INTRODUCTION
Arabic is one of the most widely spoken Semitic languages. It presents a diverse linguistic landscape distinguished by a complex phonetic and phonological system, including unique features like geminate, emphatic, uvular, and pharyngeal sounds, and marked by specific vowel durations (Lehn, 1963; Selouani & Caelen, 1998; Newman, 2002; United Nations, 2003; Watson, 2007; Qassem, 2015, 2021; Ayyad et al., 2016; Hammami et al. 2020). These features make Arabic challenging for learners and particularly relevant for speech pathology, where accurate production of such sounds is crucial. The uvular sounds, for example, are rare globally and require intricate tongue and vocal tract positioning that can be especially difficult for individuals with speech disorders (Rajouani et al., 1987). Because speech disorders affect individuals across personal, educational, and social dimensions, difficulties with Arabic’s uvular and pharyngeal sounds can deepen these challenges, potentially leading to communication barriers, social withdrawal, and anxiety (McCormack et al., 2009; Pennington and Bishop, 2009; Dougherty et al., 2013). However, although various studies have extensively examined the pathology of Arabic speech, few studies have focused on the acoustic and articulatory characteristics of uvular sounds.
This creates a gap in the literature that this study aims to address. This research provides important insights to improve clinical guidelines and develop technology-driven tools to diagnose, monitor, and evaluate speech sound disorders through detailed acoustic analysis. This ultimately improves communication outcomes for individuals with speech disorders.
The Arabic phonetic structure produces the unique articulatory and acoustic features of uvular sounds with the body of the tongue pulled back. They are raised toward the uvula, making this sound production a challenge, particularly for individuals with speech disorders, and complicating their ability to produce Arabic phonetic structures accurately (Alotaibi and Muhammad, 2010). These sounds demand precise control over specific vocal tract mechanisms, making their accurate production a significant challenge for affected speakers. Moreover, these sounds make this study area particularly relevant for phonetics and speech pathology. Difficulties in producing uvular and pharyngeal sounds can significantly impact an individual’s communication ability, highlighting the need for targeted interventions as soon as speech impairments are detected.
Speech disorders, which may arise from various causes such as delays in speech development, neurological conditions, or anatomical differences, present significant challenges across personal, academic, and social domains (Dodd, 2013). In the context of Arabic, the inability to produce sounds accurately can lead to further complications, including social anxiety and avoidance behaviors (Dougherty et al., 2013), highlighting the importance of actual therapeutic interventions. Despite the significant role of uvular sounds in Arabic phonology, there is a noteworthy lack of research on their acoustic characteristics among individuals with speech disorders. This limited research on this area is a significant challenge in understanding the acoustic characteristics of uvular sounds among these individuals. Although some studies have addressed speech impairments in Arabic, there are gaps in understanding the acoustic problems of the uvular sound.
However, existing studies such as Alaraifi et al. (2014), Terbeh et al. (2016), Hammami et al. (2020), Alqudah et al. (2020), and Shareef and Al-Irhayim (2022) have explored Arabic speech disorders in a broader sense. They highlight a significant gap in the literature as Arabic is one of the top five most widely spoken languages (Al-Zabibi, 1990). Many studies have been conducted on other natural language processing fields, such as morphological analyzers, syntactic parsers, and machine translation (Habash, 2010; Mahyoob and Al-Garaady, 2018; Mahyoob, 2020).
By focusing on these areas, the study aims to augment the scientific community’s perception of Arabic phonetics and the impact of speech disorders on language production, as well as to play a role in improving communication abilities in individuals with speech disorders.
Objectives of the study
The main goal of this study is to investigate and measure the production of uvular sounds among Arabic-speaking individuals with speech disorders within the context of Saudi Arabian speech. The study attempts to:
Identify the specific acoustic features and articulatory challenges encountered by individuals with speech impairments while producing uvular sounds and provide insights into the abnormalities noticed in their speech.
Compare the affected individuals’ uvular acoustic features with those of normal individuals to identify significant differences.
Figure out how speech disorders affect the production of uvular sounds.
Provide insights to inform the development of targeted speech therapy interventions and automated detection systems for uvular sound production discrepancies.
Lay the basis for developing automated tools to detect and track uvular sound production among individuals with speech impairments, assist them in expressing themselves in speech, assist others in understanding them, and aid in speech therapy interventions. These tools can be used to track the progress of speech therapy and adjust treatment plans accordingly.
Research questions
How do speech disorders impact the production of uvular sounds in Arabic speakers?
What are the specific acoustic features that distinguish uvular sounds in Arabic among individuals with speech disorders, and how do they differ compared with normal individuals?
How can advanced acoustic analysis methods be used to understand better the variability in the production of uvular sounds in individuals with speech disorders?
Hypotheses
Persons with speech impairments will display considerable deviations in the articulation and the acoustic properties of uvular sounds compared with normal speakers.
Acoustic analysis techniques can effectively measure the variability of uvular sound production and the acoustic characteristics that discriminate uvular sound production among persons with speech impairments.
