techniques can critically hamper innovation efforts and outcomes. As a result, innovation practitioners should have a strong understanding of the logic, practice, complementarities, and requisite rigor of these research methodologies. In educational contexts (and particularly the business schools where much innovation-related teaching takes place), achieving this depth of understanding may require rigorous coursework focused on research design and methods, and perhaps greater integration and partnership with courses and colleagues in the sciences and social sciences. In organizational contexts, the findings seem to add theoretical and empirical justification for hiring research methodologists for innovation projects.
Theoretical background
Academics and practitioners have long recognized that understanding market needs is critical to developing economically successful products and services (e.g., Daft and Lengel, 1986). Yet, as demonstrated by persistently high innovation failure rates, developing such an understanding is difficult (Fragkandreas, 2017; Storvang et al., 2020). In part – as former Apple CEO Steve Jobs famously pointed out – this is because would-be customers may themselves be unable to predict if and how they will derive value from a novel solution (Isaacson, 2012).
Market uncertainty is further compounded as the number and type of customers and other stakeholders affected by an innovation increase (Neville and Menguc, 2006). In both business-to-consumer and business-to-business contexts, a given innovation often directly impacts multiple stakeholders. One common example is situations where an innovation has distinct end users and economic buyers with divergent interests. For example, when developing a new snack food for children, innovators must ensure not only that children will eat it, but also that their parents will purchase it. Yet children may prefer the taste of nutritionally bankrupt, sugary foods, whereas parents likely prefer to feed their children healthy, nutritionally rich foods. Similarly, when it comes to business software, frontline employees may care primarily about ease of use, whereas economic buyers, such as senior managers, may prioritize price and how well the solution integrates with the company’s existing technology. Recent research suggests that the challenge of navigating competing stakeholder interests may be becoming even more difficult as nonmarket stakeholders are increasingly able to influence an innovation’s success. For example, concerns about climate change have meant environmental advocacy groups increasingly influence organizations’ products and services, even if they themselves are not end users or economic buyers (Driessen and Hillebrand, 2013).
In both academic and practitioner domains, approaches to understanding and navigating market uncertainty coalesce around two broad strategies. The first focuses on theory building, or efforts to increase the accuracy of theories and hypotheses about what products will be well-received by the market. 1 This strategy is broadly consistent with what entrepreneurship scholars refer to as a prediction or causation logic, as well as opportunity discovery (Sarasvathy, 2001; Alvarez and Barney, 2007). 2 In part based on academic research (e.g., Lange et al., 2007; Liedtka, 2015), techniques associated with this strategy have evolved over time. Traditionally, the main focus was on prediction and planning. For example, innovators might estimate the extent of market demand via market surveys and use these data to develop detailed predictions – often including detailed, formal business plans – about how the opportunity might be exploited (Sarasvathy, 2001; Lange et al., 2007). In recent years, there has been a shift away from such largely quantitative, planning-focused efforts towards more qualitative, inductive, and ethnographic techniques that focus on identifying and understanding unmet, and often latent, needs and desires (e.g., Liedtka, 2015). These inductive techniques claim to draw upon established research methodologies that use a grounded (i.e., data-driven) approach to building theory (Corbin and Strauss, 2014). Like such methodologies, these practitioner techniques attempt to generate better-informed, more accurate theories and hypotheses about what products and services will be commercially successful by developing a deep, empathic understanding of customers’ lives (Brown, 2008).
The second strategy focuses on navigating market uncertainty and ambiguity through direct testing and experimentation, or what is referred to ‘theory testing’. Rather than attempting to predict the products that customers will want, the essence of this strategy is making potential solutions available to customers and allowing them to vote with their wallets. Theory testing is broadly consistent with academic theories of effectuation, opportunity creation, trial and error, and experimental learning (Sarasvathy, 2001; Thomke, 2003; Brettel et al., 2012). In the practitioner realm, it has become instantiated in popular books (e.g., Osterwalder and Pigneur, 2010; Ries, 2011; Blank and Dorf, 2012) as well as in various practitioner-focused articles (e.g., Eisenmann et al., 2012; Blank, 2013). Drawing on the logic that underpins experiment-based academic research designs, this approach advocates that intentional, well-designed experiments are the best way to understand cause and effect, and provide the most direct and convincing evidence about the nature and extent of market demand (Camuffo et al., 2019). If we consider the underlying research methodologies upon which they are based, it seems that theory building and theory testing strategies should be most effective when used in combination (Klenner et al., 2022). For example, evidence-based forms of theory building should generate better, more accurate theories and hypotheses, which should translate into fewer, better targeted and designed experiments (Corbin and Strauss, 2014). Similarly, theory testing may precipitate new questions that can be explored inductively. For example, unexpected, null or negative experimental results might raise questions about why a theory or hypothesis was not supported. Answering these questions may lead to new and more appropriate hypotheses and, ultimately, better innovation outcomes.
To some extent, practitioners and academics have recognized that combining theory building and theory testing might lead to better innovation outcomes (Klenner et al., 2022). For example, in the practitioner sphere, popular versions of design-thinking encourage innovators to combine ethnographic techniques intended to identify unmet customer needs with direct testing of prototypes (e.g., Brown, 2008). Similarly, some academic research has demonstrated that innovators frequently combine the two strategies in various ways throughout the course of an innovation project (e.g., Bingham and Davis, 2012; Reymen et al., 2015; Eisenhardt and Bingham, 2017; Ott et al., 2017; Ott and Eisenhardt, 2020).
Despite expectations that theory building and theory testing might be best used in combination, the two strategies are often presented as competing alternatives (Sarasvathy, 2001). Moreover, even when complementarities are recognized, few recommendations are provided about how those complementarities might best be achieved, or how this might vary across innovation contexts or projects. For example, although design-thinking methodologists advocate combining inductive theory building with deductive theory testing, they are at best vague about how new product developers should switch between them, or how the nature and timing of these shifts might impact innovation outcomes (Brown, 2008; Liedtka, 2011). Similarly, although academic research has demonstrated that innovators often do switch between theory building and theory testing over the course of an innovation project, it has offered few insights about how different patterns and combinations impact project outcomes. This study begins to address these shortcomings and suggests better innovation outcomes might emerge from more rigorous application of underlying academic methodologies.
Research setting and methodology
The setting of the study is EduTech, a company that operated as a textbook publisher in the field of higher education, primarily in the United States. As this research began, the organization was attempting to reinvent itself as an educational technology company. In order to achieve this reinvention, it sought to develop new educational technology products for the US market. This task fell to members of product teams assigned to create products for specific academic disciplines (e.g., economics, marketing, management). Data collection for this research started as the organization began its innovation efforts. Initially, the author was involved with the organization in an authoring role. Interest in the research topic emerged through interactions with employees and observation of initial innovation efforts. These initial interactions and observations demonstrated that EduTech was well suited for investigating innovation strategy and process for two reasons. First, product development at EduTech occurs in twelve-month cycles tied to the academic year. This provided a natural start and end point for the study. Research fieldwork spanned 15 months, allowing observation of a full development cycle. Second, product development at EduTech occurs in teams aligned to academic disciplines. This allowed a multiple case study design, which treats cases as a series of experiments used to confirm or disconfirm emerging conceptual insights (Eisenhardt, 1989).
