1. INTRODUCTION
It has been 3 years since coronavirus disease 2019 (COVID-19) was first reported. The impact of the emerging disease remains to be determined. China recently implemented a new policy for managing COVID-19. Indeed, termination of the quarantine policy brought new challenges to this developing country with a huge population. Currently, most COVID-19 infections are mild, but there are still patients with a poor prognosis. According to a survey by the Chinese Center for Disease Control and Prevention, up to 15.8% of confirmed cases are severe or critical [1]. The fatality rate of critical cases is up to 49.0% [2], which is of great medical concern. According to previous studies [3–5], older age combined with clinical and laboratory characteristics, such as dyspnea, fever, and elevated creatine kinase, are correlated with severe and critical illnesses. Thus, it is necessary to develop an easy-to-use prediction tool for COVID-19 to improve the prognosis.
A nomogram is a line chart that displays scales for the variables included in a formula so that the appropriate values for each variable lie in a line that intersects all the scales. Nomograms have been used as a prediction tool for the prognosis of multiple diseases [6–8]. The nomogram is a better and easier risk-prediction tool in clinical practice compared to other prediction tools that require computer software [9].
Computed tomography (CT) is an essential technique for the diagnosis and management of COVID-19 [10]. Ground-glass opacities (GGOs) and consolidation distributed predominantly in the bilateral subpleural segments are considered the most common CT characteristics of COVID-19 infections [11]; however, the CT characteristics are not quantifiable, which limits prediction of disease progression. The chest CT score is a quantitative method to demonstrate the extent and nature of pulmonary lesions, and has been used in studies of severe acute respiratory syndrome [SARS] [12] and pulmonary fibrosis [13]. Some studies have also applied CT scores to evaluate COVID-19 severity, which showed that the CT score is correlated with clinical parameters indicating deterioration [14, 15]. Thus, the CT score could be an excellent method for the management of patients > 65 years of age with COVID-19 infections [16].
Several nomograms have been developed for predicting severe and critical COVID-19 cases, the majority have relied on clinical or laboratory characteristics [17, 18]. A few models with excellent performance based on CT radiomics have recently been built [19, 20]. Because CT radiomics is not available in the majority of hospitals in China, we developed a feasible and efficient nomogram using clinical and CT scores to predict COVID-19 severity and optimize the prognosis in a timely fashion.
2. METHODOLOGY
2.1. Patients
A total of 346 patients with confirmed COVID-19 infections were enrolled from the Public Health Clinical Center of Chengdu between August 2021 and December 2022. The patients were divided into non-severe (moderate and common) and severe groups based on clinical outcomes [21]. The severe group was required to fulfill at least 1 of the following criteria: (1) shortness of breath (respiratory rate ≥ 30 breaths/min); (2) arterial oxygen saturation at rest ≤93%; (3) the ratio of partial pressure of oxygen-to-fraction of inspired oxygen (PaO2:FiO2) ≤300 mmHg; (4) respiratory distress; (5) onset of shock; (6) requirement for mechanical ventilation due to respiratory failure; and/or (7) organ failure necessitating intensive care unit (ICU) admission.
2.2. Clinical data
Demographic information (age and gender) and clinical parameters (symptoms, comorbidities, and cigarette smoking history) at the time of admission were derived from medical records. Laboratory parameters included routine blood tests, arterial blood gas values, coagulation testing, cardiac enzyme levels, and hepatic and renal function tests.
2.3. CT image characteristics and CT scores
All CT images were non-contrast. Images of the first CT examination were evaluated separately by two senior radiologists. In circumstances in which there were conflicts, the final judgment was reached by consensus. The Radiology Society of North America consensus statement on reporting chest CT abnormalities related to COVID-19 was used to classify the CT signs [22]. Chest CT signs included GGOs, consolidation, nodules, the halo sign, reticular patterns, crazy-paving patterns, septal thickening, traction bronchiectasis, bronchial wall thickening, air bronchograms, lymphadenopathy, pleural thickening, and pleural effusions.
We adopted the CT scoring system designed by Salaffi et al. [23]. Bilateral lobes were assessed at 3 levels with 6 parts and divided by the carina and the inferior pulmonary vein from top-to-bottom. Each part received two scores that indicated the extent and nature of the lung lesions. The extent of involvement was graded as follows: 0 for no involvement;1 for 1%–24%; 2 for 25%–49%; 3 for 50%–74%; and 4 for ≥75%. The nature of lesions were graded as follows: 1 for normal lung without abnormal changes; 2 for at least 75% GGOs or crazy-paving pattern; 3 for the simultaneous appearance of GGOs/crazy-paving pattern and consolidation, and each contributed <75% of the total; 4 for 75% or more consolidation. The above two scores (the extent and the nature) were multiplied and totaled to achieve a final score for all six parts.
