INTRODUCTION
In Brandão and Porta Nova (2009), a technique that is built on traditional time-series approaches was suggested for evaluating continuous-time computer reactions under various nonstationary circumstances. There, methods for developing Meta models are presented, as are confidence ranges for nonstationary answers that are time-persistent, such as the quantity of entities in queues or the overall quantity stored in a system (Guo et al., 2022; Ilhan et al., 2023). These processes, however, did not apply to nonstationary time performance metrics, i.e. those available in physiological signals. The ensuing time series of physiological indicators, including heart rate, blood pressure and breathing rate, can display complicated fluctuation patterns over a range of time periods (Camm et al., 1996; Costa et al., 2002; Ivanov et al., 2002). The aim of this work is to analyse physiological signals in order to develop a mechanical system embedded with the patient’s smart bed for physiological control systems that are affected by health and illness by numerically tracking the changing dynamics and interactions among multimodal physiological signals. The heart rate variability multimodal cardiovascular coupling studies (Cohen and Taylor, 2002; Voss et al., 2009; Schulz et al., 2013) and the nonlinear dynamics of heart rate (Akselrod et al., 1981; Malliani et al., 1991; Iyengar et al., 1996; Goldberger et al., 2002) have been used to evaluate autoregulation. The frequency-dependent connections between fluctuations in heart rate, blood pressure and breathing rate have also been studied using cross-spectral methods (deBoer et al., 1985; Baselli et al., 1986). Multivariate arterial time-series coupling has been studied using nonlinear model detection methods, such as nonlinear autoregressive models (Faes et al., 2008; Riedl et al., 2010). However, their use in patient healthcare services has been constrained due to the nonstationary characteristics (Sharma et al., 2020; Wang et al., 2022) of the time-series model, which records noise and abnormalities, as well as the difficulty of measuring clinical circumstances and therapies.
Due to the complicated connections and observable patterns in the data collected without the need for specialized models, data-driven methods increasingly outperform model-based methods in the field of health management (Akaike, 1974; Hyndman and Khandakar, 2008). This study proposes a model for nonstationary time-series analysis for the signals received from a patient smart bed. Today, health management is crucial for determining how well engineering systems are performing. Numerous model-based approaches, such as those based on lifetime distribution models and Markov models, have been used to predict dependability and lifespan under hypotheses that may or may not be true in reality (Box et al., 1994). However, system-capable methods frequently encounter complicated mathematical formulae that have a significant bearing on the adjustment of their parameters and the accomplishment of their numerical solution. These variables have led to the development of data-driven, observational methods as a potent substitute for real-world, nonlinear dependability forecasting (Shen et al., 2021; Wang et al., 2021). Recent years have seen the emergence of novel dependability modelling approaches based on observational time-series methods (Ljung and Box, 1978). Conventional methods rely on the time-series stationarity assumption, which makes it impossible to accurately describe fleeting events or follow changing dynamics. The connection between various factors in nonstationary physiological time series can now be quantified using time-changing autoregressive and point process-based methods, which can also be used to derive spectrum markers of autonomic regulation (Barbieri and Brown, 2008; Gederi et al., 2014). It is unclear how these methods can be used to determine the trait-like dynamic behaviours displayed across a patient group because they analyse each time series separately.
In this article, a novel technique is suggested to evaluate the performance of the transient behaviour of various physiological signals using transfer function analysis of physiological time series. The proposed system is modelled in such a way that the physiological dynamics of a patient can be easily collected from the connected bed. In order to capture the transient changes in response to any change in the signal values, each of these dynamic modes can be used to generate directional transfer functions of multivariate time series as well as generate the indices of variability (comparable to heart rate variability analysis, blood pressure variability and oxygen level variability).
Individual time series can be split into roughly stable dynamic areas while also learning a shared set of dynamic modes, thanks to the proposed method. The proposed method recognizes comparable dynamic behaviours across numerous multivariate nonstationary time series by simultaneously modelling them in a probability framework. Additionally, it makes it possible to understand how different dynamic behaviours relate to one another in a specific therapeutic setting. In this paper, a model is proposed based on the time-series analysis using transient response in relation to the identified nonstationary dynamic modes that can be useful to provide suitable measures for heart rate, blood pressure and oxygen level variability by capturing any significant change that is present in each individual time series. Additionally, one can use the proposed dynamic model to determine the degree of relationship between various nonstationary transient modes and the directed transfer functions of the physiological signals, which have been modelled in the form of magnitude plots, phase plots and transient plots.