The study’s outcomes will provide the basis for developing automated tracking and evaluation tools to optimize the efficiency of speech therapy in producing uvular sound.
The remainder of this paper is structured as follows: Section 2 provides an overview of the related work. Section 3 outlines the methodology used for this acoustic analysis. Section 4 presents the results and discusses the implications. Finally, Section 5 offers concluding remarks and suggestions for future research.
Related work
Research on the acoustic properties of Arabic uvular sounds has consistently highlighted their unique articulation and acoustic challenges, particularly for individuals with speech disorders. This section reviews relevant research that addresses the acoustic properties of uvular sounds, speech disorders affecting these sounds, and challenges and opportunities in their acoustic analysis. For example, Alwan (1985) examined acoustic properties of the Arabic pharyngeal and uvular consonants to reveal the distinct formant trajectories and variations in voiced consonants and the perception of /ʕ/ and /ʁ/, using synthetic syllables to manipulate formants. The study highlighted that perception of /ʕ/ depends on the closeness of the first two formants, while a broader bandwidth in the first formant is vital for recognizing /ʁ/.
Weinberg and Horii (1975) examined the acoustic features of pharyngeal /s/ sounds in individuals with cleft palate, comparing them to normal /s/ sounds. Pharyngeal /s/ sounds showed distinct acoustic properties with multiple spectral peaks and energy maxima near high first formant (F1) values close to the second formant (F2), resembling the characteristics of /h/ fricatives in Arabic speakers. Similarly, Khwaileh et al. (2019) analyzed speech production in Arabic-speaking children with cochlear implants (CIs), focusing on voice onset time (VOT) for pharyngealized plosives and spectral moments for pharyngealized fricatives. Children with CIs struggled with VOTs for plosives and showed altered spectral patterns for fricatives compared with their normal-hearing peers, although they could still differentiate between fricatives. These differences were linked to the limited auditory input from CIs and reduced motor experience, highlighting the effectiveness of VOT and spectral moments in detailing subtle phonetic aspects. Shahin (2006) examined speech samples from three Arabic-speaking children with cleft palate. This study found their speech shared common traits with cleft speech in other languages, including unique features like implosive airstream and devoicing. Despite Arabic’s phonemic pharyngeals, compensatory pharyngeal and glottal articulations were produced, supporting that cleft speech characteristics are universal. In addition to these general studies on Arabic phonemes, Al-Adam (2015) studied the acoustic features of emphatic sounds produced by Arabic speakers diagnosed with Broca’s aphasia compared with normal speakers in the Palestinian context. The author investigated the acoustic correlates of emphasis in Palestinian Arabic using VOT measurements, frequency values of F1 and F2 formants, and duration of vowels. The study discovered that subjects with Broca’s aphasia could not sustain the acoustic distinction between the emphatic sounds and their plain counterparts. Prosodic features, including intonation, speech rhythm, and pitch variation, are also crucial in analyzing speech disorders. Studies on autism spectrum disorder (ASD) have highlighted prosodic differences in speech, including changes in fundamental frequency and speech rate. Patel et al. (2020) found that individuals with ASD and their parents show similar prosodic differences, such as slower speech rates and more significant pitch variation in ASD. These shared traits suggest that prosodic differences may contribute to ASD features and indicate genetic links among first-degree relatives.
Machine learning (ML) is becoming an increasingly valuable tool for diagnosing speech disorders. Specifically, in analyzing the acoustic characteristics of disordered speech, Hanani et al. (2016) studied Arabic-speaking children with articulatory disorders using Mel-frequency cepstral coefficient features and Gaussian Mixture Model (GMM) classification methods. Universal Background Model (UBM) and I-vector, especially with the [r] sound, analyze the position of [r] in the word (beginning, middle, end). The I-vector system achieves an accuracy of 75%, while GMM-UBM reaches 61%, and the combination of abnormal classes improved to 92.5% and 83.4%. Alotaibi et al. (2017) examined the mapping between 29 speech utterances, positions, and typing characteristics and their matching audio-frequency signals using ML techniques. Three techniques including deep belief networks (DBN), multilevel perception (MLP), and hidden Markov models (HMM) show satisfactory phonological-phonetics mapping performance. This indicates that a few Arabic distinctive phonetic feature components, such as affricative, alveopalatals, labiodentals, lateral palatal pharynx, and round-round uvular, have strong correlations with acoustic data. Behroozmand and Almasganj (2007) analyzed dysphonic voices in unilateral vocal fold paralysis (UVFP) patients, focusing on energy and entropy features using wavelet-packet decomposition at five levels. A genetic algorithm optimizes feature selection, and a support vector machine classifier was used for classification. Their findings showed that entropy was more effective than energy in distinguishing UVFP patients, with entropy achieving 100% accuracy, highlighting its value in UVFP diagnosis.
