Introduction
Global warming and consequent climate change are major environmental challenges currently facing society. There is strong scientific consensus that warming of the climate is caused by CO2 and other greenhouse gas (GHG) emissions from human activities (IPCC, 2021; Lynas et al., 2021). To curb climate change, reductions in global GHG emissions of 90% or greater are required in the forthcoming decades according to the Intergovernmental Panel on Climate Change (IPCC, 2018). In parallel, CO2 removal and storage (i.e. sequestration) in soils and vegetation will have to increase substantially to neutralise residual emissions (IPCC, 2018; ESABCC, 2024). By now, most countries and corporations have accepted the GHG mitigation recommendations of the IPCC. This advice has been adopted by the European Union (EU) through the bloc’s climate law (European Parliament, 2021) and incorporated into the Climate Action and Low Carbon Development Act (2021) by Irish policymakers. Both the EU and Ireland have committed under current laws to being climate neutral, that is economies with net-zero GHG emissions by 2050.
Public and private companies throughout Ireland, the EU and other countries are supporting the transition to a low GHG or carbon society. Many companies have produced climate strategies with roadmaps for net-zero emissions. Generally, these climate strategies encompass emissions from scope 1, 2 and 3 activities as defined in the GHG protocol (WRI/WBCSD, 2011). Scope 1 refers to direct GHG emissions from on-site operations. Scope 2 is indirect emissions from energy purchases, and scope 3 is other indirect emissions that occur within a company’s supply chain. The latter scope often comprises the largest share of an organisation’s total GHG emissions (Matthews et al., 2008; Hertwich & Wood, 2018). For land-based food and beverage companies, scope 3 emissions are primarily generated during the production of agricultural commodities (Hansen et al., 2022).
Grain crops are an essential agricultural commodity for a broad array of businesses. They are also a vital income stream for farmers. Based on the latest global statistics, primary production of grain crops is worth over €1.2 trillion to the world economy (FAO, 2024). In Ireland, crop production contributes about €1.3 billion per annum to the economy, supports 11,000 jobs and ranks as the third- or fourth-largest agricultural subsector in terms of goods output at basic prices (Wallace, 2020; CSO, 2024). The sector is an important component of the Irish agri-food industry, especially in regard to food security (DAFM, 2023). Presently, there are approximately 4,500 Irish farms specialising in crop production (DAFM, 2023). Grain yields on these farms are regularly among the highest in the world (Lynch et al., 2017; FAO, 2024), partly due to a favourable temperate climate. Moreover, they generate relatively low amounts of GHG emissions (Buckley & Donnellan, 2023). Hence, Irish grain crops perform well in terms of climate and environmental impacts.
Nowadays, the climate impact of agricultural production systems is increasingly measured in terms of carbon footprint, that is GHG per unit of product. The recommended method for estimating carbon footprint is life cycle assessment (LCA) (Pandey et al., 2011; ISO, 2018). In short, LCA is a compilation of the inputs, outputs and potential environmental impacts associated with a product, service or organisation throughout its life cycle (ISO, 2006a). This methodology is holistic, structured and internationally recognised. It has been applied to grain cropping systems using computer models of differing complexity. These LCA models concentrate on a single environmental impact or simultaneously consider multiple potential impacts, for example ecotoxicity, eutrophication and finite energy depletion (Goglio et al., 2018). The former type of model, that is single-impact LCA tends to evaluate GHG emissions, which is commonly known as carbon footprinting (Kral et al., 2009; Carbon Trust, 2023).
Some of the main LCA models and GHG calculators that have been deployed to quantify the carbon footprint of grain crops are the Cool Farm Tool, CAP’2ER, SALCA (Swiss Agricultural Life Cycle Assessment) and the Solagro carbon calculator (Goglio et al., 2018; COWI, 2021). The Cool Farm Tool is an online GHG calculator with tens of thousands of users in 150 countries (CFT, 2023). It combines empirical GHG models to give an overall emission estimate for crops and livestock on an area and product basis (Hillier et al., 2011). Cool Farm Tool has a decision support focus that is suitable for use by growers, companies and scientists. CAP’2ER is an LCA tool that provides users with a multi-criteria environmental diagnostic of cash crops, dairy cattle, beef cattle, sheep and goats (IDELE, 2022). This tool is widely applied in France and has been extended to other EU countries, for example Italy and Spain.
The SALCA is a modelling framework that comprises generic LCA tools for cropping systems and farms. Both have a modular structure to carry out batch calculations for dozens of crops or farms (Nemecek et al., 2015). They were engineered by Agroscope and are valid for pedo-climatic conditions in Switzerland and central Europe. The Solagro carbon calculator is an EU-wide tool that operates at the farm scale (Tuomisto et al., 2015). This LCA tool has been designed to be suitable for all the main cropping systems in the EU. It determines GHG emissions for up to five products on a farm and proposes mitigation options and carbon sequestration measures for individual farms. Solagro has been tested on farms in multiple European countries, particularly Spain, the Netherlands and the UK.
Like many agricultural LCA models, Solagro struggles to adequately reflect regional variation in farms (Tuomisto et al., 2015). In fact, none of the aforementioned tools have addressed regional or biogeographical differences between grain growers. Furthermore, several LCA models of agricultural systems rely on global average values to estimate GHG emissions from key sources (Keller et al., 2014), which are unlikely to be representative of Irish cropping systems. They also tend to focus on a limited number of farms and few evaluate commercial enterprises, except for models like CAP’2ER which has been primarily applied to beef and dairy systems (COWI et al., 2021). Therefore, the main objective of this research was twofold: (1) to redevelop an LCA model of the carbon footprint of Irish grain with country-specific information on agricultural GHG emissions and carbon sequestration and (2) to estimate the carbon footprints of native grain crops purchased by Tirlán co-operative (https://www.tirlan.com/). A bespoke LCA model of Irish grain production was expected to deliver better estimates of grain carbon footprints and to provide decision support in relation to mitigating agricultural GHG emissions.
Materials and methods
Grower dataset
The LCA methodology was applied with agricultural activity data from a random sample of grain growers supplying Tirlán co-operative in 2022. Sixty willing grain growers were included in the sample and were stratified by crop type, that is plant species, grade and sowing season. An experienced farm recorder gathered activity data from commercial grain growers. This process took about half a working day to complete when a grower had accountancy books and physical records to hand. The farm recorder electronically entered this activity data into a Microsoft Excel form. The data entry form had 65 questions per crop with response options provided in drop-down menus. It captured a maximum of 450 data points (75 per crop) under the following seven sections: (1) crop information, (2) straw and cover crops, (3) soil information, (4) crop production and protection, (5) gross income, (6) precision farming and (7) biodiversity.
The first section of the form recorded crop type, variety, area, seeding rate, rotation, yield, moisture content, drying method and storage period. Section 2 covered straw chopping, incorporation and removal and included cover crop planting rates and destruction methods. Soil information was captured in section 3 in terms of topsoil texture, pH, nutrient indexes and cultivation techniques, for example plough-based, minimum tillage and direct drill. Section 4 on crop production detailed the types and amounts of fertilisers and organic manures applied, liming rates and the number of tractor passes of fertilisers and crop protectants. Section 5 recorded grain and straw incomes. Precision farming was covered in the next section in terms of automated technologies used on farm, for example remote sensing systems. The last section detailed agricultural areas dedicated to biodiversity.
The data entry form was populated with information collected directly from the foreground system, that is grain growing. Prior to running the dataset through the LCA model, it was cleaned and verified. Where necessary, data points were cross-checked with interested growers. Following this verification step, 12 farms were removed from the sample due to inadequate information. The 48 remaining grain growers were dispersed throughout Tirlán’s catchment in the southeast, east and midlands of Ireland. Most growers (90%) were larger than the national average tillage farm (70 ha) and in the top end of the distribution according to the national farm survey (Dillon et al., 2023). The sample was hence reflective of large-scale growers, making up about 4% of Tirlán co-operative’s 1,100 grain producers for 2021 to 2022 growing season.
LCA model
A prototype LCA model, the zero warming tillage model, was revised to determine GHG emissions and removals, that is carbon sequestration in grain production systems. The basic design and components of this initially unnamed prototype model were described within an LCA study of dairy systems (O’Brien et al., 2014). It was applied with crop production data from national statistics to estimate the carbon footprint of Irish grain crops. The model was subsequently used to generate footprints for agricultural open days (Teagasc, 2019). The prototype model followed the general principles of LCA defined by the International Organisation for Standardisation (ISO 2006a, b). It adheres to the feed LCA guidelines of the Livestock Environmental Assessment and Performance Partnership of the Food and Agriculture Organization of the United Nations (FAO, 2016). The zero warming tillage model was updated based on the same guidelines and the GHG protocol (WRI/WBCSD, 2011). It was renamed the LCA grain model and structured according to the main phases in the ISO (2006a) framework being (1) goal and scope definition, (2) inventory analysis, (3) impact assessment and (4) interpretation.
Goal and scope
The primary aim of this study was to redevelop a single-impact LCA model focused on the carbon footprint of Irish grain crops. The scope of the model was restricted to the “cradle to farm-gate” perspective, where the system boundary extends from the extraction of raw materials through to the production and harvesting of crops on tillage farms. Thus, GHG sources and sinks associated with crop production up to the point grain leaves the farm were simulated, including pre-farm emissions embodied in inputs, for example manufactured fertilisers and fossil fuels. The temporal coverage of the model was an annual cropping cycle and the mode of LCA was descriptive (attributional) as it referred to past activities. The LCA grain model operated on a seasonal or annual time-step for continuous cropping system and for three to six crop rotation systems.
