Background
One of the most important features of the immune system is the complex composition involving many different types of organs, tissues, cells, and molecules that carry out immune functions. Autoimmune diseases refer to immunopathologic states in which the immune system reacts to self-organs, tissues, cells, and molecules when regulation on immune tolerance regulation is out of balance or destroyed, thus resulting in damage to self-organs [1, 2]. Autoimmune diseases have complex, varied pathogeneses [3]. Studies in recent years have shown that immune cells and their interactions have an important role in the initiation and development of diseases [4–7]; however, due to the heterogeneity of immune cells, the detailed molecular mechanisms by which these cells induce autoimmune diseases have not been established.
To date, greater than 80 types of autoimmune diseases have been identified; however, the precise causes and pathogenesis of these autoimmune diseases remain largely unknown. A large number of studies have shown that immune cells display strong heterogeneity in autoimmune diseases, yet increasing evidence also suggests that immune cells in these diseases share similarities. For example, recent studies have suggested that the monocyte phenotype is responsible for the pathogenesis of specific autoimmune diseases. Several monocyte susceptibility genes, including HLA, PTPN22, IRF5, IL-1β, IFN-λ, C-X-C motif chemokine 10 (CXCL10), and C-C motif chemokine ligand, are considered to be involved in multiple sclerosis (MS), Sjogren’s syndrome (SS), and systemic lupus erythematosus (SLE) [8–10]. Our group and others have demonstrated that monocytes also contribute to the pathogenesis of IgA nephropathy (IgAN) [11, 12]. Natural killer (NK) cells are a type of white blood cell that have an important role in the recognition and elimination of virus-infected and tumor cells, as well as in the regulation of tissue inflammation [13]. Studies have shown that NK cells are dysregulated in a number of autoimmune diseases, including IgAN and SLE [14–16]. In some autoimmune diseases, the number of NK cells is decreased and NK cell activity in response to stimuli is often impaired in these diseases, but in other diseases, the number of NK cells are positively correlated with disease progression. The mechanisms underlying the dysregulation of NK cells in autoimmune diseases have not been determined.
Single-cell sequencing technology has developed rapidly in recent years. Indeed, dozens of different single-cell transcriptomic sequencing platform shave been developed since 2009 [17–19]. Single-cell sequencing technology is a powerful and increasingly popular method by which gene expression is profiled in individual cells. Using single-cell RNA-sequencing (scRNA-seq), researchers can study differences in gene expression and other characteristics among different types of cells to provide insight into the underlying cause of the disease. The first step in scRNA-seq is to isolate individual cells, then the RNA is analyzed. The RNA is typically isolated from a tissue sample, such as a blood, then converted into complementary DNA (cDNA), which can be sequenced. The cDNA is sequenced using a variety of sequencing technologies, which provide information about the genes being expressed in each cell. This information can then be used to identify the types of cells present, the levels of gene expression levels, and other characteristics. The rapid development of high-throughput and low-cost scRNA-seq technology makes scRNA-seq technology applicable in various fields, including autoimmune diseases [20–22]. By combining this data with other data sources, such as patient medical records or imaging data, researchers can build a comprehensive picture of the underlying causes of autoimmune diseases and provide insight into the autoimmune response and the potential for future treatments.
The majority of scRNA-seq studies have often focused on one type of autoimmune disease, which did not provide sufficient information regarding the heterogeneity and similarity of the immune cells among different autoimmune diseases. Herein we have integrated and analyzed the scRNA-seq results of peripheral blood cells from five different autoimmune diseases (IgAN, Kawasaki disease [KD], MS, SS, and SLE) [11, 23–26]. We discovered that several types of immune cells are markedly altered in these diseases compared with health controls (HCs). Interestingly, the activity of several immune cell populations was significantly increased in some diseases, including classical and non-classical monocytes and NK/NK-T cells. Although immune cells in these five different autoimmune diseases showed strong heterogeneity, we found similarities in specific immune cell subsets within different diseases, suggesting that the pathogenesis of these diseases may be closely relate to these cell subpopulations. In summary, our results provide new insight to further understand the heterogeneity and similarity of immune cells in the pathogenesis among different autoimmune diseases.
Results
Integration of peripheral blood mononuclear cell scRNA-seq data from five autoimmune diseases
To generate an integrative immune cell landscape of autoimmune diseases, we integrated public-available scRNA-seq data of five typical autoimmune diseases, including IgAN, KD, MS, SS, and SLE [11, 23–26]. We downloaded the matrix and performed data quality control, batch effect correction, dimension reduction with Uniform Manifold Approximation and Projection (UMAP), and downstream analysis using Seurat ( Supplementary file 1A ). The amount of RNA per cell, the RNA signature per cell, and the number of mitochondrial and ribosomal genes per cell before and after QC were calculated ( Supplementary file 1B and C ). We observed that these scRNA-seq datasets could be clustered based on transcriptome profiles, although there were some variations between each scRNA-seq experiment. After batch effect correction, the data from the eight batches were clustered together. The batch numbers and the inter-batch integration clustering are shown in the UMAP ( Table 1, Supplementary file 1D and E ). We profiled 55,284, 29,090, 34,985, 25,373, 29,345, and 24,287 cells for HCs, and patients with IgAN, KD, MS, SS, and SLE, respectively. We first divided immune cells into 18 clusters using unsupervised methods. Of 18 clusters, we removed CD45-negative cells and erythrocytes. All cell clusters were observed in the UMAPs of HCs and five autoimmune diseases ( Figure 1A ). Major cell types were identified by the following known unique marker genes: CD14, S100A8, and S100A9 for monocytes; GNLY, NKG7, and GZMB for NK cells; NKG7 and CD3G for NK-T cells; CD3G and CD8A for CD8 T cells; CD3G and CD4 for CD4 T cells; CD19 and IGHM for naïve B cells; and CD19 and JCHAIN for memory B cells ( Figure 1B ). We then compared the cell percentage of each cell cluster among HCs and five autoimmune diseases ( Figure 1C ). Interestingly, compared to the HC cell percentage of each cell cluster, there was a significantly increased percentage of naïve CD4 T cells (cluster 0) and IGHMhigh naïve B cells (cluster 4) in patients with KD, and an increased number of classical monocytes (cluster 1) in SLE, but a significantly decreased number of NK cells (cluster 2) in patients with IgAN and KD, as well as a significantly decreased number of NK-T cells (cluster 6) in patients with KD. We also performed differentially-expressed gene (DEG) analysis of all immune cells in the five autoimmune diseases and functional enrichment of the DEGs ( Supplementary file 2 ). Functional enrichment suggested that the DEGs were primarily related to regulation of multiple immune cells. Specifically, CD14 was highly expressed in IgAN patients, allowing the involvement of monocytes in the activated immune response, as demonstrated by available data [27]. Genes associated with B-cell cloning, such as IGVC-27, were upregulated in KD patients, and functional enrichment was associated with B cell-mediated immune responses. This finding suggests a possible involvement of B cells in the disease process underlying KD, and is consistent with the results of previous study showing that CD20+ B cells were heavily infiltrated in coronary artery biopsies of patients with early-stage patients KD, while an abnormal cell count of plasma cells was present in specimens from late-stage patients [28, 29]. These data are consistent with our volcano mapping and functional enrichment results. With respect to the function enrichment results of SS, T cell activation is also consistent with the theory advanced in the literature, i.e., SS is mainly due to a large number of T cells infiltrating secretory glands, which leads to the occurrence of diseases [30, 31]. These results suggest that there is significant heterogeneity of immune cells among these autoimmune diseases.