Research design
Employing a multiple case study design required carefully selecting comparable innovation teams (Eisenhardt, 1989). The five teams were theoretically sampled (out of approximately 30), based on initial inductive data collection and analysis (Corbin and Strauss, 2014). The teams were selected for several reasons. First, each accounted for a significant share of EduTech’s overall revenue, was seen as strategically important to the company and was expected (and had funding) to be actively developing new digital products. Second, all shared the same core market – introductory courses typically required for all undergraduate business majors. Third, each team had the same structure, which was also similar to the structures used by many other organizations (e.g., Brown and Eisenhardt, 1995). One or two product managers led each team and maintained a great deal of discretion over its activities. 3 These individuals had profit-and-loss responsibility for their academic disciplines. Their backgrounds varied, but they tended to have experience in marketing, new product development or both. The rest of the team was made up of four to five content developers, two to three instructional designers and a marketing manager. Content developers typically did not have experience in the field of education or the discipline covered by the team, but were college graduates. Their job descriptions focused on coordinating the creation of products by participating in market research and product strategy discussions, managing workflows and timelines, and performing basic editorial and design tasks. Instructional designers were current or former instructors in their assigned disciplines. Most had doctor’s or master’s degrees in their field. Their job descriptions identified them as subject matter experts. They were also expected to provide extensive input on market research and product strategy. Marketing managers’ primary role was to serve as a liaison between the product teams and the sales organization, but they were also heavily integrated into innovation efforts.
The fourth reason the teams were selected was that the majority of members from each team was located in EduTech’s Midwest US office. This meant the teams were physically proximate to the same set of external stakeholders (i.e., they shared the same local universities for on-campus market research efforts). Fifth, the teams were all starting from the same point with respect to innovation. Each had digital products that used EduTech’s existing online courseware solution. This courseware digitized print textbooks and provided teams with several standard, closed-ended question types (e.g., multiple choice, true/false) they could use to create online assessments. In sum, the teams were well matched in their exposure to the organizational structure, goals and expectations emanating from the upper levels of the organization and from external stakeholders. These factors made the teams highly comparable and helped ensure any differences in outcomes were driven by variations in innovation processes, as opposed to team, organization characteristics or market-level differences (Eisenhardt, 1989).
The final reason the teams were chosen stemmed from a key difference among them: despite sharing all the characteristics described above, initial interviews and observations suggested they were beginning to develop products with markedly different approaches and characteristics, especially with respect to how they identified student and faculty interests and tested product concepts. The analysis focused on the sources of these differences, as well as their implications (George and Bennett, 2005; Yin, 2013).
Data
Data were collected during 2015 and 2016. Over 15 months of data collection, the author spent an average of 40 hours per week at EduTech offices. Three types of data were collected: how team members interpreted their experiences, what actually happened within the teams and subsequent outcomes. The data are summarized in Table 1 and described in more detail below.
Interviews
Throughout the study, 97 semi-structured interviews with individuals associated with the five focal product teams were conducted. Interviews were conducted with every member of all five teams. Also interviewed were individuals who interfaced with one or more of the teams and contributed to their innovation efforts. These individuals held one of two roles. Product directors sat at one level above product managers in the organizational hierarchy. Although product managers had profit-and-loss responsibility, product directors provided guidance and oversight, and had the final sign off on some budget decisions. Software development managers filled a liaison role, connecting the product teams to the digital technology group that built the digital products (i.e., did the programming work). Software development managers were not officially part of the product teams, but were included in many innovation-related activities. For example, they were often asked to weigh in on the feasibility and costs of various ideas. Variation across teams and roles was deliberately sought. This helped separate whether differences in interviews were driven by role, when the interview was conducted or other factors. Follow-up conversations with earlier interview participants were also held later in the research period to see if their perspectives had shifted. Interviews were recorded, transcribed verbatim and lasted, on average, an hour each.
Observations
Observations were conducted throughout the entire fifteen-month research period. Observations initially helped refine research questions and design, but subsequently were chosen to leverage the multiple case study design. For each product team, the author requested access to any meetings relevant to innovation, including brainstorming sessions, strategy discussions and market research planning, execution and analysis. While interviews provided data about individuals’ interpretations and retrospective accounts of their experiences, observations revealed how actions and interactions unfolded in real time. This helped address shortcomings with each data type and to disentangle relationships between how people think and act (Leonard-Barton, 1990). Extensive field notes were taken on a laptop, capturing as much of the dialogue as possible. In most cases directly after the meeting, but always within 24 hours, these notes were revisited in order to expand shorthand and add detail. In total, the result was 110 days of observation and over 1,000 pages of field notes.
Archival data
Over the 15 months of the study, the author had an EduTech-issued laptop, an email address and access to all of the internal IT systems available to product team members. It was possible to access the ‘team drives’ for each product team and all relevant files members posted there. The author automatically received all company-wide emails and asked to be copied on relevant intra-team emails (and meeting invitations). While it was not possible to control the extent to which members followed through on this request, a large number of emails was received from each team. Ultimately, 711 archival documents were collected from the five product teams, which amounted to over 2,000 pages. In addition to emails, these included drafts of online surveys, the contents of online discussion boards, product prototypes, financial data and strategic planning documents. 4
Analysis
The ethnographic approach resulted in a collection of data that were incredibly rich, but also highly complex. Like most process-related data, these data covered multiple levels and units of analysis with ambiguous boundaries. The data are also eclectic, and include not only actions and events, but also thoughts, feelings and interpretations (Langley, 1999). Moreover, whereas many process-focused studies look to understand the dynamics of one case, this research design intentionally drew upon multiple theoretically sampled cases intended to provide a basis for comparison (Eisenhardt, 1989). In order to make sense of these complex data, two strategies Langley (1999) articulated in her seminal article on theorizing from process data – the narrative strategy and the synthetic strategy – were combined across three analytical phases.
Narrative strategy
In the first phase of analysis, focus was on each case (i.e., the experience and outcomes of each team) individually. The data were organized and coded by team. These coded data served as an input to the development of comprehensive narratives of each team’s innovation project. These narratives triangulated on interview, observational and archival data. They outlined the concrete actions and steps each team took (e.g., market research efforts, strategy and design meetings, product launch activities), the decisions it made (e.g., in terms of which products to pursue, which features to include, how to market its products) and the outcomes that followed these actions (e.g., sales, stakeholder reactions, removal of products from the market). The narratives, which were each 30 to 50 pages long, were primarily descriptive accounts that combined raw data (e.g., quotes and screenshots) with summaries and contextualization of the data. The narratives were an input to the second analytical phase.
