Significance statement
A 2D ultrasound examination is the primary technique for follicle monitoring, but 2D ultrasound follicle monitoring has significant inter- and intra-observer variability. In this study we proposed a novel deep learning-based automated model for accurate 2D ultrasound follicle monitoring. Our results suggest that the proposed method provides an accurate and fast approach for novices to improve the reliability and receptivity for 2D ultrasound follicle monitoring in clinical practice, especially in multiple follicle cycles.
Introduction
In vitro fertilization and embryo transfer (IVF-ET) is one of the most common assisted reproductive techniques currently utilized, and serial follicle monitoring has important clinical significance during treatment [1, 2]. Serial assessments of follicle diameters have been tested to determine the time for oocyte retrieval during assisted reproductive techniques [3]. In clinical practice, a two-dimensional (2D) transvaginal ultrasound examination is the primary technique used to measure ovarian follicle diameter. Two-dimensional transvaginal ultrasound follicle monitoring allows clinicians to determine the number, diameter, and development of follicles; however, 2D transvaginal ultrasound follicle monitoring is subject to high inter- and intra-observer variability [4, 5]. Less experienced sonographers are at a greater risk of misdiagnosis, which increases the number of false positives [6, 7]. Follicle diameter measurement is highly heterogeneous, thus IVF-ET treatment results can vary [8]. In addition, with the age of infertility onset becoming progressively younger, the workload of sonographers has increased significantly and the excessive workload often leads to measurement errors, missed diagnoses, and other adverse conditions.
Automated and accurate measurements of ovarian follicle diameters are important. Thus, the use of automated ultrasound software to improve the precision of diameter measurements has garnered increased attention. As early as 1997, researchers proposed intelligent algorithms for the automated detection of follicles with a recognition rate of approximately 70% [9]. Then, some studies that focused on the recognition of follicles through enhanced algorithms to improve the follicle recognition rate were reported [10, 11]. In addition, intelligent algorithms were not only used for the automated detection of follicles, but also for estimation of the size of follicles and ovarian classification [12, 13]. With the rapid development of intelligent algorithms, research on automated detection and measurement of follicles are on the rise. These studies showed that intelligent algorithms help in the detection and measurement of follicle development of patients to assist clinicians in making medical decisions. Due to advances technology, an ultrasound-based computer-aided diagnosis system based on ultrasound image analysis techniques has been developed and introduced to commercially-available ultrasound machines [14–16]. Artificial intelligence (AI)-assisted ultrasound software can assess multimodal data and provide objective measurements, which can improve the ultrasound clinical workflow and reduce workload. Currently, the clinical utility of software has been evaluated in some studies of female fertility, such as SonoAVC (GE Healthcare, Zipf, Austria) and Virtual Organ Computer-aided Analysis (GE Healthcare, Zipf, Austria). There is no consensus on the measurement effect of these software algorithms. Time-consuming, complicated, operations and decreased accuracy are still problems which limit the clinical application of software algorithms [17, 18]. In this study, we introduced an automated model based on deep learning for fast and precise 2D ultrasound follicle monitoring, and evaluate the accuracy, repeatability, and reliability in clinical practice.
Materials and methods
Patients
Infertility female patients who underwent ovulation induction or IVF treatment in the reproductive center from January–August 2020 were prospectively recruited. The inclusion criteria were as follows: (i) both ovaries present; and (ii) no severe reproductive or systemic illnesses. The exclusion criteria were as follows: (i) incomplete information or images; and (ii) abnormal ovarian mass > 3 cm in diameter. The patients undergoing ovulation induction, who were considered to undergo single follicle cycles, received 50–100 mg of clomiphene citrate on days 5–9. Women undergoing controlled ovarian hyperstimulation (COH) before IVF, who were considered to undergo multiple follicle cycles, were treated using the long, antagonist, or mini-stimulation protocol. The gonadotropin-releasing hormone (GnRH) antagonist was continued until the day of human chorionic gonadotropin (hCG) administration. This study was conducted in strict accordance with the ethical guidelines of the Declaration of Helsinki. Ethical approval was granted by the local Ethics Committee, and each patient was informed about the aim of the present study and signed an informed consent prior to ultrasound examination.