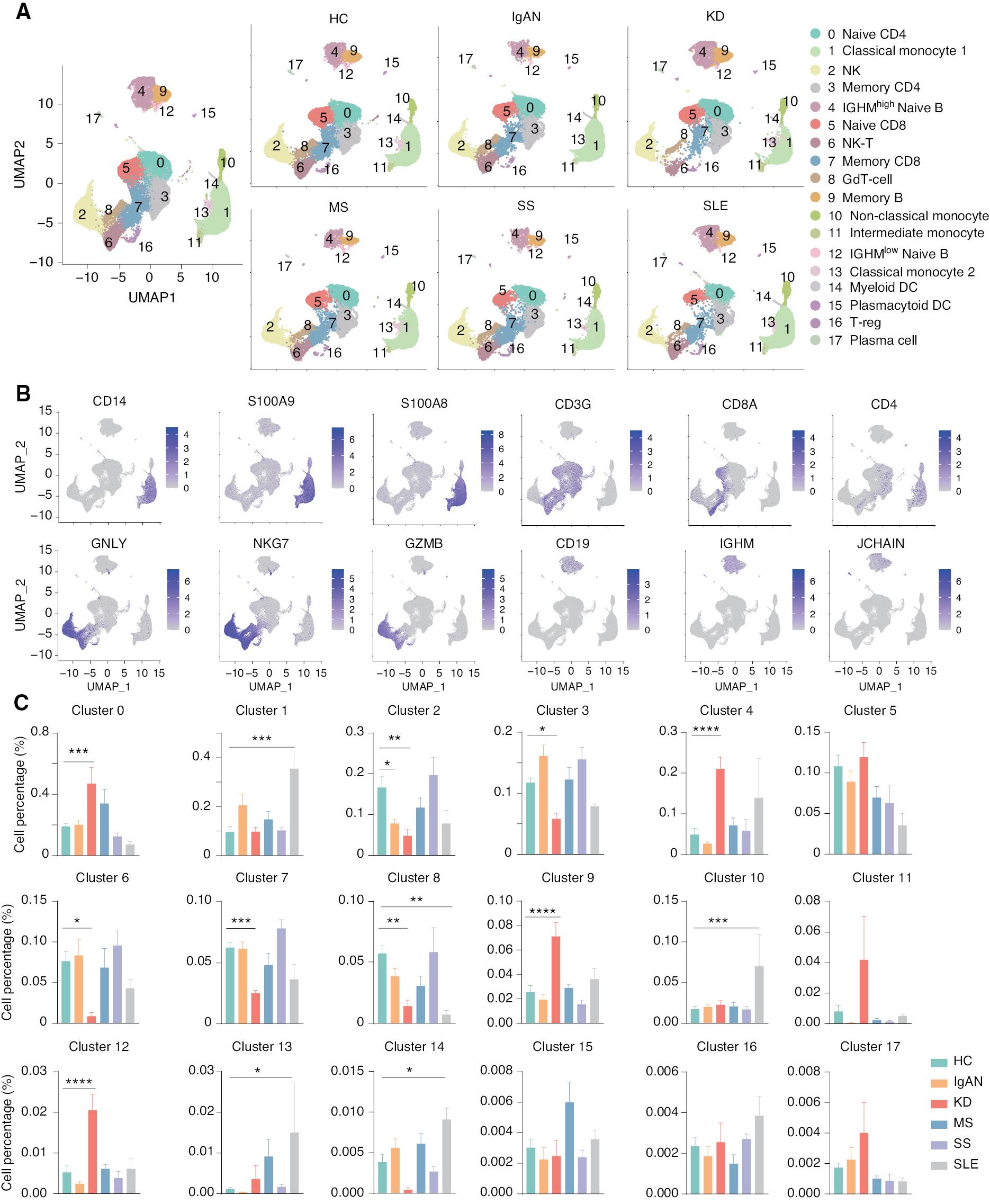
Single-cell landscape of peripheral blood mononuclear cells (PBMCs) from healthy controls and patients with five autoimmune disease. (A) UMAP illustration of the integrated scRNA-seq data clustering of PBMCs from HCs and patients with five autoimmune diseases. (B) Feature plot for identification of genes in major cell populations: CD14, S100A9, and S100A8 were used as subpopulation genes of monocytes; GNLY, NKG7, and GZMB were used as subpopulation genes of NK cells; CD3G, CD8A, and CD4 were used as subpopulation genes of T cells; and CD19, IGHM, and JCHAIN were used as a B cell subpopulation genes. (C) Histograms of the percentage of cells in each cell cluster among HCs and patients with five autoimmune diseases. *P < 0.05, **P < 0.01, ***P < 0.001,****P < 0.0001 vs. HC.