METHODS
Study design
This study employed acoustic analysis to investigate the distinctive features of Arabic uvular sounds among individuals with speech disorders. The recorded speech samples were analyzed using the PRAAT Software, which specializes in detailed phonetic and acoustic analysis (Boersma and Weenink, 2001). The acoustic features analyzed included VOT, pitch, intensity, formant frequencies (F1, F2, F3), and duration of the uvular articulation. Beyond these essential measures, this study assesses speech variability and quality by implying more measures such as jitter, shimmer, and harmonics-to-noise ratio in the voice signal. These are critical for differentiating the production of uvular sounds in disordered and normal speech patterns.
The authors used SPSS statistical software due to its robust analytical potential for performing analysis statistics and visualization (Field, 2013; Pallant, 2016). In our study, we conducted descriptive analyses to compute statistics and provide comprehensive measures of the data, significant patterns, and correlations by conducting Tukey’s honestly significant difference (HSD) post hoc test.
The study aims to derive a nuanced understanding of speech characteristics, enabling a thorough investigation into the distinctions and similarities in speech production among individuals with and without speech disorders.
Data collection
This study employed a parallel-group design to explore the acoustic characteristics of Arabic uvular sound among persons with speech impairments compared to typical speakers. For the subject demographics, this investigation tries to study a set of recorded voice samples collected from a group of Saudi participants (n = 20 males and females) with an age range between 6 and 37 years to distinguish between healthy and disordered speech. Fortunately, there were no attrition or dropouts among the participants during the study. All 20 subjects completed the data collection procedures as planned. The speech disorder group comprised people diagnosed by a certified speech-language pathologist and who were native speakers of Arabic. The participants’ vocalizations were captured in an acoustically controlled environment utilizing premium recording devices (smartphones, laptops, and microphones.). To ensure the reliability of results, the authors used a modern computer equipped with ample RAM and an SSD for efficient data handling, along with a high-quality built-in sound card for optimal audio fidelity.
The participants were allocated to groups based on their clinical diagnosis with an allocation ratio of 1:1 as follows:
Speech disorder group: This group involved 10 participants diagnosed with speech disorders mainly affecting uvular sounds.
Control group: Ten vocally healthy individuals: This controlled group involved members with no history of speech or hearing impairments who were carefully matched with the speech disorder group regarding age and gender to control for potential confusing variables and minimize the impact of age-related variations in speech production.
There was no randomization in the group assignment, as participants were selected according to predefined inclusion criteria, including Arabic Saudi speakers, 10 groups diagnosed with articulation disorders primarily affecting uvular sounds and 10 vocally healthy individuals. The acoustic analysis investigators were blinded to the participants’ group assignments to condense potential bias during data analysis. This blinding aimed to avoid any biased opportunities from affecting the explanation of the acoustic data.
To capture a natural, clear sound, which is ideal for analyzing speech characteristics and ensuring the reliability and validity of the recorded data for our speech analysis. We optimized sound quality and minimized distortion, ambient noise, and other interferences to achieve the best microphone positioning for precise and reliable speech data. The microphone was placed approximately 15-30 cm (6-12 inches) from the speaker’s mouth. This range allows for a clear capture of the speaker’s voice without overwhelming the microphone, preventing distortions that can occur when the microphone is too close. The microphone’s angle is slightly off-center from the speaker’s mouth (around 30 to 45 degrees). This avoids direct air blasts from certain sounds. We adjusted the microphone stand or boom for seated speakers to align with the mouth without causing the speaker to hunch or tilt their head. The record was in a quiet, acoustically treated room to minimize ambient noise and echo.
The authors also follow a set of specifications and criteria:
Sampling at a frequency of 44,100 Hz
Digitizing the audio at 16 bits per sample
Recording and storing it on laptops as a. wav file
The group size of 10 members per group was selected based on practical criteria and in line with sample sizes used in similar previous studies. A formal power calculation was not performed; however, the sample size was considered adequate for examining analysis to identify essential variations in uvular sound production between groups.
After the trial commencement, the authors noticed no critical changes to the study’s methods. The eligibility criteria, data collection procedures, and analysis methods were established before the study. All contributors met the initial inclusion criteria, and the methodology was adhered to as planned to preserve the study’s integrity and validity.
Ethical considerations
Ethical protocols were strictly followed throughout the study. Ethical approval was obtained from the institutional ethics committee of Taibah University in Saudi Arabia. Informed consent was obtained from all participants prior to the data collection process. The confidentiality and anonymity of participant data were ensured throughout the study, and all recordings were used solely for research purposes.
Code availability
No custom code was established in this study, and for conducting the acoustic analysis, the standard functions within PRAAT were utilized (Boersma and Weenink, 2001).
Data availability
The collected data is deposited in the ARASA. Due to its sensitive nature, privacy, and ethical considerations, the corresponding author can access the data upon rational request.
This research does not require RRID (antibodies, cell lines, oligonucleotides, organisms, and plasmids).