Ultrasound examinations
All ultrasound examinations were performed using Acclarix LX9 (Edan Medical, Shenzhen, China) with a 3.0∼10.0 MHz E10-3HQ probe. Examinations and ultrasound image collection were performed by two experts who had 8 years of clinical experience in the evaluation of gynecologic ultrasound data. The boundary of each targeted follicle was clearly visualized during image acquisition. The mean diameter of the follicles was calculated by measuring the vertical lines on the plane of maximum area ( Figure 1 ). During the ultrasound monitoring of single follicle cycles, 5–10 ultrasound images of the leading follicle were collected from each cycle before ovulation, whereas 20–36 follicle ultrasound images were randomly collected from each multiple follicle cycle before oocyte retrieval. The images were exported to an external computer in a JPG file and digital imaging and communications in medicine (DICOM) format.
Statistical analysis
Statistical analysis was performed with SPSS version 25.0 and MedCalc version 20.2. Quantitative data are presented as the mean ± standard deviation. Kappa analysis was used to detect differences between the experts. The follicle border segmentation result evaluated by experts was regarded as the gold standard; only the consistency of the two experts was stable. Bland-Altman plots were used to assess the agreement between automated software, novices, and experts. The maximum allowed difference between methods was set at 2 mm. ICCs and 95% confidence intervals (CIs) were used to assess inter-observer repeatability of ovarian follicle diameter measurements. Repeatability was defined as the consistency between repeated ovarian follicle diameters. A P <0.05 was considered statistically significant.
Model establishment and validation
We introduced a cascaded, fine-grained boundary rendering scheme to address the challenges for follicle and ovary segmentation raised by the ambiguous boundaries in ultrasound images. This scheme selectively identified and refined the pixels with high uncertainty around the boundary. The pixels with predicted probabilities of approximately 0.5 were identified as the candidates to be refined. The refinement was then performed by rendering the uncertain predictions based on the fine-grained feature representations, which were re-encoded from the feature activations of Unet. The re-encoding block consisted of two convolutional layers and a lightweight multi-layer perceptron as the prediction head. To determine the context of information and better address the over- and under-segmentation problems, we proposed to further implant the rendering module into a cascaded scheme. Within the cascade, several deep neural networks were stacked stage-by-stage for enhancement of segmentation performance. To further the use of the deep learning model in clinical practice, we packed the algorithm into automated software. Clinical validation was used to evaluate the value of automated software. The software was loaded onto the ultrasound equipment, and automatic follicle mean diameter measurements were performed in each patient ( Figure 2 ). Assessment of accuracy, reliability, and repeatability were performed by two experts with 8 years of ultrasound follicle monitoring experience and 1 novice with 1.5 years of ultrasound follicle monitoring experience.
Results
Patient characteristics
Fifty-eight patients undergoing multiple follicle cycles were excluded from the study; 40 patients have incomplete information or images and 18 patients had cysts or abnormal ovarian masses > 3 cm in diameter during monitoring. Three hundred infertility patients, including 130 undergoing single follicle cycles and 170 undergoing multiple follicle cycles, who agreed to participate in this study. In the final dataset, there were 228 follicle samples in the single follicle cycle group and 1065 follicle samples in the multiple follicle cycle group. Table 1 shows the baseline characteristics of the two groups. There were no significant differences in age, weight, height, and body mass index between the two groups (P > 0.05).