The current focus of this research is on understanding the mechanistic basis for smart beds to observe the time-varying dynamics of physiological signals such as heart rate measurement, blood pressure measurement and oxygen level measurement in patients. The bodily signs that are immediately connected to patient monitoring from the smart bed are used in the suggested time-series model.
The coherence function (Carter, 1987), partial coherence (Bendat and Piersol, 2011) or methods based on nonstationary, transient versions of the coherence function (Muma et al., 2010; Orini et al., 2012) are standard methods for analysing the relationship between multidimensional biological data. The coherence function, however, is unable to provide the direction of the information movement because it is not a directional metric.
With the help of the proposed model, nonlinear relationships can be uncovered between multidimensional time series of the heart rate, blood pressure and oxygen level signals. The proposed approach is analysed for the transient analysis of physiological signals.
The paper is structured as follows: the first section is Introduction, the next section is The Proposed Model followed by the Relationship Between the Transfer Functions and Physiological Signals, the Data Collection and Evaluation of This Study, Results and Discussion and finally, the Conclusion.
THE PROPOSED MODEL
Analogous to the method used for their continuous-time equivalents, this work suggests a method for studying time-verifying and nonstationary signals. The first stage of the process, which involves creating aggregated time series, differs significantly from the second and third steps. The output is sampled at normal time periods, but this does not result in a legitimate time-series dynamic analysis. Figure 1 shows the proposed nonstationary model for smart beds.
One of the crucial stages of the process is to get approximations of the anticipated value of the nonstationary transient problem for each recorded physiological signal that is evenly separated. To guarantee that each interval includes at least one sample, an appropriate time period should be chosen. The anticipated value of the answer for each time interval is calculated by dividing its (defined) total by the duration of the interval.
By multiplying the interval duration by the average of the data gathered for each type of signal that came during the time interval, the anticipated value of the time-varying transient response is calculated using the proposed model. As a consequence, the value of the transient response that is produced represents the average of the measurements made during the same time period across 100 samples.
The suggested method is based on dynamic transient model analysis in a nonstationary situation, i.e. time-dependent physiological signals. This paper uses time-varying responses to simulate physiological time-series groups of signals collected from the smart bed by using basic linear dynamical systems. Let y(n, t) be a function of the observed physiological sign readings at time t(t = 1….T(n)). It is assumed that there exists a variable number, D, of potential dynamic modes for heart rate (H(t)), blood pressure (B(t)) and oxygen level (O(t)), monitoring which is specified by D = {H(t), B(t), O(t)}, where
H(t)={Hp(t)}Pp=1, B(t)={Bp(t)}Pp=1
Assume that s(n, t) is a switching variable that represents the nth case being a highly active dynamic mode at time t and changes in accordance with a dynamic distribution and transition matrix. These criteria lead to the formulation of the proposed model for the nth case such that
where ε is the volatility term and is supposed to be Gaussian distributed.