Uvular consonants
The Arabic language, both in its classical form and in modern standard Arabic (MSA), contains three primary uvular consonants: Ghain /ɣ/, /غ/, Qaaf /q/, /ق/, and Khaa /x/, /خ/. These consonants are unique to Arabic and are absent in Latin-based languages like English (Al-Ani, 2014). These sounds are produced in the posterior region of the vocal tract and possess distinctive acoustic features that set them apart from other phonemes in the Arabic language as shown in Table 1 below.
Distinctive features of uvular sounds in Arabic.
Sound in Arabic | IPA symbol of the sound | Place of articulation | Manner of articulation | Voicing | Consonantal | Sonorant | Continuant | Dorsal | Pharyngeal |
---|---|---|---|---|---|---|---|---|---|
خ (Khā’) | x | Uvula | Fricative | Voiceless/non-emphatic | Yes | No | Yes | Yes | No |
غ (Ghayn) | ɣ | Uvula | Fricative | Voiced/emphatic | Yes | No | Yes | Yes | No |
ق (Qaaf) | Q | Uvula | Plosive | Voiceless/non-emphatic | Yes | No | Yes | Yes | No |
Ghain /ɣ/, /غ/
Some Arabic linguists have classified the /ɣ/ sound as a velar consonant (Alkhouli, 1990), while others have categorized it as a palatal sound (Bisher, 1990). However, a third group of linguists identify it as a uvular consonant (Nour-Aldeen, 1992; Alghamdi, 2001). This is because/ɣ/, /q/, and /x/ share the same place of articulation when pronounced in similar word settings. We can describe /ɣ/ as a voiced, non-emphatic, uvular fricative.
Qaaf /q/, /ق/
Although one Arabic phonetician classified /q/ as a pharyngeal consonant (Alkhouli, 1990), most researchers agree that it is a uvular sound (Bisher, 1990; Omar, 1991; Nour-Aldeen, 1992; Alghamdi, 2001). This consonant is typically described as a voiceless, non-emphatic, uvular stop. The results suggest that /q/ is a particularly challenging sound for native Arabic speakers, likely because it is voiceless and can be masked by surrounding noise or nearby sounds.
Khaa /x/, / خ/
The sound /x/ is unvoiced, non-emphatic, and uvular fricative, although its classification is debated among phoneticians. Some sources describe /x/ as a velar sound, others as a palatal, but a third group, including Alghamdi (2001) and Nour-Aldeen (1992), categorize it as uvular, a classification adopted in this context focusing on the Saudi dialect. The variation in classification likely arises from regional dialectal differences. However, the uvular classification is considered most fitting here, given that /x/, /q/, and /ɣ/ share the same articulation point in similar phonetic settings.
Uvular sound variations among affected people
Table 2 documents how the production of Arabic uvular sounds—Ghain (/ɣ/, /غ/), Qaaf (/q/, /ق/), and Khaa (/x/, /خ/)—are affected and altered by speech disorders, detailing substitutions, distortion, and instances of correct articulation. It notes that Ghain and Qaaf may be substituted by Haa (/h/, /ه/) and Khaa (/x/, /خ/), while Khaa itself is sometimes substituted by Haa. This comprehensive documentation of error patterns is crucial for developing targeted speech therapy strategies and enhancing the development of detection and recognition tools. By providing detailed insights into typical deviations from standard pronunciation, the table aids in creating more effective speech recognition software and assisting tools, thereby improving therapeutic outcomes and diagnostic capabilities for Arabic-speaking patients with speech disorders.
RESULTS
The acoustic data were analyzed by comparing the variability of uvular sound production between the two groups to identify patterns and deviations that may characterize speech impairments in the production of uvular sounds. Measures of central tendency and variability were calculated for key acoustic parameters, and statistical analyses were performed to identify significant differences between the speech disorder and control groups and the challenges individuals with speech disorders face when producing these specific sounds. Variability in formant frequencies and VOT across individuals was examined to determine how speech disorders affect uvular sound production.
Figure 1 shows four different spectrogram analyses for the speech sounds /x/ from the control and speech disorder groups. A spectrogram is a visual representation of the spectrum of frequencies of a signal as it varies with time. Normal speech’s pitch appears relatively steady, and the formants are visible, indicating typical speech production. There might be slightly more pitch variability in the control and speech disorder groups, and the formants appear less distinct, indicating some speech production issues. The Abnormal 2 shows significant gaps in voicing (visible as large dark spaces in the spectrogram where the pitch track disappears), suggesting periods of unvoiced speech. The pitch is less stable, and the formants are less clear, which could correspond to a more severe speech disorder. In the case of Abnormal 3, there is less pitch variation than in Abnormal 2, and formants are somewhat more defined. However, there are still signs of disordered speech characteristics, such as potential irregularities in voicing or breathy emissions.
Table 3 represents the data for the sound /x/ pronounced by the normal individual and three individuals with speech disorders. It provides a comparative overview of the acoustic features of uvular sound across normal and disordered Arabic speech, highlighting the significant differences in pitch, pulse, and quality indicators such as jitter, shimmer, and harmonicity.
The statistical analysis for the sound /X/ using pitch, pulse, and voicing parameters.