2.4. Statistical analysis
Frequencies and percentages were used to describe categorical variables. Clinical, laboratory results, CT findings, and CT scores were compared between the severe and non-severe types with a χ2 test or Fisher’s exact test. A two-sided α < 0.05 was considered a statistically significant difference. The efficiency of the CT score predicting the risk of severity was assessed using receiver operator characteristic (ROC) curve analysis.
To identify the independent risk factors, univariable and multivariable logistic regression analyses were utilized. The initial selection of all data was performed using univariate logistic regression analysis. Variables with P-values < 0.05 were incorporated into the multivariable logistic regression analysis. The final features in the multivariable logistic regression analysis were selected as risk factors with a P < 0.05.
The collected risk factors were used to build a nomogram model in R (version 3.6.3) along with the rms statistical tools. The model performance was then verified using a calibration curve and decision curve (DCA).
3. RESULTS
3.1. Clinical and laboratory findings
A total of 346 patients in all were included in our study. Table 1 displays the demographic and clinical factors. The non-severe and severe cohorts consisted of 300 patients with a median age of 35 years (age range, 4-78 years) and 46 patients with a median age of 58 years (age range, 34-89 years), respectively. Patients with a severe COVID-19 infection had a higher median age. The gender and cigarette smoking histories of the two cohorts were comparable. Patients in the severe cohort were more likely to experience cough, fever, and dyspnea than patients in the non-severe cohort (78.26% vs. 16.33%, 71.74% vs. 21.67, and 13.04% vs. 3.67%, respectively). The severe cohort had a significantly higher incidence of co-morbidities (hypertension [34.78% vs. 6.0%], diabetes [17.39% vs. 3.33%], coronary heart disease [17.39% vs. 0.67%], and surgical history [19.57% vs. 1.0%]) than the non-severe cohort.
Clinical characteristics of patients with COVID-19.
Characteristic | Non-severe NO. (%) | Severe NO. (%) | P |
Age, Y | 35 (4~78) | 58 (34~89) | <0.01 |
Gender, | 0.212 | ||
Female | 78 (26.0) | 16 (34.78) | |
Male | 222 (74.0) | 30 (65.22) | |
Smoking history | 0.811 | ||
Yes | 67 (22.33) | 11 (13.04) | |
No | 233 (77.67) | 35 (86.96) | |
Fever | <0.01 | ||
Yes | 49 (16.33) | 36 (78.26) | |
No | 251 (83.67) | 10 (21.74) | |
Cough | <0.01 | ||
Yes | 65 (21.67) | 33 (71.74) | |
No | 235 (78.33) | 13 (28.26) | |
Dyspnea | 0.006 | ||
Yes | 11 (3.67) | 6 (13.04) | |
No | 289 (96.33) | 40 (86.96) | |
Chest pain | 0.656 | ||
Yes | 4 (1.33) | 1 (2.17) | |
No | 296 (98.67) | 45 (97.83) | |
Headache | 0.947 | ||
Yes | 7 (2.33) | 1 (2.17) | |
No | 293 (97.67) | 45 (97.83) | |
Anorexia | 0.165 | ||
Yes | 8 (2.67) | 3 (6.52) | |
No | 292 (97.33) | 43 (93.48) | |
Fatigue | 0.395 | ||
Yes | 15 (5.0) | 1 (2.17) | |
No | 285 (95.0) | 45 (97.83) | |
Sore muscles | 0.324 | ||
Yes | 6 (2.0) | 2 (4.35) | |
No | 294 (98.0) | 44 (95.65) | |
Hypertension | <0.001 | ||
Yes | 18 (6.0) | 16 (34.78) | |
No | 282 (94) | 30 (65.22) | |
Diabetes | <0.001 | ||
Yes | 10 (3.33) | 8 (17.39) | |
No | 290 (96.67) | 38 (82.61) | |
Cardiovascular disease | <0.001 | ||
Yes | 2 (0.67) | 8 (17.39) | |
No | 298 (99.33) | 38 (82.61) | |
COPD | |||
Yes | 3 (1.0) | 1 (2.17) | 0.488 |
No | 297 (99.0) | 45 (97.83) | |
History of surgery | <0.001 | ||
Yes | 3 (1.0) | 9 (19.57) | |
No | 297 (99.0) | 37 (80.43) |
The laboratory findings are shown in Table 2 . Seventeen characteristics were evaluated with significant differences between the two cohorts.
