INTRODUCTION
Employment, as outlined in Sustainable Development Goal 8 (SDG8), focuses on achieving productive employment and ensuring decent work opportunities for all individuals. However, individuals with disabilities face significant unemployment and job instability, largely driven by entrenched socioeconomic challenges such as poverty, social exclusion, and marginalization [1]. These concerning trends exacerbate and perpetuate the inequalities and discrimination experienced by this vulnerable population in the labor market, leading to enduring impacts on their life trajectories and overall economic progress. Globally, the employment rate of individuals with disabilities remains significantly lower compared to those without disabilities, with multiple barriers that reduce their access to quality job opportunities, career advancement, and fair wages [2]. This gap is not only a personal hardship but also a societal issue, as it leads to an underutilization of potential and hinders economic growth.
At the same time, in an era marked by growing inequality, AI technologies have rapidly gained prominence, emerging as a key priority in the global market and a transformative tool for enhancing the quality of life [3, 4]. While AI holds promise in supporting people with disabilities through assistive technologies and flexible employment models, it can pose complex challenges. The rapid advancement of AI has introduced new skill requirements and raised concerns about potential biases within automated hiring processes, which may inadvertently exclude candidates with disabilities [5–7]. This dual effect of AI on employment for individuals with disabilities underscores the need to evaluate how governance frameworks moderate AI’s influence, ensuring that technological advancements support, rather than hinder, inclusive labor markets.
Empirical studies on AI’s impact on employment reveal various positive and negative outcomes, with some studies suggesting inconclusive effects. For instance, using data from highly technologically developed nations, Guliyev et al. [8] showed a negative association between various indicators of AI and unemployment. Specifically, the study observed that increases in the Google Trends Index for AI-related technologies correlate with declining unemployment rates, suggesting that AI can foster economic growth by improving productivity and generating new opportunities for jobs. Similarly, Yang [9] examined the impacts of AI on firm productivity and employment, concluding that AI increases productivity and employment in Taiwan. This aligns with Aghion et al. [10], who investigated the role of AI and automation in increasing employment in France, noting that robotization reduced employment, especially for workers with lower educational levels. These studies suggest that AI may increase employment under specific conditions, though its impact can vary based on policy contexts and workforce characteristics. In contrast, other studies have found no significant association between AI and employment. Felten et al. [11] highlighted that while AI technologies led to wage increases, they did not necessarily affect employment rates. Likewise, Acemoglu and Restrepo [12] studied the impacts of industrial robots on wages in the United States, demonstrating a negative association between both variables. In India, Alexiadou [1] found that AI-driven technologies can directly improve accessibility in the workplace for disabled individuals. Also, AI-powered assistive devices, speech recognition tools, and adaptive technologies make tasks easier to perform, helping disabled individuals. They underscored that while the potential of AI for enhancing accessibility is substantial, it also presents emerging legal challenges, particularly in countries like India, where regulatory frameworks must adapt to these technologies. The authors advocated for policies that ensure AI advancements in disability support remain inclusive, emphasizing the importance of a human rights framework in guiding future AI applications for this demographic. These studies reveal the diverse and complex impacts of AI on employment. Yet, a critical gap remains in understanding how AI affects employment opportunities for people with disabilities, especially through nonlinear and conditional pathways. Most research has not examined how AI may enhance or hinder employment for this group. Addressing this gap is essential, as it could uncover critical insights into the conditions under which AI contributes positively to employment for individuals with disabilities and where it may unintentionally create new barriers. This area remains significantly underexplored, signaling an urgent need to examine the interaction between AI advancements, governance quality, and employment inclusivity for disabled individuals.
Effective governance is increasingly recognized as a critical factor in ensuring that AI’s potential benefits are maximized and its adverse effects minimized, especially for the employment of people with disabilities. Governance quality, encompassing economic, political, and institutional dimensions, plays a pivotal role in shaping inclusive labor markets by fostering equitable access to AI-driven opportunities. For Brazil, Russia, India, China, and South Africa (BRICS) countries, Saba and Ngepah [13] demonstrated the importance of government intervention in aligning AI development with job creation and economic growth. The study shows that by prioritizing AI integration into governance, BRICS nations can enhance short- and long-term employment outcomes. Yet, the authors caution that AI may not contribute positively to employment without careful attention to the interplay between AI and good governance. They urged BRICS governments to implement policies that promote the development of AI while addressing potential challenges arising from interactions with political and institutional governance. Similarly, Knox [14] highlighted the role of good governance in supporting AI-driven educational initiatives in China, where policies that support AI innovation while providing regulatory clarity have fostered skill development on a national scale. This research emphasizes that the positive effect of AI on educational and employment outcomes depends significantly on adaptive, transparent, and inclusive governance structures. Governance, therefore, should not be viewed merely as a mitigating factor but as a proactive driver of inclusivity. By establishing robust regulatory frameworks, promoting equitable labor policies, and ensuring transparency in AI adoption, governance can actively shape the labor market to be more inclusive for people with disabilities. Economic governance, through measures such as government effectiveness and regulatory quality, can create an enabling environment for AI technologies that support accessible employment. Institutional governance, including the rule of law and control of corruption, ensures that labor markets remain fair and transparent, reducing discrimination and fostering equitable opportunities. Political governance, while less directly impactful, provides the stability and accountability necessary for the broader acceptance and implementation of inclusive policies.
By filling these gaps, this article makes several key contributions to understanding and optimizing the effect of AI on employment for people with disabilities in developed countries from 2010 to 2022, particularly emphasizing the role of governance as a proactive enabler of inclusivity. First, unlike previous studies, it examines both the linear and nonlinear impacts of AI on employment for disabled people, recognizing that developed countries present a unique context for AI implementation due to their advanced technological infrastructure, substantial resources, and comprehensive regulatory frameworks. By analyzing both direct and complex effects, the study provides a nuanced understanding of how AI can support or hinder employment inclusivity for disabled individuals. This approach moves beyond a simplistic view of AI as universally beneficial or detrimental. Second, this article explores how different governance dimensions—economic, political, and institutional—can actively shape the effects of AI on employment for disabled people, amplifying its positive impacts while mitigating potential adverse outcomes. Economic governance includes policies around investments and incentives for inclusive AI development, while political governance covers the legal protections and frameworks that prevent discrimination and ensure accessibility. Institutional governance refers to the structures within organizations and governments that uphold these standards. By investigating how these governance facets interact with AI deployment, the study sheds light on the conditions that allow AI to serve as an inclusive tool rather than an exclusionary force. This examination underscores the importance of a holistic governance approach, where economic, political, and institutional factors are aligned to maximize AI’s social benefits for people with disabilities. Third, the study addresses these impacts for specific groups within the disabled labor force, focusing on differences between male and female disabled workers. This gendered analysis is precious as it recognizes that AI may affect subgroups within the disabled community in distinct ways, shaped by underlying societal and workplace inequalities. By disaggregating data for male and female disabled workers, the study can reveal how gender influences the accessibility of AI-driven employment opportunities, providing insights that can inform targeted policies to ensure equitable AI benefits across demographic lines. This approach adds depth to the analysis and supports the development of more tailored strategies, enabling policymakers and stakeholders to address both gender and disability considerations in AI governance.
The article proceeds as follows. The Materials and Methods section details the methodology and data, focusing on analyzing AI’s linear, nonlinear, and conditional impacts on employment for disabled individuals under various governance dimensions. The Results and Discussions section presents and discusses the results. The Conclusion and Implications section concludes with policy recommendations for enhancing inclusive AI practices in employment.