Baseline Characteristics of the Patients in Single and Multiple Follicle Cycles
Characteristics | Multiple Follicle Cycles | Single Follicle Cycles | P Value |
---|---|---|---|
Age (year) | 31.3 ± 4.4 | 32.2 ± 5.1 | 0.236 |
Weight (kg) | 54.1 ± 7.5 | 55.4 ± 9.6 | 0.336 |
Height (cm) | 157.8 ± 4.7 | 158.0 ± 5.7 | 0.805 |
BMI (kg/m2) | 21.7 ± 2.8 | 22.2 ± 3.7 | 0.340 |
Values were presented as the mean ± standard deviation. BMI: body mass index. P < 0.05 was considered a statistically significant difference.
Gold standard and model performance
Kappa analysis showed good consistency between the two experts in assessment of follicle border segmentation with a kappa value of 0.790. The accuracy of follicle boundary recognition by the automated model reached 0.931. The reliability of follicle diameter measurements estimated by calculation of the ICC is shown in Table 2 . Compared with the novice, the automated model had a higher ICC in mean diameter measurements during single and multiple follicle cycles. According to the 95% limits of agreement, there were no significant differences between the ICCs.
Reliability of Follicle Mean Diameter Measured by a Novice and Automated Model in Single and Multiple Follicle Cycles
Multiple Follicle Cycle | Single Follicle Cycle | |||||
---|---|---|---|---|---|---|
ICC | 95% CI | P Value | ICC | 95% CI | P Value | |
Automated model vs. expert | 0.984 | 0.945–0.993 | <0.001 | 0.970 | 0.961–0.977 | <0.001 |
Novice vs. expert | 0.963 | 0.896–0.981 | <0.001 | 0.965 | 0.937–0.978 | <0.001 |
There was no inter- or intra-observer variation for the automated model because the model always outputs the same segmentation result. Bland-Altman plots were used to estimate the repeatability of mean follicle diameter measurements obtained by the automated model, the novice, and experts ( Figure 3 ). The 95% limits of agreement between the automated model and experts (−2.02 to 2.39 mm) was lower than the novice (−1.69 to 2.74 mm) in single follicle cycles. The mean difference values were 0.19 mm and 0.52 mm, respectively. The 95% limits of agreement between the automated modal and experts (−0.68 to 1.50 mm) was lower than the novice and experts (−0.58 to 1.73 mm) in multiple follicle cycles, and the mean difference values were 0.41 mm and 0.57 mm for the automated model and novice, respectively.