Synthetics strategy
The second analytical phase focused on moving from the construction of individual team narratives to developing a variance-oriented theory (Langley, 1999). Taking the different innovation project outcomes as the starting point, the narratives (and the data underlying them) were compared to explore variables associated with different outcomes. The term ‘variable’ is used loosely here, as it was not necessary to look for measurable, quantifiable factors, but rather to parse different understandings, approaches and processual steps to disentangle how they influenced innovation outcomes. The author was guided by the language of the data: for example, such terms as ‘empathy’, ‘ethnographic’, ‘design sprint’, ‘prototype’ and ‘experiment. Particular attention was paid to how different teams discussed such terms, as well as if and how their interpretations shaped the actions they took. It was through such comparisons – across cases and between the cases and the literature – that it proved possible to make a theoretical leap (Klag and Langley, 2013) and move towards a higher level of abstraction.
Formalizing the theory
In the third analytical phase, the results of the second phase were used to develop the theory presented in this paper. Specifically, contrasting the interpretations, processes and outcomes of the five teams made visible the dynamic relationship between theory building and theory testing. The appropriate sequencing and depth of each strategy was driven by the number and interrelationships of various stakeholder interests, which underpinned theorizing about multiplicity and its impact on innovation. In this phase, iteration continued between the data and the literature to consider how and why it inadequately addressed the issue of multiplicity, as well as how these shortcomings might be practically addressed – namely, by more closely rooting common innovation techniques in the underlying academic research methods.
Findings
Inductive analysis shows that the five teams differed in the extent to which, when and how they combined theory building and theory testing throughout their innovation projects, and that these differences were associated with divergent outcomes. These outcomes – and the effectiveness of different combinations of theory building and theory testing – were shaped by multiplicity in the innovation context. Multiplicity – the presence of multiple customer types or stakeholders with diverse, potentially conflicting interests – increased the value of rigorous theory building while also increasing the risks and costs of testing poorly grounded theories.
The findings are presented in three connected subsections. In the first, the role of multiplicity in innovation is developed by describing how the existence of multiple customers with multiple interrelated and potentially contradictory interests complicated innovation efforts. The second describes the efforts of the teams to illustrate how inadequate grounded theory building can contribute to poor innovation outcomes. Finally, a counterfactual (Omega team) helps theorize how rigorous, grounded theory building, supported by theoretical saturation and maintaining distance, can help teams effectively navigate multiplicity.
Multiplicity and innovation
My analysis suggests innovation outcomes were critically shaped by teams’ understanding (or lack thereof) of relevant stakeholders’ interests, where ‘interests’ refers to the broad set of needs, desires and concerns relevant to a product concept. In particular, the findings highlight the importance of understanding the relationships among these interests. Together, the constellation of interests and the relationships among them reflect the relative multiplicity of the innovation context (Neville and Menguc, 2006). Multiplicity makes innovation more challenging since new products or services must effectively balance competing priorities. Successfully navigating market uncertainty and ambiguity ultimately depended on the teams’ ability to recognize the extent to which interests were aligned or could be in tension for a particular stakeholder or across different stakeholders, as well as how the stakeholders prioritized their various interests. These insights are made evident by considering the interests – and the relationships among them – eventually identified by the five innovation teams. 5
Number of stakeholder types
By the end of the study, the teams all recognized their innovation success required balancing the interests of two key stakeholder groups or, in this case, customer types: students and faculty. Students were end users for any product since they would be the ones completing any assignments delivered through that product. Students were also typically the ones who purchased the products, usually as a required component of a course. As the designers of a course, faculty were typically the ones making decisions about which products students would be required to purchase. They were also end users to the extent that they needed to select, assign and evaluate readings and activities delivered through the products. New products needed to navigate the interests of both groups:
When I started it was all about professors. Nobody ever talked about students, nobody ever talked to students … Then there was a huge shift and it was like, ‘No, talk to the students … They’re both the customer and we can’t ignore the professor because they’re making the decision. Can’t ignore the student because they’re the one using it. (Content Developer [101] Gamma)
Number and relative prioritization of interests
In addition to being distinct customer types, students and faculty each had multiple interests. These interests were often in tension with one another, both for individual students/faculty and across the two customer types. The interests can be split into two categories: means and ends (see Table 2).
Interests in the ends category relate to outcomes or goals associated with the use of EduTech products. For example, teams found that both faculty and students hoped products would allow students to develop useful knowledge and skills that would help them obtain and perform well in a job:
The instructors are looking for, certainly to cover certain knowledge … in [our discipline], what we actually talk about as an outcome is getting students to think like [business people]. We kind of think that’s what the ideal is for instructors. Students, I think that would be ideal for them too. … Students are looking for outcomes from the class. …They’re looking for skills. …Skills are a big component of what they’re looking for … things that will translate into my getting a job and my doing well in a job … I need to be able to say when I’m done with this program, I can check off these competencies or skills, or I am certified in this subject matter area. (Product Manager [15] Beta)
Means-related interests are associated with actual use of a product. In general, these interests relate to the ease with which desired ends can be achieved. By the end of the study, the teams had collectively uncovered several means-related interests that were shared by students and faculty. Two stood out, in particular. The first was a desire to minimize the time and effort required to achieve a particular end. For example, students consistently expressed a desire to minimize the time and effort expended on readings and assignments, and to obtain what they viewed as an acceptable grade:
The grade is the ultimate fruit for [students], they want to have that. … The grade is the ultimate thing, but if in getting to the grade, they end up wounded and bleeding to death, that is going to be painful. You can get the same grade if you read in the book versus if you do digital, [but] digital [can] make it a bit easier and faster for you to do it. (Software Development Manager [10] Gamma)
Similarly, faculty expressed a desire to minimize the time and effort they spent setting up assignments, grading and monitoring student effort and performance:
Essentially [faculty want] things to make their lives easier. So, rather than them having to assign a paper quiz that they want to give out, they can do a digital quiz that will be graded for them. So … where we might have provided a test bank in the past, now an instructor could automatically incorporate that into their digital path. And again, [have it] automatically graded. … Just in terms of assigning content and grading, [making] it a lot easier for them. (Content Development Manager [8] Beta)
Such interests were intertwined with interests related to the user experience. For example, this included the extent to which a product was easy or difficult to navigate and use, whether the product contained technical glitches, and whether it was consistent with other aspects of users’ workflow (i.e., when and how students and faculty preferred to complete their work):