MATERIALS AND METHODS
Model specifications and research method
Sustainable Development Goal 8 aims to achieve full and productive employment and decent work for all. In this context, AI has a transformative effect by enhancing productivity, creating new job opportunities, and improving labor market practices. Thus, a theoretical model can be developed to explore the direct impacts of AI on employment dynamics among people with disabilities:
where Ejit (0, 1, and 2) is the total employment of people with disabilities, employment of men with disabilities, and employment of women with disabilities. Z is the vector of control variables, which includes gross domestic product (GDP) growth as a measure of economic growth, government spending, education quality, and inflation. λ represents the coefficient of the AI impacting employment among disabled people. Economic growth is widely recognized as a key driver of employment. Higher growth typically leads to more demand for goods and services, which, in turn, creates jobs. According to Aghion et al. [15], GDP growth fosters job creation by stimulating investment and consumption. Government expenditure, especially in infrastructure, health, and education, tends to boost employment directly and indirectly by creating public-sector jobs and encouraging private-sector growth. Easterly and Rebelo [16] highlighted that infrastructure investments improve productivity and employment in emerging economies. Similarly, Pereira and Andraz [17] found that government spending on infrastructure creates significant employment multipliers, particularly in regions with underdeveloped infrastructure. Moreover, education quality, particularly beyond the basic level, allows persons with disabilities to acquire specialized skills that raise the likelihood of employment and job retention. For example, education creates opportunities in technology-driven and knowledge-based sectors where activities involve making provisions for various abilities. This observation is supported by Charlot and Malherbet [18], who point out that investment in education indirectly encourages employment through attempts at creating private-sector activity and improving individual productivity. However, inflation can undermine such gains through eroded real incomes and reduced purchasing power, hence sluggish demand for goods and services. It is under these conditions that unemployment might deteriorate even more, as it may affect vulnerable groups, for example, people with disabilities, who face some obstacles systemically in finding a job.
As mentioned in the Introduction section, this study also seeks to demonstrate the nonlinearity relationship between AI and employment for people with disabilities. Therefore, Equation (1) can be rewritten as follows:
This equation indicates that the impact of AI on the employment of disabled people may vary depending on the level of AI advancement. At the early stages of AI integration, the effect on employment could be negative or neutral (∂AI it /∂E it ≤ 0), meaning that initial AI improvements might reduce or have no effect on employment opportunities for this group. This could be due to factors like automation replacing certain jobs or accessibility barriers in early AI implementations. However, once AI development surpasses a certain threshold, its influence shifts positively (∂AI2/∂E > 0), suggesting that more advanced AI may improve employment opportunities. This shift could occur as AI becomes more sophisticated, inclusive, and better equipped to support diverse employment needs, potentially creating accessible job roles and assisting people with disabilities in overcoming work-related challenges.
The effects of AI on the employment of disabled people, both linear and nonlinear, indicate that specific thresholds are necessary to realize significant effects. As outlined by Alfehaid et al. [19], this threshold can be determined using Equation (3):
To examine the possibly modulating effect of good governance on the linkage between AI and employment among disabled persons (see Fig. 1), we rewrite Equation (1) as follows:
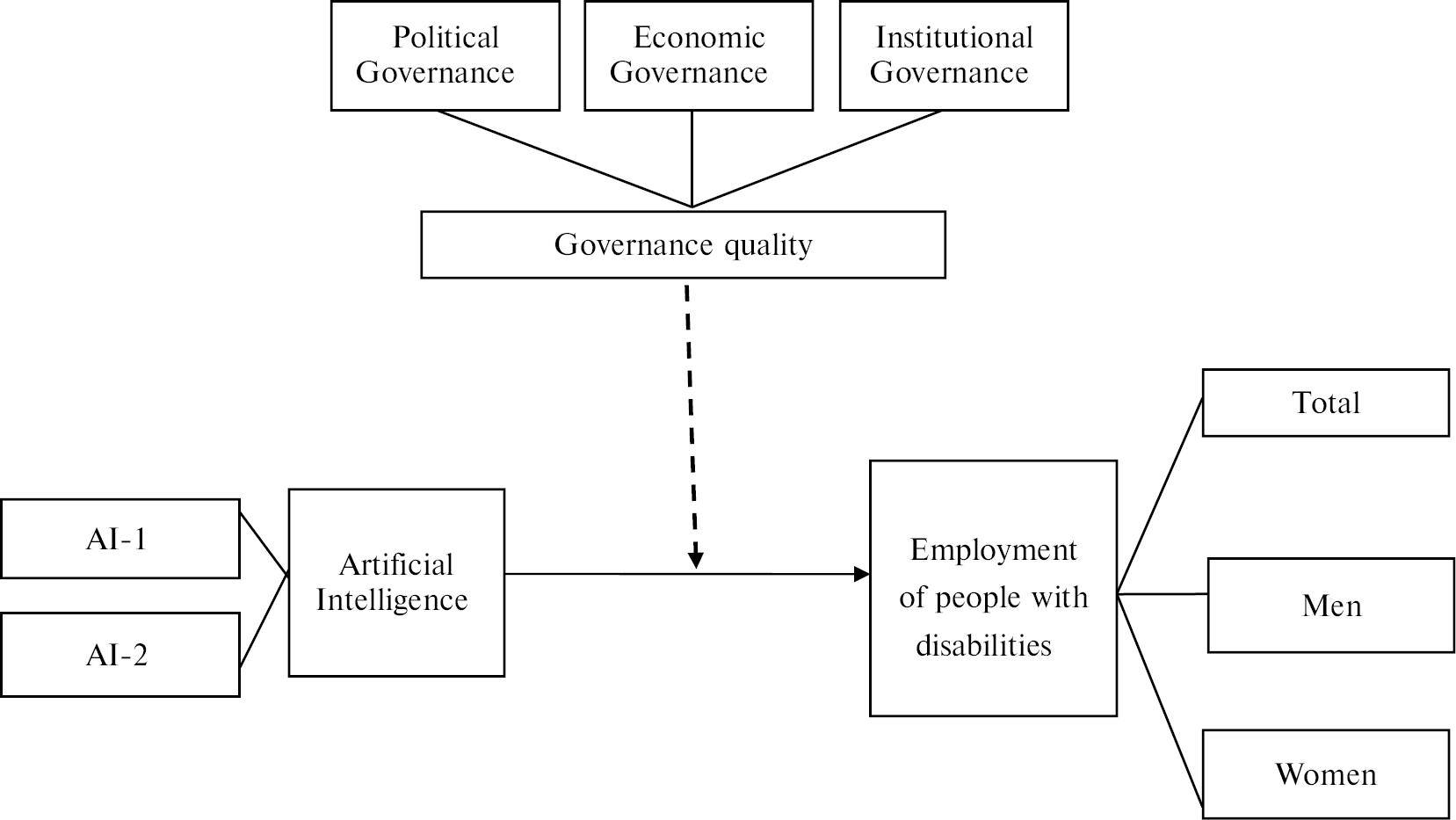
A theoretical model illustrating the moderating effect of government dimensions on the relationship between AI and employment outcomes for individuals with disabilities. Abbreviation: AI, artificial intelligence.
where Gov denotes governance quality (economic governance, political governance, and institutional governance), AI * Gov is the interaction between AI and the three dimensions (economic, political, and institutional) of governance. As argued above, we expect these three dimensions to complement AI and increase employment among people with disabilities. π is the coefficient of this moderating effect. By differentiating Equation (4) by AI, we obtain Equation (5) as follows:
where ∂ denotes the partial derivative operator. A one-unit increase in the employment rate, influenced by changes in AI, is contingent upon the signs and values of λ and π. The overall impact can be evaluated only if both the direct and indirect coefficients are significant and show opposite directions:
Equation (5) is only feasible if λ and π have opposite signs and are both statistically significant, where Ω is the average of the policy moderating variable. If these conditions are satisfied, a policy threshold for the moderating variable, as illustrated in Equation (6), exists such that:
Therefore, the retained threshold is as specified in Equation (7):
The specified threshold is calculated only if the values lie within the range of the moderating variable outlined in the summary statistics in Table 2.