Sample Batch Numbers in this Study
Sample Id | Group | Project Source | Batch |
---|---|---|---|
HC_09 | HC | HRA000831 | 1 |
HC_10 | HC | HRA000831 | 1 |
IgAN_01 | IgAN | HRA000831 | 1 |
IgAN_02 | IgAN | HRA000831 | 1 |
HC_11 | HC | HRA000831 | 2 |
IgAN_03 | IgAN | HRA000831 | 2 |
IgAN_04 | IgAN | HRA000831 | 2 |
IgAN_05 | IgAN | HRA000831 | 2 |
HC_12 | HC | HRA000831 | 3 |
IgAN_06 | IgAN | HRA000831 | 3 |
IgAN_07 | IgAN | HRA000831 | 3 |
IgAN_08 | IgAN | HRA000831 | 3 |
IgAN_09 | IgAN | HRA000831 | 3 |
HC_13 | HC | HRA000831 | 4 |
IgAN_10 | IgAN | HRA000831 | 4 |
HC_01 | HC | GSE157278 | 5 |
HC_02 | HC | GSE157278 | 5 |
HC_03 | HC | GSE157278 | 5 |
HC_04 | HC | GSE157278 | 5 |
HC_05 | HC | GSE157278 | 5 |
pSS_01 | SS | GSE157278 | 5 |
pSS_02 | SS | GSE157278 | 5 |
pSS_03 | SS | GSE157278 | 5 |
pSS_04 | SS | GSE157278 | 5 |
pSS_05 | SS | GSE157278 | 5 |
SLE_01 | SLE | GSE142016 | 6 |
SLE_02 | SLE | GSE142016 | 6 |
SLE_03 | SLE | GSE142016 | 6 |
HC_06 | HC | GSE168732 | 7 |
HC_07 | HC | GSE168732 | 7 |
HC_08 | HC | GSE168732 | 7 |
KD_01 | KD | GSE168732 | 7 |
KD_02 | KD | GSE168732 | 7 |
KD_03 | KD | GSE168732 | 7 |
KD_04 | KD | GSE168732 | 7 |
KD_05 | KD | GSE168732 | 7 |
KD_06 | KD | GSE168732 | 7 |
MS_01 | MS | GSE138266 | 8 |
MS_02 | MS | GSE138266 | 8 |
MS_03 | MS | GSE138266 | 8 |
MS_04 | MS | GSE138266 | 8 |
MS_05 | MS | GSE138266 | 8 |
scRNA-seq analysis revealed the dynamics of the cell-to-cell communication network among five autoimmune diseases
Intercellular communication of immune cells is an important feature of host immunity. Mounting evidence links changes in the cell-to-cell communication network to the varied pathogeneses of autoimmune diseases [32, 33]. Therefore, we compared the cell-to-cell communication network in HCs and patients with five autoimmune diseases by evaluating the interaction strength and probability (i.e., information flow) using CellChat. First, we plotted the interaction strengths of the incoming and outgoing signals in each cell cluster of HCs and patients with the five autoimmune diseases. Three cell clusters, including NK (cluster 2), naïve CD8 (cluster 5), and NK-T (cluster 6) cells, displayed the strongest incoming and outgoing interaction strength, while the other cell clusters showed similar levels of interaction strength in HCs. As expected, this interaction strength pattern was significantly affected in the five autoimmune diseases ( Figure 2A ). Classical monocytes (cluster 1) exhibited the strongest incoming and outgoing interaction strength in IgAN and SLE. This observation is consistent with previous studies [11, 26], suggesting classical monocytes have an important role in IgAN and SLE. Interestingly, the interaction strength of NK (cluster 2) and NK-T (cluster 6) cells was decreased in IgAN, KD, and SLE. In contrast, the interaction strength of NK (cluster 2) and NK-T (cluster 6) cells was remarkably increased in SS, suggesting the activity of NK and NK-T cells may be reduced in IgAN, KD, and SLE, but increased in SS. In addition, the interaction strength of CD22high naïve B cells was increased in KD, suggesting that this subset of naïve B cells may be closely related to the pathogenesis of KD. Unlike the above-mentioned autoimmune diseases, MS exhibited a similar interaction strength pattern with HCs; the interaction strength of naïve CD8 (cluster 5) and NK-T (cluster 6) cells was slightly decreased. We subsequently dissected the interaction strength of the identified signaling pathways in each cell cluster by aggregating the incoming and outgoing strengths. The interaction strength of the individual signaling pathway in each cell cluster of HCs and patients with the five autoimmune diseases was plotted as a heat map ( Figure 2B ). The heat maps exhibited changes in the interaction strength of the corresponding signaling pathways in immune cells of HCs and patients with the five autoimmune diseases. Each disease displayed a distinct phenotype compared to HCs. For example, consistent with Figure 2A , several important signaling pathways, including MIF, BAFF, and OCLN, were only increased in classical monocytes (cluster 1) in patients with IgAN and SLE. Several signaling pathways, including OX40, CD30, and TWEAK, were exclusively increased in NK (cluster 2) and NK-T (cluster 6) cells in patients with SS. To further investigate the contribution of each signal pathway in the diseases, we evaluated the intercellular communication probability by calculating overall information flow in each signal pathway. Surprisingly, we found that there are distinct signaling pathways in each autoimmune disease ( Figure 2C ). The strength of the interactions of each cluster of cells in the pathways were exhibited in heatmaps ( Supplementary file 3 ). For example, CNTN, CDH5, FSH, NGF, IFN-I, GDNF, and BMP10 pathways were exclusively present in patients with IgAN. JAM, CD34, VTN, NMU, and VEGI were exclusively present in patients with KD, while MTSN were present in patients with IgAN and KD. OX40, CD30, and TWEAK were exclusively present in patients with SS, while THY1 and WNT were present in patients with IgAN and SS. CD226 and APRIL were present in patients with KD and SS. In addition, BAFF was increased in patients with SLE. These results revealed the distinct dynamics of the intercellular communication network in these autoimmune diseases.
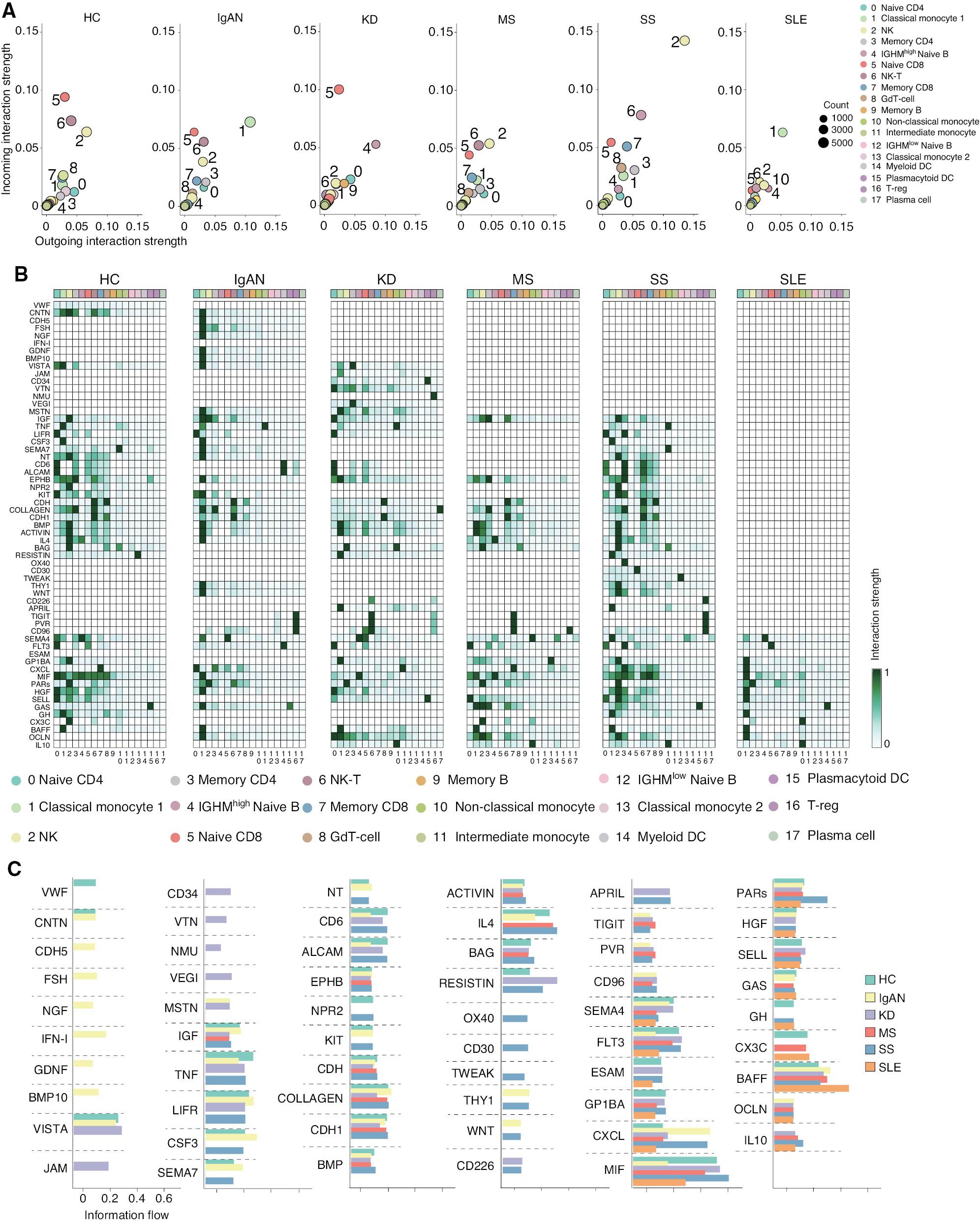
CellChat analysis of the intercellular communication networks in PBMCs from HCs and patients with five autoimmune diseases. (A) Dot plots of the cell-cell interaction strength in each cell cluster of different groups. (B) Heat maps of the interaction strength for the identified pathway across the cell clusters in each group. (C) Information flow intensity histograms of the major interaction pathways among different groups.