The most common grain crops grown in Ireland were assessed with the model, namely barley, oats, wheat, oilseed rape (OSR) and beans. Minor Irish crops were considered as well, that is peas, triticale, linseed and rye. Major grain crops were distinguished based on sowing date (i.e. spring or winter) and grade, for example feed, malt or food quality. Emissions associated with each crop were expressed in term of a functional unit, that is 1 tonne of grain to determine gross carbon footprint. Carbon sequestration through straw incorporation was scaled to grain yield as well and taken from gross emissions to compute net carbon footprint. The functional unit was product-based, as this type of indicator is considered appropriate for GHG emissions, given their global effects (de Boer, 2003). It was calculated by standardising the moisture content of grain crops to 15% for cereals and to 9% for OSR. In addition to producing grain, cropping systems harvest straw and other by-products. Allocating GHG emissions and removals among the outputs of multifunctional agricultural systems is challenging. To avoid complexity, the LCA grain model attributes GHG emissions and carbon sequestration entirely to the primary crop of a production system.
Inventory analysis
Greenhouse gas emissions, that is CO2, nitrous oxide (N2O) and methane (CH4), and carbon sequestration were computed in the second stage of the LCA model with activity data and emission factors. Activity data encompassed resources used and produced on farm and included tillage farming operations. Resource and operation data points were recorded with the data entry form. Where activity data were not available at a crop level for important resource inputs, that is manure nutrients, fuel and crop residues, gaps were filled with information from relevant literature sources.
Nutrient contents for manures and other organic materials were taken from the Teagasc book on major and micro nutrient advice (Wall & Plunkett, 2020). Fuel use was estimated with secondary information from Teagasc (2011), Williams et al. (2006) and AILE (2013). These reports gave the diesel consumption rates for machinery operations listed in the (Appendix Table A1). The amount of diesel consumed by an operation was calculated from the number of field passes, work rate or yield. Soil texture was also considered for cultivation operations using the approach of Williams et al. (2006). Briefly, fuel consumed by cultivation practices done on light and heavy soils was adjusted relative to a medium soil with the modification factors in Appendix Table A2. The volume of diesel used for cultivation was added to fuel for other operations to determine the annual fuel usage per crop.
Crop residues were derived using default data for above and below ground residue to yield ratios. These data were obtained from the national GHG inventory and varied by crop type (Duffy et al., 2022). The N content of residues was obtained from an Irish feed calculator (Teagasc, 2022) and from Duffy et al. (2022). Emissions from crop residues and other on-farm sources of GHG were determined with the factors and equations shown in Appendix Table A3. Nitrous oxide losses from crop residues were estimated as 1% of residue N in the prototype model. This default factor, known as a tier 1 emission factor, was reduced to 0.6% of crop residue N based on the current Intergovernmental Panel on Climate Change (IPCC, 2019) guidelines for national GHG inventories. The same update was made to the direct N2O emission factor for organic manure application. Direct N2O loss from this input was based on available N in manure, which was computed by taking housing and storage-related emissions from the total pool of N in manure management systems.
Country-specific, tier 2 emission factors reported by Roche et al. (2016) for arable soils were employed instead of the global default emission factor to compute direct N2O losses from fertiliser N application. The Irish tier 2 emissions factors disaggregated direct N2O loss by fertiliser N type. For CAN and nitrate-based compounds, the tier 2 N2O emission factor was 0.35% of N applied, which was 65% below the tier 1 emission factor. The tier 2 emission factor for direct N2O loss for urea application was 73% lower than the tier 1 factor and 80% lower for protected urea i.e. urea applied with an approved urease inhibitor.
Indirect N2O emissions associated with N inputs, fertiliser, residues and manure were estimated with tier 1 emission factors for two pathways: (1) after re-deposition of atmospheric NH3 and NOx on soils and water and (2) following leaching and run-off of N. For pathway 1, atmospheric deposition, the same N2O emission factor was applied as the prototype model, that is 1% of N volatilised on farm (IPCC, 2019). For pathway 2, 1.1% of N leaching and run-off (IPCC, 2019), instead of 0.75% in the prototype model, was estimated to be indirectly lost as N2O. The rate of N leaching and run-off from crop residues, and from fertiliser and manure application was computed as 10% of available N based on national research by Ryan et al. (2006) and Richards et al. (2009).
Nitrogen volatilised from the spreading of organic manures was quantified with the volatilisation factors in the national informative inventory report (Hyde et al., 2022). Ammonia volatilisation factors for the application of manure were expressed in terms of available total ammoniacal N (TAN) and were dependent on the season (Appendix Table A3). The method of application was also taken into account for NH3 losses from liquid manure spreading by utilising the mitigation factors reported by Buckley et al. (2020) for trailing shoe and band spreaders. Ammonia losses from the application of CAN, urea, protected urea and other N fertilisers were quantified with the volatilisation factors reported in the Irish informative inventory (Hyde et al., 2022).
Carbon dioxide emissions from urea and protected urea application and from lime spreading were calculated with tier 1 emission factors in the national GHG inventory report (Duffy et al., 2022). The GHG inventory also provided tier 1 and 2 emission factors to determine CO2 from the consumption of fossil fuel. Emissions of CH4 and N2O from fuel combustion were estimated with tier 1 factors (IPCC, 2006). Carbon sequestration was added to the LCA model for the incorporation of cereal straw into soil. This farming practice was assumed to increase C sequestration over a 20-yr period. The rate of C sequestration for straw incorporation varied depending on straw yield and soil type. It was quantified according to the approach of Lanigan et al. (2023) using the following equation:
The C content of straw dry matter (DM) was assumed to be 44% based on Teagasc (2016a). Three soil types, sandy, loamy and clay soils, were represented with the coefficient in equation 1. The coefficients were 0.0069 for sandy soil, 0.0111 for loamy soil and 0.0165 for clay soil. Straw yield was estimated from grain yield using a standard harvest index (proportion of crop biomass harvested as grain). Barley, wheat, spring oats and winter oats (WO) were estimated to have an harvest index of 54%, 53%, 48% and 44%, respectively (Teagasc, 2016a, b, c). For oats and wheat, 50% of the remaining material (stem, chaff, leaves and shoots) was harvestable as straw. For barley crops, the share of remaining material harvested as straw was about 60% (Teagasc, 2016b).
Pre-farm sources of GHG emissions were quantified with emission factors in Appendix Table A4. Irish literature was used, where possible, to calculate GHG emissions embodied in inputs, for example electricity generation (SEAI, 2022). Otherwise, pre-farm emissions were calculated with information from relevant international reports or LCA databases, for example Ecoinvent (2015). For fertiliser production, embodied GHG emissions were computed with European reference data that came from surveys of all of the members of fertilisers Europe (Brentrup et al., 2016). The GHG emissions from fossil fuel production were quantified with UK emission factors for the well-to-tank stage (DESNZ, 2023). Pre-farm GHG emissions related to the production of crop protectants and limestone were calculated with data from the footprint expert tool of the Carbon Trust (2012). Emissions embodied in seed and other inputs, for example plastic, were computed with data from Ecoinvent (2015).
Impact assessment
Emissions were classified into environmental impacts in the initial step of impact assessment. After classification, GHG emissions were translated to GWP100, which measures the global warming potential (GWP) of a GHG over 100 yr on a mass basis relative to CO2. This GWP metric is widely used to report GHG in terms of CO2 and was computed with characterisation factors from the IPCC (2021). The characterisation factors taken from this report to convert GHG emissions to CO2 equivalents were 1 for CO2, 27.9 for biogenic CH4 and 273 for N2O.
Statistical and sensitivity analysis
The influence of the major crop types (barley, wheat, oats, OSR and beans) on carbon footprint was evaluated with the one-way analysis of variance technique, following checking for normality with the statistical analysis system (SAS, 2014). Normality was tested with the Shapiro–Wilk test at the 5% significance level and by visually inspecting residual plots. Homogeneity of variance across major crops was assessed with Levene’s test (P < 0.05). Variation in gross and net carbon footprints was analysed separately with the general linear model (GLM) procedure in SAS. Pairwise comparisons were done following the GLM procedure with the Tukey test (P < 0.05). Within crop type, paired t-tests were used to compare gross and net carbon footprints.
Sensitivity of grain carbon footprints to model input parameters and relationships was analysed by replacing emission factors in the LCA grain model with data from the prototype model and by changing yields and functional units. Specifically, country-specific tier 2 emission factors for fertiliser application were changed to default tier 1 factors. For fertiliser production, emission data for European plants were replaced with values for another global region. Asia was selected as the alternative global region as it was reported to have the largest emission factors for fertiliser manufacture in an international analysis (Brentrup et al., 2016). On an N basis, this change increased the emission factors for fertiliser N to 10.6 kg CO2e for CAN and other N compounds, and to 5.5 kg CO2e for urea and protected urea. The emission factor for P fertiliser was increased to 1.2 kg CO2e/kg P.
The influence of yield on the carbon footprint of grain was tested by altering annual output by 50%. In addition, the functional unit for grain carbon footprints was changed from a mass to an energy basis. This alteration to the functional unit was calculated by converting yields to megajoules (MJ) of gross energy based on the energy density of a crop product. Gross energy was defined as the total amount of energy stored in a crop product.