STATISTICAL METHOD AND DATA ANALYSIS
The statistical method used in the analysis is the Augmented Mean Group (AMG) method, a two-stage panel estimator developed by Eberhardt [20], which accounts for cross-sectional dependence (CD) and slope heterogeneity. Before applying this method, we initiated our empirical analysis by testing for CD to detect any potential correlation across entities. Recognizing the possibility of bias in standard CD tests, we implemented the bias-adjusted LMadj test suggested by Pesaran et al. [21], which is particularly suited for large panels. The CD is essential in panel data settings, as it influences subsequent methodological choices. Next, before applying conventional panel methodologies, we evaluated the validity of the slope homogeneity assumption, as maintaining homogeneity is essential for obtaining unbiased estimates in heterogeneous panel data contexts. Pesaran and Yamagata [22] introduced the delta tilde-adjusted ( ˜Δadj ) and delta tilde ( ˜Δ ) tests to assess slope homogeneity, which we employed to confirm whether the assumption held for our panel. Testing for CD and slope homogeneity ensures we approach the data with the correct assumptions and methodological robustness.
Conventional panel cointegration methods often overlook CD in the data. To address this limitation, the AMG estimator, developed by Eberhardt [20], effectively accounts for CD, making it a more suitable approach for panels where dependence across units is present. The AMG method accommodates slope heterogeneity and CD, producing reliable results even if the variables are not cointegrated. This flexibility eliminates the need for preliminary unit root and cointegration tests before applying the AMG method [23, 24]. Moreover, the AMG method efficiently tackles potential endogeneity issues and offers reliable estimates to determine whether the relationships are consistent or vary across units [25]. It involves a two-stage process. In the first stage, it estimates the model by calculating the first differences of the variables, capturing changes between consecutive periods. Additionally, time dummies are included to control for time-specific effects consistent across all entities in the dataset, helping to account for standard shocks or trends affecting all units simultaneously:
where ∆ represents the first-difference operator, capturing the change in a variable across consecutive time periods. Here, Yit and xit denote the dependent and independent variables, respectively. Time dummy variables, Dt , are included to control for economy-wide shocks, with δt as the associated coefficients. In the second stage, the δt coefficients derived from the time dummies are transformed into a new variable, θi , as specified in Equation (11). This two-stage approach enables the model to incorporate heterogeneity through individual slopes αi while also addressing unobserved CD by using the constructed θi variables derived from the time dummies.
The empirical analysis is based on a sample of 25 developed countries, including Austria, Belgium, Czech Republic, Denmark, Estonia, Finland, France, Germany, Greece, Hungary, Ireland, Italy, Latvia, Lithuania, Netherlands, Norway, Poland, Portugal, Slovakia, Slovenia, Spain, Sweden, Switzerland, UK, and the USA. The study focuses on the period from 2010 to 2022, a timeframe chosen to capture significant advancements in AI and evolving governance structures in developed economies. The dependent variable is the employment rate of people with disabilities. Employment impacts were also measured by gender: employment rate of men with disabilities and employment rate of women with disabilities. These metrics are expressed as the percentage of employed individuals with disabilities in the total labor force, sourced from the International Labour Organization (ILOSTAT). The key independent variables include two proxies for AI activity: (i) annual patent applications related to AI (AI-1), reflecting innovation and technological progress, and (ii) the number of industrial robots in use (AI-2), capturing industrial automation. Data for these variables were obtained from Our World in Data (OWID) and the International Federation of Robotics (IFR), respectively. These variables collectively represent both the development and adoption aspects of AI technologies. Moreover, governance quality serves as a moderator variable in this study, measured across three dimensions: economic governance (government effectiveness and regulatory quality), political governance (voice and accountability and political stability), and institutional governance (rule of law and control of corruption). These indicators are sourced from the World Governance Indicators (WGI). Control variables were included to account for other factors influencing employment outcomes. These are economic growth [GDP growth, sourced from the World Development Indicators (WDI)], government spending (general government final consumption expenditure as a percentage of GDP, sourced from WDI), educational attainment (gross tertiary school enrollment, sourced from WDI), and inflation (growth rates of the annual consumer price index, sourced from WDI). All datasets supporting this study’s findings are accessible from ILOSTAT, OWID, IFR, and WGI. Direct links to the sources are provided in Table 1. This study did not involve human or animal subjects, and thus, ethical approval was not required. Countries with missing data values exceeding 10% were excluded from the analysis.
Critical resources, data sources, and software, including RRIDs and links.
Resource | Description | RRID/link |
---|---|---|
STATA v.17.0 | Statistical software used for AMG method | RRID: SCR_012763 |
ILOSTAT | Employment data for people with disabilities | https://ilostat.ilo.org |
Our World in Data (OWID) | AI-related patent data | https://ourworldindata.org |
International Federation of Robotics (IFR) | Data on industrial robots | https://ifr.org |
World Governance Indicators (WGI) | Governance quality metrics | https://worldbank.org |
Table 2 details all variables’ measures, sources, and summary statistics, ensuring a comprehensive approach to analyzing the interplay between AI, governance, and employment outcomes for people with disabilities. Data were analyzed using the STATA software. This table shows that, for the dependent variable, the total employment rate of people with disabilities has a mean of 2.204, indicating a relatively low but variable labor force participation. The standard deviation of 2.848 highlights substantial disparities across the sampled countries, with values ranging from a minimum of 0.059 to a maximum of 25.803. When disaggregated, the employment rate for men with disabilities shows a mean of 1.121 and a range of 0.036-14.598, while women with disabilities exhibit slightly lower figures, with a mean of 1.091 and a range of 0.024-11.204. These differences underline the gender disparities in employment among people with disabilities. For the independent variables, the first proxy for AI, AI-1 (annual patent applications related to AI), has a mean of 8.567, with a standard deviation of 2.183, demonstrating moderate innovation activity across the countries. The values range from a minimum of 1.946 to a maximum of 12.807, indicating variability in AI technological development. The second proxy, AI-2 (industrial robots), reveals significant disparities, with a mean of 408.426 and a very high standard deviation of 1884.228. The range, from 1 to 13,873, reflects the uneven adoption of robotics across industries. The moderator variable, governance quality, reveals diverse performance across its dimensions. Economic governance shows means of 1.238 and 1.190, respectively, with ranges indicating disparities in administrative and regulatory capacities (0.124-2.235 for government effectiveness and 0.135-1.900 for regulatory quality). Political governance variables, such as voice and accountability (mean: 1.132, range: 0.259 to 1.781) and political stability (mean: 0.694, range: −0.318 to 1.620), highlight variability in democratic processes and stability. Institutional governance measures, like the rule of law (mean: 1.190, range: −0.159 to 2.125) and control of corruption (mean: 1.100, range: −0.381 to 2.403), underscore governance quality disparities.
Variables, measurements, sources, and summary statistics.