scRNA-seq revealed a CCL3high classical monocyte subset in patients with IgAN and SLE
Accumulating evidence indicates that monocytes are involved in the pathogenesis underlying IgAN and SLE, but the specific mechanism has not been established. According to cell-to-cell communication network analysis, the activity of monocytes was shown to be increased in patients with IgAN and SLE. Therefore, we re-analyzed the classical monocyte cluster and divided the cluster into the following 3 subsets according to transcriptional profiles: CCL3high monocyte (cluster 0); CCL3low monocyte (cluster 1); and HSPA1high monocyte (cluster 2; Figure 3A ). The expression and distribution of the fractionated marker of classical monocytes were in UMAP ( Supplementary file 4 ). CCL3high monocytes expressed a high level of pro-inflammatory genes, including CCL3, CXCL8, and IL1B, while HSPA1high monocytes expressed a high level of heat shock protein genes, including HSPA1A, HSPA1B, and HSP90AA1; cluster 1 monocytes expressed a low level of both gene sets ( Figure 3B ). Surprisingly, HSPA1high monocytes (cluster 2) were exclusively present in HCs, but not any patients with autoimmune diseases. HSPA1 encodes heat shock 70 kDa protein 1 (Hsp72), a member of the heat shock protein 70 family, which facilitates the proper folding of newly-translated proteins and stabilizes or degrades mutant proteins [34]. In addition, Hsp72 also facilitates DNA repair [35]. Hsp72 functions contribute to biological processes, including signal transduction, apoptosis, protein homeostasis, and cell growth and differentiation [36]. This result indicates that monocytes in autoimmune diseases have an impaired ability to regulate misfolded proteins and DNA damage. The number of CCL3low monocytes (cluster 1) was significantly increased in patients with SS and SLE, while the number of CCL3high monocytes (cluster 0) was increased in patients with IgAN and SLE, although there was no statistical difference ( Figure 3C and D ). We then compared the level of marker gene expression in HCs and patients with autoimmune diseases. Two pro-inflammatory genes, including CCL3 and IL1B, were increased in the cluster 0 monocytes in patients with IgAN and SLE, but decreased in patients with KD and MS. LY6E was increased in clusters 0 and 1 monocytes of patients with SLE. We also found that two interferon-related genes (IFI6 and IFITM3) were increased in the cluster 0 monocytes of patients with KD, SS, and SLE ( Figure 3E and F ). In addition, we compared the gene expression differences in patients with SLE and IgAN and high CCL3 expression in cluster 0 compared to other subgroups and performed functional enrichment. These results indicated that there is a group of classical monocytes with high CCL3 expression in patients with SLE that may enable classical monocytes to function in the disease process by enhancing cell chemotaxis, response to interferon (IFN)-γ, and cytokine production through high expression of CCL3 and IFI6 genes. We simultaneously compared the expression of differentially-expressed genes in cluster 1 with lower CCL3 expression to other groups in the SLE groups and performed functional enrichment analysis of the resulting DEGs, suggesting that the genes in this cluster also function in interferon signaling. Interestingly, in the group of cells with high expression of CCL3 in patients with IgAN, CCL3L3 was differentially-expressed and CCL3L3 gene was specifically upregulated with HLA-E gene expression, which may be related to the cellular response to IFN-γ ( Supplementary