Laboratory findings of patients with COVID-19.
Characteristic | Non-severe | Severe | P |
---|---|---|---|
LDH U/L | 167.5 (95~374) | 266 (145~671) | <0.001 |
CK | 80 (16~614) | 100.5 (27~1360) | 0.038 |
CK-MB | 10 (3~43) | 13 (2~33) | <0.001 |
HBDH | 129 (80~311) | 189.5 (75~502) | <0.001 |
ALT | 26 (6~407) | 27.5 (4~153) | 0.56 |
AST | 21 (10~184) | 33 (13~126) | <0.001 |
GGT | 22 (7~213) | 24.5 (12~148) | 0.031 |
ALP | 66 (15~278) | 67 (30~175) | 0.429 |
TBIL | 9.65 (1.4~107) | 7.75 (2~28.8) | 0.182 |
DBIL | 0.47 (0~86) | 0.73 (0.06~9.6) | 0.024 |
BUN | 3.88 (1.09~7.79) | 4.56 (2.05~25.69) | 0.006 |
Creatinine | 67 (27~390) | 67.75 (32.8~969) | 0.395 |
Glucose | 5.19 (2.61~24.79) | 7.23 (4.32~1057) | <0.001 |
WBC 109 | 6.31 (2.14~17.84) | 5.47 (1.84~14.84) | 0.125 |
Neutrophil count 109 | 3.68 (0.65~323) | 3.8 (0.6~12.7) | 0.262 |
Lymphocyte count 109 | 1.81 (0.44~6.24) | 0.74 (0.2~4.21) | <0.001 |
Monocyte Count | 0.46 (0.11~7.7) | 0.41 (0.12~325) | 0.150 |
PLT | 225 (1.66~502) | 148.5 (69.5~380) | <0.001 |
RBC | 4.91 (1.52~2089) | 4.6 (2.09~156) | 0.003 |
Hb | 147 (12.5~184) | 129 (60~210.18) | <0.001 |
CRP | 0.8 (0.5~129.55) | 0.5 (174.17) | <0.001 |
PaO2 | 87.85 (30.2~211.5) | 83.6 (36.5~178.6) | 0.157 |
PCO2 | 44.5 (31.1~71.2) | 40.1 (1.1~54.7) | <0.001 |
D-dimer ug/ml | 0.64 (0.28~6.43) | 1.07 (0.32~21.11) | <0.001 |
APTT | 28.7 (19.8~50.2) | 28.95 (20.6~74.4) | 0.231 |
FDP | 1.9 (0~19.5) | 3.25 (1.6~42.8) | <0.001 |
PT | 1.3 (13~18.7) | 13 (11.6~99.3) | 0.134 |
Abbreviations: LDH: lactate dehydrogenase; CK: creatine kinase; CK-MB: creatine kinase-MB; HBDH: hydroxybutyrate dehydrogenase; ALT: alanine aminotransferase; AST: aspartate aminotransferase; GGT: gamma-glutamyl transpeptidase; ALP: alkaline phosphatase; TBIL: total bilirubin; DBIL: direct bilirubin; BUN: blood urea nitrogen; PLT: platelets; Hb: hemoglobin; CRP: C-reactive protein; APTT: activated partial thromboplastin time; FDP: fibrinogen degradation products; PT: prothrombin time.
3.2. CT findings and CT scores
Table 3 lists the CT findings and CT scores. Patients with a severe COVID-19 infection had a higher incidence of GGOs (P<0.001), consolidation (P<0.001), reticular opacities (P<0.001), a crazy-paving pattern (P=0.012), interlobular septal thickening (P<0.001), bronchial wall thickening (P=0.006), and air bronchograms (P<0.001). Furthermore, patients in the severe cohort had a higher incidence of lymphadenopathy and pleural effusions (P<0.001 for both) than patients in the non-severe cohort.
CT findings and CT scores of patients with COVID-19.
