INTRODUCTION
Autism spectrum disorder (ASD) is a neurodevelopmental disease that typically manifests in human beings between the ages of 1 and 3 years (Landa et al., 2013). Limited interests and repetitive conduct are other hallmarks of the disorder, as are difficulties in social interaction and communication (Belmonte et al., 2004). Those who have ASD struggle to comprehend the emotions and thoughts of others. People with ASD have a difficult time interacting with other people for various reasons. According to data provided by the World Health Organization, the prevalence of ASD is estimated to be somewhere between 1 in 270 and 1 in 450 persons worldwide (GBD 2019 Diseases and Injuries Collaborators, 2020). Every person with ASD has their own set of distinctive qualities, and some of them have outstanding intellectual, visual, or musical capabilities. In this research context, the most critical procedures that need to be undertaken are those that are necessary to discover ASD and ensure that appropriate treatment begins as soon as possible. These actions are useful for reducing the consequences of this ailment and improving their condition overall. Many sorts of observations may be used to determine whether an individual has ASD.
On the other hand, while early identification is beneficial for providing better therapy for ASD patients, a great amount of time and effort is required. In recent years, approaches in the field of machine learning (ML) have become more prevalent in the process of assessing the symptoms of a variety of serious illnesses, such as heart disease, diabetes, and cancer, among others. As a consequence, several research works have looked at various methods for identifying people with ASD and lessening their impacts in a more focused way (Hyde et al., 2019; Usta et al., 2019). There are a variety of tools available for differentiating ASD, but behavioral tests are the gold standard and the most widely acknowledged. These evaluations are arduous and time-consuming procedures that need the participation of teams with expertise across several disciplines (multidisciplinary teams) (Usta et al., 2019). This is due to the fact that ASD diagnoses are notoriously difficult to pin down in the first place, as it encompasses a diverse set of symptoms. This has prompted several efforts across disciplines, including genetic determination (Hyde et al., 2019), primary analysis of brain pictures, and physiological techniques, all with the aim of creating objective and reliable diagnostic procedures.
In order to enhance the quality of life for a person with ASD, it is crucial to diagnose and treat the condition. Unfortunately, because of the time lapses that occur between the first worry and the diagnosis, a significant amount of precious time is lost while the illness is not identified. ML techniques not only help provide a rapid and accurate assessment of the risk for ASD, but they could also be vital for streamlining the whole process of diagnosis and assisting families in gaining access to treatments more quickly. Many recent articles (Alom et al., 2019) have shown the promise of automated categorization approaches based on ML techniques, which were developed using the uniqueness of voice features. This is due to the fact that ML algorithms have progressed and improved to the point where they can successfully carry out state-of-the-art classification and discriminating tasks (Song et al., 2019). Previous works (Santos et al., 2013) have employed several different acoustic–prosodic variables to illustrate the different kinds of ML classifications. The signal’s fundamental frequency, formant frequencies, harmonics, and root mean square energy were all taken into account. The classifiers used in the research, which consisted of both support vector machines (SVMs) and probabilistic neural networks, did an excellent job of separating children with ASD from typically developing children. The authors in (Li et al., 2019) used three classification methods based on artificial intelligence algorithms, namely, recurrent neural networks (RNNs) and convolutional neural networks (CNNs) and RNNs to detect children with ASD.
ML can be used to separate the population into those with and without ASD with a high degree of accuracy. By analyzing large amounts of data, ML algorithms can identify patterns and characteristics that are common among individuals with ASD. These algorithms can then be used to develop predictive models that can accurately classify individuals as either having or not having ASD, based on their unique characteristics and behaviors. However, it is important to note that ML should not be used as a substitute for clinical diagnosis by a trained healthcare professional. Rather, it can be used as a tool to assist in the diagnostic process and provide additional insights into the underlying mechanisms of ASD. Overall, ML methods use complex algorithms to analyze large amounts of data and identify patterns that are associated with ASD. By using these patterns to create a predictive model, ML methods can accurately separate the population into those with and without ASD.