Parameter | Normal | Abnormal 1 | Abnormal 2 | Abnormal 3 | |
---|---|---|---|---|---|
Pitch | Median (Hz) | 234.018 | 265.395 | 320.313 | 312.512 |
Mean (Hz) | 231.244 | 261.904 | 321.983 | 313.640 | |
Std. deviation (Hz) | 49.329 | 9.913 | 12.398 | 20.660 | |
Minimum (Hz) | 133.043 | 224.299 | 296.440 | 277.370 | |
Maximum (Hz) | 328.432 | 274.027 | 356.805 | 352.534 | |
Pulses | Number of pulses | 66 | 128 | 130 | 196 |
Number of periods | 63 | 124 | 119 | 185 | |
Mean period (s) | 4.270146E-3 | 3.875405E-3 | 3.169284E-3 | 3.188109E-3 | |
Std. deviation of period (s) | 0.902252E-3 | 0.421198E-3 | 0.554398E-3 | 0.241475E-3 | |
Voicing | Unvoiced frames (%) | 4.301 | 7.051 | 40.408 | 2.551 |
Voice breaks | 0 | 0 | 1 | 0 | |
Degree of voice breaks | 0 | 0 | 11.404 | 0 |
Pitch statistics
The pitch statistics in Table 3 revealed significant differences between the control and speech disorder groups. In normal conditions, the mean and median noise levels are approximately 234 Hz and 231 Hz, respectively, indicating a stable and moderate noise level. However, the noise level values increase rapidly in abnormal conditions. Abnormal 2 shows the highest mean and average noise levels at around 320 Hz and 322 Hz. The standard deviation, which measures the noise level variability, is significantly higher in normal conditions (49 Hz) compared with abnormal conditions. This value is significantly lower. This indicates a higher level of monotony in abnormal conditions. Even in abnormal conditions, the range of sound is narrow, with minimum, low, and maximum values in normal conditions. Furthermore, it also emphasizes the reduced elasticity of the voice during abnormal sounds.
Pulses statistics
Statistical pulse analysis in Table 3 also indicates differences between control and speech disorder groups. This contrasts with normal conditions, which show a balanced pulse number and duration, with values of 66 and 63, respectively, indicating a typical vocal fold vibration pattern. In particular, abnormal 3 shows significantly increased pulses and durations, with values as high as 196 and 185, possibly a condition that is associated with the higher tone observed. The average duration of vocal fold vibration is shorter in abnormal conditions, which indicates a faster cycle. At the same time, the standard deviation of the duration is lower in abnormal situations. This indicates fewer natural fluctuations. Furthermore, there may be more forceful or unnatural vocal cord behavior.
Voicing statistics
The voicing statistics in Table 3 showed essential insights into the continuity and quality of sound. Under normal conditions, the percentage of unvoiced sound is approximately 4.3%, indicating periods of apparent silence or noise during speech. However, in abnormal 2, this percentage increases to 40.4%, indicating interruptions in sound. Significantly, this may be due to breathing sound tension or interruption of the vibration of the vocal folds, in contrast to those that indicate a severe disruption of the speech sound continuum. Normal conditions and other abnormal symptoms suggest that there is no audio loss. abnormal 3 shows the percentage of frames that do not have abnormal audio loss, indicating continued pronunciation and possible vowel stress.
Jitter analysis
Jitter represents the variation in frequency from one pitch frame to another and is an essential measure of sound level stability. Table 4 shows that normal conditions have moderate jitter values. It has a local jitter of 3.42%. However, anomalous situations show significant variance. abnormal 1 has the most negligible jitter, which indicates more stable vocal fold vibration. Meanwhile, abnormal 2 has the highest jitter of 4.76%, suggesting uncertainty in vocal reproduction. This may be due to stress or vocal abnormalities. It has a higher value in stressful or erratic vocal situations, indicating more significant variability and less consistent vibrations of the vocal folds.
The statistical analysis for the sound /X/ using jitter, shimmer, and harmonicity parameters.