These dynamic modes can be used to explain how collections of nonstationary multidimensional time series change over time, with each mode giving a locally durable linear model. This study finds a significant basis from the inference which was used in Murphy (1998) to discover the set of variables (i.e. time-series segmentation) and the modes (i.e. the parameters). Using expectation-maximization (EM), the maximum-likelihood set of model parameters and a derived estimate of the probability distribution over the hidden switching variables were discovered (Murphy, 1998) It was possible to share the dynamics across the entire group by merging all calculated and estimated data from every person to obtain a shared collection of modes in the M phase. The EM method was iterated through several stages, and the end result was the learning of a collection of all dynamic modes for each smart bed. The amount of time the patient spends in the jth mode is what is referred to as the mode proportion, i.e. mpj(n, t). It is assumed that
mpj(n,t)=1T(n)T(n)∑t=1Pr(s(n,t)=j)
RELATIONSHIP BETWEEN THE TRANSFER FUNCTIONS AND PHYSIOLOGICAL SIGNALS
The characteristic of transfer functions in terms of magnitude and its transient behaviour between any of the variables can be driven using the dynamic mode coefficients, i.e. H(t), B(t), O(t) at time t. And the parametric power spectra of each variable can be extracted. The magnitude and phase dependency can be measured for any one variable on other variables using the transfer function analysis. It is proven that the transient analysis is the measure for the stability criteria of any model as well, for instance, the transfer function between blood pressure and heart rate (denoted as T(B → H)) which explains the impact of variations in blood pressure on the heart rate. The block transfer function can be defined as a function of dynamic mode coefficients (Nemati et al., 2011) such that
H(t)=P∑p=1Hp(t).e−2π√−1fp
Similarly,
B(t)=P∑p=1Bp(t).e−2π√−1fp
As an illustration, this work uses the Windkessel function (Cohen and Taylor, 2002) or circulation dynamics (Khoo, 2008) to define a transfer function. Let us consider a situation where the transfer function
T(B→H)=B(t)1−H(t)
DATA COLLECTION AND EVALUATION OF THIS STUDY