file 5 ). Previous studies have suggested that the expression of IL-1β, IFN-γ, chemokines, and chemokine ligands is upregulated in the macrophages of SLE patients, which leads to inflammation. Our findings are in agreement with previous studies [37–39]. In previous studies of mouse models of autoimmune arthritis, it was reported that excessive production of chemokines by macrophages exacerbate the disease and accelerate macrophage infiltration into the joints, thus leading to increased inflammation [40–42]. Therefore, we presumed that this group of cells exacerbated the disease in patients with IgAN and SLE by expressing large amounts of the CCL3 chemokine gene. These results suggested that CCL3high classical monocytes are closely associated with IgAN and SLE.
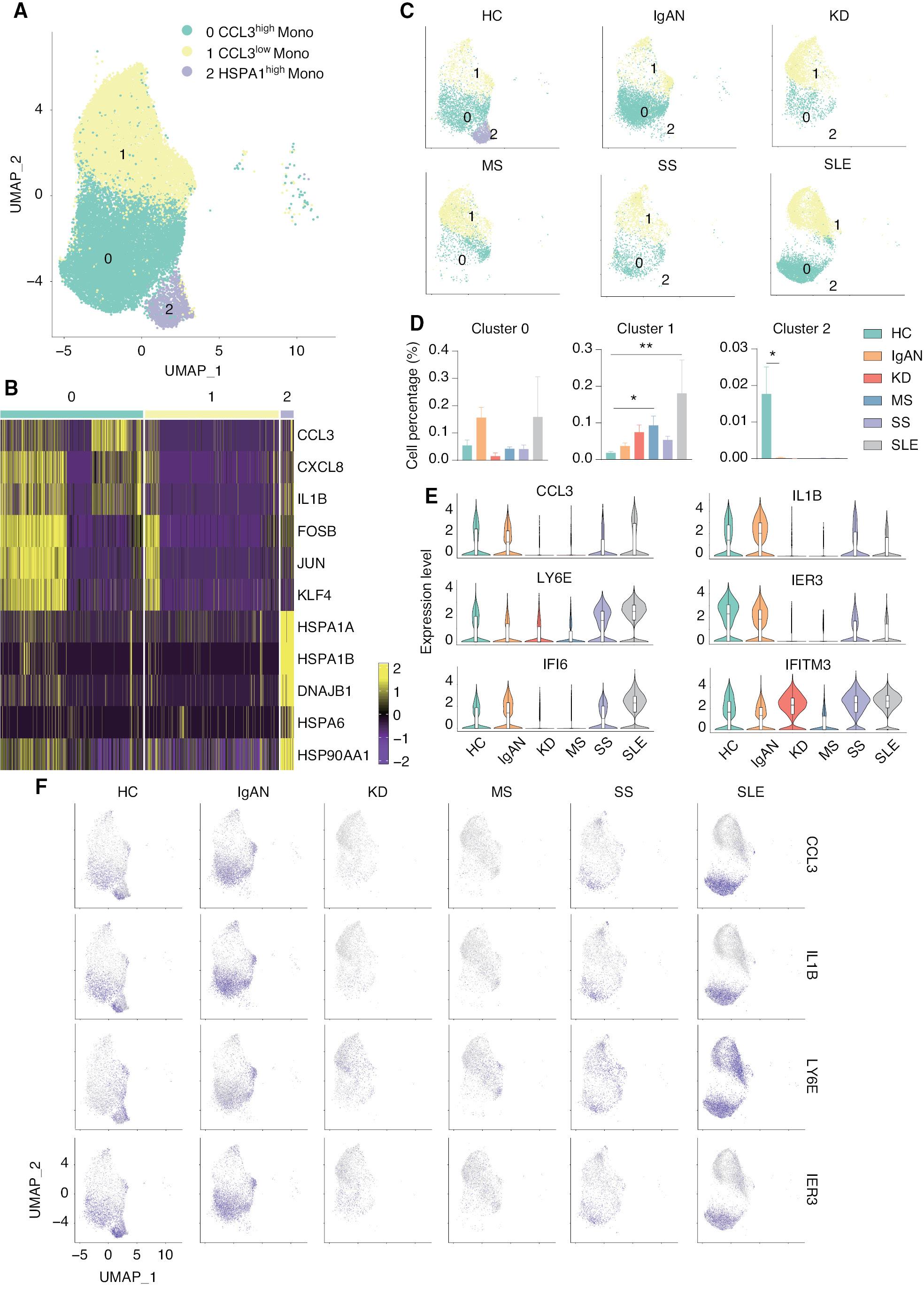
scRNA-seq identified the CCL3high classical monocyte subset as closely associated with IgAN and SLE. (A) UMAP illustrating classical monocyte subsets of all groups. (B) Heat map showing the signature genes of each classical monocyte subset. (C) UMAP illustrating the distribution of the classical monocyte subsets in each group. (D) Histograms of the percentage of cells in each monocyte subset among the groups. *P < 0.05, **P < 0.01 vs. HC. (E) Violin plots illustrating SLE-related genes in HCs and patients with five autoimmune diseases. (F) Feature plots of the upregulated genes in IgAN and SLE.
scRNA-seq identified four non-classical monocyte subsets closely associated with SLE
It was previously concluded that non-classical monocytes also have important roles in the initiation and progression of autoimmune diseases [43]. Therefore, we re-analyzed and divided the non-classical monocytes into 5 subsets: LYPD2highVMO1high (cluster 0); CCL3highS100A8high (cluster 1); CRIP1low (cluster 2); and CXCL10highGBP1high (cluster 3) monocytes ( Figure 4A and B ). The expression and distribution of the fractionated marker for non-classical monocytes are shown in Supplementary file 6 . All groups contain these five subsets of non-classical monocytes; however, compared to HCs, four of these subsets were significantly increased in SLE ( Figure 4C and D ). To further explore the transcriptomic changes in the immune cells of SLE, we analyzed the DEGs of non-classical monocytes between SLE and the other groups. The volcano plot indicated that several interferon-related genes, including ISG15, IFI6, IFI27, and LGASL1, were significantly upregulated in the non-classical monocytes of SLE ( Figure 4E ). Furthermore, the violin plots showed that ISG15, IFI27, LY6E, and FYB were specifically upregulated in SLE, while IFI6 and IFI44L were upregulated in SLE and IgAN. In addition, CXCL8, CD74, and CST3 were shown to be down-regulated in SLE ( Figure 4F ). These results highlight that non-classical monocytes are closely associated with SLE compared with the other four autoimmune diseases.
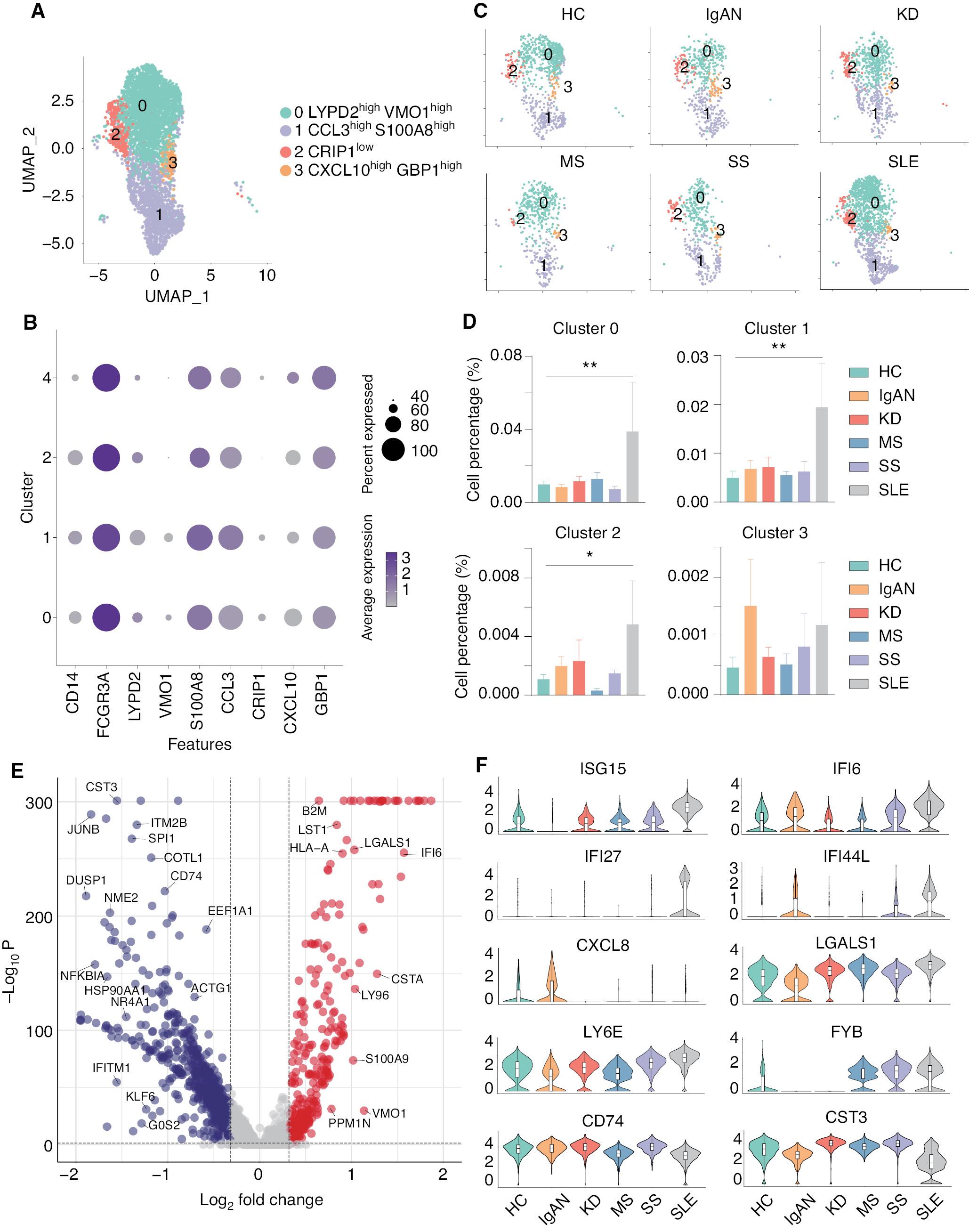
scRNA-seq linked four non-classical monocyte subsets with SLE. (A) UMAP illustrating non-classical monocyte subsets of all groups. (B) Dot plot of marker genes in each subset of non-classical monocytes. (C) UMAP illustrating the distribution of the non-classical monocyte subsets in each group. (D) Histograms of the percentage cells in the non-classical monocyte subsets in each group. *P < 0.05, **P < 0.01 vs. HC. (E) Volcano plot showing the DEGs of SLE versus all other groups. Cut-off P value < 0.05 and log2FC > 0.32. (F) Violin plots illustrating SLE-related genes in HCs and patients with five autoimmune diseases.