[Faculty and students want] consistency. Reliability … By far the largest thing that I hear, is that they want to be able to do what they go [into the product] to do, right? You can give all of the frills and everything, awesome, then we attract them for maybe 10 seconds, but if that frill works and the rest of our product doesn’t, it is not going to be much use. … If we end up making their lives harder in any way, then they don’t want it. (Software Development Manager [10] Gamma)
Second, both students and faculty were interested in minimizing the monetary cost of obtaining a particular outcome. In fact, teams often attributed EduTech’s recent financial struggles to such concerns. They suggested that students and faculty perceived traditional textbooks’ rapidly escalating prices not only as unreasonable, but as evidence of price gouging. They argued that innovations would need to counter such perspectives:
The other problem [with our print textbooks] is we raised prices, prices got too high. I mean you are talking $200 or $300 or more for a book … Even instructors said, ‘You know what, I don’t need to roll to the next edition, the examples might be a little out of date, but I can still stay with [the previous edition] and my students can get used books for under $20 if not for free’ … High prices and quick revision cycles have caused our own demise … We have to right price the digital … I’ll just say maybe on average in the company at $100. In [our discipline] we [are aiming] for $85 directly to the student. (Product Manager [15] Beta)
Together, the various means and ends interests complicated innovation because they were not perfectly aligned and often appeared to be in tension or direct conflict. First, ends-related interests associated with learning outcomes often seemed to be in tension with means-related interests. For example, generating better learning outcomes often seemed to require costly technological investments that, to be profitable, would require higher prices:
In terms of innovation, it [might be] a brilliant idea, but in terms of the company’s ability to answer that need … it requires a huge infrastructure that we don’t have. This functionality [may be] necessary, but … right now, the [potential profitability] of it cannot sustain cost of building it. So, in terms of building a digital product that meets the needs of the market. I mean we were showing wireframes to people and they were lining up, saying, when will it be ready, we want it now. So … that’s kind of the frustration of it, I guess [what we can build and offer affordably]. (Product Manager [6] Delta)
Second, tensions existed because students and faculty did not always seem to prioritize their interests in the same ways, or consistently over time. For example, students seemed to put more weight on means-related concerns, particularly the speed and ease with which a particular learning task could be completed:
A challenge for us is that we don’t necessarily want to teach to the test, [which is often] what the students [say they] really want … What we’re finding is that students today struggle with ambiguity. They just really want to know, between A, B, C or D which is the most obvious and right [answer] … They almost grade teachers based on that. ‘Instructor was great because they gave us a study guide. They told us exactly what’s going to be on the test’. Not because that instructor forced me to think of things differently and really challenged me … [At the same time] we’re trying to help instructors elevate thinking … through [our innovations]. (Product Manager [15] Beta)
This prioritization, at least in part, seemed to reflect students’ perception of grades as confirmation that they had achieved learning, as well as a signal of that learning to potential employers. Students, however, often seemed to focus on the signal and to view obtaining high grades as a sort of interim end that could contribute to the larger goal of securing a job. As a result, at least in the moment, students often seemed more sensitive to how quickly and easily they could obtain a particular grade than what they actually learned in the process:
Students want a good grade. That’s the outcome … I mean I would like to say that they really care about learning, but I think that is a small population of students. In general, they would rather just have an A, or a B, or whatever they think is like the minimum necessary grade to get their degree … Okay, so that is kind of jaded. I mean I do think that students want to learn. But [most] want to learn enough that they can go out in the world and get their degree and get a job … It is a means to an end. (Content Development Manager [8] Beta)
In contrast, although faculty undoubtedly hoped their students obtained good jobs, they tended to have a different – and perhaps better informed – perspective on learning and grades. Faculty tended to express a belief that learning is, to some extent, necessarily difficult and challenging. Consistent with academic research, they often indicated that learning could be time-consuming and may even require some degree of discomfort and struggle, particularly to obtain deeper, more valuable forms (Sandberg and Barnard, 1997):
We’re constantly balancing what students want versus what professors want. I think both are important … Sometimes what students want is important but they don’t always know what the best way is … Seeing the broader picture about what your education should be isn’t as clear. (Product Assistant [103] Omega)
As a result, although they, too, viewed grades as an indicator of learning, teams found faculty were concerned that making grades easier to obtain could diminish the value and relevance of grades as an indicator:
If [an innovation] is not rigorous enough or it spoon-feeds students, then [faculty] won’t buy it … For example, with [product idea], it is a clear, clear need that the students … want more help. But, the instructors … want the students to struggle. They believe that struggle is a good thing … Whenever I have heard [faculty] say that they wouldn’t use something … the biggest complaint is that it’s not challenging enough. (Product Manager [6] Delta)
At a minimum, such conflicting viewpoints raise questions about how the innovation teams might effectively satisfy both customer types. Yet, these sorts of tensions were often even more nuanced, entangled and difficult to navigate. For example, faculty members themselves often expressed conflicting feelings about how to balance ends-related learning objectives against students’ means-related concerns. In particular, teams found that faculty viewed their own job security and career advancement as partially determined by student evaluations. They expressed concern that pedagogically effective, but challenging or time-consuming, assignments might result in poor student evaluations, which might threaten their career progress:
Instructors also want to get a good evaluation. They think about their own careers a lot. A lot of times their incentives or pay are based on their evaluation process, which students are involved in. (Product Manager [4] Alpha)
Faculty admitted that such concerns factored into their own decisions about learning materials and tools and, thus, potentially impacted the market success of new products:
The problem is even though the instructors love [an educationally rigorous product] … they also tell us [negative] feedback from students … can be very damaging [to their career growth]. (Marketing Manager [80] Omega)
By the end of the study, the teams universally recognized that failing to effectively navigate these and other tensions generally meant a new product was destined to fail:
The challenge is [not just] hearing what students are saying, but being able to interpret that. And then also hearing what instructors are saying and matching those up. Because we are in the position where the instructor makes the adoption decision but the student buys [the product]. So, we have a gatekeeper that before we reach our end purchase, there’s somebody that has to say yes, I want you guys to buy this. That … can be a challenge … Students tell us a lot of things like, ‘Oh that would be really awesome if you did this’, and the instructors say, ‘No, I don’t like that’ or ‘That’s not appropriate for my class’ or whatever. (Product Director [17] Beta)
Yet, most of the teams did not recognize this reality until after they had launched a product – and had seen it fail in critical ways.
Theory building, theory testing and multiplicity
This section explores what contributed to each team’s understanding (or lack thereof) and navigation of multiplicity. The cross-case comparisons suggest that poor outcomes were associated with insufficiently developed or poorly grounded theories and a bias towards theory testing. The unsuccessful teams failed to address two critical tenets of effective grounded theory building in academic research: purposive sampling and maintaining distance.