Parameter | Normal | Abnormal 1 | Abnormal 2 | Abnormal 3 | |
---|---|---|---|---|---|
Jitter | Local (%) | 3.420 | 1.270 | 4.760 | 1.889 |
Local, absolute (s) | 146.033E-6 | 49.218E-6 | 150.861E-6 | 60.218E-6 | |
Rap (%) | 1.747 | 0.443 | 2.214 | 0.919 | |
Ppq523 (%) | 1.723 | 0.518 | 2.016 | 0.776 | |
Ddp | 5.240 | 1.330 | 6.643 | 2.757 | |
Shimmer | Local (%) | 15.767 | 9.550 | 19.877 | 10.224 |
Local, dB | 1.363 | 1.028 | 1.597 | 1.054 | |
Apq3 (%) | 8.493 | 4.321 | 11.765 | 5.155 | |
Apq5 (%) | 8.665 | 6.645 | 15.917 | 7.268 | |
Apq11 (%) | 13.364 | 9.166 | 27.585 | 9.919 | |
Dda | 25.479 | 12.963 | 35.295 | 15.465 | |
Harmonicity | Mean autocorrelation | 0.785541 | 0.857430 | 0.669294 | 0.818221 |
Mean noise-to-harmonics ratio | 0.317014 | 0.243424 | 0.619715 | 0.344900 | |
Mean harmonics-to-noise ratio (dB) | 6.459 | 10.839 | 4.083 | 10.946 |
Shimmer analysis
Shimmer measures amplitude variations in vocal fold vibrations, which is essential in evaluating sound quality. Under normal conditions, it shows a moderate amount of shimmer. The local scintillation value is 15.77%, indicating the degree of natural amplitude fluctuation. In abnormal situations, incredibly Abnormal 2, the shimmer value was significantly higher, reaching 19.88%, indicating greater amplitude instability that may be related to vocal problems such as breathing. Difficulty, hoarse voice, and effort to speak indicate various oscillation-related measures that also revealed increased amplitude changes in the presence of irregular vowels. Especially in disorder 2, emphasis is placed on vocal tension or pathology.
Harmonicity analysis
Harmony measures, including average autocorrelation and harmonic-to-noise ratio (HNR), provide insights into sound clarity and tonal quality by comparing the harmonic components (as distance) and noise (periodicity) of sound under normal conditions. Harmony values are balanced, with an average HNR of 6.46 dB. Anomalous conditions indicate that the tones differ: abnormal 1 and abnormal 3 have higher HNR values, indicating clearer tones and more harmonics. In contrast, abnormal 2 has a significantly lower HNR (4.08 dB) and average autocorrelation. This shows a louder and less stable sound quality. This may be due to some sound problems. These harmonic measures are essential in identifying deviations from usual sound quality by revealing the noise of the sound and decreasing the presence or consistency in erratic vocal situations.
Figure 2 comprehensively compares jitter, shimmer, and harmonicity parameters in the Normal and Abnormal groups. Normal values reflect a balanced, natural tone quality with moderate jitter, shimmer, and harmonicity levels. Moreover, in the first abnormal situation, the sound is suggested to be more controlled and stable with less jitter and shimmer, and higher harmonics indicate a clearer sound that may be less dynamic. abnormal 2 indicates a significant sound quality degradation with high jitter and shimmer, indicating possible vocal tension or pathology. Abnormal 3 shows more stability but less dynamics. The tones are more harmonious than usual, suggesting a more transparent tone with some obstacles to this change in frequency. This comparison reveals how different acoustic conditions can affect important acoustic characteristics. It provides an understanding of the vocal quality and potential problems.
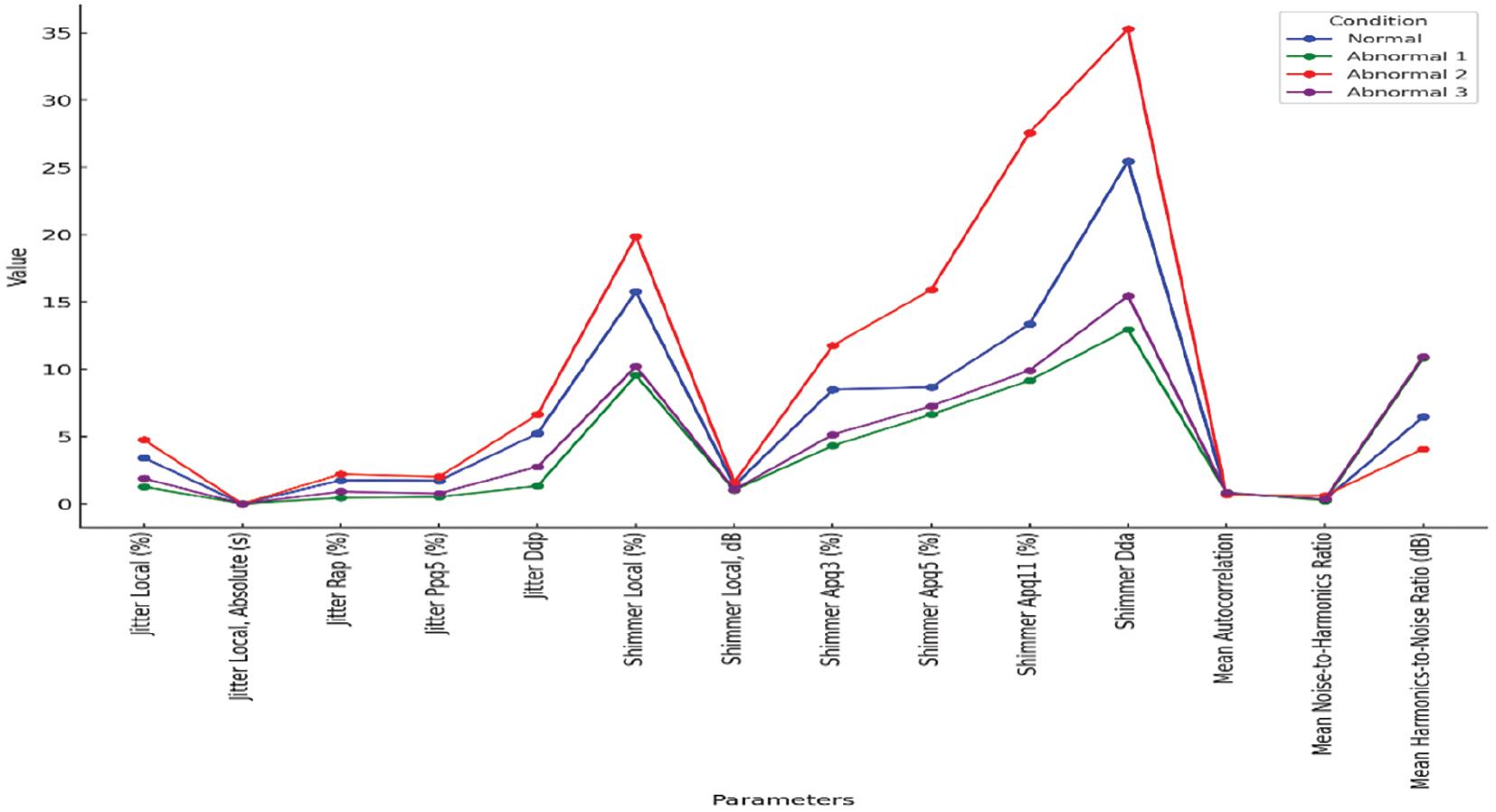
The combined data for jitter, shimmer, and harmonicity parameters across different conditions.