The ML algorithms were proposed in this research to investigate ASD in children at an early stage. The proposed model also investigates the individual characteristics of these groups of people in a more efficient manner. The results were then compared, and the classifier with the highest average score was selected for each age group, as well as the SVM and random forests (RF) methods with the best overall performance. The statistical analysis test was also used to assess the performance of these classifiers. A condensed overview of the study’s contributions is as follows:
We suggested an effective approach to ML for detecting ASD with a high level of accuracy at an early stage.
We focused our attention on locating the essential feature that elaborates on the distinctions between various features to understand whether specific characteristics contribute to accurate ASD diagnoses.
To provide a justification for the classifier’s performance, we used a statistical approach and examined the significance of the classifier.
Using this strategy helps diagnose ASD in a manner that is both straightforward and adaptable.
BACKGROUND OF THE STUDIES
There are several screening techniques available for assessing ASD in children, infants, and adults (Baron-Cohen et al., 2001; Baron-Cohen et al., 2005; Woodbury-Smith et al., 2005; Eaves et al., 2006; Eriksson et al., 2013; Sappok et al., 2015; Thabtah, 2018; Thabtah and Peebles, 2019; Chakraborty et al., 2020). In a clinical sample consisting of 132 patients who had been submitted for clinical diagnostic evaluation, Kenny and Alison (2016) found that the autism-spectrum quotient (AQ) scores did not change based on whether the patients were diagnosed with ASD or a diagnosis other than ASD, following the comprehensive examination. The predictive efficacy of the AQ was recently studied by Adamou et al. (2021), who compared it with the final diagnosis formulation by expert interdisciplinary teams. This study was conducted on a sample of people who were referred to a specialized diagnostic service. The sensitivity of the AQ was assessed at 74%, while its specificity was reported to be 30.3%. There was no discernible evidence of a significant connection between the AQ scores and the diagnostic results. In a sample of 476 individuals with ASD, Ashwood et al. (2016) found that the values of sensitivity (77%) and specificity (29%) were comparable. Only 17% of the sample in a study of AQ scores in people diagnosed with ASD who were of average or below-average intelligence met the diagnostic threshold on the AQ. The study was conducted on persons with ASD with average or below-average intelligence. This once again demonstrates a much-reduced sensitivity in comparison to the one found in the first research (Bishop and Seltzer, 2012). The purpose of the research was to investigate the AQ scores of persons who had been diagnosed with ASD and who had average or below-average intelligence. The Autism Diagnostic Interview-Revised and the Vineland scale are two more common tools for assessing ASD, although it has not been shown that AQ scores correlate with these instruments. These evaluations include the Vineland scale and the Autism Diagnostic Interview-Revised. Thabtah et al. (Thabtah et al., 2018; Satu et al., 2019; Akter et al., 2021a; 2021b) created a mobile application for iOS and Android devices called ASDTests for the purpose of gathering information on ASD in children. This system was developed using the Q-CHAT and AQ-10 diagnostic tests to determine whether a person has ASD. They used this program to compile information on ASD, which was subsequently added to machine learning repository ML database. Omar et al. (2019) presented a practical method for ML; in their study, they used RF, classification and regression trees, and random forest-Iterative Dichotomiser 3 to analyze the AQ-10 dataset as well as 250 real-world datasets, and they came to the following conclusions: (ID3). Sharma (2019) used the chi-square feature selection (CFS)-greedy stepwise feature selector to conduct research on these datasets. To analyze these datasets, the researchers used a variety of ML techniques, including stochastic gradient descent, naive Bayes (NB), k-nearest neighbors (KNN), random tree, and K-star. Researchers in the study by Satu