Results
Key agricultural characteristics for Tirlán grain growers are illustrated on a grower and crop basis in Tables 1 and 2, respectively. On average, Tirlán growers had 240 ha of land that primarily comprised medium soils (mean 58%) followed by heavy soils (mean 29%). The majority of crops were established with a full plough-based system (mean 69.6%). On the bulk of the remaining area, minimum tillage was practised (30.3%). The number of crops grown on individual arable farms ranged from three to six (mean 4.5). About 90% of crops were grown in rotation cycles of three or more years. Across grains crops, fertiliser N application and diesel consumption averaged 179 kg N/ha and 95 L/ha, respectively. The quantity of grain produced by Tirlán growers averaged 2,195 t/annum and varied from 544 to 5337 t/annum.
Descriptive statistics of general characteristics for a random sample of large-scale Tirlán grain growers (n = 48) for the 2021/22 growing season
Mean | s.d.1 | s.e.2 | Inter-decile range | |
---|---|---|---|---|
Grain crops, no. | 4.5 | 1.01 | 0.1 | 3–6 |
Growing area, ha | 240 | 198 | 29 | 67–607 |
Soil type %3 | ||||
Light soil | 13 | 30.3 | 4.4 | 0–85.3 |
Medium soil | 58 | 40.7 | 5.9 | 0–100 |
Heavy soil | 29 | 37.2 | 5.4 | 0–100 |
Optimum4 soil P, % | 81 | 34.9 | 5.0 | 0–99.5 |
Optimum soil K, % | 65 | 44.7 | 6.5 | 0–98.9 |
Optimum soil pH, % | 64 | 39.2 | 5.7 | 0–99.0 |
Cultivation system, %3 | ||||
Plough based | 69.6 | 38.8 | 5.6 | 0–100 |
Minimum tillage | 30.3 | 38.9 | 5.6 | 0–100 |
Direct drill | 0.1 | 1.0 | 0.2 | 0–7 |
Fertiliser N, kg/ha | 179 | 24.1 | 3.5 | 134–208 |
CAN, % N | 65.7 | 27.1 | 3.9 | 0–88.8 |
Urea, % N | 0.3 | 2.1 | 0.3 | 0–0 |
Protected urea, % N | 6.3 | 16.3 | 2.4 | 0–30.5 |
Compound, % N | 27.7 | 23.0 | 3.3 | 10.7–87.6 |
Fertiliser P, kg/ha | 24 | 10 | 1.4 | 14–39 |
Fertiliser K, kg/ha | 99 | 29 | 4.2 | 49–148 |
Diesel, l/ha | 95.0 | 19 | 2.8 | 70–114 |
Grain output, t/grower | 2195 | 1800 | 260 | 544–5337 |
Oats | 182 | 206 | 30 | 0–471 |
Barley | 1003 | 724 | 105 | 196–2377 |
Wheat | 791 | 1112 | 161 | 0–2563 |
Oilseeds | 142 | 236 | 34 | 0–487 |
Beans and peas | 42 | 80 | 12 | 0–135 |
Cover crop, %3 | 14 | 25 | 3.6 | 0–69 |
Straw incorporation, %3 | 27 | 17 | 2.4 | 2–56 |
1Standard deviation.
2Standard error.
3Percentage of growing area.
4Soil P and K indexes above 2 were considered the optimum range. Optimum pH range for crops is 6.5–7.0.
Main inputs and outputs for a random sample of large-scale Tirlán grain growers (n 1 = 48) by crop type for the 2021/2022 growing season (means with standard error in parentheses)
Winter | Spring | |||||||||||
---|---|---|---|---|---|---|---|---|---|---|---|---|
Oats (n = 28) | Wheat (n = 38) | Barley (n = 47) | Oilseed rape (n = 29) | Beans (n = 3) | Rye (n = 7) | Oats (n = 8) | Malting barley (n = 24) | Feed barley (n = 9) | Beans (n = 12) | Oilseed rape (n = 3) | Wheat (n = 2) | |
Area, ha/grower | 29 (5) | 90 (18) | 72 (8) | 45 (9) | 17 (1) | 23 (5) | 21 (6) | 58 (11) | 46 (15) | 23 (5) | 21 (1) | 21 (7) |
Seeds, kg/ha | 160 (3) | 171 (3) | 169 (5) | 3 (0) | 205 (13) | 80 (14) | 161 (9) | 183 (2) | 182 (5) | 219 (7) | 4 (1) | 170 (4) |
Fertiliser N, kg/ha | 142 (3) | 216 (3) | 193 (2) | 179 (5) | 0 (0) | 180 (5) | 85 (8) | 152 (4) | 125 (11) | 2 (3) | 150 (13) | 126 (30) |
CAN, % N | 64 (7) | 72 (5) | 66 (4) | 56 (6) | 0 (0) | 71 (15) | 21 (12) | 68 (4) | 36 (12) | 0 (0) | 53 (25) | 0 (0) |
Urea, % N | 0 (0) | 0 (0) | 0 (0) | 3 (3) | 0 (0) | 0 (0) | 0 (0) | 0 (0) | 0 (0) | 0 (0) | 0 (0) | 0 (0) |
Protected urea, % N | 4 (3) | 5 (3) | 7 (3) | 2 (3) | 0 (0) | 16 (11) | 0 (0) | 1 (1) | 41 (14) | 0 (0) | 0 (0) | 93 (6) |
Compound, % N | 32 (6) | 23 (4) | 27 (4) | 39 (6) | 0 (0) | 12 (5) | 79 (12) | 32 (4) | 22 (5) | 100 (8) | 47 (25) | 7 (6) |
Fertiliser P, kg/ha | 23 (2) | 23 (2) | 26 (2) | 17 (3) | 26 (2) | 28 (4) | 27 (7) | 25 (2) | 19 (4) | 27 (3) | 29 (5) | 10 (7) |
Fertiliser K, kg/ha | 107 (7) | 104 (5) | 110 (5) | 79 (8) | 96 (10) | 116 (18) | 90 (4) | 91 (7) | 57 (11) | 89 (7) | 75 (16) | 99 (5) |
Diesel, l/ha | 96 (5) | 101 (4) | 94 (3) | 89 (4) | 57 (6) | 82 (12) | 74 (6) | 95 (5) | 96 (8) | 87 (8) | 84 (13) | 80 (6) |
Yield, t/ha | 9.3 (0.2) | 10.9 (0.2) | 9.8 (0.1) | 5.1 (0.1) | 5.7 (1) | 10.8 (0.4) | 7.5 (0.4) | 8.4 (0.2) | 8.2 (0.3) | 5.7 (0.3) | 3.2 (0.8) | 7.4 (0) |
Moisture content, % | 15.7 (0.4) | 16.3 (0.3) | 16.5 (0.3) | 9.6 (0.2) | 16.5 (1.7) | 16.8 (1.3) | 16.5 (0.8) | 15.3 (0.4) | 17.1 (1) | 17.3 (0.5) | 11.2 (2.1) | 20 (0) |
Standard yield, t/ha | 9.2 (0.2) | 10.7 (0.2) | 9.6 (0.1) | 5.0 (0.1) | 5.6 (0.9) | 10.5 (0.3) | 7.3 (0.3) | 8.4 (0.2) | 8.0 (0.3) | 5.6 (0.3) | 3.1 (0.8) | 7.0 (0) |
Cover crop, %2 | 0 (0) | 0 (0) | 0 (0) | 4 (3) | 0 (0) | 0 (0) | 24 (13) | 80 (10) | 53 (15) | 71 (15) | 0 (0) | 0 (0) |
Straw incorporated, %2 | 83 (8) | 2 (5) | 11 (4) | 86 (5) | 100 (0) | 11 (14) | 100 (0) | 9 (4) | 52 (15) | 91 (8) | 63 (33) | 0 (0) |
1 n is number of growers.
2Percentage of growing area.
In total, the sample of growers made up about 3% of the national tillage area (11,537 ha) in 2022, had 14 different crop types and produced 103.5 kt of grain. On a weight basis, 98% of grain output consisted of eight crop types, namely winter wheat (WW, 35.5%), winter barley (WB, 31.2%), spring malting barley (SMB, 11.4%), WO (7.1%), winter OSR (WOSR, 6.3%), spring feed barley (SFB, 3.2%), spring beans (1.5%) and spring oats (SO, 1.2%). Mean yields for winter crops of wheat, barley, oats and OSR were 10.9, 9.8, 9.3 and 5.1 t/ha, respectively (Table 2). Spring barley, SO and spring beans had mean yields of 8.4, 7.5 and 5.7 t/ha, respectively. On average, crop yields for spring barley and WW were greater than national average yields in 2022 by 4%, and 13%, respectively. Conversely, for SO, the mean yield was 5% below the national average. Winter wheat, WO and OSR had similar mean yields as the Irish average (Table 3).