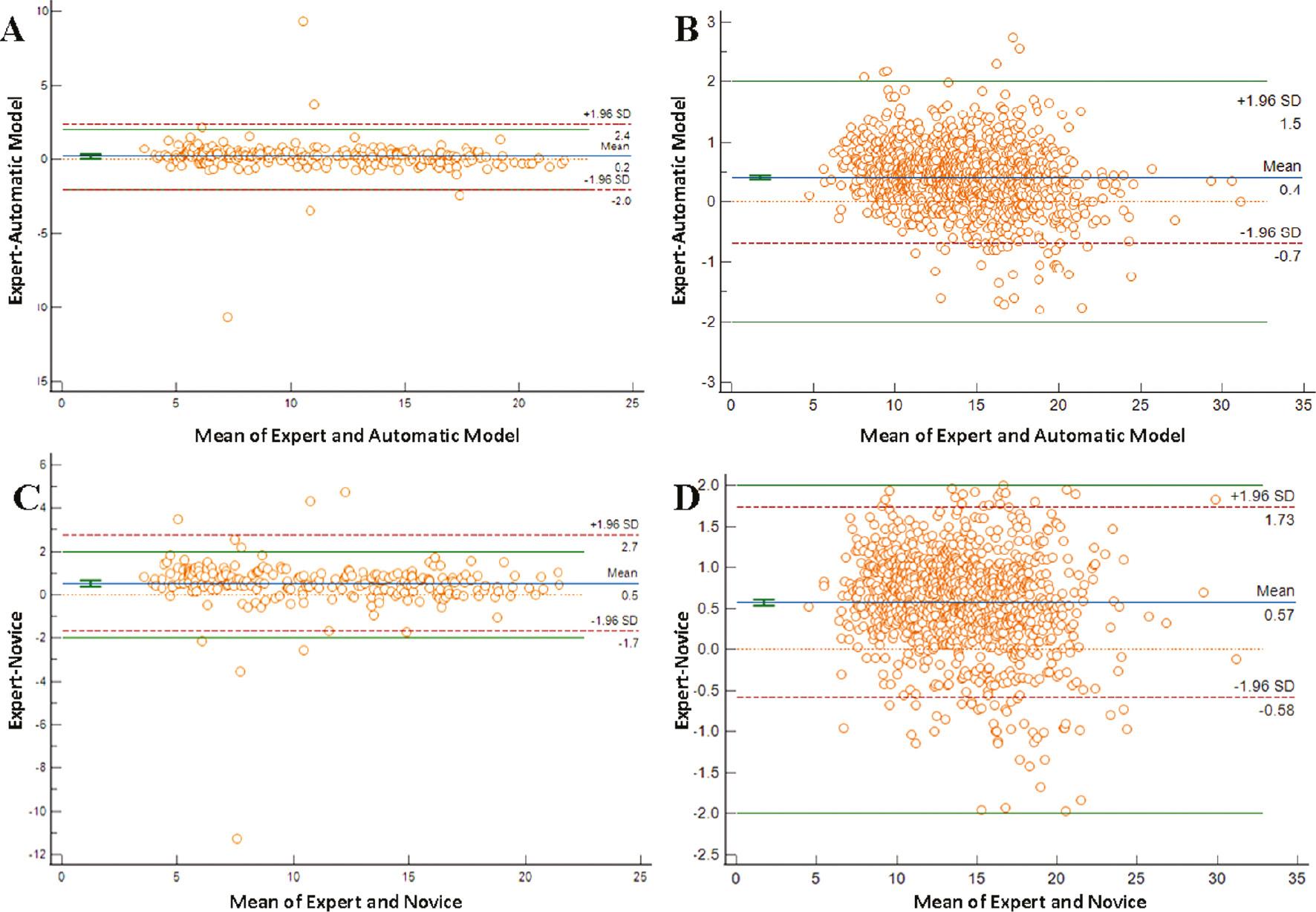
Bland-Altman plots for assessment of repeatability in mean diameter measurements. Plots represent the difference between observers’ measurements and mean measurements. The top and bottom lines show the 95% limits of agreement; the middle line shows the mean difference. A and C. single follicle cycles; B and D. multiple follicle cycles.
The criterion for a higher fertilization rate was a mean follicle diameter > 10 mm. In this study, reliability of follicular mean diameter (measured by automated model) greater than or less than 10mm estimated by calculation of the ICC, were 0.967 and 0.834 in single follicular cycles, 0.970 and 0.609 in multiple follicular cycles, respectively ( Table 3 ). ICC value of follicular diameter ≥10 mm calculated by automated model was significantly higher than measurement of follicular diameter <10 mm in multiple follicular cycles. In single follicular cycles, there were no significant differences between the two groups.
Reliability of a Follicle Mean Diameter Greater than or Less than 10 mm Estimated by an Automated Model in Single and Multiple Follicle Cycles
Multiple Follicle Cycle | Single Follicle Cycle | |||||
---|---|---|---|---|---|---|
ICC | 95% | P Value | ICC | 95% | P Value | |
Follicle diameter ≥10 mm | 0.970 | 0.829–0.998 | <0.001 | 0.967 | 0.796–0.988 | <0.001 |
Follicle diameter <10 mm | 0.609 | 0.352–0.754 | <0.001 | 0.834 | 0.114–0.946 | <0.001 |
Discussion
Ultrasound is an essential and common approach to monitor the development of follicles in the treatment of infertility. Given the significant time required for the measurement of ovarian follicle diameter and variability between different clinicians, AI-assisted technology for monitoring follicles is necessary [19]. In this study we have introduced and validated a novel deep learning-based automated model for fast and accurate segmentation of follicles on 2D ultrasound images. We showed that this automated model improved follicle boundary recognition and achieved higher repeatability and reliability, especially in multiple follicle cycles of patients undergoing COH treatment before IVF.