et al. (2021) collected data from a wide range of young people (16-30 years old), evaluated them using many tree-based classifiers, and extracted numerous rules for normal and autism. KNN, SVM, and RF were all tested on the same datasets in the work by Erkan and Thanh (2019); however, RF was the most effective in spotting cases of ASD. Thabtah et al. (2019) constructed multiple feature subsets of ASD adults using significant chi-square (CHI) and information gain (IG) approaches. After that, they used logistic regression (LR) to diagnose ASD within those feature subsets. Before making any changes to the sets, Akter et al. (2019) collected data from preschoolers, children, teenagers, and adults. The data were then put through a number of different classifiers, with SVM demonstrating the highest level of performance for the toddler, and Adaboost showing the highest level of performance for both children and adults. Glmboost also worked very effectively with individuals in their teenage years. Hossain et al.’s (2020) CFS, CHI, IG, One-R, and Relief-F are only some of the methods that have been used to generate subsets of linked datasets for the purpose of conducting research. Linear regression, multilayer perceptron, and sequential minimum optimization (SMO) were all suggested. Then, SMO’s highest accuracy was found on datasets containing adults (97.58%), followed by scores of 91% on datasets containing children (91%), and 99.9% on datasets containing adolescents (91%). Raj and Masood (2020) used SVM, LR, NB, and a CNN to examine these datasets; however, they did not include children under the age of 3 years. CNN had the highest accuracy at 98.30% for children, 96.88% for adolescents, and 99.53% for adults.
METHODOLOGY
Figure 1 shows the framework of the diagnosis of children with ASDs based on ML approaches.
Dataset
An app for autism screening named ASDTests was created by Thabtah et al. (2018) from the Nelson Marlborough Institute of Technology. This app was utilized for data gathered from the target population of children. The dataset was collected from an ML repository. The dataset contains 20 features and classes (normal or ASD). The AQ-10 questionnaires and demographic information about the children were used to evaluate the variables that increase the likelihood of having ASD. Table 1 contains a summary of the particular characteristics of these datasets that were taken into account throughout the study.
Features of the dataset.
Attributes | Type |
---|---|
Age | Integer |
Gender | String |
Ethnicity | String |
Born with jaundice | Boolean |
Family member with PDD | Boolean |
Who is completing the test | String |
Country of residence | String |
Used the screening app before | Boolean |
Screening method type | Integer |
QSA1 | Binary |
QSA2 | Binary |
QSA3 | Binary |
QSA4 | Binary |
QSA5 | Binary |
QSA6 | Binary |
QSA7 | Binary |
QSA8 | Binary |
QSA9 | Binary |
QSA10 | Binary |
Class | Integer |
Abbreviation: PDD, pervasive developmental disorder.
Figure 2 shows the percentage of people from each gender—male (0) and female (1)—who were on the autism spectrum. As females make up the vast majority, we may safely assume that males are more likely to be diagnosed with ASD.
The most common age of ASD started from year 1 to year 7, as shown in the dataset. Figure 3 displays the sex ratio of autistic patients and may be seen in its entirety here. While the collected data demonstrate a gender balance that is equal for healthy patients, one can conclude that there is an imbalance between the sexes for patients who are influenced by autism. The small sample size may have contributed to this result.
The dataset was collected from different countries, and Figure 4 shows ASD data for most of the world’s countries. Most Arabic countries rank near the average. It shows the percentage of ASD in different countries worldwide.
Preprocessing
Data preprocessing is a phase in the process of data mining and data analysis that involves clearing the dataset from null values, converting the categorical features to numerical features, using an encoding method, and transforming raw data into a format that can be understood by computers and analyzed through ML. This step takes raw data and transforms it into a format that can be used. Figure 5 shows the processing steps.