Comparison of fertiliser N advice and average crop yields for Tirlán grain growers in 2022 with national average yields and N use statistics. Fertiliser N advice is for crops grown on soils in the optimum nutrient and pH range
The mean rate of fertiliser N application for WW, WB, WO and WOSR was 216, 193, 142 and 179 kg N/ha, respectively (Table 2). The majority (94%) of fertiliser N was spread in the form of CAN and compound N for winter and spring crops. Fertiliser N application for spring cereals varied from a mean of 85 kg/ha for SO to a mean 152 kg N/ha for SMB. The average level of fertiliser N applied to cereal and OSR crops exceeded the amount required for mineral soils in the optimum nutrient and pH range (Table 3). The mean N fertiliser rate for these crops was also above the most recent national average estimates. Spring beans received very little or no fertiliser N (mean 2 kg N/ha) as recommended and had the lowest mean diesel consumption (87 L/ha). For spring cereals, winter cereals and OSR, mean diesel usage was 93, 98, and 89 L/ha, respectively.
Cover cropping rates averaged 24%, 53%, 80% and 71% for SO, SFB, SMB and spring beans, respectively (Table 2). Cover crops were generally not planted prior to winter crops. Straw incorporation was conducted on all the area under SO and winter beans. For WO, WOSR, spring beans and spring OSR, straw incorporation rates averaged 83%, 86%, 91% and 63%, respectively. The mean rate of incorporation for barley and wheat straw was below 12%, apart from SFB straw which averaged 52%.
Gross carbon footprint
The gross carbon footprints of the principal crop types by weight for 48 commercial Tirlán grain growers in 2022 are presented in boxplots in Figure 1. In each boxplot, the middle coloured parts correspond to footprints that lie between the first and third quartile of the sample. The tails of the plots represent the minimum and maximum values with outliers excluded. Outliers are shown as dots above or below the tails. For oat crops, gross carbon footprint averaged 207 (s.e. 4) kg CO2e/t regardless of sowing season and ranged from 158 to 264 kg CO2e/t. Across barley crops, gross carbon footprint averaged 229 (s.e. 4) kg CO2/t for WB, 236 (s.e. 5) kg CO2/t for SMB and 238 (s.e. 8) kg CO2e/t for SFB. The range in gross carbon footprints for WB, SMB and SFB was 118, 81 and 67 kg CO2e/t, respectively. Mean values for gross carbon footprints of WW and spring beans were 217 (s.e. 4) and 214 (s.e. 11) kg CO2e/t, respectively. Gross carbon footprints for WW ranged from 167 to 269 kg CO2e/t (mean 217 kg CO2e/t) and from 149 to 273 kg CO2e/t for spring beans (mean 214 kg CO2e/t). For WOSR, gross carbon footprint averaged 384 (s.e. 16) kg CO2e/t and ranged from 307 to 680 kg CO2e/t.
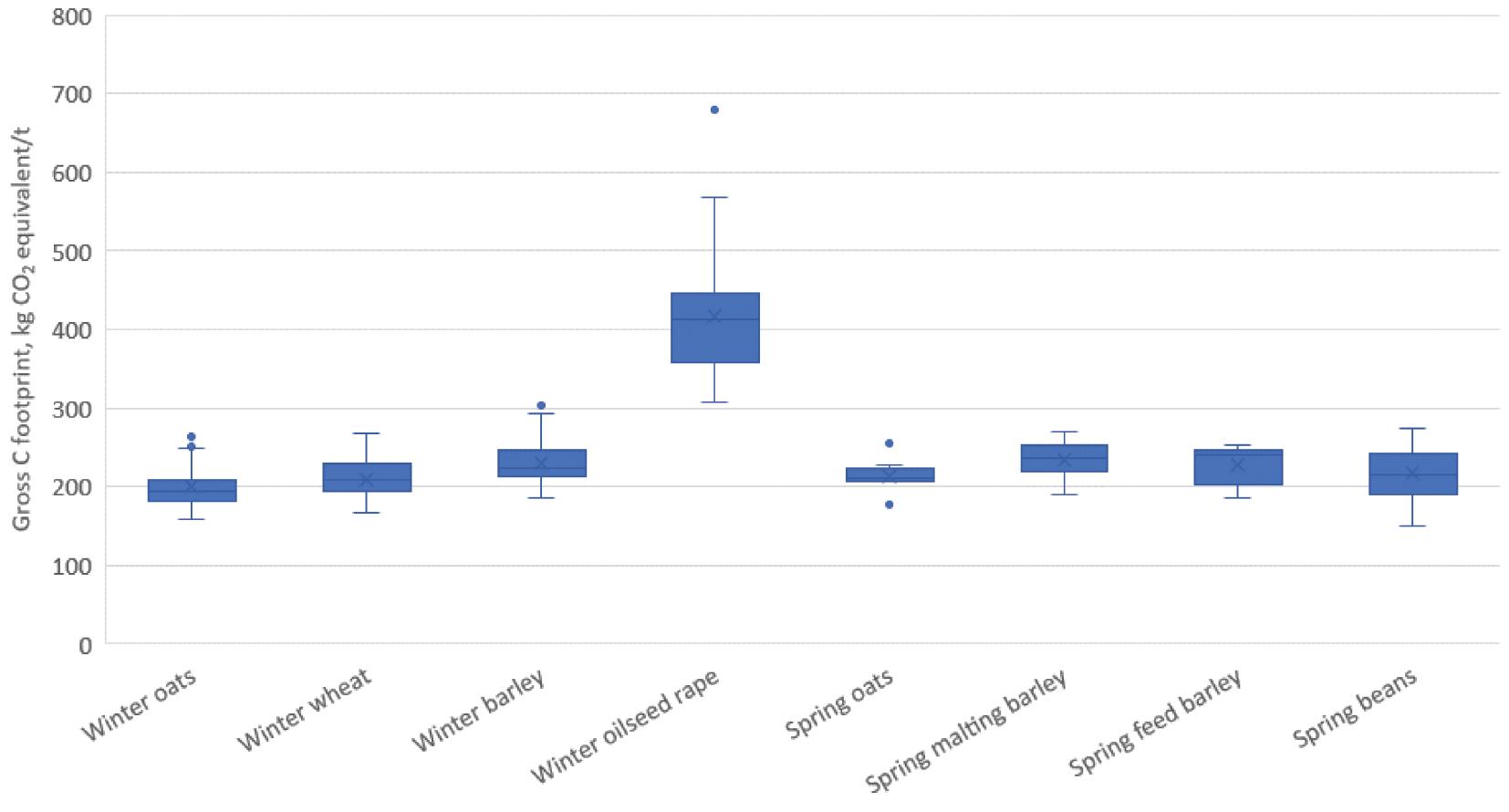
Box plots for gross carbon footprints of Tirlán grains in kg CO2 equivalent per tonne. Gross carbon footprints were for a random sample of 48 large-scale Tirlán grain growers for the 2021/2022 growing season. Note: Moisture content was adjusted to 15% for cereals and beans and to 9% for winter oilseed rape.
There were no statistically significant differences between the gross carbon footprints of barley, oats, wheat and beans. Sowing season had no effect on the gross carbon footprint of barley and oat crops. OSR had a greater gross carbon footprint than beans and the major cereal crops (P < 0.05). Excluding spring OSR, minor crop types had gross carbon footprints within the range of the main cereal and bean crops by weight. The gross carbon footprint of minor grain crops averaged 188, 192, 245 and 524 kg CO2e/t for rye, winter beans, spring wheat and spring OSR, respectively.
The main GHG emissions associated with the production of grain crops were CO2 and N2O (Figure 2). Nitrous oxide was the largest GHG emission for all crops, accounting for 63–80% of gross carbon footprint. Carbon dioxide was responsible for most of the rest of the gross carbon footprint for crops (18–37%). Methane’s contribution to GHG emissions from grain production was negligible (1–2%; Figure 2). Across cereal crops, fertiliser and lime manufacture were the principal sources of GHG emissions (23–40%). Diesel fuel and fertiliser N application were generally the next most important sources of GHG, together accounting for 27–37% of the gross carbon footprint for cereal crops (Figure 3). These sources and fertiliser production also made up the bulk of the gross carbon footprint for WOSR and spring OSR (75% and 78%, respectively).