Characteristic | Non-severe NO. (%) | Severe NO. (%) | P |
Ground-glass opacity (GGO) | <0.001 | ||
Yes | 168 (56.00) | 45 (97.83) | |
No | 132 (44.00) | 1 (2.17) | |
Consolidation | <0.001 | ||
Yes | 15 (5.00) | 17 (36.96) | |
No | 285 (95.00) | 29 (63.04) | |
Reticular pattern | <0.001 | ||
Yes | 102 (34.00) | 40 (86.96) | |
No | 198 (66.00) | 6 (13.04) | |
Nodules | 0.397 | ||
Yes | 76 (25.33) | 9 (19.57) | |
No | 224 (74.67) | 37 (80.43) | |
Crazy-paving pattern | 0.012 | ||
Yes | 6 (2.00) | 4 (8.70) | |
No | 294 (98.00) | 42 (91.30) | |
Septal thickening | 0.001 | ||
Yes | 16 (5.33) | 9 (19.57) | |
No | 284 (94.67) | 37 (80.43) | |
Halo sign | 0.938 | ||
Yes | 6 (2.00) | 1 (2.17) | |
No | 294 (98.00) | 45 (97.83) | |
Bronchial wall thickening | 0.006 | ||
Yes | 1 (0.33) | 2 (4.35) | |
No | 299 (99.67) | 44 (95.65) | |
Traction bronchiectasis | 0.695 | ||
Yes | 1 (0.33) | 0 (0) | |
No | 299 (99.67) | 46 (100) | |
Air bronchogram | <0.001 | ||
Yes | 3 (1.00) | 10 (21.74) | |
No | 297 (99.00) | 36 (78.26) | |
Pleural thickening | <0.001 | ||
Yes | 24 (8.00) | 13 (28.26) | |
No | 276 (92.00) | 33 (71.74) | |
Pleural effusion | <0.001 | ||
Yes | 6 (2.00) | 13 (28.26) | |
No | 294 (98.00) | 33 (71.74) | |
Lymphadenopathy | 0.695 | ||
Yes | 1 (0.33) | 0 (0) | |
No | 299 (99.67) | 46 (100) | |
CT score, median (range) | 2 (0~34) | 20 (2~51) | <0.001 |
The median CT score was 21.67±11.58 in the severe cohort and 4.27±6.02 in the non-severe cohort (P<0.001). Analysis of the ROC curve yielded an area under the ROC curve (AUC) for the CT score of 0.933%. (95% CI, 0.901–0.965; P<0.001), and a Youden index of 0.737, with 6.5 serving as the ideal cut-off value for identifying a severe COVID-19 infection (sensitivity, 95.70%; specificity, 78%; Figure 1 ).
3.3. Nomogram construction and validation
The clinical and laboratory characteristics combined with CT scores were included in the univariable analysis, and a total of 26 features were included in the multivariable logistic regression analysis. Five features, including CT score, age, lactic dehydrogenase (LDH) and hydroxybutyrate dehydrogenase (HBDH) levels, and hypertension were extracted as risk factors for severe COVID-19 infections. Based on these 5 risk factors, a nomogram for predicting the severity of a COVID-19 infection was created ( Figure 2 ).
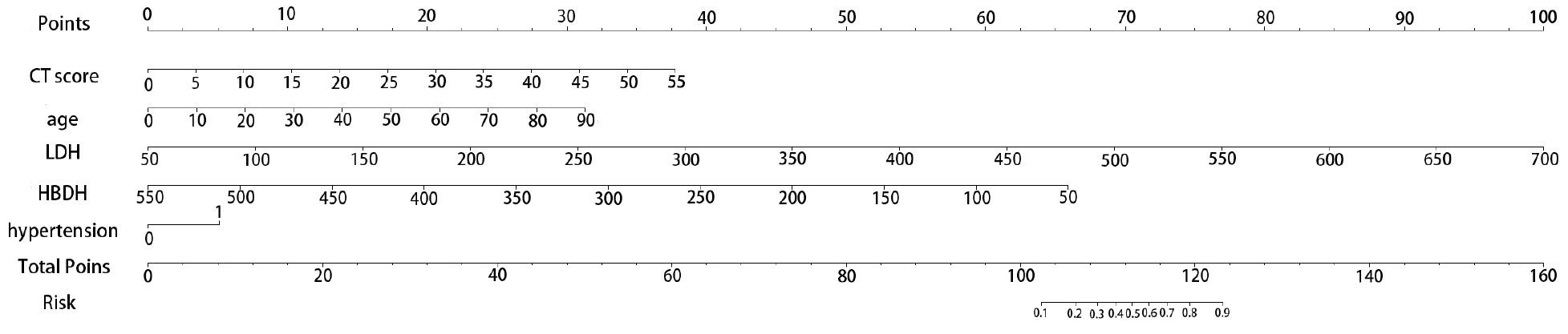
The nomogram for predicting severe COVID-19.