The experiments include heart rate measurement, blood pressure measurement and oxygen level measurements from 10 smart beds. Patients were initially positioned flat. The body was tilted from a horizontal to a perpendicular posture before returning to the normal lying position. Every reading was captured at a sampling rate of 250 Hz for roughly 60 minutes (ranging from 55 to 74 minutes) per smart bed. Using an automatic beat recognition method, each pulse was noted, and time-series samples of blood pressure were taken for the suggested time intervals at the moments of the wave maxima. Any variation in the steady state time-series behaviour that is slower than 100 beats per cycle was removed from the heart rate measurement and the blood pressure measurement. From the freely accessible PhysioNet (Goldberger et al., 2000) database, time series of heart rate values and breathing values were taken for 100 recorded samples [as shown in Figures 2–4, which plot the 95% confidence interval (CI) for 100 simulations for heart rate, blood pressure and oxygen level, respectively]. The experiments were made at a sampling frequency of 250 Hz. To create time sequences of peak-to-peak (RR) periods, they were automatically identified, visibly confirmed and peak value adjusted, and the transient (magnitude, phase and step response) was studied, as shown in Figure 5. Figure 5 plots the transient response of (a) the function yH(n, t), (b) the function yB(n, t), (c) the function yO(n, t), (d) the transfer function T(B → H), (e) the transfer function T(H → B), (f) the transfer function T(H→O), (g) the transfer function T(O → H), (h) the transfer function T(B → O) and (i) the transfer function T(O → B). The transient response model, which includes both linear and nonlinear analyses, is taken into consideration for nonlinearity evaluation. This work considers the magnitude, phase and step responses that depict the relationships between heart rate, blood pressure and oxygen level and are anticipated to be expressed by the variation patterns accordingly. The nonlinear variation of these parameters can also produce some leftover linear patterns, and the variation is only anticipated to manifest in the linear dependency patterns.
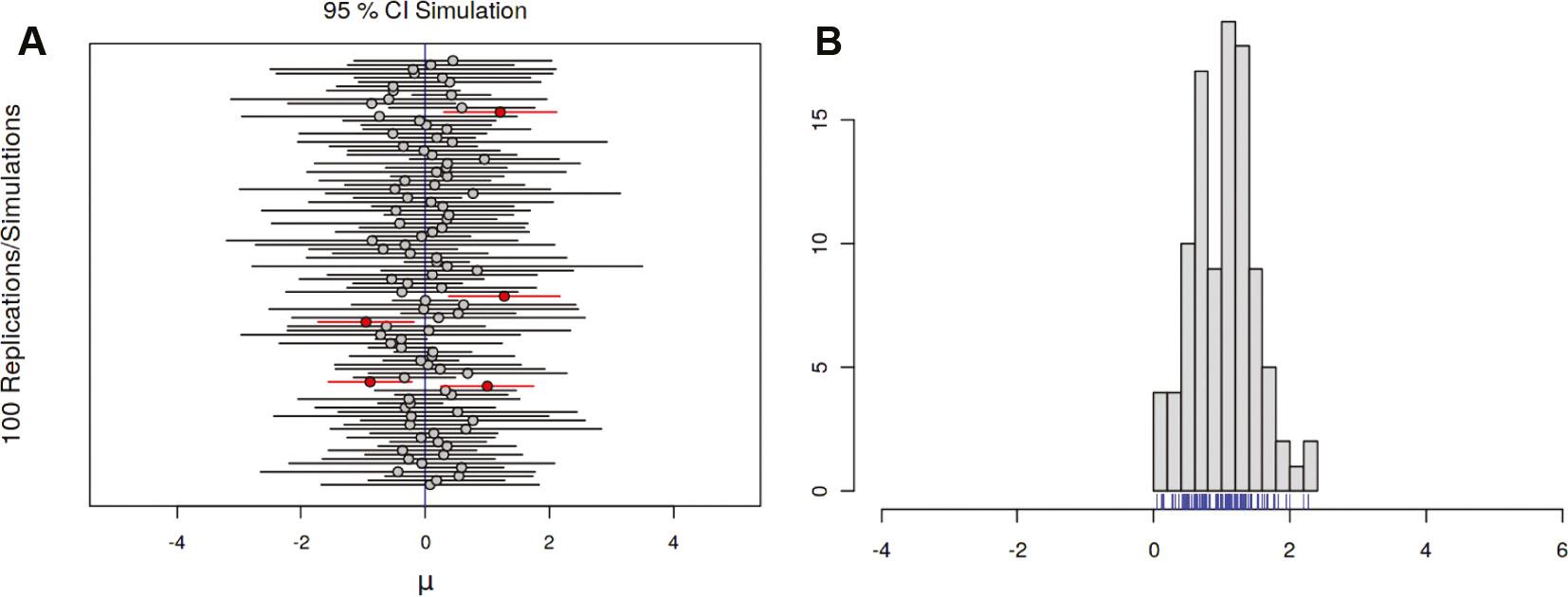
(A) 95% CI for heart rate values. (B) Simulated sample distribution of the mean for heart rate values. Abbreviation: CI, confidence interval.
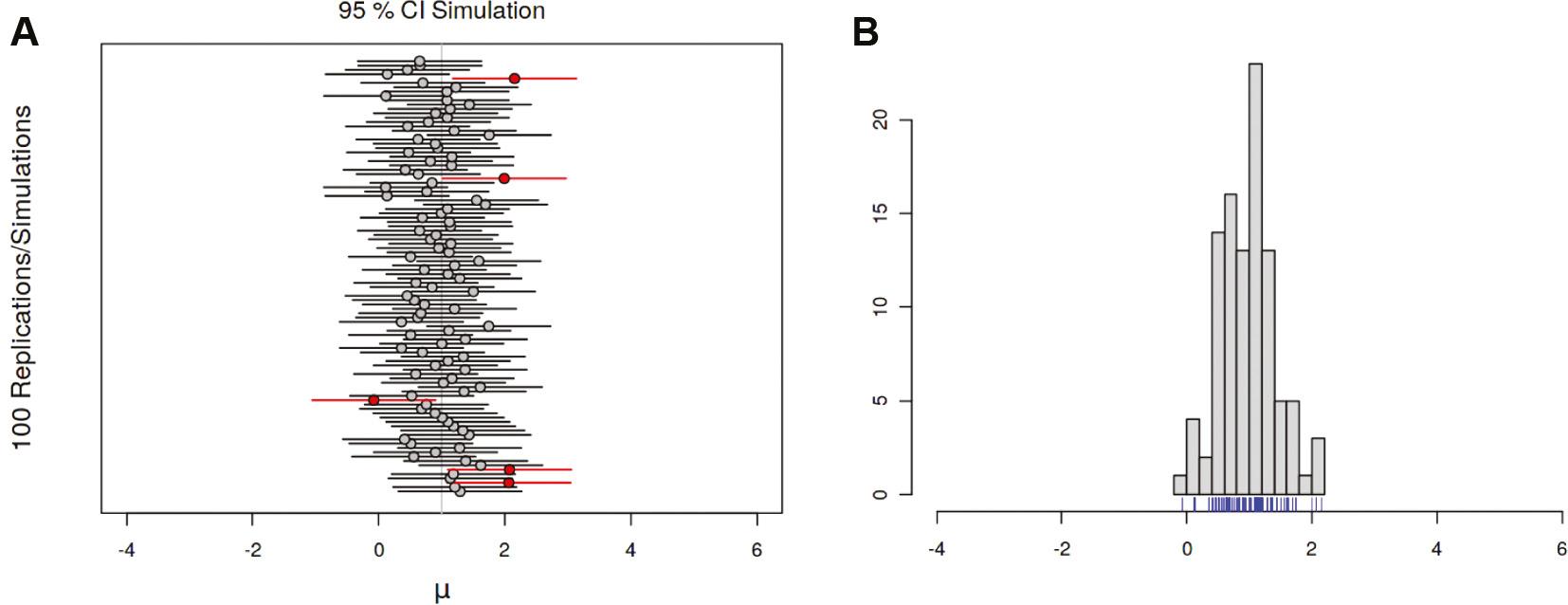
(A) 95% CI for blood pressure values. (B) Simulated sample distribution of the mean for blood pressure values. Abbreviation: CI, confidence interval.