scRNA-seq revealed the opposite phenotypes of NK and NK-T cells in five autoimmune diseases
Previous studies indicated that NK cells, which are key components of the innate immune system, have been implicated in the development of multiple autoimmune diseases, including SLE [44, 45]. We also demonstrated that both the number and cell-cell communication networks are affected in autoimmune diseases, including IgAN, SS, and SLE. We then re-analyzed the NK cells and divided the NK cells into 5 clusters: CLIC3high (cluster 0); HLA-DRB1high (cluster 1); GZMKhigh (cluster 2); and IL7RhighLTBhigh (cluster 3; Figure 5A and B ). The expression and distribution of the identified marker for non-classical monocytes are shown in Supplementary file 7 . Interestingly, the number of CLIC3high (cluster 0) NK cell subsets was significantly reduced in IgAN, and was also reduced in KD, although there was no statistical difference. The number of HLA-DRB1high (cluster 1) and GZMKhigh (cluster 2) NK cell subsets was significantly reduced in KD, and was also increased in SS and SLE, although there was no statistical difference ( Figure 5C and D ). Violin plots indicated that receptors for NK cells, including KLRC2, KLRC3, and KIR3DL2, were significantly upregulated in SS. CTSD was upregulated in MS and SS; however, NR4A2, FYB, CXCR4, and NKG7 were downregulated in IgAN and KD ( Figure 5E ), indicating impaired NK cell development and functions in these diseases. The feature plots showed that KLR2 and NKG7 are increased in SS and decreased in IgAN and KD ( Figure 5F ).
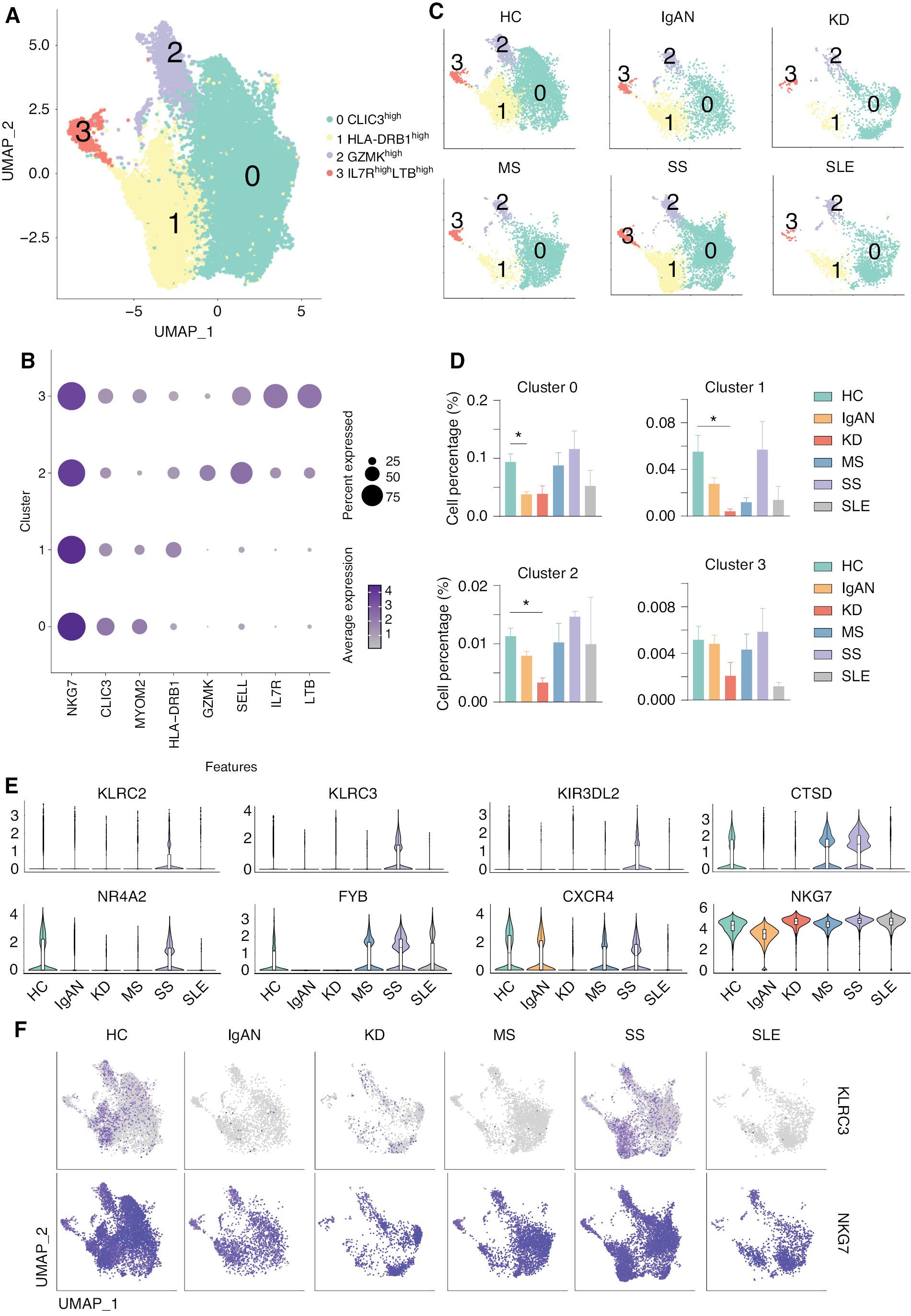
scRNA-seq revealed the different phenotypes of NK cells in five autoimmune diseases. (A) UMAP illustrating NK cell subsets of all groups. (B) Dot plot of marker genes in each subset of NK cells. (C) UMAP illustrating the distribution of NK subsets in each group. (D) Histograms of the percentage of cells in the NK subsets in each group. *P < 0.05 vs. HCs. (E) Violin plots of the specific upregulated genes in patients with MS and SS, and downregulated genes in patients with IgAN and KD. (F) Feature plots of specific upregulated genes in patients with SS and downregulated genes in patients with IgAN.