Purposive sampling
Inductive, ethnographic academic research – particularly research in the tradition of grounded theory – relies on purposive, or theoretical, sampling strategies (Corbin and Strauss, 2014). Unlike strategies in quantitative research, such as random sampling, purposive techniques are not intended to generate a sample that is statistically representative of the population under study. Rather, the goal is to uncover and understand all of the relevant sources of variation in the context (Small, 2009). Further, the goal is generally not to quantify the effects of one variable on another, but rather to understand how and why variables are related. This is typically achieved by reaching theoretical saturation, or continuing to collect data until subsequent efforts fail to uncover any new relationships or sources of variance (Corbin and Strauss, 2014). Scholars have long advocated saturation as a safeguard against developing theories that fail to account for or explain the full range of perspectives, relationships and situations relevant to the research context (Glaser and Strauss, 1967).
All five teams began their innovation efforts with the sort of inductive, ethnographic market research that has been popularized as a way to identify latent market needs, but three (Alpha, Gamma and Delta) did not engage in purposive sampling or reach saturation. Rather, they only collected data until they identified one unaddressed customer interest they believed represented a viable market opportunity. At that point, the teams immediately shifted to ideating and testing solutions. Initially, this rapid shift to theory testing generated support for the teams’ hypotheses; however, these early ‘successes’ later proved to have created costly path dependence. More specifically, because initial tests provided evidence that proposed solutions did effectively address the identified interest, the teams continued to invest in these solutions. These investments later proved misguided when the solutions were eventually tested against other customer interests and proved incompatible with them.
Delta’s experience clearly demonstrates this pattern. The team recognized that existing digital learning products were poorly aligned with students’ preferred workflow. The team discovered this very early in the innovation process through ethnographic research – it had students walk through how they completed assignments:
We were asking students … when they were doing their homework, what did their desk look like? And then [we] had them draw it out, everything. And then we asked them what would your sort of ideal education, homework product look like? They had to draw out the screen, what they would see if they were to log into one of our products. And they drew the homework on one side and all the resources on the other side. Or the homework on top and all the resources at the bottom. But they were consistently drawing the kind of framework [with the] homework and then resources to help out with that homework. (Instructional Designer [12] Delta)
Based on this insight, the team quickly began testing possible solutions. Initially, this testing was low-cost and low-fidelity, such as showing students paper mockups of the idea, or asking students to draw their own solutions:
[To test the product with students], we started out by giving a piece of paper … that look[ed] like iPads, and computers, and stuff. We just threw that to students and were like, ‘Pick one of these, whatever you use the most, and draw us what you wish you had for your course right now. What would that online solution look like?’ About 90% of them drew [our product] … they all were drawing [our product]. Then, we could turn around and show our mock-up. They were like, ‘Yeah, that’s exactly what we want.’ (Content Developer [96] Delta)
These initial tests generated positive feedback for the team’s proposed dual-screen solution, so the team escalated its commitment and began building out a working digital version.
Throughout all the testing, the team assumed – but did not verify – that a large percentage of faculty would embrace a product that students liked. Interestingly, the team recognized that they were assuming faculty would accept the product, and that its success, in part, hinged on this assumption. However, the team was highly confident that the assumption was accurate:
Being student centered is the best way to please as many people as possible. I mean [students] are who we are trying to improve outcomes for already, but I think just in terms of long-term success in being in as many classrooms as possible, I think having empathy for the students and making products that really work for them … I think it is possible to please the instructor, but not students. I think it is going to be hard to please all of the students and not at the same time be a hit with the instructors and the department and everything. It is our best shot … We can come from a sort of empathetic design standpoint where we are thinking about, okay, how are students going to use it? (Instructional Designer [12] Delta)
Delta team’s (over)confidence meant it did not engage in any substantive inductive research with faculty. Instead, the team quickly developed a theory (i.e., a potential product idea) without reaching the theoretical saturation grounded theory researchers seek. Unfortunately, when the team tried to convince faculty to adopt the product for their courses, it became clear that its assumptions were wrong:
We didn’t realize it until we started talking with professors and started going to some conferences … We ran focus groups and just talking to professors throughout the week … [we learned] there’s a lot of author devotion … One of the things we heard a lot with [new product] is So is EduTech teaching the students now, not the authors, not me? … Who are these EduTech people that are writing this material? (Content Developer [19] Delta)
Unfortunately for the team, the learning came so late that the key concerns could no longer be addressed without completely altering the product:
[Faculty] have a ton of feedback. None that we were able to incorporate, we just didn’t have time for engineering work … We did a summit [to show the product to faculty] at [a conference] last year … and it was a disaster … That was pretty eye-opening, when we did the summit. We thought we went into it so solid. We’re like, ‘Yes, here is our demo. We have our story down’. We had this cool activity. We had 25 attendees that we paid to fly in early, and all of that jazz. We’re like, ‘This is so solid. We’re going to walk out with all of these instructors, like, Can’t wait to purchase it,’ and it was a huge fail. (Content Developer [96] Delta)
At this point, the only viable option appeared to be torpedoing the project. The product was taken off the market before the author left the field, with EduTech taking approximately $1 million in development costs as a loss.
Maintaining distance
Another key tenet of grounded theory research methodologies is the importance of maintaining distance, of avoiding ‘going native’ (Bernard, 2002, p. 328). Like achieving theoretical saturation, maintaining distance is critical to ensuring that researchers recognize and incorporate the full range of perspectives, relationships and situations as they develop new theories. Going native is a concern in that it causes researchers to lose the ability to contextualize data and abstract away from informants’ points of view, which can lead to biased or incomplete understanding of the phenomena being investigated (Bernard, 2002). In particular, biases can prevent researchers from adequately understanding the complex relationships between different informants and their interests.
At its core, a failure to maintain distance often stems from misunderstandings about the role of empathy in theory building. Developing empathy (or seeing the world through another’s eyes) helps a researcher understand how the other person makes decisions and the rationale behind their actions and behaviors (Brown, 2008). However, empathy is a means to an end. The ultimate goal is not to understand the specific individual’s (or group’s) perspective, but rather to understand how that perspective, and the decisions and actions associated with it, influence larger social dynamics (Corbin and Strauss, 2014). Although practitioner-oriented innovation methodologies do not directly contradict this version of empathy, they do tend to emphasize the benefits of getting close to customers, as opposed to the need to maintain distance.
The dangers of inadequate distance were evident in four of the five innovation projects. Beta’s experience in particular demonstrates the danger of not maintaining distance even when sampling saturation is achieved. The team worked hard to develop empathy for both students and faculty and uncovered a wide range of means-related and ends-related interests before starting to develop a product concept:
Really our goal is to be able to do what we call ‘ethnographic’ research … We want to almost be on our student’s shoulder. Looking over our student’s shoulder, we want to see when they’re doing this, when they study and exactly how they study and what. You know, where they’re clicking to study … We want to really understand how students are studying using digital now … The ideal is to get that ethnographic [insight]. (Product Manager [15] Beta)
The issue with these efforts was that the team’s empathy led it to try and address every interest it uncovered. The team failed to contextualize its data and recognize the need to balance tensions associated with the various interests. The result was a convoluted and, ultimately, failed product.