Variables | Unit of measurement | Signs on | Sources | Summary statistics | |||||
---|---|---|---|---|---|---|---|---|---|
E0it | E1it | E2it | Mean | Std. Dev. | Min. | Max. | |||
Total employment rate of people with disabilities | Employed people with disabilities as a share of the total labor force | ILOSTAT | 2.204 | 2.848 | 0.059 | 25.803 | |||
Employment rate of men with disabilities | Employed men with disabilities as a share of the total labor force | ILOSTAT | 1.121 | 1.524 | 0.036 | 14.598 | |||
Employment rate of women with disabilities | Employed women with a disability as a share of the total labor force | ILOSTAT | 1.091 | 1.380 | 0.024 | 11.204 | |||
AI | AI-1: Annual patent applications related to AI | (+/−) | (+/−) | (+/−) | OWID | 8.567 | 2.183 | 1.946 | 12.807 |
AI-2: Industrial robots | (+/−) | (+/−) | (+/−) | IFR | 408.426 | 1884.228 | 1 | 13,873 | |
Economic governance | Government effectiveness | (+) | (+) | (+) | WGI | 1.238 | 0.502 | 0.124 | 2.235 |
Regulatory quality | (+) | (+) | (+) | WGI | 1.190 | 0.449 | 0.135 | 1.900 | |
Political governance | Voice and accountability | (+) | (+) | (+) | WGI | 1.132 | 0.359 | 0.259 | 1.781 |
Political stability | (+) | (+) | (+) | WGI | 0.694 | 0.395 | −0.318 | 1.620 | |
Institutional governance | Rule of law | (+) | (+) | (+) | WGI | 1.190 | 0.603 | −0.159 | 2.125 |
Control of corruption | (+) | (+) | (+) | WGI | 1.100 | 0.795 | −0.381 | 2.403 | |
Economic growth | GDP growth (annual %) | (+) | (+) | (+) | WDI | 1.780 | 3.471 | −11.6 | 23.305 |
Government spending | General government final consumption expenditure (% of GDP) | (+) | (+) | (+) | WDI | 11.490 | 2.172 | 7.112 | 16.257 |
Educational attainment | School enrollment, tertiary (% gross) | (+) | (+) | (+) | WDI | 78.631 | 3.808 | 66.683 | 86.627 |
Inflation | Annual growth rate of the consumer price index | (−) | (−) | (−) | WDI | 2.172 | 2.891 | −1.736 | 19.705 |
Abbreviations: E0it , E1it , and E2it , the total employment rate of people with disabilities, the employment rate of men with disabilities, and the employment rate of women with disabilities, respectively; IFR, International Federation of Robotics; ILOSTAT, International Labour Organization; WGI, Word Governance Indicators; WDI, World Development Indicators.
RESULTS AND DISCUSSIONS
The empirical analysis begins with checking for CD to verify the robustness and validity of the results. That is an important aspect, given that panel data analysis should not find any reason to address this matter lest it yield biased estimates and untrustworthy statistical inferences. It does appear from adjusted Lagrange multiplier (LMadj) test results in all models shown in Table 3 that there is statistically significant CD: Model AI-1 and AI-2 LMadj values were between 19.422 and 66.427 and between 18.839 and 44.046, respectively; at the same time, reported P values were statistically significant at the 1% level, thus showing interdependencies among the variables involved in the study and postulating an analytical framework integrating the feature of cross-sectional dependency to infer and arrive at valid and sound results. Beyond this, the homogeneity test should be essential to understand the variation in the relationships across contexts. All models show a statistically significant P value at a 1% significance level against the null hypothesis of homogeneous slopes in the results of the homogeneity tests in Table 4. For example, the adjusted test statistics for Model AI-1 vary between 9.037 and 29.161, indicating highly heterogeneous slope coefficients. Such heterogeneity of slopes, in fact, captures varied relationships between the dependent and independent variables across entities. To address these issues, robust estimation methods, such as the AMG estimator. Data analysis was performed in STATA (RRID: SCR_012763). This approach ensures reliable estimates despite CD and slope variability.
Results of cross-sectional dependence analysis.
Test | Stat. | P values | |
---|---|---|---|
E0it model | |||
Model AI-1 | LMadj | 19.422* | (0.000) |
Model AI-2 | LMadj | 24.220* | (0.000) |
E1it model | |||
Model AI-1 | LMadj | 66.427* | (0.000) |
Model AI-2 | LMadj | 44.046* | (0.000) |
E2it model | |||
Model AI-1 | LMadj | 21.100* | (0.000) |
Model AI-2 | LMadj | 18.839* | (0.000) |
Statistical significance (P values) is reported in parentheses.
Abbreviation: LMadj, adjusted Lagrange multiplier test.
*Statistical significance at a 1% level.
Results of the homogeneity test.
Test | Stat. | P values | |
---|---|---|---|
E0it model | |||
Model AI-1 | ˜Δ | 9.037* | (0.000) |
˜Δadj | 11.133* | (0.000) | |
Model AI-1 | ˜Δ | 15.948* | (0.000) |
˜Δadj | 21.031* | (0.000) | |
E1it model | |||
Model AI-1 | ˜Δ | 23.559* | (0.000) |
˜Δadj | 29.161* | (0.000) | |
Model AI-1 | ˜Δ | 15.920* | (0.000) |
˜Δadj | 19.231* | (0.000) | |
E2it model | |||
Model AI-1 | ˜Δ | 16.445* | (0.000) |
˜Δadj | 19.226* | (0.000) | |
Model AI-1 | ˜Δ | 13.049* | (0.000) |
˜Δadj | 17.112* | (0.000) |
Statistical significance (P values) is reported in parentheses.
Abbreviations: ˜Δ , delta tilde; ˜Δadj , delta tiled-adjusted.
*Statistical significance at 1% level.
Table 5 highlights the AI’s linear and nonlinear effects on employment by people with disabilities—total employment, employed men, and employed women. The linear results show that AI-1 (annual patent applications related to AI) and AI-2 (industrial robots) have statistically significant negative effects on employment. Specifically, in the case of total employment, AI-1 decreases employment by 0.179% (P < 0.01), while in the case of AI-2, a reduction of 0.144% (P < 0.01) is recorded. Similarly, a similar trend is noticed for employed men and women with disabilities; AI-1 decreases by 0.202% and 0.130%, while AI-2 decreases their employment by 0.207% and 0.130%. The outcome means that early adoption of AI technologies tends to substitute employment among people with disabilities because automation is replacing some jobs that had been performed by this population traditionally. Then, comparing both sexes in these results indicates that the initial adoption of AI technologies disproportionately affects men with disabilities compared to women, likely due to their higher representation in sectors more susceptible to automation, such as manufacturing and physical labor-intensive industries. In the case of employed women with disabilities, the slightly lower negative linear effects of AI-1 and AI-2 relative to men could reflect that among employed women with disabilities, their concentration is in activities or industries less immediately affected by AI-induced automation—for example, caregiving, education, and service-oriented industries. This only suggests a slightly reduced impact but does not dent the broader finding of substantial displacement effects from AI adoption across both sexes. The negative impact of AI on employment confirms that advancements in AI fundamentally reshape labor markets by reducing the demand for low-skilled labor while simultaneously fostering structural imbalances. Smith and Anderson [26] highlighted that nearly half of experts in the technology field believe robots can replace most standardized and programmed tasks, putting numerous jobs at risk. This aligns with the findings of Acemoglu and Restrepo [27], who noted that while AI creates new, complex roles in emerging industries, it simultaneously eliminates traditional labor-intensive jobs. These transitions are particularly harsh on low-skilled workers, as evidenced by Grartz and Michaels [28], who observed that robots disproportionately displace tasks performed by low-skilled labor, compared to their medium- and high-skilled counterparts. In the same vein, the study by Humlum [29] on Denmark reinforces this point, showing that the use of industrial robots led to job losses among traditional production workers while creating opportunities for skilled workers. Similarly, Cai and Chen [30] assert that AI-driven technological advancements, as part of the fourth industrial revolution, favor high-skilled labor, leaving low-skilled workers at a significant disadvantage and deepening structural labor market inequalities.