Machine learning algorithms
K-nearest neighbor algorithm
KNN is an example of a technique under the category of supervised ML. The fundamental idea is as follows: if the majority of the samples that are k-nearest to a sample also belong to a certain category in the feature space, then the sample itself must also belong to that particular category if it is to be consistent with the rest of the k-nearest samples. These are the major elements that make this up: the first step is to determine how similar the test sample is to all of the training samples; the second step is to identify the k-training samples that are most similar to the test sample, and the third step is to assign a category to the test sample based on the category that was assigned to the k-training samples by applying the minority-obeys-the-majority principle. The new data point x is the one for which we want to make a prediction about the label (Saadatfar et al., 2020). KNN’s primary role is to find the k-training data points closest to x in terms of a Euclidean distance metric. The KNN algorithm then holds a vote with a simple majority to decide what label should be assigned to the new data point x:
where x 1, x 2, x 3, and x 4 are the input data variables.
Linear discriminant analysis
The linear discriminant analysis (LDA) method is used to determine the linear discriminant function that most effectively classifies, differentiates, or separates two classes of data points. LDA is a supervised learning method, which means that in order for it to learn the linear discriminant function, it needs a training set of data points that have been labeled with their respective values (Zheng et al., 2020). After the linear discriminant function has been learned, it may be used to forecast the class label of fresh data points. This can be done once the function has been learned. LDA is comparable to principal component analysis, in that it works to minimize the number of dimensions being considered. The primary objective of LDA is to locate the line (or plane) that most effectively partitions the data points that belong to the various classes. LDA’s central tenet is that the decision boundary ought to be selected in such a way that it maximizes the distance between the means of the two classes while simultaneously reducing the variation within each class’s data, often known as within-class scatter. Figure 6 shows how the LDA algorithm works to classify the classes, the graphical representation of the two classes: normal and ASD.
Support vector machine
The SVM technique is used in the classification of ASD. It is predicated on the concept of finding the hyperplane that separates a certain dataset into two groups in the most effective manner possible. The margin refers to the distance that must be traveled from the hyperplane to the next available training data point (Bala et al., 2022). The SVM seeks to locate the separation hyperplane that provides the highest possible margin for the training data. We started our training with a linear RBF kernel, then compared it with a nonlinear kernel later on. We found that the linear kernel produced better results overall:
The notation X, X′ denotes a feature vector that is used in the process of training an algorithm on a dataset. This feature vector is also used in the evaluation process of the dataset. The Euclidean distance method used to find a difference between two feature inputs is referred to as an
(X−X′∥2)
Random forest tree
The RF tree, which is widely considered to be one of the most well-known ML algorithms, falls within the broader category of supervised learning. One must first have some experience with decision trees in order to make any headway toward having a thorough comprehension of RFs. A decision tree is a hierarchical structure that is used for the purpose of classifying data from the most relevant to the least relevant (Ahsaan et al., 2022). This is accomplished by placing the most relevant data at the top of the tree. The primary object here is to arrange everything in the simplest way that may be done effectively within the constraints of the situation. The RF classifier is an adaptable technique that may be used for a variety of purposes, including classification, regression, and others. In order for it to function, numerous decision trees are constructed based on random data points. After obtaining the forecast from each tree, voting was used to choose the option that provided the best results.
Evaluation metrics
It was proposed that the effectiveness of the diagnosis system be evaluated using a variety of metrics, including precision, recall, F1-score, mean square error (MSE), determination coefficient (R2), and root mean square error (RMSE). The following are some common types of measuring systems:
where FP, FN, TP, and TN are false positive, false negative, true positive, and true negative, respectively, and yi,target is the target value while yi,pred is the prediction value.
Experiments
Here, we compare the accuracy, precision, recall, F1-score, MSE, RMSE, and R2 of the KNN, LDA, SVM, and RF classification methods. The classification algorithms were used through their paces in both a binary classification scenario and a multiclass environment. The binary classification looked at the dataset from two different perspectives: normal or ASD. Using a collection of 20 features, the researchers were able to make binary classifications, and the correlation method was utilized to establish the strength of the association between the features. The results from the proposed algorithms were compared with those of several other existing methods. The following section details a more extensive experiment performed as part of this study.