Figure 3 shows harmonicity (HNR) over time, using a gradient to show changes in harmonic levels. The x-axis represents time, while the y-axis represents harmonicity in decibels (dB); the gradient changes as the data progress over time. It reveals changes in the harmonic content of the speech signal; darker gradients indicate less harmony. This can indicate increased noise or breathiness of the sound. Brighter colors correspond to more aesthetics, indicating more precise and stable sound quality. This figure helps identify vocal stability or instability patterns in dynamic contexts such as speech, communication, or therapeutic monitoring.
Tukey’s HSD pairwise pitch median comparisons
This extremely small P value indicates significant differences between the groups’ pitch medians (control and speech disorder groups). In other words, the variation in pitch between the groups is not due to random chance, and at least one group’s pitch median differs significantly from the others.
Significant differences are found between most group pairs, except Abnormal 2 and Abnormal 3, where no significant difference is observed, as shown in Table 5.
Tukey’s HSD pairwise pitch median comparisons of the groups.
Group 1 | Group 2 | Mean difference (Hz) | P value | 95% confidence interval (lower bound) | 95% confidence interval (upper bound) | Significant? |
---|---|---|---|---|---|---|
Normal | Abnormal 1 | −31.377 | 0.000 | −45.632 | −17.122 | Yes |
Normal | Abnormal 2 | −86.294 | 0.000 | −100.549 | −72.039 | Yes |
Normal | Abnormal 3 | −78.494 | 0.000 | −92.749 | −64.239 | Yes |
Abnormal 1 | Abnormal 2 | −54.917 | 0.000 | −69.172 | −40.662 | Yes |
Abnormal 1 | Abnormal 3 | −47.117 | 0.000 | −61.372 | −32.862 | Yes |
Abnormal 2 | Abnormal 3 | 7.800 | 0.498 | −6.455 | 22.055 | No |
Abbreviation: HSD, honestly significant difference.
The control group differs significantly from Abnormal 1, Abnormal 2, and Abnormal 3. Abnormal 1 also differs considerably from Abnormal 2 and Abnormal 3. This post hoc analysis confirms that most groups have significantly different pitch medians. Tukey’s HSD post hoc test was performed to identify which specific group pairs have statistically significant differences in their pitch medians after the overall analysis of variance showed significant differences between the groups, as discussed in the following sections.
Control and speech disorder groups
Normal versus Abnormal 1: There is a significant difference in the pitch medians between these two groups, with Normal having a lower mean pitch than Abnormal 1. The difference is −31.38 Hz, and the P value is highly significant (P < 0.001). This suggests that individuals in the Abnormal 1 group exhibit significantly higher pitch than the Normal group.
Normal versus Abnormal 2: The difference between Normal and Abnormal 2 is much more significant (−86.29 Hz), indicating that the Abnormal 2 group has a considerably higher pitch median than the Normal group. This difference is also statistically significant (P < 0.001).
Normal versus Abnormal 3: The comparison between Normal and Abnormal 3 shows a difference of −78.49 Hz, again showing that Abnormal 3 has a significantly higher pitch than the Normal group (P < 0.001).
Speech disordered groups comparison
Abnormal 1 versus Abnormal 2: The difference between these two groups is −54.92 Hz, which is statistically significant (P < 0.001). This suggests that Abnormal 2 has a notably higher pitch median than Abnormal 1.