We then performed differential gene expression and functional enrichment of whole NK cells from patients with IgAN, KD, and SS ( Supplementary file 8 ). The results of functional enrichment showed that KLRC3 genes that were downregulated in IgAN were mainly associated with cellular defense responses of biological processes, while other downregulated genes, such as HLA-A and HLA-B, were associated with negative regulation of immune cells. Similarly, downregulated genes, such as HLA-A and KIR family genes (KIR2DL1) were associated with intrinsic immune response and cell killing by NK cells in KD patients. In contrast, KLRC3 gene expression was upregulated in NK cells of SS patients, while KIR family genes, such as the HLA-A gene, showed opposite trends to NK cell expression in IgAN. This finding suggests that NK cells exhibit different phenotypes and may have exercises different functions in the IgAN, KD, and SS groups. Previous studies indicated that classical HLA I molecules (HLA-A, HLA-B, and HLA-C) are key regulatory factors for NK cell activation, and enable NK cells to recognize self or non-self and diseased cells by presenting peptides from intracellular proteins on the cell surface [46]. In addition, classical HLA I molecules interact with inhibitory and activating NK cell receptors of the KIR family. During functional maturation of NK cells, the interaction with members of the inhibitory KIR (iKIR) family results in activation of NK cells, and activated NK cells are more responsive to the activation of potential target cells than non-activated NK cells [47, 48]. The results of our differential gene expression analysis showed a consistent downregulation of HLA class I molecules and KIR family genes in IgAN and KD patients, whereas these genes showed an upregulated trend in SS patients. Given that the proportion of NK cells was downregulated in IgAN and KD and upregulated in SS, we concluded that NK cells in IgAN and KD exhibit a different phenotype compared to SS.
Natural killer T (NK-T) cells are a heterogeneous group of T cells that share properties of T and NK cells, which has been shown to be closely related to innate and adaptive immunity [49–51]. We therefore analyzed the NK-T cells and divided the NK-T cells into 8 clusters ( Figure 6A ). Cluster 1 expressed high level of CD4, while the other clusters expressed a high level of CD8 ( Figure 6B ). As can be seen in the clustering of NKT cells in each group, clusters 1, 2, 4, 6, and 7 were not equally represented in HCs and other groups ( Figure 6C ). Interestingly, we showed that HSPA1Bhigh (cluster 2), TRBV24-1high (cluster 4), and TRBV15high (cluster 7) NK cell subsets were highly present in HCs, but not in patients with autoimmune diseases, while CD4high (cluster 1) and CMC1high (cluster 6) NK cell subsets were highly present in SS, but not the other autoimmune diseases ( Figure 6D ). Cluster 1 expressed high levels of IL7R and CD40L, which might increase the development of this NK cell subset and stimulate other immune cells through the CD40-CD40L pathway [52, 53]. Cluster 6 expressed high levels of CD8, TRAV12-2, and CMC1 ( Figure 6E ). TRAV12-2 belongs to the TCR family and the V alpha gene segments belong to 12 different subfamilies, each containing 1-7 members [54]. We found that TRAV12 family genes were highly expressed in the cluster 6 NK cell subset, which was highly related to SS. CMC1, a component of the mitochondrial translation regulation assembly intermediate of cytochrome c oxidase complex (MITRAC) complex, regulates cytochrome c oxidase assembly [55]. A study showed that CMC1 regulates the turnover of newly-synthesized COX1 [56], indicating the NK and NK-T number and mitochondrial function might be affected in SS. Taken together, these results suggest opposite phenotypes of NK and NK-T cells in different autoimmune diseases.
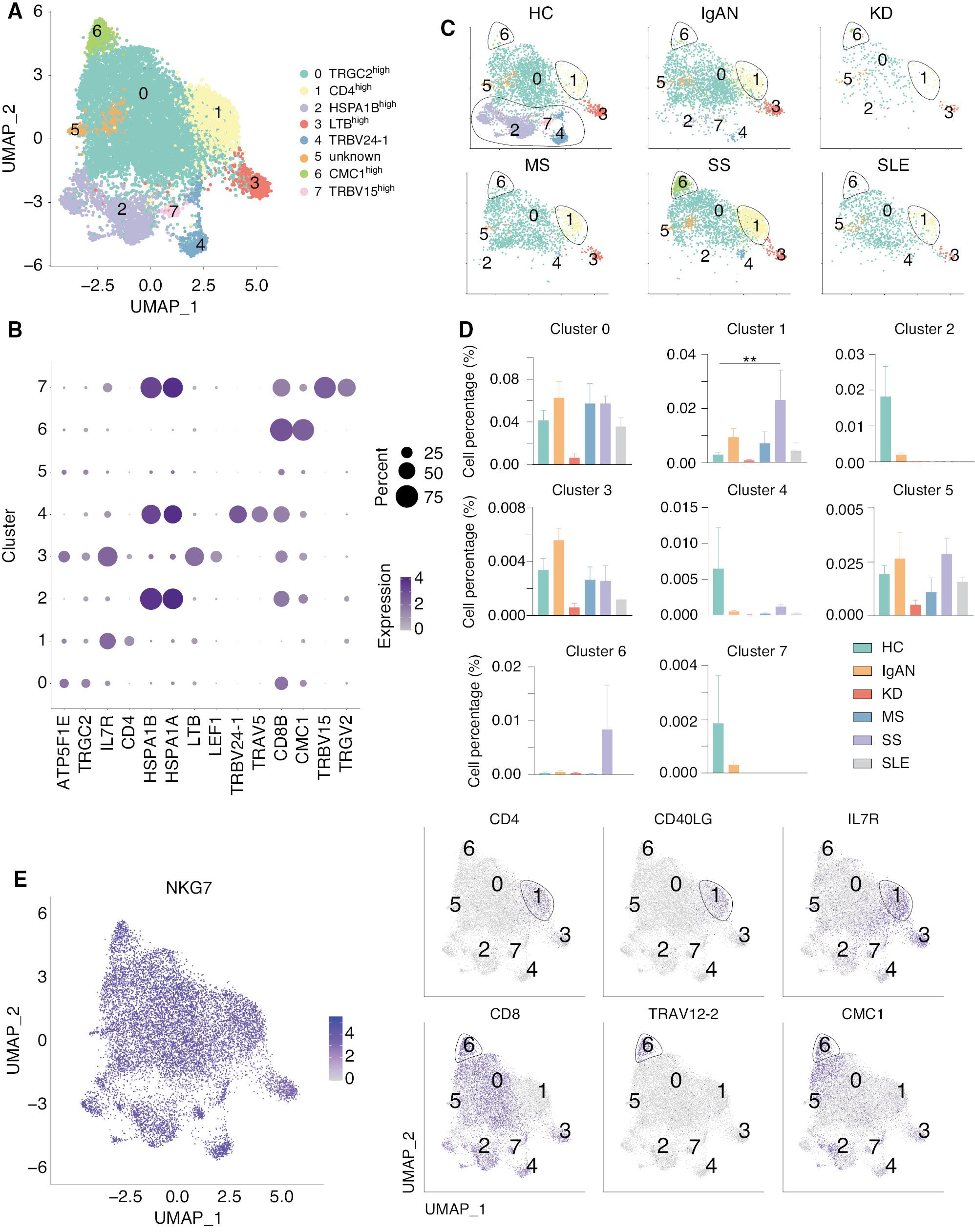
scRNA-seq identified two unique NK-T subsets in SS. (A) UMAP illustrating NK-T cell subsets in all groups. (B) Dot plot of the marker genes in each subset of NK-T cells. (C) UMAP illustrating the distribution of the NK-T subsets in each group. Clusters 2, 4, and 7 were present in HCs, but not present in patients with five autoimmune diseases, while clusters 1 and 6 existed in patients with SS, but not the other diseases. (D) Histograms of the percentage of cells in the NK subsets among each group. **P < 0.01 vs. HCs. (E) Feature plots showing the marker genes for NK cells, T cells, and clusters 1 and 6.