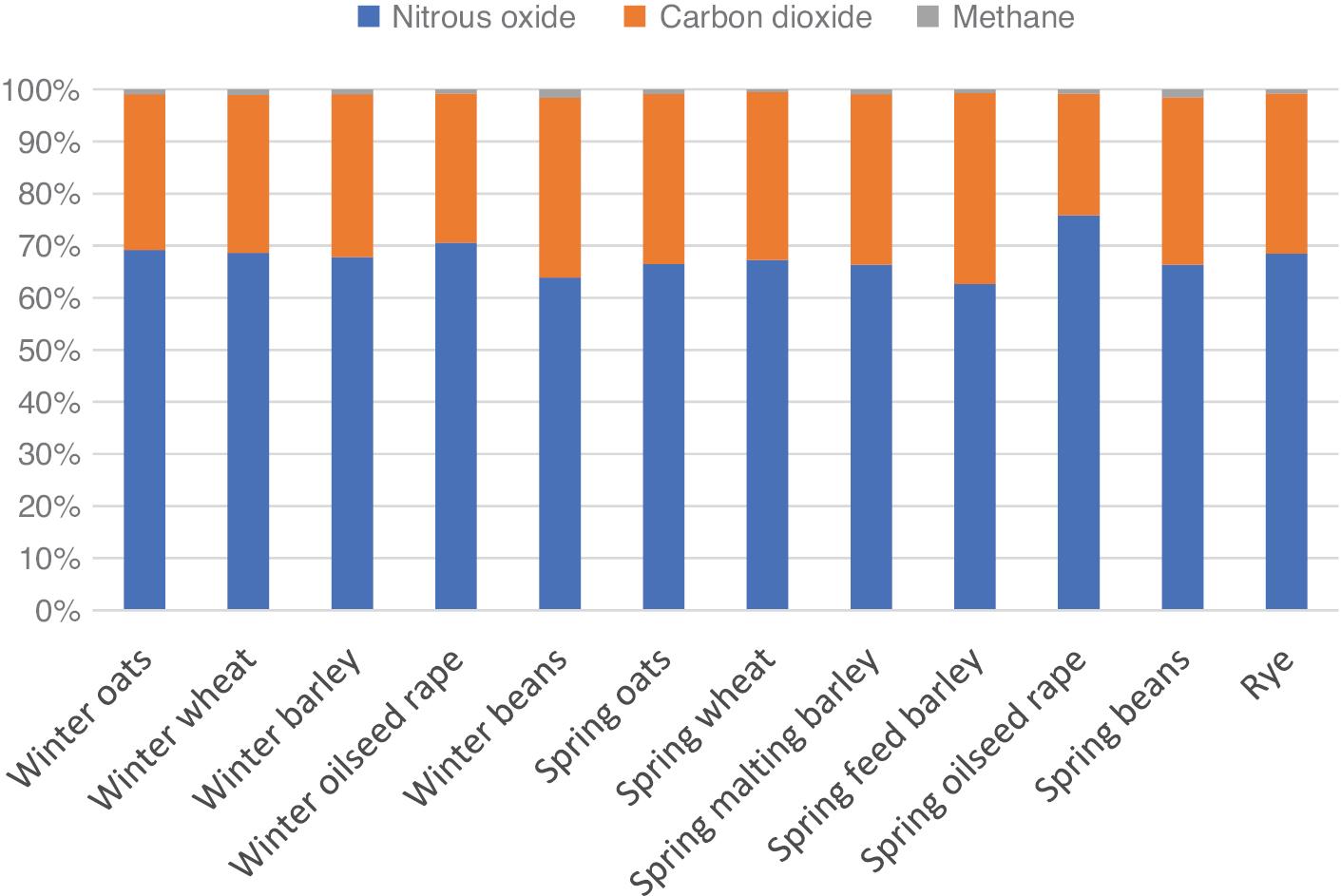
Relative contribution of greenhouse gases, nitrous oxide, carbon dioxide (CO2) and methane (CH4) to the gross carbon footprint of crops produced by a random sample of Tirlán grain growers (n = 48) in 2022.
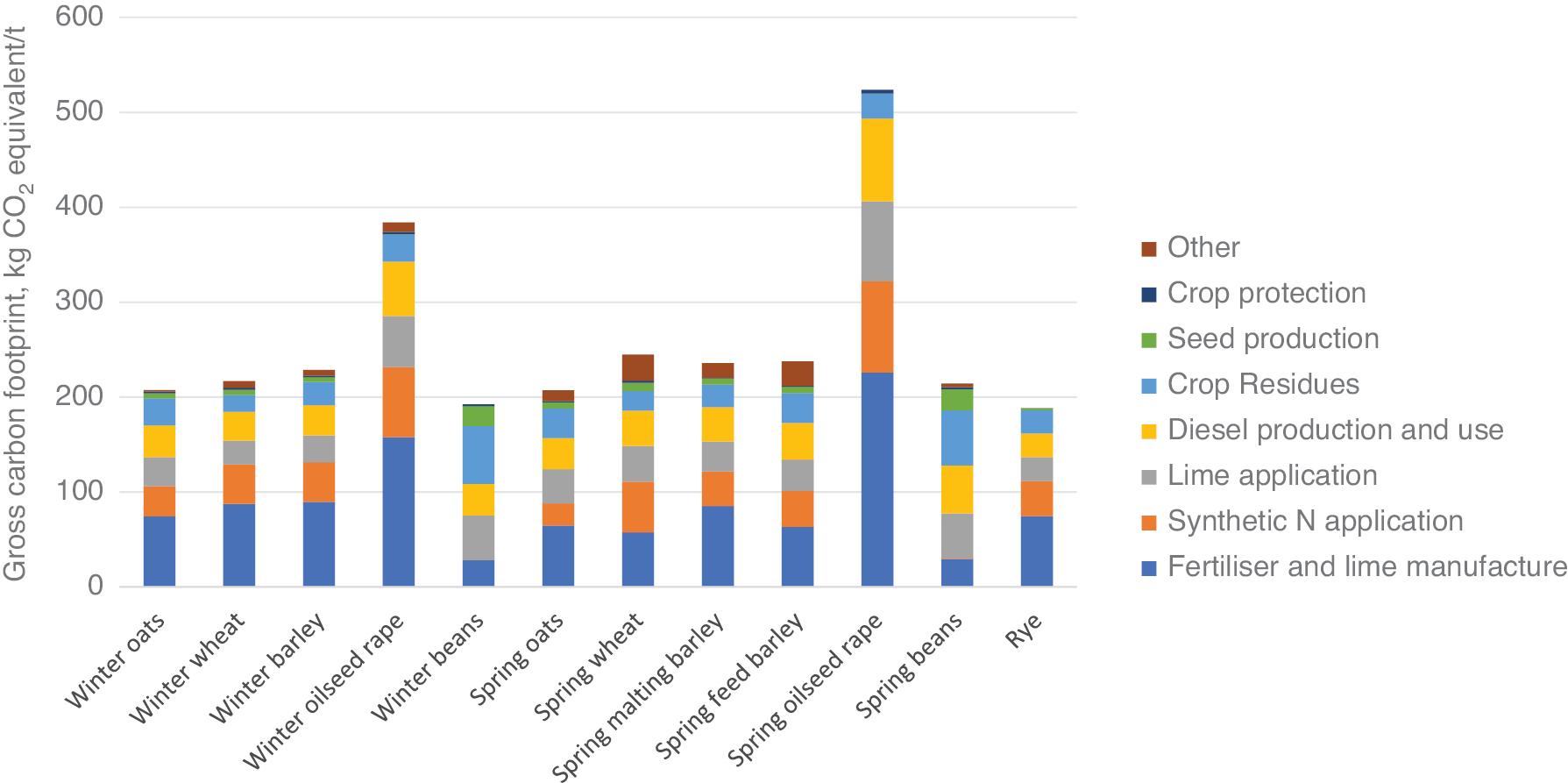
Breakdown of gross carbon footprint of crops by source (in kg CO2 equivalent per tonne) for a random sample of 48 large-scale Tirlán grain growers in 2022. Note: Moisture content was adjusted to 15% for cereals and beans and to 9% for winter and spring oilseed rape.
Regarding spring and winter beans, crop residues were the largest source of GHG emissions (27–32%), followed by diesel production and use (17–24%) or lime application (22–25%). Unlike cereals and oilseeds, fertiliser manufacture and application were minor components of the gross carbon footprint of beans. The remaining GHG emissions related to bean production mostly came from lime and seed production (23–24%). Organic manure also contributed to GHG emissions from bean and cereal production, particularly spring barley crops (7–11% of gross carbon footprint). The rest of GHG emissions from cereal and OSR cropping systems were primarily generated by crop residues, seed production and manufacturing of crop protectants.
Net carbon footprint
Incorporation of straw into soil resulted in both WO and SO having an average carbon sequestration rate of −170 (s.e. 16) kg CO2e/t per year (Tables 4 and 5). For WOSR, straw incorporation led to an average carbon sequestration rate of −76 (s.e. 5) kg CO2e/t per year. Annual carbon sequestration ranged from 0 to −116 kg CO2e/t for WOSR and from 0 to −283 for kg CO2e/t for oat crops. The average annual rate of carbon sequestration for WW, WB, SMB and SFB was −4 (s.e. 9), −19 (s.e. 6), −14 (s.e. 7) and −67 (s.e. 22) kg CO2e/t, respectively. Carbon sequestration was low for WW, WB and SMB due to high levels of straw removal. For minor crop types, average carbon sequestration rates were zero or close to zero apart from spring OSR (−71 kg CO2e/t per year).