Nonlinear effects of artificial intelligence on employment for people with disabilities.
Total employment ( E0it ) | Employed men ( E1it ) | Employed women ( E2it ) | ||||
---|---|---|---|---|---|---|
AI-1 | AI-2 | AI-1 | AI-2 | AI-1 | AI-2 | |
AI-1 | −0.179* (0.002) | −0.151* (0.000) | −0.202* (0.000) | |||
AI-2 | −0.144* (0.000) | −0.130** (0.021) | −0.207* (0.000) | |||
AI-12 | 0.127** (0.011) | 0.117* (0.001) | 0.135* (0.000) | |||
AI-22 | 0.021* (0.000) | 0.019* (0.000) | 0.024* (0.000) | |||
Thresholds | 11.098 | 360.544 | 11.030 | 360.110 | 11.083 | 359.375 |
Economic growth | 0.164* (0.000) | 0.177* (0.000) | 0.238* (0.000) | 0.259* (0.000) | 0.186* (0.000) | 0.193* (0.000) |
Government spending | 0.134** (0.018) | 0.154* (0.000) | 0.139* (0.000) | 0.147* (0.000) | 0.121* (0.000) | 0.134* (0.000) |
Education attainment | 0.109*** (0.053) | 0.112** (0.041) | 0.144* (0.000) | 0.151* (0.000) | 0.133** (0.026) | 0.119** (0.011) |
Inflation | −0.099 (0.127) | −0.103 (0.102) | −0.083 (0.176) | −0.089 (0.181) | −0.072 (0.209) | −0.109 (0.104) |
Constant | 0.682 (0.000) | 1.029 (0.000) | 1.238 (0.000) | 1.482 (0.000) | 0.837 (0.000) | 0.965 (0.000) |
Statistical significance (P values) is reported in parentheses.
*,**,***Statistical significance at 1%, 5%, and 10% levels, respectively.
However, the squared terms of AI-1 and AI-2 reveal positive and significant coefficients, indicating a nonlinear relationship between AI and the employment of people with disabilities. For example, AI-12 increases total employment by 0.127% (P < 0.05), and AI-22 raises employment by 0.021% (P < 0.01), suggesting that at higher levels of AI adoption, these technologies begin to enhance employment opportunities. The same pattern is observed for employed men and women, where the squared terms of AI-1 and AI-2 show significant positive impacts, indicating a quadratic relationship, where the impact of AI on employment initially declines but eventually stabilizes or even reverses, becoming positive at higher levels of AI adoption. The positive coefficients of the squared AI term indicate that as AI advances beyond a certain threshold, its influence transitions from reducing employment to enhancing it. This threshold determines the point under which the quadratic relationship between AI and employment shifts from negative to positive. Recognizing these thresholds enables policymakers to develop strategies for AI adoption that optimize its benefits while mitigating initial adverse effects. The results in Table 5 indicate that the thresholds for AI-1 are around 11 annual patent applications related to AI, and for AI-2, around 360 industrial robots. These thresholds fall within the ranges reported in the summary statistics, providing important policy implications and confirming the nonlinear impact of AI on employment outcomes for individuals with disabilities. The findings suggest that increasing AI adoption enhances employment opportunities up to these thresholds, highlighting the need for balanced AI integration strategies to optimize its benefits while minimizing potential negative effects at lower levels of adoption. These results align with Abid et al. [31], who found a nonlinear relationship between AI and unemployment, revealing that AI’s impact on unemployment among people with disabilities depends on surpassing specific thresholds. Their findings underscore the importance of targeted policy interventions that promote AI adoption while ensuring inclusive access to its benefits. These findings suggest that achieving significant employment gains through AI requires investments that enable economies and demographic groups to surpass critical adoption thresholds, fostering a more equitable distribution of AI-driven opportunities. Similarly, Mutascu [32] demonstrates a nonlinear relationship between AI and unemployment using a panel threshold regression model, with inflation as the threshold variable. The study finds that AI significantly reduces unemployment when inflation is below the threshold by enhancing productivity and creating new job opportunities. However, when inflation surpasses the threshold, the impact of AI on unemployment becomes neutral or inconclusive. This highlights the conditional nature of AI’s influence, emphasizing the importance of stable macroeconomic conditions to fully realize its employment benefits. For this reason, this study also aims to demonstrate the conditional impact of governance quality to ensure that AI positively contributes to employment. A critical gap exists in understanding how governance quality and AI adoption interact to outline employment for individuals with disabilities. Specifically, the instruments underlying the complementarity effects of these factors on inclusive employment creation remain largely unexplored.
The control variables—economic growth, government spending, educational attainment, and inflation—exhibit varying degrees of influence on the three employment variables: total employment of people with disabilities, employment of men, and employment of women. Economic growth positively and significantly affects all three employment variables, with coefficients indicating that higher GDP growth correlates with increased employment opportunities for individuals with disabilities. For example, economic growth contributes 0.164% to total employment, 0.238% to male employment, and 0.186% to female employment. These results align with the notion that economic expansion fosters job creation, benefitting diverse demographic groups, including those with disabilities. Government expenditure also positively influences employment rates across all groups. The coefficients for government spending are slightly lower than those for economic growth, yet still significant, highlighting its role in providing public-sector jobs and enabling programs that support the employment of disabled individuals. For example, government spending increases total employment by 0.134%, male employment by 0.139%, and female employment by 0.121%. Similarly, educational attainment demonstrates a consistent positive impact on employment outcomes for all three groups. This variable’s influence is particularly pronounced in male employment, contributing 0.144%. For women and total employment, the contributions are 0.133% and 0.109%, respectively. These results underscore the importance of education in equipping individuals with disabilities with the skills needed to compete in the labor market, particularly in an AI-driven economy. In contrast, inflation shows a negative and statistically insignificant impact across all three employment variables, with coefficients like −0.099% for total employment and −0.083% for male employment. This suggests that inflationary pressures may not significantly hinder employment rates for people with disabilities in the studied context, but it might indirectly affect real wages and living standards. Overall, these findings highlight the importance of fostering economic growth, increasing government spending in employment-supporting initiatives, and enhancing educational opportunities to improve employment outcomes for individuals with disabilities while mitigating the adverse effects of inflation.