Splitting dataset
High accuracy performance was achieved by splitting the dataset into training and testing halves of 80% and 20%, respectively. Table 2 shows the splitting of the dataset. We recognize the complexity of ASD data, and the suggested ASD system has been built on specialized hardware and software.
RESULTS
Table 3 shows the results of KNN to classify ASD in children. The result of KNN was not satisfactory; it scored only a 52% accuracy. The accuracy performance of KNN between the target value and the predicted value is presented in Figure 7. It is noted that there was high misclassification; therefore, the performance of KNN was not good. Finally, the KNN approach is an appropriate classification approach for detecting ASD.
Performance of KNN approach to detect ASD.
Labels | Precision % | Recall % | F1-score % |
---|---|---|---|
Normal | 42 | 68 | 52 |
Autism | 57 | 31 | 41 |
Accuracy | 52 | ||
Weighted average of KNN | 51 | 47 | 45 |
Abbreviations: ASD, autism spectrum disorder; KNN, k-nearest neighbors.
Among the many effective linear ML methods for dealing with linear data, the LDA stands out. The LDA algorithm was applied to detect ASD in children. Table 4 shows the results of LDA in classifying ASD. The algorithm achieved a high percentage better than the KNN approach, where the accuracy is 88%. The performance of the LDA method is displayed in Figure 8.
Performance of LDA approach to detect ASD.
Labels | Precision % | Recall % | F1-score % |
---|---|---|---|
Normal | 82 | 89 | 86 |
ASD | 92 | 86 | 89 |
Accuracy | 88 | ||
Weighted average of LDA | 88 | 88 | 88 |
Abbreviations: ASD, autism spectrum disorder; LDA, linear discriminant analysis.
The results of the linear SVM strategy for detecting ASD are shown in Table 5. SVM obtained a high percentage of accuracy (100%), as shown. Finally, linear SVM is appropriate for ML to detect ASD. The performance of linear SVM is shown in Figure 9. The target values are close to the predicted values; therefore, the linear SVM approach is one of the algorithms suited for detecting ASD.
Performance of SVM approach to detect ASD.
Labels | #Precision % | #Recall % | #F1-score % |
---|---|---|---|
#Normal | 100 | 100 | 100 |
#ASD | 100 | 100 | 100 |
#Accuracy | 100 | ||
#Weighted average of SVM | 100 | 100 | 100 |
Abbreviations: ASD, autism spectrum disorder; SVM, support vector machine.
The result of the RF algorithm is presented in Table 6. The RF approach scored a high percentage of 100% with respect to all the metrics, namely, precision, recall, F1-score, and accuracy. Figure 10 shows the performance of the RF tree algorithm.
Performance of RF approach to detect ASD.
Labels | Precision % | Recall % | F1-score % |
---|---|---|---|
Normal | 100 | 100 | 100 |
ASD | 100 | 100 | 100 |
Accuracy | 100 | ||
Weighted average of RF | 100 | 100 | 100 |
Abbreviations: ASD, autism spectrum disorder; RF, random forests.
In Figure 11, we see the confusion matrix for ML, namely, KNN, linear discriminant, SVM, and RF methods. Many measures, including false negatives, true positives, and true negatives, are reported in the matrix to indicate the algorithm’s effectiveness. The two possible classes are “normal” and “ASD” [0, 1]. The TP rate from the KNN approach is 21.59%, TN is 28.41%, and FP is 36.36%, indicating that the FP is very high. In the LDA approach, TP is 46.59%, TN is 38.64%, and FP is 11.36% and it is better than the KNN approach, whereas the false positive of SVM and RF tree was 0.00, showing the high performance of the SVM and RF algorithms.