Breakdown of average values for net carbon footprint of Tirlán grain crops by source in kg CO2e/t. Net carbon footprints were estimated for 48 large Tirlán grain growers for the 2021/2022 growing season. Note: Moisture content was corrected to 15% for cereals and beans and to 9% for winter and spring oilseed rape
Winter | Spring | |||||||||||
---|---|---|---|---|---|---|---|---|---|---|---|---|
Oats (n = 28) | Wheat (n = 38) | Barley (n = 47) | Oilseed rape (n = 29) | Beans (n = 3) | Rye (n = 7) | Oats (n = 8) | Malting barley (n = 24) | Feed barley (n = 9) | Beans (n = 12) | Oilseed rape (n = 3) | Wheat (n = 2) | |
Fertiliser manufacture | 74 | 88 | 90 | 158 | 28 | 74 | 65 | 85 | 63 | 29 | 226 | 58 |
Seed production | 5 | 5 | 5 | 0 | 21 | 2 | 7 | 6 | 7 | 23 | 0 | 9 |
Pesticides | 2 | 2 | 2 | 2 | 2 | 0 | 1 | 1 | 1 | 2 | 4 | 2 |
Fuel production | 6 | 6 | 6 | 11 | 6 | 5 | 6 | 7 | 7 | 10 | 16 | 7 |
Crop residues | 28 | 17 | 25 | 29 | 61 | 24 | 31 | 24 | 31 | 58 | 26 | 21 |
Fertiliser N application | 32 | 41 | 42 | 74 | 0 | 37 | 23 | 36 | 38 | 1 | 96 | 53 |
Organic N application | 1 | 7 | 6 | 10 | 0 | 0 | 11 | 15 | 26 | 4 | 0 | 28 |
Lime application | 30 | 25 | 28 | 54 | 47 | 25 | 36 | 31 | 33 | 47 | 84 | 38 |
Fuel use | 27 | 25 | 26 | 47 | 27 | 20 | 27 | 30 | 31 | 41 | 71 | 30 |
Straw incorporation | −170 | −4 | −19 | −76 | 0 | 0 | −170 | −14 | −67 | 0 | −71 | 0 |
Net C footprint | 38 | 213 | 209 | 309 | 192 | 188 | 37 | 222 | 170 | 214 | 453 | 245 |
Mean rate of carbon sequestration through straw incorporation (SI) for Tirlán grain by crop type (standard error in parentheses). The rate of sequestration for 48 large Tirlán grain growers was determined per tonne and per hectare for the 2021/22 growing season. Note: Moisture content was corrected to 15% for cereals and beans and to 9% for winter and spring oilseed rape
Season | Crop | Straw incorporation | C Sequestration | |||
---|---|---|---|---|---|---|
n 1 | % area | kg CO2/t | kg CO2/ha | |||
Winter | Wheat | 4 | 2 | −4 (9) | −38 (90) | |
Oats | 21 | 83 | −170 (19) | −1558 (181) | ||
Barley | 3 | 11 | −19 (6) | −185 (57) | ||
Oilseed rape | 27 | 86 | −76 (5) | −380 (26) | ||
Spring | Malting barley | 1 | 9 | −14 (7) | −120 (58) | |
Feed barley | 2 | 52 | −67 (22) | −541 (171) | ||
Oats | 8 | 100 | −170 (17) | −1248 (148) | ||
Oilseed rape | 2 | 63 | −71 (34) | −222 (157) |
1Number of growers.
Figure 4 shows that accounting for carbon sequestration in the GHG budget of grain production systems led to WO and SO having average net carbon footprints of 38 (s.e. 19) and 37 (s.e. 19) kg CO2e/t, respectively. Net carbon footprints varied from −85 to 251 kg CO2e/t for WO and from −21 to 115 kg CO2e/t for SO. Mean values for the net carbon footprint of WW, WB, SMB and SFB were 213 (s.e. 8), 209 (s.e. 7), 222 (s.e. 8) and 170 (s.e. 20) kg CO2e/t, respectively. For WW, net carbon footprint varied from 16 to 269 CO2e/t (mean 213 kg CO2e/t), and for WB, it ranged from 4 to 303 kg CO2e/t (mean 209 kg CO2e/t). The range in net carbon footprint for SMB and SFB was 204 and 183 kg CO2e/t, respectively. Net carbon footprint of WOSR averaged 309 (s.e. 15) kg CO2e/t and varied from 232 to 567 kg CO2e/t.
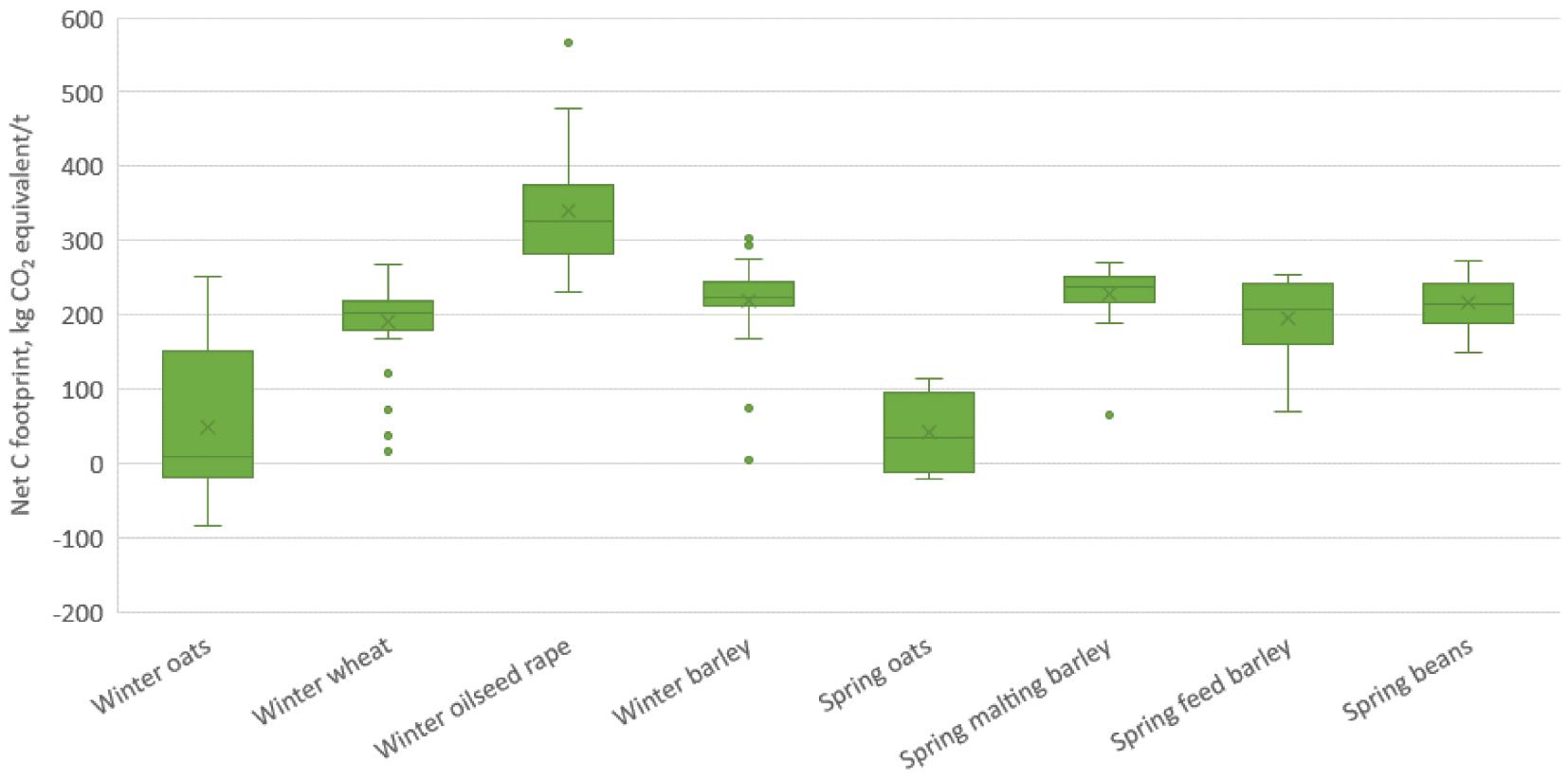
Box plots for the net carbon footprints of Tirlán grains in kg CO2 equivalent per tonne. Net carbon footprints were for a random sample of 48 large-scale Tirlán grain growers for the 2021/22 growing season. Note: Moisture content was adjusted to 15% for cereals and beans and to 9% for winter oilseed rape.
For minor crops, rye, winter beans, spring wheat and spring OSR, net carbon footprints averaged 188, 192, 245 and 453 kg CO2e/t, respectively. Net carbon footprint did not differ significantly from gross carbon footprints for wheat and bean crops. Net carbon footprint was significantly lower than gross carbon footprint for oats, barley and OSR (P < 0.05). OSR had a greater net carbon footprint than cereals and beans (P < 0.05). Net carbon footprint for oats was lower than the carbon footprints of wheat, barley and bean crops (P < 0.05).
Model sensitivity
Table 6 illustrates the influence of emission factors, yield and functional unit on the carbon footprint results of the LCA grain model. Regarding emission factors, applying the default tier 1 factor for fertiliser application in the model increased the baseline results for the gross carbon footprints of barley, wheat, oats and OSR by an average of 24%, 26%, 20% and 26%, respectively. Switching from Irish to tier 1 emission factors for fertiliser application caused a similar relative increase in the net carbon footprint of barley, wheat and OSR. It caused a large relative increase (112%) in the net carbon footprint of oats due to the low baseline (37 kg CO2e/t) and had no effect on the carbon footprint of beans. For fertiliser production, changing emission factors from European to Chinese plants had very little effect on the carbon footprint of beans (+2%). The average carbon footprints of cereal and OSR crops were more sensitive to emission factors for this source, increasing by 49–62% on a gross basis, and by 61–271% on a net basis. Oats had the largest percentage increase in net carbon footprint and this was again due to the low baseline.