As discussed above, this study addresses a gap in the existing literature by examining how differences in governance quality levels can either worsen or mitigate the employment challenges associated with AI adoption. By addressing this gap, the article aims to offer deeper insights into the complex interactions between governance quality and AI, enabling policymakers to design more effective interventions and strategies to foster inclusive labor markets and support the professional integration of individuals with disabilities. By examining how governance quality—economic, political, and institutional measures—moderates the relationship between AI and the employment of people with disabilities, the study aims to highlight the importance of supportive governance structures in this relationship. The net effects of each dimension of governance (economic, political, and institutional) and AI (AI-1 and AI-2) on the total employment of people with disabilities, employment of men with disabilities, and employment of women with disabilities are reported in Tables 6–8, respectively. In Table 6, which focuses on total employment, the interplay between AI and economic governance shows significant positive effects. For example, the net effect of AI-1 combined with government effectiveness is 0.017 [(0.148 * 1.238) + (−0.166)], and with regulatory quality, it is 0.014 [(0.134 * 1.190) + (−0.145)]. In these formulas, 1.238 and 1.190 are the mean values of government effectiveness and regulatory quality, respectively, and 0.148 and 0.134 are the marginal effects on the total employment of people with disabilities from increasing government effectiveness and regulatory quality; −0.166 and −0.145 are the unconditional impacts of AI on the total employment of people with disabilities. Similarly, AI-2’s interaction with government effectiveness and regulatory quality yields, respectively, net effects of 0.016 and 0.018, reflecting that stronger economic governance enhances AI’s positive employment impact. Institutional governance, measured by the rule of law and control of corruption, also shows positive net effects. The net effect of AI-1 combined with the rule of law is 0.016, while AI-2 combined with control of corruption yields 0.019, indicating that institutional governance plays a vital role in strengthening the AI-employment nexus for total employment. However, interactions with political governance (voice and accountability, political stability) show statistically insignificant results, suggesting a limited influence on total employment. For the employment of men with disabilities, Table 7 shows that AI-1’s interaction with economic governance yields a net effect of 0.063 for government effectiveness, showcasing its strong moderating role. Similarly, AI-2 combined with regulatory quality shows a significant positive net effect of 0.051. Regarding institutional governance, the interaction of AI-1 with the rule of law results in a net effect of 0.043, while AI-2 combined with control of corruption produces 0.039. These results highlight that institutional governance enhances the employment opportunities for men by creating a transparent and equitable labor market environment. Table 8 focuses on the employment of women with disabilities, revealing that economic governance remains significant, with regulatory quality interacting positively with AI-1 (net effect equal to 0.021). However, institutional governance is even more prominent in enhancing female employment. The interaction of AI-1 with the rule of law produces a net effect of 0.023, while AI-2 combined with control of corruption shows a net effect of 0.017. These findings underscore that institutional governance mechanisms, such as anticorruption measures and the enforcement of legal frameworks, are crucial for promoting gender-inclusive employment in AI-driven labor markets.
Complementary effects of artificial intelligence and governance quality on total employment of people with disabilities.
Total employment of people with disabilities ( E0it ) | ||||||||||||
---|---|---|---|---|---|---|---|---|---|---|---|---|
AI-1 | AI-2 | |||||||||||
Economic governance | Political governance | Institutional governance | Economic governance | Political governance | Institutional governance | |||||||
GE | RQ | VA | PS | RL | CC | GE | RQ | VA | PS | RL | CC | |
AI-1 | −0.166* (0.000) | −0.145* (0.000) | −0.131** (0.020) | −0.129* (0.000) | −0.157* (0.000) | −0.161* (0.000) | ||||||
AI-2 | −0.137** (0.013) | −0.138* (0.000) | −0.151* (0.000) | −0.144* (0.000) | −0.149* (0.000) | −0.141* (0.000) | ||||||
Government effectiveness (GE) | 0.094*** (0.055) | 0.088** (0.021) | ||||||||||
Regulatory quality (RQ) | 0.101** (0.017) | 0.092*** (0.062) | ||||||||||
Voice and accountability (VA) | 0.076 (0.134) | 0.093 (0.117) | ||||||||||
Political stability (PS) | 0.058 (0.189) | 0.081 (0.127) | ||||||||||
Rule of law (RL) | 0.099 (0.112) | 0.101 (0.104) | ||||||||||
Control of corruption (CC) | 0.103** (0.027) | 0.091** (0.034) | ||||||||||
AI-1 * GE | 0.148* (0.000) | |||||||||||
AI-1 * RQ | 0.134** (0.012) | |||||||||||
AI-1 * VA | 0.102 (0.111) | |||||||||||
AI-1 * PS | 0.093 (0.137) | |||||||||||
AI-1 * RL | 0.141* (0.000) | |||||||||||
AI-1 * CC | 0.156* (0.000) | |||||||||||
AI-2 * GE | 0.124** (0.027) | |||||||||||
AI-2 * RQ | 0.131* (0.000) | |||||||||||
AI-2 * VA | 0.119 (0.102) | |||||||||||
AI-2 * PS | 0.127 (0.100) | |||||||||||
AI-2 * RL | 0.142* (0.000) | |||||||||||
AI-2 * CC | 0.155* (0.000) | |||||||||||
Net effects | 0.017 | 0.014 | n.a. | n.a. | 0.010 | 0.011 | 0.016 | 0.018 | n.a. | n.a. | 0.20 | 0.029 |
Economic growth | 0.203* (0.000) | 0.181* (0.000) | 0.226* (0.000) | 0.211* (0.000) | 0.194* (0.000) | 0.203* (0.000) | 0.179* (0.000) | 0.193* (0.000) | 0.202* (0.000) | 0.222* (0.000) | 0.191* (0.000) | 0.229* (0.000) |
Government spending | 0.129* (0.000) | 0.132* (0.000) | 0.121** (0.030) | 0.098*** (0.057) | 0.118*** (0.060) | 0.131* (0.000) | 0.127* (0.018) | 0.091** (0.022) | 0.127** (0.011) | 0.088** (0.027) | 0.130* (0.000) | 0.142* (0.000) |
Education attainment | 0.139* (0.000) | 0.161* (0.000) | 0.171* (0.000) | 0.193* (0.000) | 0.169* (0.000) | 0.181* (0.000) | 0.196* (0.000) | 0.204* (0.000) | 0.182* (0.000) | 0.159* (0.000) | 0.168* (0.000) | 0.199* (0.000) |
Inflation | −0.106 (0.114) | −0.094 (0.127) | −0.085 (0.138) | −0101 (0.122) | −0.093 (0.100) | −0.113 (0.101) | −0.069 (0.193) | −0.071 (0.150) | −0.088 (0.116) | −0.092 (0.121) | −0.099 (0.127) | −0.089 (0.147) |
Constant | 11.367* (0.000) | 6.330* (0.000) | 0.983* (0.000) | 2.603* (0.000) | 5.337* (0.000) | 1.449* (0.000) | 0.461* (0.000) | 1.820* (0.000) | 3.466* (0.000) | 9.387* (0.000) | 1.376* (0.000) | 2.447* (0.000) |
AI-1 and AI-2 are annual patent applications related to AI and industrial robots, respectively. The mean values of GE, RQ, VA, PS, RL, and CC are 1.238, 1.190, 1.132, 0.694, 1.190, and 1.100, respectively. Statistical significance (P values) is reported in parentheses.
Abbreviation: n.a., not applicable.
*,**,***Statistical significance at 1%, 5%, and 10% levels, respectively.
Complementary effects of artificial intelligence and governance quality on employment outcomes for men with disabilities.