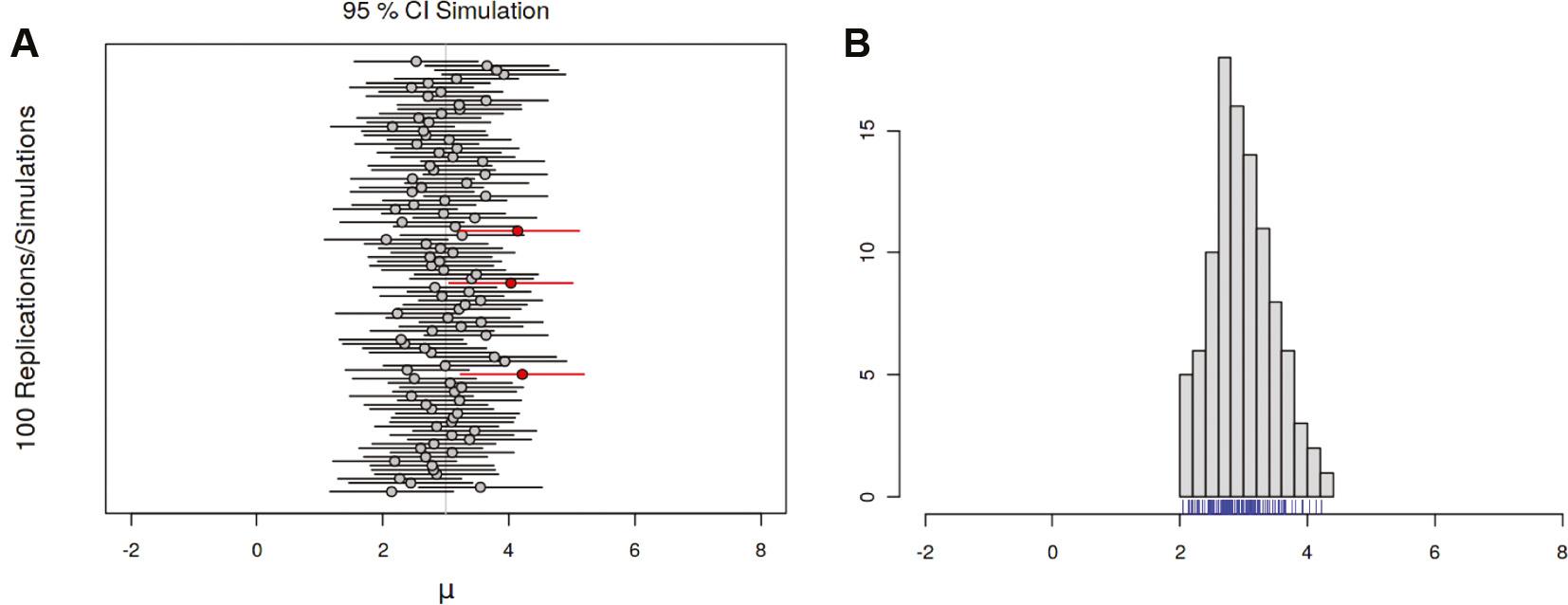
(A) 95% CI for oxygen level values. (B) Simulated sample distribution of the mean for oxygen level values. Abbreviation: CI, confidence interval.