Beta’s product concept focused on groupwork, a type of assignment faculty suggested could be incredibly valuable from a pedagogical perspective, but was challenging to manage:
One of the big challenges we found was groupwork. Instructors wanted to do it, but didn’t have an efficient way to do it. (Product Director [17] Beta)
The team also recognized that students found groupwork frustrating. In fact, the team conducted extensive research to uncover all of students’ and instructors’ groupwork-related frustrations:
Students didn’t like groupwork and the two reasons that they gave was, the biggest reason … was they said they get assigned to a group and they can’t find the time to all get together. They all have conflicting schedules and it makes it really difficult. The second reason … is there’s no accountability in group work, there are social loafers. (Product Director [17] Beta)
The team then set out to develop a solution that addressed all of the frustrations. For example, it addressed student freeloading concerns with features that allowed faculty to watch recordings of group meetings and track group members’ contributions. It addressed faculty concerns about the time and effort required to grade projects by creating a peer grading system. The team also addressed student concerns about coordinating busy schedules – and faculty complaints about having to deal with such issues – by adding a number of scheduling tools.
Despite the team’s efforts to address all of the concerns it identified, the product failed on the market and was no longer being offered to customers by the end of the study. In Beta’s case, the failure appeared to be driven by three key mistakes, each of which was rooted in inadequate understanding of how customer interests were interrelated. The first mistake was failing to understand tensions between faculty members’ ends-related pedagogical aims and students’ means-related interests. Although faculty complained about dealing with students’ coordination and freeloading concerns, the team eventually learned that such challenges were a key reason faculty assigned groupwork in the first place. They wanted students to struggle through such issues to prepare them for the workplace:
Professors, they’re saying you have to work in a group in a workplace. There’s no getting around that. You’re going to work with other people. You need to develop this skill. (Product Director [17] Beta)
Second, the team made a similar mistake in attempting to address means-related faculty concerns. Specifically, although the peer grading feature was intended to address faculty complaints about the time and effort grading required, the feature created an additional frustration for students, who were now expected to spend time grading other group members:
The [peer grading] is just throwing in [a student’s] face that I have more work to do while my teacher has less work to do, and that just pisses me off so I don’t want to use it anyway. (Product Assistant [20] Beta)
Further, the team also eventually learned this solution did not satisfy faculty, who anticipated complaints from students who believed their peers were grading unfairly.
Third, by trying to address every interest they identified, Beta team created a product that had myriad features, but was confusing and time-consuming to use. After releasing the product, the team realized the difficulty of using the product was perceived to outweigh any benefits:
One of the biggest problems … is [that the product is] not intuitive …You’re using something that’s difficult to use to try to [do] something that’s difficult … We’re not really making [it] easier for you to use so that you can just focus on learning … It is difficult to use at every single step … Ideas around usability and around making something easier were ignored. (Product Assistant [20] Beta)
More effective theory building
Omega Team’s innovation efforts and outcomes provide a strong counterfactual and offer insights into how to combine theory building and theory testing in innovation contexts with high multiplicity. Although the similarities were not necessarily intentional, the team’s approach to theory building was broadly consistent with rigorous, academic grounded-theory methodologies. Three specific aspects of the team’s approach stand out.
Reflection and review
Whether academics are conducting inductive research (which is typically aimed at theory building) or deductive research (which is more typically focused on theory testing), they generally do not launch a study on a whim. Rather, academics usually begin with a careful review of what is already known about a particular topic; for example, by completing a thorough literature review. They then form a research question and study design based on perceived gaps or contradictions in the literature. Like academics, Omega did not immediately launch into its innovation project, but instead engaged in an extended period of reflection and review. The team accomplished this via a book club modeled after a graduate school seminar:
I run [the book club] like a graduate seminar, because that’s where I come from. I told them that up front. ‘Look … it’s going to be part graduate seminar. We’re going to learn about disruption, we’re going to learn about innovation itself’. … It definitely helps our products and thoughts about product development in the digital space. It gets us thinking more deeply about what we do. (Instructional Designer [70] Omega)
The book club had two key components. First, the team discussed the innovation context in general, including who the key stakeholders were and what the team was attempting to achieve:
We’re talking about all these different disruptive products, but are the people we deal with really open to disruptive products? Because higher education is kind of a very slow evolving monstrosity. We have very frank discussions about the course our discipline should take, the product models we should be focusing on, questioning assumptions and learning about our own business. (Instructional Designer [70] Omega)
Second, the team conducted an inventory of EduTech’s existing technologies, including identifying what capabilities already existed, if and how the technologies had been used previously, and the results (i.e., market performance). In essence, this was like a practitioner version of a literature review. It provided insights about potential pitfalls, as well as about existing capabilities the team might potentially leverage with its innovations:
One thing we did is we looked at other areas of the company that we don’t always communicate with … We tried to find digital products that we didn’t know about, so we could get a better grasp of what we, as a whole, are capable of doing. That really helped with … and is helping with [product] development. (Content Developer [95] Omega)
Focus on relationships