Abnormal 1 versus Abnormal 3: Similarly, the difference between Abnormal 1 and Abnormal 3 is −47.12 Hz, which is also significant (P < 0.001), indicating that Abnormal 3 has a higher pitch than Abnormal 1.
Abnormal 2 versus Abnormal 3: Interestingly, no significant difference exists between Abnormal 2 and 3. The mean difference is only 7.80 Hz, and the P value is 0.498, which indicates that these two groups have similar pitch medians.
Higher pitch in speech disordered groups: Across all comparisons, the Abnormal groups tend to have significantly higher pitch medians than the Normal group. This suggests that pitch might be a distinguishing feature between normal individuals and those with specific speech abnormalities.
Differences between Abnormal groups: While Abnormal 1 differs significantly from both Abnormal 2 and Abnormal 3, the two latter groups (Abnormal 2 and Abnormal 3) do not vary considerably. This might imply that Abnormal 2 and 3 represent similar degrees of deviation from normal pitch behavior, while Abnormal 1 may be less deviant. The significant differences in pitch among the groups suggest that pitch measurements could be a useful diagnostic indicator for distinguishing different types of speech disorders.
DISCUSSION
This study significantly advances the acoustic analysis of challenging Arabic sounds, especially uvular sounds, among individuals with speech disorders. This area has not been explored before, as most previous research have focused on the acquisition and production of these sounds by native and non-native speakers without focusing on speech disorders. On the contrary, this study highlights the complexity of producing Arabic’s most difficult uvular phonemes for people with speech disabilities. The observed variation in phoneme production highlights the need to investigate other uvular phonemes further. The findings also indicate the potential for developing artificial intelligence-based tools to predict and detect sounds, providing valuable insights and supporting clinical practice. Expanding this research to include other phonemes will deepen our understanding and open new opportunities to improve speech interference.
This study critically explains vocal health by comparing acoustic parameters under control and speech disorder groups. Findings indicate that in abnormal conditions, pitch increases, vocal fold vibrations are faster and less variable, and there are frequent, severe vocal fold breaks. These changes suggest underlying stress and strain, highlighting the importance of pitch stability, jitter, shimmer, and harmonicity as critical indicators for assessing vocal health. Moderate jitter, shimmer, and HNR reflect a balanced, natural tonal quality in normal conditions. For instance, the local jitter at 3.42% and shimmer at 15.77% indicate healthy variations in frequency and amplitude. An average autocorrelation of 0.785 and HNR of 6.46 dB further support a balanced harmony in the sound, reflecting natural tonal variation in healthy speech.
In contrast, abnormal vocal conditions show distinct shifts in these parameters. Condition 1 is characterized by reduced jitter and shimmer values (1.27% and 9.55%, respectively), suggesting a more controlled tone with less dynamic range, potentially indicative of vocal tension. Condition 2, however, displays increased jitter and shimmer (4.76% and 19.88%), coupled with lower autocorrelation (0.669) and HNR (4.08 dB), pointing to severe vocal quality degradation often associated with hoarseness or pathology. Condition 3 reflects a slightly more stable but less natural tone, with moderate improvements in jitter and shimmer but reduced harmonicity, suggesting some difficulty maintaining natural tonal variation. These observations of uvular sound characteristics could advance the development of Arabic speech recognition systems and inform targeted therapeutic approaches in speech therapy, enhancing tools for both clinical and technological applications.
CONCLUSION
This study provides a deeper understanding of the acoustic properties of uvular sounds and the analysis of speech production by individuals with speech impairments in Arabic. It also paves the way for creating predictive models and diagnostic tools that can be integrated into everyday clinical practice. The analysis of pitch, pulse, voicing, jitter, shimmer, and harmonicity provides a comprehensive view of vocal quality and health. Stable and healthy voices are characterized by moderate pitch variations, consistent pulse, and voicing patterns, low jitter and shimmer levels, and high harmonicity, all contributing to a clear and balanced vocal output. In contrast, voices with extreme values—such as high jitter and shimmer or low harmonicity—indicate instability, strain, and potential vocal issues, leading to a rough, unclear, or breathy sound. This integrated approach to analyzing these acoustic parameters offers valuable visions for diagnosing vocal disorders, improving vocal training, and optimizing therapeutic interventions.
Limitations of the study and future work
This study is limited to one uvular sound, /x/, produced by individuals with speech disorders to analyze the sounds comprehensively. This investigation of uvular phonemes considers their complexity and diversity. However, the analysis conducted in this paper focused on /x/ and not /ɣ/ and /q/, which also vary among those individuals according to the collected data. Acoustic characteristics and challenges are presented for the analyzed sound. For this reason, the results of this study cannot be generalized to all uvular sounds in Arabic or to other speech formats that affect different sound types.
In future research, we plan to expand the scope to include a comprehensive analysis of the remaining two uvular sounds, /ɣ/ and /q/, allowing for a more holistic understanding of the production challenges. Further studies could examine comparisons between different population groups and types of speech disorders.