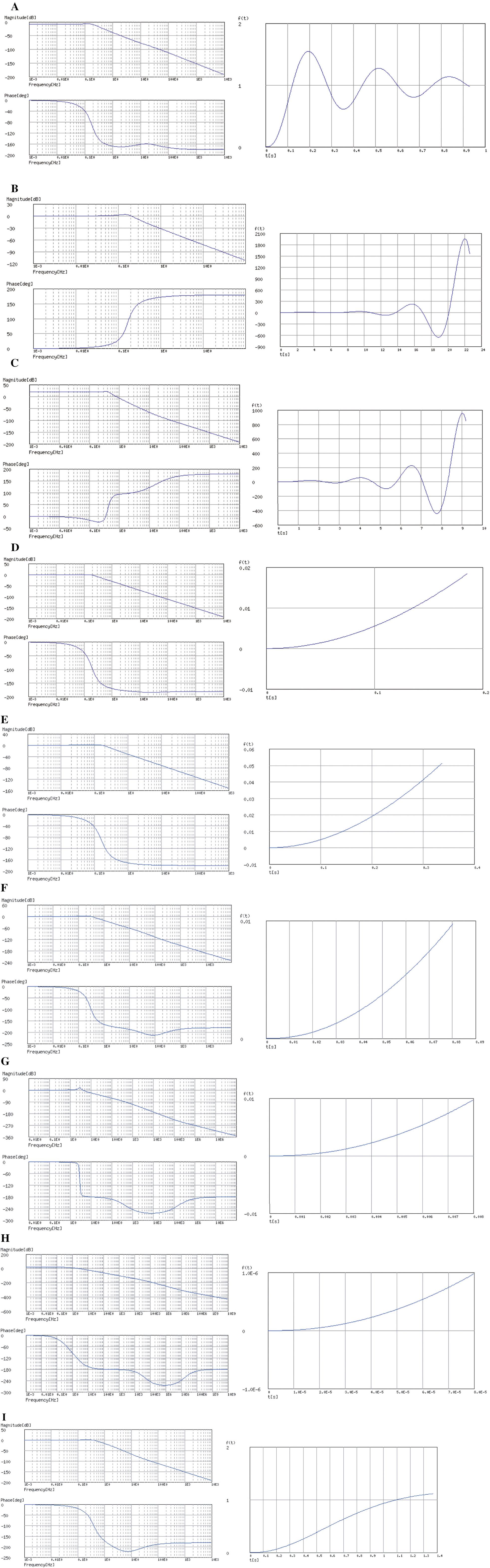
(A) Magnitude, phase (left) and step (right) response of the function yH(n, t). (B) Magnitude, phase (left) and step (right) response of the function yB(n, t). (C) Magnitude, phase (left) and step (right) response of the function yO(n, t). (D) Magnitude, phase (left) and step (right) response of the transfer function T(B → H). (E) Magnitude, phase (left) and step (right) response of the transfer function T(H → B). (F) Magnitude, phase (left) and step (right) response of the transfer function T(H → O). (G) Magnitude, phase (left) and step (right) response of the transfer function T(O → H). (H) Magnitude, phase (left) and step (right) response of the transfer function T(B → O). (I) Magnitude, phase (left) and step (right) response of the transfer function T(O → B).
RESULTS AND DISCUSSION
This research work first assesses the dynamic parameter estimation’s precision and presents a transfer function-based approach to show the dependency among the studied physiological signals in relation to their standard values (i.e. normal heart rate, normal blood pressure and normal oxygen level). To achieve a similar quality standard, it is important to calculate the mean square error (MSE) of the estimated parameter coefficients which is given as
MSE(H)=1T(n)P∑p=1(Hp(s(n,t))−Hnormal)2
Values of various evaluation metrics for the dynamic parameters (heart rate, blood pressure and oxygen level).
MSE | 95% CI | Marginal probabilities Pr(s(n,t) = j) | |
---|---|---|---|
Heart rate | 0.001 | 0.78 | 0.73 |
Blood pressure | 0.005 | 0.81 | 0.78 |
Oxygen level | 0.004 | 0.84 | 0.82 |
Abbreviations: CI, confidence interval; MSE, mean square error.