After several months of book club meetings, Omega began inductive data collection and analysis. This included ethnographic observation and interviews with faculty and students. This research was guided by a sense of empathy, but also a conscious effort to maintain distance. The primary focus was on understanding the relationships between the two customer groups and their interests:
Why would we go to a student who I recognize is telling me, ‘I just want to get the best score. I’m fine with a C’? Why would I build a product around that? I would not build a product around that. I build tools and features within [a product] that cater to [instructor objectives], but then bring the students in and get feedback on what you’re building so, “Hey, is this helpful to you? No? Why not?’ ‘It could be shorter’. ‘Okay, we’ll try and make it shorter’. Or, ‘No, you should put it here instead of here’. Things like that. (Product Manager [56] Omega)
To develop this understanding, the team used a simple process akin to the development of themes in grounded theory research (Corbin and Strauss, 2014). It used a Trello board to create different cards for faculty and students. 6 On these cards, team members posted patterns and insights gleaned from the data, with links to supporting evidence. Team meetings revolved around these cards, with discussion not only about points of overlap, but also tensions and inconsistencies. Based on these discussions, the team concluded that it could not, and should not, address every interest:
What do we do? Do we help the student get what the student wants out of the product, or do we force them to deal with what the instructor wants them to get out of the product? …
That’s why we keep having these meetings … Digital is going to happen no matter what. It’s just a matter of where we position ourselves, and how far can we make the students happy without ticking off the instructors or making them think they’re being alienated. (Content Developer [100] Omega)
Theory/hypothesis testing
Omega team used the themes discussed above to formulate an overarching theory, which in this case was a basic product concept that it believed (it turns out correctly) would be well-received by both customer types. The concept sought to address a shared end-related interest; for students to develop job relevant skills with popular business software:
What we’ve tried to do through research … through faculty interviews, surveys, student intercepts, student focus groups, [we learned everyone cares about] skills. This [software] is a skill that business school students need to develop, because employers and hiring firms are requiring it … [they] want the skill … [for] students to be able to use [the software] for interpreting the data and analyzing it and communicating the results of what it means. (Product Manager [56] Omega)
At the same time, the team recognized the need to consider means-related interests. It was at this point that the team shifted from theory building to theory testing. Specifically, it began to conduct tests that sought to validate the overarching concept, but also to understand the potential tensions and contradictions. The testing was largely focused on determining what means-related tradeoffs would be acceptable to students and faculty in order to achieve the shared ends-related interest. Further, perhaps because it maintained distance from customers, the team was open about these tradeoffs. For example, one key issue the team identified was that, in order to support deep learning, problems delivered through the solution needed to be fairly complex. However, this made it impossible to (cost-effectively) provide auto-grading functionality. Thus, instructors would need to grade manually any complex problems, a requirement in tension with their means-related interest in minimizing the time and effort spent on such tasks. Rather than assume that faculty would or would not accept that tradeoff, the team tested it directly by showing them sample problems and seeing which options were preferred. The team validated that faculty were, on average, willing to spend time grading in order to generate more learning:
Too often … we’ve gone into a planning meeting for a product and said, ‘It’s like a check list. You do this, this, this’. We’ve started to test some of those ideas, even with faculty to say, ‘Look, we know in an ideal world you’d love to have every problem in the book in there with all of this [auto-grading], but if we gave you these choices, what would you pick?’ and actually test preferences on that. In most cases, they’re like, ‘We’d much rather have the rich[er] problems’. (Product Manager [56] Omega)
Contrasting outcomes
As mentioned above, Delta’s and Beta’s new products had been taken off the market by the time fieldwork ended, and the teams missed their annual revenue and profit targets by a wide margin. 7
Although Alpha and Gamma were not forced to abandon any products, 8 these teams similarly missed revenue and profit targets. In contrast, Omega surpassed its targets. The team’s product garnered positive attention both inside and outside EduTech. Internally, Omega was named product team of the year based on its innovation. Externally, the product had already been named a finalist for an industry award. Moreover, these positive outcomes were achieved with surprisingly low product development costs. Some programming was required to embed the commercial business tool within EduTech’s courseware, but the team consciously chose to use a free, online version of the software to minimize licensing and other costs. The team was also able to explain its vision to the developer of the commercial software. The developer recognized the benefit of having students learn its software, which encouraged it to participate in the development efforts and share the associated costs.
Discussion
This study examines how theory building and theory testing approaches to navigating market uncertainty might be effectively combined during innovation projects. Inductive investigation of five theoretically sampled innovation projects suggests that the appropriate balance between these approaches is influenced by the level of multiplicity in the innovation context. As depicted in Figure 1, as multiplicity – or the presence of multiple stakeholders and/or multiple overlapping, potentially conflicting interests (Neville and Menguc, 2006; Hillebrand et al., 2015) – increases, the value of rigorous theory building increases. This is because multiplicity increases the potential risks and costs of path dependence associated with testing poorly grounded hypotheses.
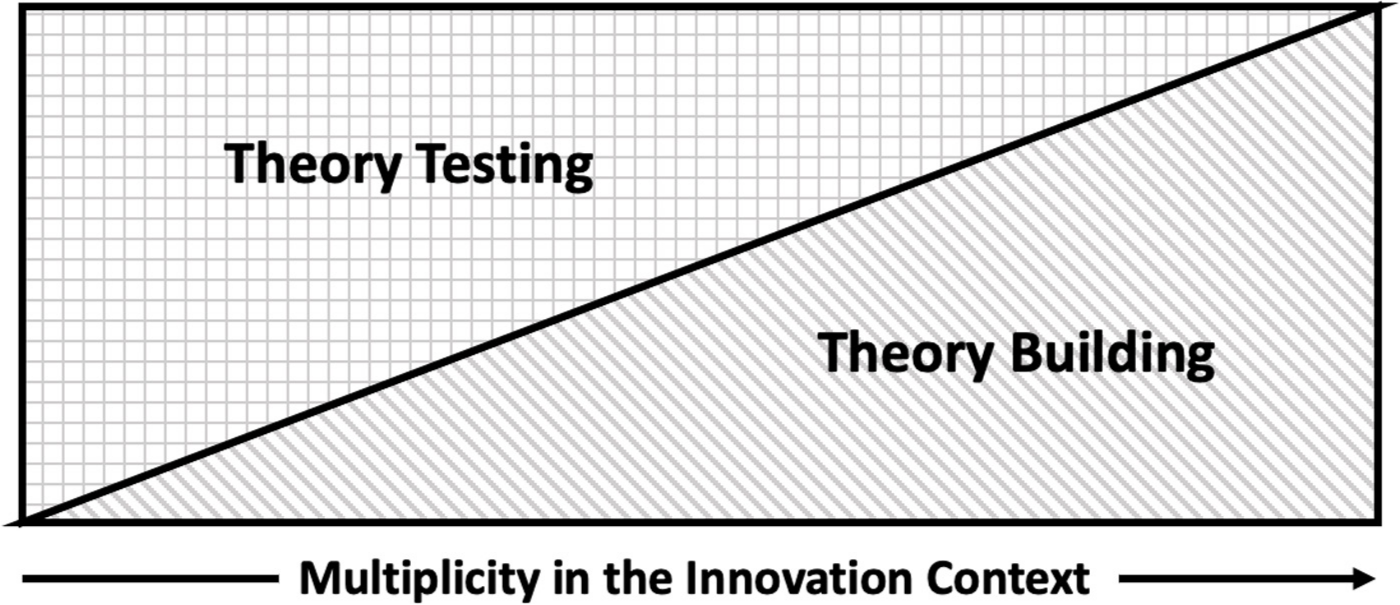
Innovation context multiplicity and the balance of theory building and theory testing approaches
The findings also illustrate the value of pursuing innovation with the logic and rigor of social science research methodologies. Evidence from this study demonstrates that the same tradeoffs and risks long recognized and agonized over in academic settings are relevant to practitioner adaptations of research methods. As a result, practitioners would be well-advised to develop an understanding of such methods, and to ensure they apply those methods appropriately and rigorously.