Sensitivity of average gross and net carbon footprints of Tirlán grain crops to changes in functional unit, fertiliser emission factors and crop yield. Excluding functional unit, effect of parameter change calculated as percentage difference relative to baseline situation. Baseline corresponds to average carbon footprint of crops in kg CO2e/t with standard error in parentheses. Moisture content was corrected to 15% for cereals and beans and to 9% for oilseed rape (OSR)
Carbon footprint | Crop | Baseline | Functional unit, energy (kg CO2e/GJ) | Fertiliser application, tier 1 N2O EF1 | Fertiliser production, Asia EF | Crop yield, −50% |
---|---|---|---|---|---|---|
Gross | Oats | 207 (4.1) | 12.3 (0.2) | 20% | 49% | 100% |
Wheat | 217 (4.1) | 13.7 (0.3) | 26% | 62% | 100% | |
Barley | 231 (2.8) | 14.8 (0.2) | 24% | 56% | 100% | |
Beans | 211 (9.9) | 13.4 (0.6) | 0% | 2% | 100% | |
OSR | 388 (19.0) | 16.6 (0.7) | 26% | 62% | 100% | |
Net | Oats | 37 (15.6) | 2.8 (0.9) | 112% | 271% | 100% |
Wheat | 213 (8.3) | 12.6 (0.5) | 27% | 63% | 100% | |
Barley | 210 (5.3) | 14.1 (0.3) | 26% | 61% | 100% | |
Beans | 211 (9.9) | 13.4 (0.6) | 0% | 2% | 100% | |
OSR | 313 (19.1) | 13.7 (0.7) | 32% | 77% | 100% |
1Default tier 1 emission factor (EF) for nitrous oxide (N2O) loss from fertiliser application equated to 1% of N (IPCC, 2019).
Decreasing crop yields by 50% without changing input levels resulted in a twofold increase in the gross and net carbon footprints of cereal and bean crops. Coupling this change with increases in emission factors for fertiliser application and production increased the gross amount of GHG emitted from barley, wheat, oats, beans and OSR production by an average of 598, 600, 494, 220 and 1074 kg CO2e/t, respectively. Increasing emission factors for fertiliser application and production resulted in beans having a lower gross carbon footprint than cereal crops on a mass basis (P < 0.05). Switching the functional unit from mass to energy reduced the average relative difference between the gross carbon footprint of beans and OSR from 84% to 24%. Changing functional unit decreased the average difference between the gross carbon footprint of OSR and cereal crops by 52–56 percentage points. It also resulted in no differences between the net carbon footprints of OSR and grain crops, except oats. The energy unit showed, like the baseline, that oats had the lowest net carbon footprint.
Discussion
Many multinational food and beverage companies have pledged to become climate neutral by the middle of the 21st century. To achieve this ambitious target, scope 3 emissions related to the production of agricultural commodities need to be reduced to net zero. Mitigating GHG emissions and the carbon footprint of agricultural products is challenging, given that the majority of emissions are generated by complex biological processes (Flysjö et al., 2011). Nevertheless, reductions in agricultural carbon footprints are possible by implementing carbon farming practices that improve productive efficiency (Jones & Sands, 2013; Khatri-Chhetri et al., 2023). Further carbon footprint reductions can be achieved by using low-emission technologies and through enhancing carbon sequestration in biomass and soils (CHAP, 2022; Lanigan et al., 2023).
In this LCA study, the adoption of carbon farming practices increased carbon sequestration by incorporating more organic material (straw) into arable soils. This resulted in growers of oats having climate-neutral crops. Straw incorporation, however, was not practiced on the bulk of crops as it adds to the cost of producing grain (Lanigan et al., 2023). In Ireland, financial aid is provided for incorporation of cereal straw (DAFM, 2023), but the intention is to change the practice of straw removal for barley and wheat, which is required for bedding for animal welfare purposes. This implies that alternative approaches are required to produce climate-neutral grain. The planting of cover crops could contribute significant carbon sequestration, particularly before spring sown cereals, but, it was excluded from this analysis, as there is no established methodology to estimate the carbon content of these crops.
Irrespective of the carbon sequestration potential of crops, our analysis of Tirlán growers illustrated that beans and cereal grains had considerably lower carbon footprints on a mass basis than OSR. In agreement with prior agricultural LCA studies (e.g. Williams et al., 2006; Sørensen et al., 2014), this was mainly caused by beans and cereals using less fertiliser N or producing more grain for the same level of N input as WOSR. Excluding sequestration, the average carbon footprint of large Tirlán cereal growers on a tonnage basis was 161 kg CO2e below the average footprint of WOSR, which is far less than the 906–1022 kg CO2e difference estimated in Williams et al. (2006) and Sørensen et al. (2014). The divergence can be explained by the lower yields and N efficiency of WOSR in these studies and changes to the LCA method. For instance, the GWP of N2O decreased from 298 to 273 between 2006 and 2023, and the emission factor for CAN fertiliser manufacture in Western Europe has decreased by about 50% over the period. Together, these changes have narrowed the gap between the carbon footprint of OSR and other crops.
Similar to Xu et al. (2018) LCA of carbohydrate-rich foods, comparisons of the carbon footprints of grain crops were strongly influenced by the choice of functional unit. For instance, using an energy-based unit instead of a mass-based unit brought the gross carbon footprint of OSR much closer to cereals and beans. This change of functional unit also caused OSR to have a similar or lower net carbon footprint than the other crops assessed. Expressing carbon footprint on an energy basis was more favourable for OSR as it is high in fat (45%) and thus had a 48–57% greater energy density than cereals and legumes (Potter, 1986; FAO, 2001). An energy-based unit is of greater relevance than mass for crops such as oilseeds, as it better reflects their primary purpose. This implies that multiple functional units are required to appropriately analyse the carbon footprint of crop products with contrasting functions or in different contexts.
Besides functional unit, emission factors for fertiliser N application and manufacture had a substantial influence on the carbon footprints of grain crops. The gross carbon footprint of beans was about 25% less than cereals when default tier 1 emission factors were utilised for fertiliser N spread onto soil, which supports the findings of a few environmental assessments of legume cropping systems (Aguilera et al., 2015; Yue et al., 2017; CHAP, 2022). However, when country-specific emission factors were used for this source, the carbon footprints of beans and cereals were similar, except for the net carbon footprint of oats. The reason for the change in the ranking of crop carbon footprints was country-specific emission factors, which only decreased N2O emissions estimates for cereal and OSR production. They had little or no effect on N2O emissions from beans as this crop produced N through biological fixation, whereas cereals required more N from synthetic fertilisers. Biological N fixation does not generate GHG emissions (IPCC, 2019), which explains the relatively low carbon footprint of beans, despite yielding less than other crops.
Differences in chemical and biological N inputs for crops caused cereals to have a larger carbon footprint than beans as manufacturing of fertiliser moved from European plants to less efficient plants in Asia. Sourcing fertiliser from the latter region increased GHG emissions by 27–30% more than switching to default emission factors for fertiliser application. Thus, the gap between the carbon footprint of cereals and beans widened with the change of origin of fertiliser to Asia. Fertiliser N and organic N inputs were GHG hotspots, making up about 70% of the overall carbon footprint of grain production. Hillier et al. (2009) also made similar observations when quantifying the carbon footprint of crops grown by Scottish farmers. The use of N inputs, specifically fertiliser and manure, and their relationship with yield explained much of the variability within the gross carbon footprints of OSR and cereals in this LCA. This finding suggests that there is scope to decrease grain carbon footprint by optimising N use. Further increasing the N efficiency of large Tirlán growers will be challenging as scale had limited impact on crop yield or nutrient inputs and most had adopted good agronomic practices, for example nutrient management planning. Nevertheless, gains in N efficiency can potentially be achieved through greater uptake of cover crops and via targeted N application with precision agriculture technologies. The latter option is among the most promising, with recent studies reporting carbon footprint reductions nearing 20% (e.g. Medel-Jiménez et al., 2024).
Irish and international comparisons
Life cycle analyses of agricultural commodities are challenging to compare because of variation in local conditions, production systems and modelling methodologies (de Boer, 2003; Sieverding et al., 2020). Some of the main divergences in LCA modelling methods have been outlined, that is functional units and emission factors. Further examples of inconsistencies in modelling methodology include alternate system boundaries and environmental impact categories. Modelling differences complicate comparisons of agricultural LCAs, but can be overcome somewhat through careful benchmarking of results. Cautious LCA comparisons are useful for validating model outcomes where measurements are lacking and are helpful for identifying emission reduction and removal strategies. Hence, the results of the LCA grain model were validated against similar Irish and international studies, particularly the estimates of the Global Feed LCA Institute (GFLI, 2024).
Cross-validation of the LCA grain model with national literature was limited due to the small number of relevant Irish studies, that is Courtney (2013) and Wilson (2019). Courtney (2013) applied the Cranfield LCA model to quantify GHG emissions from arable farms located in the mid-east of the country within the Boyne catchment. The study estimated emissions associated with the production of cereals, beans and OSR between 2007 and 2009. Mean carbon footprints reported by Courtney (2013) ranged from 329 to 510 kg CO2e/t for cereals and varied from 501 kg CO2e/t for beans to 1,154 kg CO2e/t for OSR. Wilson (2019) reported similar carbon footprints for cereals and greater values for beans (610 kg CO2e/t) and OSR (2,090 kg CO2e/t). The comparison shows that on average the gross carbon footprints for large-scale Tirlán grain producers were below the range of reported outcomes. This was due to differences in productivity and LCA methodology. Large Tirlán growers were more productive than average Irish tillage farms due to economies of scale, which diluted fixed costs. Both were more efficient than cereal producers in past Irish LCA studies, outyielding them by an average of 1.9 to 3.0 t/ha, despite using similar or lower levels of inputs. In addition, large Tirlán growers had greater bean (+2.2 t/ha) and OSR (+1.6 t/ha) yields than earlier studies. Coupling these yields with refinements to emission factors for fertiliser application and manufacture resulted in low grain carbon footprints. Reverting to default IPCC emission factors increased carbon footprints to fit within the lower range of Irish estimates. Further sensitivity analysis with reduced yields showed that Tirlán grain growers had carbon footprints equivalent to or greater than Courtney (2013).