Currently, measurement of the mean follicle diameter on 2D ultrasound images remains the preferred method to assess follicle size [5]. This method still faces the problem of insufficient standardization; thus, there are significant differences between follicle diameter measurements [20]. Earlier studies constructed models to recognize and measure the follicle [21] that are less time-consuming than manual measurement, especially for irregularly-shaped follicles [22]. One challenge in AI-assisted ultrasound follicle monitoring is to achieve better clinical applicability. Although previous studies have improved the efficiency of follicle recognition and measurement by enhancing existing algorithms or applying new algorithms, the majority of these methods are still in preclinical studies [23]. In our previous study, we proposed a deep learning-based algorithm of CR-Unet for follicle segmentation in which the dice similarity coefficients (DSCs) reached 0.858 [24]. In the present study the results of ICC and Bland-Altman analyses indicated that the variation between the automated model and expert measurements was less than the variation between the novice and expert measurements. The automated model provides a potential approach to improve the accuracy of follicle monitoring, especially for novices and areas lacking medical resources. Our findings are similar to the previous studies [25, 26]. This is the first study to validate a deep learning-based follicle monitoring model performed on 2D ultrasound equipment in clinical practice.
One of the common problems of AI-assisted algorithms is the clinical application efficiency is lower than the laboratory efficiency. Because industrialization is the final goal, the integration among multiple domains and industries is of great significance [27]. In this prospective pilot study, we determined whether an automated model based on a deep learning algorithm could be used to fulfill the clinical demand. This study showed that automated software has achieved a high rate (> 0.90) on the boundary recognition of follicles. We reviewed the dataset and showed that the boundary recognition of 31 multiple and 3 single cycles were incorrect using the automated model. The main reason for this finding was poor image quality, in which the boundaries of the follicles were not clearly recognized.
In studies addressing the impact of follicle monitoring on fertility outcome, it was reported that the fertilization rate increases when the follicular diameter is > 10 mm, and this criterion is also regarded as predicting mature oocytes in ultrasound follicle monitoring [28]. In the current study we drew the follicle samples in two groups based on a mean follicle diameter greater than or less than 10 mm, and further estimated the reliability of measurement obtained by the automated model in both single and multiple follicle cycles. Interestingly, we found that the automated model was conducive to follicle diameter measurement which was ≥ 10 mm. The accurate measurement rate of follicles in multiple follicle cycles was higher than single follicle cycles because the boundary of the follicle diameter ≥ 10 mm is more distinct. It was noted that automated software had inferior performance in follicle diameters < 10 mm in multiple follicle cycles. Follicle overcrowding can cause follicle compression and elongation in one plane, and errors in the measurement of diameters will be exacerbated because of confusion and subjectivity of 2D diameter measurements of follicles in multiple follicle development during COH cycles [29]. In the early stage of follicle development in multiple follicle cycles the emphasis of an AI-assisted model of ultrasound follicle monitoring should be follicle counting, while during the late stage of follicle development, it should be the precise segmentation of follicles ≥ 10 mm.
There were some limitations in our study. The model was verified in a single center, rather than in a multi-center setting. In addition, the image quality was not quantitatively evaluated. Because the automated model was proved to be more applicable to follicles ≥ 10 mm, the performance of deep learning-based segmentation in small follicle tracking and counting was not considered. Continuous improvement of the algorithm will be performed in our corollary studies.
Conclusion
An automated model for 2D ultrasound follicle monitoring, which avoids inter- or intra-observer variation, provides a reliable technique for the novice to improve monitoring accuracy, feasibility, and repeatability, especially in multiple follicle cycles. The automated model for 2D ultrasound follicle monitoring belongs to a class of automated systems that may be useful for ensuring consistency of repeated ovarian follicle diameter measurements, standardize measurement criteria for large cohort studies, and improve the quality of data collection.