Together, these insights make three key contributions to the theory and practice of innovation. First, the study deepens our understanding about how teams might most effectively engage with users, customers and other stakeholders throughout innovation projects. The study reaffirms the value of early engagement and the use of ethnographic techniques to develop an understanding of user needs (e.g., Liedtka, 2015). However, the findings also illustrate potential detrimental effects of drawing on such methodologies in ways that are incomplete or lack rigor. This includes failing to recognize the importance of theoretical saturation, or identifying the full set of relevant stakeholders and developing a full understanding of their interests and needs before developing product-related theories or hypotheses. Achieving saturation has long been a key tenet of ethnographic research (e.g., Glaser and Strauss, 1967). Yet, although it is not inconsistent with common practitioner-oriented theory-building models, such models rarely emphasize saturation (e.g., Brown, 2008; Liedtka, 2018). As a result, particularly in contexts of high multiplicity, innovators may fail to develop the requisite understanding of stakeholder interests, and how these interests overlap and intersect. This, in turn, can result in poorly grounded product concepts (i.e., theories) and, ultimately, contribute to innovation failures.
The findings similarly illustrate the danger of too much empathy (Bloom, 2016). In qualitative research methodologies, empathy allows researchers to understand subjects’ perspectives and concerns. This understanding is not typically the end goal, however. Rather, it is an input that aids researchers’ efforts to develop broader, generalizable theory. This usually entails contextualizing individual perspectives within a larger social system (Corbin and Strauss, 2014). For example, such theories often consider how individual-level perceptions and decision making interact and aggregate to shape larger social outcomes (e.g., Langley, 1999; Gioia et al., 2013). Once again, such aims are not inconsistent with practitioner-oriented innovation techniques; however, these techniques tend to underemphasize the relational aspects of such research goals and may consciously or unconsciously focus would-be innovators on one particular set of stakeholders – usually end-users (e.g., Brown, 2008). Instead, innovators should focus on the larger picture. This requires maintaining a sense of distance in order to identify and navigate potentially conflicting interests (Corbin and Strauss, 2014; Bloom, 2016). The study also suggests the value of being open and honest with stakeholders about such conflicting concerns. For example, Omega benefitted from being open with faculty about tradeoffs among convenience, rigor and cost. Such discussions also helped them design tests to understand instructors’ real priorities.
Second, the study adds empirical credence to nascent concerns about the overemphasis on action in the teaching and practice of entrepreneurship and innovation (Felin et al., 2019; Gans et al., 2019). The study reaffirms the notion that directly testing ideas is valuable and appropriate. However, the findings also highlight how decisions based on test results can generate path dependence that escalates costs and can be difficult to reverse. As a result, testing and experimentation are not always the most cost-effective approaches to learning about market demand. In addition, the findings illustrate how and why innovators’ orientation towards experimentation is important. The study suggests the appropriate focus of experimentation might be disconfirming beliefs, not confirming that assumptions or hypotheses are correct. Such a focus parallels the logic of academic experiments which do not focus on proving a hypothesis, but rather demonstrating that the alternative (i.e., the null hypothesis) is statistically unlikely (Krueger, 2001).
Third, the study suggests a need to rethink key aspects of how we teach innovation. Efforts to develop innovation capabilities have exploded in recent years, at a variety of levels and in a variety of formats. Most business schools offer undergraduate and graduate courses – and sometimes entire degree-granting programs – focused on innovation (Katz, 2003). Increasingly, such offerings reach beyond business schools. For example, university programs focused on the arts, engineering, education and other areas increasingly cover these topics. Similarly, a growing number of schools offer coursework or extracurricular activities focused on innovation and entrepreneurship (Regele and Neck, 2012). A range of workshops and professional development opportunities focused on innovation methods are also offered to corporate employees, nonprofit employees and public administrators. These initiatives tend to draw heavily on practitioner-oriented texts and techniques, such as design-thinking guides developed by IDEO, Ries’s lean startup methodology, Osterwalder’s business model canvas, and similar tools (Osterwalder and Pigneur, 2010; Ries, 2011; IDEO.org, 2015). Despite supposedly being rooted in academic research methods, these resources pay little attention to the logic and rigor underpinning these methods. Indeed, innovation is often portrayed as something that can be – and often is – taught in a day or less. Consistent with such views, programs also tend, implicitly or explicitly, to suggest the most effective way to learn about innovation is to just ‘dive in’. This study highlights the potential dangers of teaching innovation via quick and dirty approaches and failing to address the underlying logic associated with inductive qualitative research and experimental research designs.
The dangers of current pedagogy should perhaps not surprise us, given that academics spend years in doctoral programs developing capabilities with research methodologies, in part to avoid generating poorly grounded theories or reaching inappropriate conclusions. This reality suggests the potential value of rethinking how we talk about and teach innovation. First, we should be more thoughtful and explicit about the relationship between speed and accuracy. Practitioners tend to be highly interested in speed – i.e., getting products to market quickly – which is thought to result in lower costs (in time and resources) and greater revenues ( Chen et al., 2012). Yet, often there may be a tradeoff between speed and accuracy. Indeed, this is a key reason why rigorous academic research often proceeds slowly. This study illustrates how the costs of being wrong can often outweigh the benefits of moving fast, a reality that could be more explicitly addressed in innovation pedagogy. Second, the findings suggest we might benefit by shifting from teaching innovation largely as a mindset or set of tools to a greater focus on the logic, strengths and weaknesses, and design considerations of relevant academic research methodologies. Admittedly, such a shift may mean that the pedagogy becomes more diffuse, difficult and time-consuming; however, if it helps address persistently high innovation failure rates, these costs might be justified. This shift may also require business school curricula more tightly integrated with what the rest of the university is doing. Perhaps all entrepreneurship and innovation majors (or all business majors?) should be required to take courses on research design and methods.
Future directions
As with most inductive, qualitative work, this study in some ways produces as many questions as answers. The multiple case study design and grounded theory approach were well-suited for developing exploratory insights; however, these insights require further development and testing. This should probably include further elaboration of the multiplicity concept. For example, in this case, the multiplicity revolved around the interests of end users and economic purchasers. In other contexts, other stakeholders and interests might also be salient – for example, such nonmarket stakeholders as environmental or social advocates (Driessen and Hillebrand, 2013). But multiplicity in such contexts is likely to create similar or greater challenges, and would have similar implications for the balance of theory building and theory testing. In addition to extending and refining the theory developed here, additional work is needed to test the theory, quantify its implications and identify its boundary conditions. Quantitative studies, including experimental designs that directly evaluate outcomes of different approaches to balancing theory building and theory testing, might help address such questions.
Conclusion
Despite the extensive attention researchers and practitioners have devoted to innovation, many would-be innovators continue to misunderstand innovation and ineffectively navigate market uncertainty. The result is persistently high innovation failure rates. This study builds new theory about the causes of, and potential solutions to, such failures. It distinguishes between theory building and theory testing approaches to navigating market ambiguity and uncertainty. It also suggests that the appropriate balance between these two approaches is determined, at least in part, by the degree of multiplicity in the innovation context (the number of, and relationships between, stakeholders and their innovation-relevant interests). Finally, the study highlights how the benefits of both theory building and theory testing approaches may emerge only if innovators have a strong understanding of the underlying methodological epistemologies and deploy the approaches with a rigor consistent with their academic applications.