Discussion
Immune cell heterogeneity has a major role in the development of autoimmune diseases, such as IgAN, MS, and SLE. The immune system is composed of various cell types, including T cells, B cells, macrophages, and NK cells, which have different roles in the defense against foreign antigens. While a healthy immune system effectively recognizes and responds to invading pathogens, an autoimmune response occurs when the immune system incorrectly attacks the body’s own cells and tissues. In the case of autoimmune diseases, the immune system misidentifies self-tissues, resulting in an inflammatory response that causes damage to cells and tissues.
With the development of single-cell sequencing technology, researchers have a deeper understanding of the varied pathogeneses underlying autoimmune diseases. More-and-more studies have applied this technology to explore the novel immune cell populations and the genes that may contribute to the pathogenesis of autoimmune diseases, including IgAN, KD, MS, SS, and SLE [11, 23–26]. The motivation to perform scRNA-seq integration analysis was based on the observation that in different autoimmune diseases, even though immune cells have strong heterogeneity, the immune cells also share similarities. For example, aberrations in monocyte/macrophage number and function are increasingly recognized in both mice and humans with SLE. Studies have shown that monocytes have a positive role in accelerating inflammation and injury in skin and glomerular lesions [57]. Our group and other groups both demonstrated that the number and function of monocytes in patients with IgAN were highly associated with the pathogenesis underlying IgA [11, 12]. Together, these results indicated that monocyte share similar phenotypes in patients with IgAN and SLE, which partially explained why targeting the monocyte/macrophage-derived molecule, BAFF, is effective in these two diseases [58, 59].
In addition to monocytes, recent studies have provide evidence for the involvement of NK cells in the pathogenesis of SLE. The number of NK cells is significantly reduced in Lpr mice, a model of SLE, and adoptive transfer of NK cells delays the onset of autoimmunity, suggesting a protective role of NK cells in SLE [60]. Some studies also demonstrated that NK cells delay the onset of SLE by inhibiting the secretion of autoantibodies in B cells. Increased cytotoxic and proinflammatory phenotypes of NK cells are associated with downregulation of CD3ξ expression in SLE patients [61]. Our group also demonstrated that NK cell number and cytotoxicity function are decreased in IgAN [11]. These results indicate that NK cells may share similar function in different autoimmune diseases.
After integration analysis of five autoimmune diseases, we showed that all samples contained 18 different immune cell subsets, although the cell cluster populations were different among five diseases. Based on intercellular communication network analysis, we showed that classical and non-classical monocyte signaling was significantly enhanced in IgAN and SLE, and further analysis demonstrated that non-classical monocytes were highly associated with SLE. Transcriptomic analysis of classical and non-classical monocyte subsets further revealed that pro-inflammatory cytokines and interferon-related genes, including CCL3, IL1B, ISG15, and IFI6, were specifically increased in IgAN and SLE. Although the signals of NK and NK-T cells were reduced in IgAN and SLE, the number and function of NK and NK-T cells were increased in SS, indicating an opposite immune phenotype of NK cells in these diseases.
Conclusions
In summary, by integration of the scRNA-seq results, we discovered various changes in the immune cell landscape of five different autoimmune diseases with respect to immune cell subsets, populations, DEGs, pathway enrichment, and cell-cell communication network. Our data provide new insight to further determine the heterogeneity and similarity among different autoimmune diseases.
Methods
Data acquisition
All single cell RNA seq matrices were obtained from the Gene Expression Omnibus (GEO) public database. The HC matrix were obtained from the IgAN, KD, and SS cohorts. The scRNA-seq matrix of 10 IgAN patients and 6 age-matched HCs were retrieved from The BIG Submission (BIG Sub [No. HRA000831]) [11]. The scRNA-seq matrix of 6 KD patients and 3 HCs were obtained from the GEO (accession number, GSE168732) [23]. The scRNA-seq matrix of 5 MS patients were acquired from GEO (accession number, GSE138266) [24]. The scRNA-seq matrix of 5 SS patients and 5 age-matched HCs were obtained from GEO (accession number, GSE157278) [25]. The scRNA-seq matrix of 3 SLE patients were obtained from GEO (accession number, GSE142016) [26].
Data processing and analysis
Quality control and data filtering
We used the Seurat v4.0.2 Bioconductor package [62] for quality control, normalization, dimensional reduction, batch effect removal, clustering, and visualization. The count data of all inter-cluster samples were used for the following criteria: all unique molecular identifiers (UMI) between 200 and 2500; and < 15% of mitochondrial genes to quality control and filter low-expression cells.
Integration of count data
We grouped the data from number HRA000831 into batches 1-4 according to the original literature description, and the data from all samples from GSE157278, GSE142016, GSE168732, and GSE138266 were sequentially coded as batches 5-8. We then used the code-numbered data with canonical correlation analysis (CCA), an analysis of identifying the cross-sample pairs in matching biological states as anchors, which correct for technical differences between samples. We used default parameters to retain as much biological heterogeneity as possible between groups, except the non-classical monocyte cluster. Because of the lower cell number of non-classical monocytes, we amended the k.weight of IntegrateData () function parameer in analyzing the batch effect of non-classical monocytes.
Clustering
The integrated matrix was scaled and the first 30 dimensions derived from principal component analysis (PCA) were used for unified stream shape approximation and projection (UMAP). We used the Wilcox test to find differential expression between diverse immune cells. The cut-off of output result follows the standard, min.pct=0.25, logfc.threshold=1.
Differentially-expressed gene (DEG) analysis
We used the function FindMarkers () with the default Wilcox test to find the differential expression for a single cluster compared to all other cells. DEGs had the following criteria: (1) P-value ≤ 0.05; and (2) log2 FC ≥ 0.32, where log2 FC means the log fold-change of the average expression between the two groups.
Intercellular communication network analysis
We used the R package of Cellchat to perform the intercellular communications between immune cells [63]. In brief, we used the backend database and network modeling to predict major signaling between input and output communications, and also classified the signaling pathway in the diversity biological environment to identify context-specific pathways.
Enrichment
The R package (clusterProfiler 4.1.4) [64] was applied to Gene Ontology (GO) [65] to perform functional enrichment of differential gene expression. It is possible that the DEGs were abundantly enriched with mitochondrial- and ribosomal-related genes due to the different sequencing depth and annotation version of the raw data. Therefore, the entries related to immune cell function were selected for functional enrichment results.