At international level, the average carbon footprints of large Tirlán grain growers were below the range of values reported for Irish crops within the public database of the GFLI (2024). The GFLI database is recognised as a global reference for feed LCA data by the private and public sectors (GFLI, 2023). It is a collection of over 1,800 LCA datasets for crops and feeds from various world regions and countries. The GFLI (2023) approach is aligned with FAO (2016) feed guidelines and the Feed Product Environmental Footprint Category Rules (PEFCR) of the European Commission (2020). The database covers the environmental impacts of crops from cradle to farm-gate, as well as from cradle to processing-gate for feed products. Average carbon footprints for large-scale Tirlán grain growers were evaluated against GFLI results for dried crops with a system boundary until the farm-gate.
Drying of Tirlán grain crops was accounted for by adding 25 kg CO2e to a tonne of product. This emission represented the diesel required to reach the target moisture content for safe storage of grain. The estimate was similar to GFLI values for Irish crops, which had greater carbon footprints than Tirlán grains for two reasons. First, the GFLI used default N2O emission factors for fertiliser application and higher emission factors for fertiliser manufacture. Second, the average Irish producer in the GFLI was not as productive as Tirlán growers in 2022. Controlling for these factors, the results of this study were in line with the GFLI outcomes. The GFLI database showed that the carbon footprint of Irish cereal and bean crops was near or at the bottom of the international range, which implies that the footprints of large-scale Tirlán grain growers are among the lowest in the world. The carbon footprint of Irish OSR was in the middle of the global range, but using country-specific emission data suggested it was actually in the lower range of estimates. This finding highlights the need to undertake more research on agricultural emissions at a national or regional level.
Additional comparisons with the international literature through the study of CHAP (2022) agreed that Tirlán grain growers performed better than most regions in terms of gross carbon footprint. CHAP (2022) referred to gross carbon footprint as emission intensity when establishing the range of estimates for UK crops in the scientific literature. The group related carbon footprints to representative European and global data. Typically, gross carbon footprints for the main UK crops, that is wheat, barley, OSR and oat, were between 310 and 470 kg CO2e/t, which was similar to or below major crop-producing regions, for example USA. The results of CHAP (2022) were greater than this research for the same reasons as outlined for the GFLI comparison, apart from beans. Tirlán growers had a larger gross carbon footprint for beans than the UK average (100 kg CO2e/t) in CHAP (2022), because liming was a much larger source of emissions. Nevertheless, the carbon footprints of Tirlán beans were substantially below international comparators such as soybeans from South America (CHAP, 2022). Inclusion of domestic bean ingredients in animal rations has potential to improve the climate performance of the livestock sector and remove reputational issues around feeding imports grown on deforested lands. Growing beans also serves to break disease cycles in crop rotations with cereals and decreases fertiliser N usage (Nemecek et al., 2015).
Research limitations
Contemporary agricultural LCA models such as CAP’2ER (IDELE, 2022) simultaneously determine multiple environmental impacts associated with crop products. The new Irish LCA grain model has this capacity as well, but it was only applied to determine the carbon footprints of grain crops. Restricting LCA to one impact category, that is carbon footprint or water footprint is common (Sieverding et al., 2020), but it is also important to consider the effects of crop production on additional environmental metrics, for example water quality and biodiversity. This broad form of LCA is important for evaluating the trade-offs related to changing farming practices. Thus, the LCA grain model needs to be expanded to evaluate overall environmental performance. Furthermore, it should have the ability to relate environmental emissions to socio-economic indicators, as farm decisions depend on financial returns and lifestyle choices. This can be achieved by coupling LCA models with representative socio-economic datasets as demonstrated by Thomassen et al. (2009) for Dutch farms. A major benefit of socio-economic information is it helps identify cost-effective options for complying with environmental regulations. The potential to use financial and social data with the LCA grain model will be investigated in the future.
Further limitations regarding the scope of this research included the restricted system boundary, which went from cradle to farm-gate, and the duration and level of the analysis. The system boundary of the farm-gate was chosen because on-farm drying is rare in Ireland and the GHG costs of drying would be included in the purchaser’s scope 1 or 2 emissions. Consistent with agricultural LCA studies, the model omitted GHG emissions related to the transport of grains to merchants or feed mills, nor did it estimate emissions from processing or other downstream stages. The carbon footprints of Tirlán grain crops therefore do not represent the full product footprint. However, they do provide a good indication of the total product carbon footprint given that the agricultural stage is where most emissions tend to arise (Dijkman et al., 2018; Sieverding et al., 2020).
Generally, agricultural LCAs are carried out at the single crop level (Costa et al., 2020). This level of analysis is required where an LCA concentrates on food products such as gluten-free oats, but tillage farmers attempt to optimise the output of cropping systems. To analyse tillage farms at this level, the full crop rotation should be included in an LCA (Nemecek et al., 2015). This can be done through (1) expanding the boundary of an LCA to take the entire crop rotation into account and (2) using area-based along with product-based functional units (Peter et al., 2017). Integrating crop rotation into LCA better accounts for the benefits of this practice like enhanced weed suppression and improved soil health and is an additional lever to improve carbon footprint (Costa et al., 2020).
Ideally, crop rotations and crops should be evaluated across multiple years. This LCA study was limited to a single production year, 2022, where favourable weather conditions contributed to above average yields on tillage farms (DAFM, 2023). This may have resulted in below average crop carbon footprints for Tirlán growers. Undertaking a long-term, for example 3–5 yr, environmental assessment is an effective way of controlling for variability related to weather (Buckley & Donnellan, 2023). Repeating this LCA for a greater number of years is therefore necessary to establish more accurate carbon footprints for Tirlán growers at the single crop level. Moreover, the LCA grain model should be applied to small, medium and large tillage farms in the national farm survey (NFS; Dillon et al., 2023) over multiple years. The NFS is conducted annually on a nationally representative sample of tillage farms. A wealth of data is collected from tillage farms through the NFS that could enable the sector to monitor the temporal change in the carbon footprints of grain crops and facilitate robust country-level comparisons.
As well as activity data, the carbon footprints of agricultural products depend on emission factors and modelling approaches, that is IPCC tier 1, 2 or 3 methods (Hillier et al., 2011; Tuomisto et al., 2015). The IPCC tier 1 methodology is the simplest approach; designed for compiling national GHG inventories. It allows nations to estimate emissions and removals where there is limited information. The method does this by combining minimal activity data with default emission factors (IPCC, 2006). The LCA grain model uses tier 1 emission factors for crop residues, organic manure and lime application. Tier 1 emission factors are very uncertain for biogenic sources (IPCC, 2006). The default emission factor for lime application is a good example in this respect with a fixed uncertainty of −50%. The input can even result in an uptake of CO2 depending on soil conditions (West & McBride, 2005). Hence, the tier 1 approach in the LCA grain model may have overestimated GHG emissions from lime and other sources.
Using tier 1 emission factors for Irish growers was unavoidable as country-specific tier 2 emission factors did not exist for key GHG sources and there was insufficient activity data to use process-based tier 3 models. The latter method is generally impractical on commercial farms (Hillier et al., 2011), but the former approach is possible as demonstrated for fertiliser N application. To make better use of tier 2 methodology in the model, more field measurement of GHG fluxes is required, especially for key sources and sinks. This research is costly to do, nonetheless, it should benefit growers in terms of more accurate carbon footprint estimates for agricultural products. Carbon footprint is an indicator of growing importance to the agricultural community that may in time command a premium from purchasers with strong ambition to decrease supply chain emissions.
Conclusions
This study showed through a redeveloped LCA model of Irish grain crops that the carbon footprints of large-scale Tirlán grain growers are low by international standards. The outputs of the new model for legumes were very similar to the prototype version but were substantially (41–47%) lower for cereal and OSR crops. This was due to applying new emission factors for fertiliser manufacture, representative of current technology, as well as moving to a higher tier IPCC methodology for fertiliser N application. The latter reason highlights the possible benefits of investing in the development of country-specific emission factors. More country-specific emission factors are clearly needed for the LCA grain model to reduce reliance on tier 1 methods, as these methods potentially lead to LCAs overestimating crop carbon footprints. The main determinants of gross carbon footprint across all grain crops were yield and N inputs, particularly fertiliser N for cereals and OSR. Tirlán growers performed well in terms of crop yields and managed fertiliser N effectively. However, they can further improve N efficiency through greater use of cover crops, legumes and precision farming technologies. Combining cover cropping with greater levels of straw incorporation has the potential to increase carbon sequestration in arable soils. This can bring carbon footprint close to or below net zero for grain crops as evidenced for oat crops. Producing grain crops with net-zero carbon footprints is likely to add value to agricultural products once recognised monitoring, reporting and verification processes have been established for the farming sector. The next steps for this research are to incorporate the LCA grain model into AgNav, (Herron et al., 2023) a digital sustainability platform, and to develop decision support features to help growers contribute to national and global efforts to mitigate climate change.