Employment of men with disabilities ( E1it ) | ||||||||||||
---|---|---|---|---|---|---|---|---|---|---|---|---|
AI-1 | AI-2 | |||||||||||
Economic governance | Political governance | Institutional governance | Economic governance | Political governance | Institutional governance | |||||||
GE | RQ | VA | PS | RL | CC | GE | RQ | VA | PS | RL | CC | |
AI-1 | −0.158* (0.000) | −0.161* (0.000) | −0.141* (0.000) | −0.149* (0.000) | −0.160* (0.000) | −0.162* (0.000) | ||||||
AI-2 | −0.146* (0.000) | −0.153* (0.000) | −0.160* (0.000) | −0.150* (0.000) | −0.155* (0.000) | −0.137* (0.000) | ||||||
Government effectiveness (GE) | 0.107** (0.038) | 0.119* (0.000) | ||||||||||
Regulatory quality (RQ) | 0.094** (0.017) | 0.120* (0.000) | ||||||||||
Voice and accountability (VA) | 0.076 (0.134) | 0.116 (0.106) | ||||||||||
Political stability (PS) | 0.089 (0.121) | 0.096 (0.152) | ||||||||||
Rule of law (RL) | 0.123* (0.126) | 0.131* (0.003) | ||||||||||
Control of corruption (CC) | 0.117* (0.000) | 0.120** (0.015) | ||||||||||
AI-1 * GE | 0.179* (0.000) | |||||||||||
AI-1 * RQ | 0.155* (0.000) | |||||||||||
AI-1 * VA | 0.110 (0.129) | |||||||||||
AI-1 * PS | 0.106 (0.113) | |||||||||||
AI-1 * RL | 0.149* (0.000) | |||||||||||
AI-1 * CC | 0.140* (0.000) | |||||||||||
AI-2 * GE | 0.139* (0.000) | |||||||||||
AI-2 * RQ | 0.139* (0.000) | |||||||||||
AI-2 * VA | 0.077 (0.169) | |||||||||||
AI-2 * PS | 0.069 (0.204) | |||||||||||
AI-2 * RL | 0.144* (0.000) | |||||||||||
AI-2 * CC | 0.136** (0.012) | |||||||||||
Net effects | 0.063 | 0.023 | n.a. | n.a. | 0.009 | 0.005 | 0.026 | 0.012 | n.a. | n.a. | 0.016 | 0.013 |
Economic growth | 0.179* (0.000) | 0.192* (0.000) | 0.169* (0.000) | 0.203* (0.000) | 0.199* (0.000) | 0.229* (0.000) | 0.183* (0.000) | 0.171* (0.000) | 0.186* (0.000) | 0.194* (0.000) | 0.177* (0.000) | 0.291* (0.000) |
Government spending | 0.095*** (0.022) | 0.114** (0.010) | 0.099* (0.001) | 0.122* (0.000) | 0.137* (0.000) | 0.147* (0.000) | 0.141* (0.000) | 0.116** (0.020) | 0.131* (0.000) | 0.098** (0.044) | 0.110** (0.029) | 0.133* (0.002) |
Education attainment | 0.130* (0.000) | 0.147* (0.000) | 0.160* (0.000) | 0.172* (0.000) | 0.160* (0.000) | 0.166* (0.000) | 0.173* (0.000) | 0.188* (0.000) | 0.180* (0.000) | 0.163* (0.000) | 0.154* (0.000) | 0.170* (0.000) |
Inflation | −0.073 (0.159) | −0.102 (0.155) | −0.066 (0.179) | −0.103 (0.120) | −0.070 (0.122) | −0.102 (0.125) | −0.081 (0.166) | −0.070 (0.182) | −0.082 (0.133) | −0.089 (0.167) | −0.104 (0.105) | −0.081 (0.151) |
Constant | 0.837* (0.000) | 1.339* (0.000) | 0.662* (0.000) | 0.520* (0.000) | 0.927* (0.000) | 1.103* (0.000) | 0.938* (0.000) | 0.438* (0.000) | 0.551* (0.000) | 0.828* (0.000) | 1.584* (0.000) | 1.103* (0.000) |
AI-1 and AI-2 are annual patent applications related to AI and industrial robots, respectively. The mean values of GE, RQ, VA, PS, RL, and CC are 1.238, 1.190, 1.132, 0.694, 1.190, and 1.100, respectively. Statistical significance (P values) is reported in parentheses.
Abbreviation: n.a., not applicable.
*,**,***Statistical significance at 1%, 5%, and 10% levels, respectively.
Complementary effects of artificial intelligence and governance quality on employment outcomes for women with disabilities.
Employment of women with disabilities ( E2it ) | ||||||||||||
---|---|---|---|---|---|---|---|---|---|---|---|---|
AI-1 | AI-2 | |||||||||||
Economic governance | Political governance | Institutional governance | Economic governance | Political governance | Institutional governance | |||||||
GE | RQ | VA | PS | RL | CC | GE | RQ | VA | PS | RL | CC | |
AI-1 | −0.139** (0.013) | −0.144* (0.000) | −0.129** (0.027) | −0.138* (0.000) | −0.140* (0.000) | −0.139* (0.000) | ||||||
AI-2 | −0.133** (0.026) | −0.135* (0.000) | −0.146* (0.000) | −0.139* (0.000) | −0.133* (0.000) | −0.130* (0.000) | ||||||
Government effectiveness (GE) | 0.091** (0.033) | 0.110* (0.000) | ||||||||||
Regulatory quality (RQ) | 0.122* (0.000) | 0.117* (0.001) | ||||||||||
Voice and accountability (VA) | 0.088 (0.105) | 0.106 (0.100) | ||||||||||
Political stability (PS) | 0.074 (0.131) | 0.081 (0.117) | ||||||||||
Rule of law (RL) | 0.117** (0.026) | 0.109*** (0.050) | ||||||||||
Control of corruption (CC) | 0.112** (0.018) | 0.108** (0.013) | ||||||||||
AI-1 * GE | 0.126** (0.010) | |||||||||||
AI-1 * RQ | 0.133* (0.000) | |||||||||||
AI-1 * VA | 0.118 (0.104) | |||||||||||
AI-1 * PS | 0.102 (0.137) | |||||||||||
AI-1 * RL | 0.135* (0.000) | |||||||||||
AI-1 * CC | 0.129** (0.016) | |||||||||||
AI-2 * GE | 0.123* (0.000) | |||||||||||
AI-2 * RQ | 0.118** (0.035) | |||||||||||
AI-2 * VA | 0.089 (0.136) | |||||||||||
AI-2 * PS | 0.077 (0.159) | |||||||||||
AI-2 * RL | 0.126* (0.000) | |||||||||||
AI-2 * CC | 0.119** (0.021) | |||||||||||
Net effects | 0.017 | 0.014 | n.a. | n.a. | 0.021 | 0.003 | 0.019 | 0.005 | n.a. | n.a. | 0.017 | 0.001 |
Economic growth | 0.222* (0.000) | 0.251* (0.000) | 0.198* (0.000) | 0.211* (0.000) | 0.264* (0.000) | 0.186* (0.000) | 0.209* (0.000) | 0.199* (0.000) | 0.206* (0.000) | 0.214* (0.000) | 0.196* (0.000) | 0.230* (0.000) |
Government spending | 0.103** (0.027) | 0.134* (0.000) | 0.128* (0.001) | 0.142* (0.000) | 0.156* (0.000) | 0.140* (0.000) | 0.157* (0.000) | 0.139* (0.000) | 0.148* (0.000) | 0.133* (0.000) | 0.129* (0.001) | 0.140* (0.000) |
Education attainment | 0.161* (0.000) | 0.159* (0.000) | 0.181* (0.000) | 0.165* (0.000) | 0.186* (0.000) | 0.169* (0.000) | 0.192* (0.000) | 0.171* (0.000) | 0.165* (0.000) | 0.182* (0.000) | 0.172* (0.000) | 0.169* (0.000) |
Inflation | −0.057 (0.204) | −0.069 (0.181) | −0.048 (0.278) | −0.073 (0.137) | −0.051 (0.203) | −0.048 (0.246) | −0.072 (0.135) | −0.056 (0.212) | −0.049 (0.243) | −0.060 (0.185) | −0.069 (0.178) | −0.066 (0.173) |
Constant | 0.427* (0.000) | 0.331* (0.000) | 0.294* (0.000) | 0.321* (0.000) | 0.416* (0.000) | 0.539* (0.000) | 0.327* (0.000) | 0.421* (0.000) | 0.270* (0.000) | 0.329* (0.000) | 0.514* (0.000) | 0.421* (0.000) |
AI-1 and AI-2 are annual patent applications related to AI and industrial robots, respectively. The mean values of GE, RQ, VA, PS, RL, and CC are 1.238, 1.190, 1.132, 0.694, 1.190, and 1.100, respectively. Statistical significance (P values) is reported in parentheses.