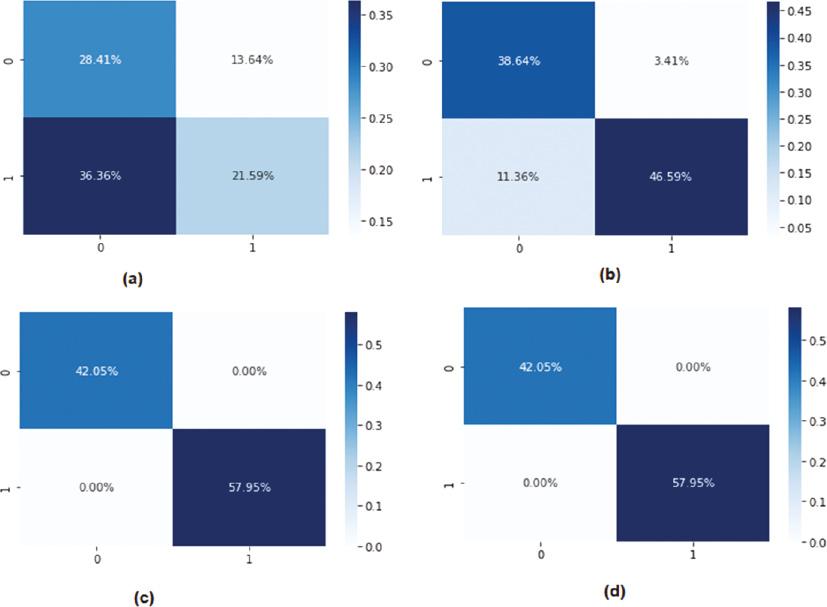
Confusion metrics of ML: (a) KNN, (b) LDA, (c) SVM, and (d) RF approaches. Abbreviations: KNN, k-nearest neighbors; LDA, linear discriminant analysis; ML, machine learning; RF, random forests; SVM, support vector machine.
Result of statistical analysis
A method known as sensitivity analysis may be used to calculate an estimate of the degree of uncertainty associated with the input data variables. Examining the data that were used in the study is of great assistance when it comes to the process of identifying the patterns within a dataset. A sensitivity analysis may determine the different kinds of normal and ASD in the dataset. Furthermore, it may be used instead of the traditional technique to attribute changes in the system outputs to particular sources of uncertainty in the system inputs. This can be done in place of the conventional method. This study revealed, with the use of Pearson’s correlation coefficient, that there was a substantial association between the quality of input and the classes to which individuals belonged. There were significant correlations between the features of datasets and classes, as presented in Figure 12. The statistical analysis results between the target values and prediction that were obtained from ML algorithms are presented in Table 7. SVM and RF approaches scored R 2 = 100%, according to the coefficient of determination.
DISCUSSION
ASD, sometimes known simply as autism, is a neurodevelopmental condition that causes individuals to have poor interaction and communication abilities. Autism may be diagnosed at any point in a person’s life; however, owing to the high incidence of early-onset symptoms, the majority of autism diagnoses are made during the first 2 years of life. ASD is a developmental disease that is thought to make its first appearance in early childhood and continues throughout adulthood. Research on ASD has been pushed forward by the increasing use of methodologies that include ML, in medical diagnostic research.
In this piece of research, an effort was made to investigate the viability of using KNN, LDA, SVM, and RF trees to anticipate and analyze ASD-related difficulties in a child. The suggested methods were examined using three distinct datasets that are not clinically relevant and are accessible to the public. The suggested adjustment to the ML was investigated using the standard. The dataset concerning the screening of children for ASD has 292 different cases and 21 different features. After employing a variety of ML strategies and accounting for missing data, the results strongly suggest that SVM- and RF-based prediction models work better on datasets, displaying a higher accuracy of 100% in terms of screening children within the data for ASD. This was discovered after applying four types of ML approaches.