The susceptibility to anomalies or abnormalities in the data is a significant issue when determining time series and dynamic parameters. Since errors or anomalies frequently taint biological signals (Möller et al., 2003; Giannakakis and Nikita, 2008; Faes et al., 2010; Varotto et al., 2012; Zoubir et al., 2012), researchers frequently exclude polluted observations, which cause substantial data loss. A robust statistical measure has been used, i.e. the maximum bias curve (MBC), which is used to assess the findings’ resilience against outliers (Zoubir et al., 2012). This signifies the greatest level of exponential bias that can arise from a specific sequence of data points. The suggested model cannot be easily applied to the traditional meaning of MBC (Maronna et al., 2006) because the nonstationarity of the data must be taken into consideration which yields MBC(H → B) = max{T (B → H), T(H → B)}, MBC(H → O) = max{T(O → H), T(H → O)}, MBC(B → O) = max{T(O → B), T(B → O)}, is a set of nearby points in the space of circulation dynamics, representing variations in dynamic parameters that are interrelated. The MBC thus also catches brief stability brought on by variations in the data in the nonstationary situation in terms of values recorded. Table 2 presents the values of various evaluation metrics.
Values of various evaluation metrics.
Parameter | Recorded value | Nonsevere value | Severe case values |
---|---|---|---|
Gain | 0.04 | <0.05 | >0.05 |
MBC(H→B) | 0.001 | <0.01 | >0.01 |
MBC(B→H) | 0.012 | <0.01 | >0.01 |
MBC(H→O) | 0.015 | <0.01 | >0.01 |
MBC(O→H) | 0.011 | <0.01 | >0.01 |
MBC(B→O) | 0.013 | <0.01 | >0.01 |
MBC(O→B) | 0.011 | <0.01 | >0.01 |
In reality, the MBC cannot be calculated mathematically, not even for straightforward (stationary, lower-order) dynamic models (Maronna et al., 2006). Therefore, this work used a Monte Carlo method similar to that described in Maronna et al. (2006) to estimate at each time step. Over all Monte Carlo simulations and temporal occurrences, the empirical MBC shows the worst-case bias. The actual MBC maintains the meaning of the specification provided in the equation by selecting any variation in the outlier-producing process for all time instances.
The evaluations of the time-series responses for the parameters H, B and O have been observed to be an approximation that is marginally closer to the actual parameter value, and the precision is very comparable. The outcome of the nonlinear analysis for the proposed model effectively captures the time-varying partial connectedness between signals. The experiments used 100 simulations of the data to assess statistical significance, and the 95% confidence level of the empirical distribution under the null hypothesis of nonlinearity was found to be 0.05.
Transfer functions are a useful tool for ensuring more accuracy in smart bed monitoring when dealing with variability across small datasets. Transfer functions are mathematical models that may be used to anticipate the output of a system based on the input data. This can be done by transferring information from one system to another. This work develops a model that accounts for the variability present in your limited dataset by making use of transfer functions and then utilises that model to generate more accurate predictions using the data.
CONCLUSION
The suggested technique is applied to analyse the biological time-series signals from patients’ smart beds. Continuous tracking of bodily signs, such as heart rate, blood pressure and oxygen level, has evolved into the gold standard in intensive care facilities for patients. Because the heart rate, blood pressure and oxygen level readings and their interdependencies can be analysed using the proposed model from real smart bed settings and the instruments are susceptible to patient motions and smart bed orientations, for this work, 10 cases were taken into account. Three signals—heart rate, blood pressure and oxygen level—have been observed. To measure the linear and nonlinear information transmission among HR, BP and oxygen level patients, the proposed method is used. For actual information, choosing the best model settings is more complicated than it is for synthetic data. MBC evaluation is used as an average optimal option that can be identified because this work deals with three types of data. The characteristics of a time-varying system and its transient behaviour have been plotted. Using the proposed estimate, it was possible to predict the ideal values of the dynamic variability. The proposed method has the advantage that it allows researchers to see both linear and nonlinear changes in physiological data at the same time. The observed condition of the patient is found to be quite similar to the expected linear and nonlinear causes. However, there are situations when a lot of nonlinear variability was seen despite there being very little linear fluctuation. In other words, no linear variational measure can identify the existence of nonlinear correlations between physiological data.
The significance of modelling in machine learning (ML) and artificial intelligence (AI) technologies cannot be overstated. ML, a kind of AI, may automate the creation of analytical models using data, thereby contributing to the expansion of the economy. Each method has its place, whether it is for detecting spam-like emails or for segmenting customers for use in marketing or other business tactics. The ultimate objective of artificial intelligence is to train computers to mimic human intelligence by engaging in “intelligent behaviours.” However, in this paper, the mechanistic basis for smart beds to observe the time-varying dynamics of physiological signals has been observed and the application using AI or ML could be an exciting future work.