Abbreviation: n.a., not applicable.
*,**,***Statistical significance at 1%, 5%, and 10% levels, respectively.
Overall, the results across Tables 6–8 emphasize the importance of economic and institutional governance in maximizing AI’s employment benefits for people with disabilities. While economic governance is crucial for driving AI’s general impact on employment, institutional governance fosters inclusivity by ensuring fair and equitable workplace policies, particularly for women. Specifically, the findings underscore the critical role of economic governance in moderating the relationship between AI and employment for people with disabilities. Economic governance, measured through government effectiveness and regulatory quality, ensures that AI adoption is accompanied by robust policies and frameworks that promote inclusive labor market participation. Strong economic governance creates an enabling environment by reducing structural barriers, facilitating accessible technological innovations, and supporting skills development for individuals with disabilities. This is consistent with the findings of Kaufmann et al. [33], who emphasize that effective regulatory frameworks enhance resource allocation and labor market efficiency, particularly in technologically advanced economies. Moreover, integrating AI within a robust economic governance structure ensures that the benefits of AI technologies are distributed equitably, fostering broader participation of marginalized groups, including those with disabilities. On the other hand, political governance, encompassing voice, accountability, and political stability, appears to play a less significant role in the AI-employment nexus. Although political governance is essential for fostering democratic processes and societal trust, its limited direct influence on employment outcomes for people with disabilities suggests that broader structural factors, such as economic and institutional frameworks, are more impactful. This finding aligns with the observations of Rodrik [34], who argues that while political governance is critical for macro-level stability, its direct role in labor market outcomes is often mediated through economic and institutional channels. The results further highlight the complementary role of institutional governance, including the rule of law and control of corruption, in promoting equitable employment opportunities. Institutional governance mechanisms ensure transparency and fairness in hiring practices, providing a foundation for inclusive AI adoption. Anticorruption measures and the enforcement of labor rights are particularly crucial for reducing discrimination and ensuring that AI-driven advancements benefit both men and women with disabilities. This aligns with North’s [35] institutional theory, which underscores the importance of strong institutions in reducing transaction costs and fostering inclusive economic growth. In conclusion, while economic governance has the most pronounced moderating effect on AI-driven employment outcomes, institutional governance plays a vital supportive role, particularly in fostering gender inclusivity. These findings emphasize the need for a multifaceted governance approach to maximize the potential of AI in creating inclusive labor markets for people with disabilities.
CONCLUSION AND IMPLICATIONS
This study investigated how AI and governance quality impact the employment of individuals with disabilities, focusing on a sample of 25 developed countries from 2010 to 2022. The findings reveal both linear and nonlinear effects of AI on employment outcomes, with significant moderating roles played by economic, political, and institutional governance dimensions. The results underscore that while AI has the potential to enhance employment opportunities, its impacts are highly conditional upon governance quality. Specifically, economic governance demonstrates the strongest moderating effect, facilitating the adoption of inclusive AI technologies and fostering accessible labor markets. Institutional governance, which includes the rule of law and control of corruption, also plays a vital role by ensuring equitable hiring practices and transparency. However, political governance, encompassing variables such as voice and accountability and political stability, exhibits a relatively limited impact on employment outcomes for people with disabilities, highlighting the importance of structural rather than political interventions in labor markets. These findings provide critical insights for policymakers aligning technological advancements with social inclusion objectives.
The results have several policy implications. First, strengthening economic governance is crucial to ensure that AI technologies are accessible and inclusive. Policymakers should prioritize enhancing regulatory frameworks that promote fair labor market practices and reduce structural barriers for individuals with disabilities. This includes implementing AI-specific guidelines to prevent bias in automated hiring processes and incentivizing firms to adopt AI technologies that support accessible employment. Furthermore, governments should invest in skill development programs tailored to the needs of people with disabilities, ensuring that they are equipped to thrive in an AI-driven economy. Second, institutional governance mechanisms should be reinforced to foster a transparent and equitable labor market. Anticorruption initiatives and enforcing labor rights are essential for reducing discrimination and ensuring that the benefits of AI are equitably distributed. Establishing independent oversight bodies to monitor the impact of AI on employment and labor practices can further enhance governance accountability. Third, while political governance plays a less direct role in shaping employment outcomes, ensuring political stability and public trust is essential for the broader acceptance and implementation of governance reforms. Governments should engage with civil society and disability advocacy groups to co-create policies that reflect the lived experiences and needs of people with disabilities. Finally, the nonlinear relationship between AI and employment, as revealed by the study, highlights the importance of identifying and surpassing critical thresholds for AI adoption. Policymakers should design targeted interventions that support economies and demographic groups in reaching these thresholds, ensuring that the positive impacts of AI on employment are realized while minimizing potential displacement effects. They also should implement AI regulations to prevent bias in hiring, ensuring transparency and accountability in AI-driven recruitment. Strengthening workforce inclusion policies through disability employment quotas, tax incentives, and AI-driven accessibility solutions will promote equitable job opportunities. Establishing digital accessibility standards will ensure AI-enabled workplace technologies and online platforms accommodate persons with disabilities through universal design principles. Additionally, targeted training programs focusing on AI literacy and digital upskilling will equip persons with disabilities with essential skills for an evolving job market. Integrating these policy mechanisms will maximize AI’s potential to foster inclusive labor markets while minimizing employment disparities. To conclude, investments in inclusive technological infrastructures and robust governance mechanisms can enable AI to catalyze inclusive labor markets. These insights contribute to the growing discourse on the role of governance in shaping equitable and sustainable technological transitions, emphasizing the need for holistic and adaptive policy frameworks to maximize AI’s potential for fostering employment inclusivity.
While this study provides valuable insights into the relationship between AI, governance quality, and employment outcomes for people with disabilities, it has certain limitations that warrant further exploration. Specifically, the analysis focuses on 25 developed countries due to the unavailability of comprehensive AI-related data for most developing economies. This focus limits the study’s applicability to regions with differing governance structures, labor market dynamics, and levels of technological development. Future research could expand the scope by including more developed and developing countries and conducting comparative analyses across regions, offering a broader and more nuanced understanding of how AI and governance interact to influence inclusive employment outcomes globally. Moreover, the study considers three governance dimensions—economic, political, and institutional—without exploring potential interactions or synergies between these dimensions. Future research could examine how these governance aspects dynamically interact to amplify or mitigate AI’s employment effects, providing a more holistic understanding of governance’s role in shaping AI-driven labor markets. Additionally, while this study highlights the moderating role of governance quality, it does not fully account for industry-specific variations or sectoral differences in AI adoption. AI’s impact on employment is likely to vary across sectors, with certain industries presenting greater risks or opportunities for people with disabilities. Future research could conduct sectoral analyses to identify which industries are most affected by AI advancements and where governance interventions are most needed to ensure inclusive employment policies. Furthermore, the study does not explicitly include certain micro-level factors, such as legal frameworks, workplace accessibility, and assistive technologies, due to data unavailability at the macro level. These variables are critical in shaping employment opportunities for persons with disabilities, and future research could incorporate them to provide a more granular analysis of AI’s role in workforce inclusion. Finally, we recognize that labor market discrimination, AI ethics, and employer attitudes could further enhance the explanatory power of the model. These factors play a crucial role in determining whether AI technologies promote or hinder employment for people with disabilities. Future empirical investigations should integrate discrimination metrics, AI fairness indicators, and employer perceptions of disability employment to develop a more comprehensive framework for understanding AI’s labor market effects.