The obtained results of the proposed ML is compared with comparable earlier research and are shown in Table 8. The majority of the previous studies were conducted using the version-1 ASD datasets that were created by Thabtah et al. (2018). The enhanced SVM and RF scored an accuracy of 100%, which is better than the existing ASD systems (Fig. 13). Early diagnosis of ASD was improved by using the proposed ML framework, which was shown to be both accurate and time-saving. Recognizing the signs of ASD in children is a costly and time-consuming procedure that is frequently put off because of the challenges involved in making the diagnosis. In this study, ML algorithms performed very well in identifying the early signs of autism.
Evaluation of the suggested ASD system versus existing methods for dealing with ASD.
References | Algorithms | Dataset | Accuracy % |
---|---|---|---|
Chen et al. (2022) | Random forest | Same dataset | 77.75 |
Bala et al. (2022b) | SVM | Same dataset | 98.62 |
Bala et al. (2022a) | SVM | Same dataset | 99.61 |
Akter et al. (2019) | SVM | Same dataset | 97.20 |
Proposed system | SVM_RF | Same dataset | 100 |
Abbreviations: ASD, autism spectrum disorder; RF, random forests; SVM, support vector machine.
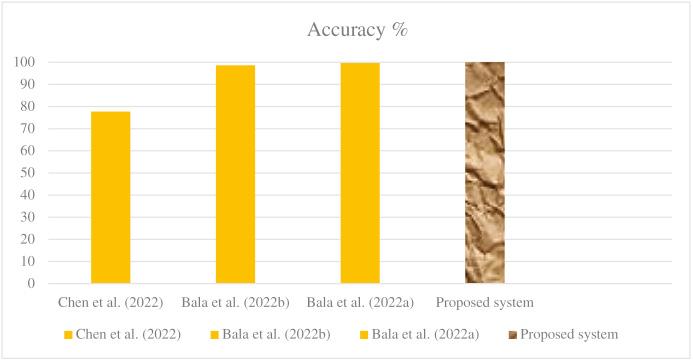
Performance of ASD system against existing ASD system in terms of accuracy. Abbreviation: ASD, autism spectrum disorder.
Limitation of research work
Furthermore, this study did not take into account the potential influence of the cultural and linguistic differences on the accuracy of the model. As ASD is a complex and multifaceted condition, it is possible that certain cultural or linguistic factors will affect the presentation of symptoms and thus influence the accuracy of diagnostic models.
Additionally, the study only focused on a limited set of variables and did not consider other potential factors that may contribute to the development or diagnosis of ASD. For example, environmental factors such as exposure to toxins or prenatal complications may also play a role in the development of this condition.
Future research should focus on validating the model with external databases and testing methods to determine its generalizability and potential for widespread use in detecting ASD. This research can also be extended by collecting clinical data from Saudi Arabian hospitals.
CONCLUSION
Screening at an earlier age is one of the factors that contributes to the identification of children with ASD at a younger age. We conducted comprehensive screening for ASD in a community setting with a sizable number of participants over a period of time. The goals of this study were to determine the diagnostic rate of ASD screening and to determine the effectiveness of a model for ASD screening in a community-based sample. It is essential to detect ASD at an early age by developmental monitoring, screening, and assessment to ensure that a child who has ASD can receive the necessary care and support in order to flourish. Because of this, we are working on developing a system that is capable of independently diagnosing autism and obtaining a definitive, correct diagnosis without the need for any interaction with a human being. We hope that this technique will be of great use to schools and other professionals, as well as for calming the minds of nervous parents who are interested in the growth and locations of their children. According to the findings of the experiments, the best results may be achieved using a classifier SVM and RF. It is even more important that the SVM and RF algorithm performance measures led to 100% accuracy across the board for datasets. On the contrary, the KNN approach yielded the worst possible outcomes. Our results provide credible data that may be used for assessing the incidence of ASD detection and determining the viability of a community-based screening methodology. This research can also be extended by collecting clinical data from Saudi Arabian hospitals.