Each of the variables can be located on the corresponding variable axis. The point of each variable was determined by vertically referring to the top point line. By summing up the total points of each corresponding variable, the total point was calculated, and the risk of severe COVID-19 was determined by reading against the risk axis.
We verified the model performance using a calibration curve and DCA. As shown in Figure 3 , the nomogram demonstrated excellent performance.
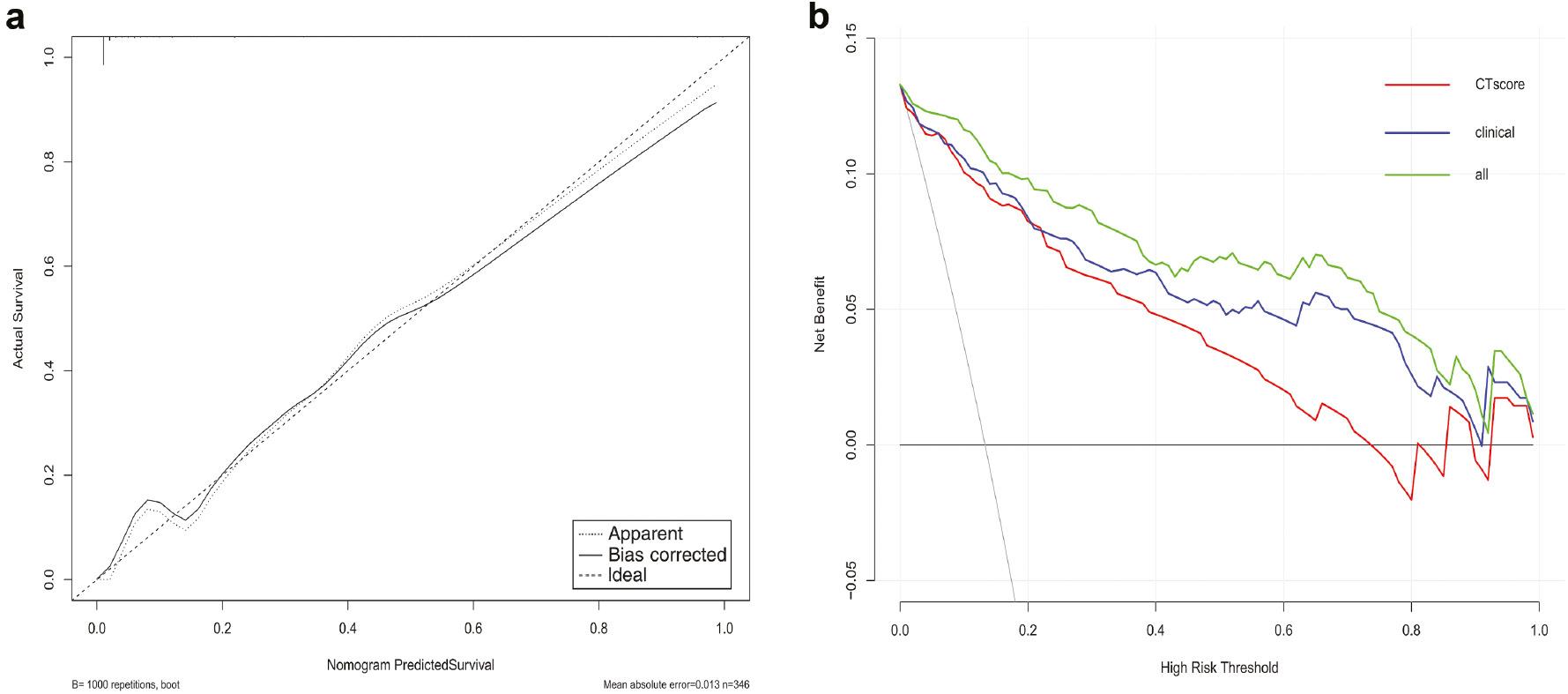
The calibration curves and DCA of the nomogram in predicting severe COVID-19.
The calibration curves of the nomogram (a), the model predicted severe COVID-19 was plotted on the x-axis, and the actual disease progression probability was plotted on the y-axis. DCA of the nomogram (b), compares the net benefits of three models in predicting severe COVID-19: The y-axis represents the net benefit. The x-axis denotes the threshold probability. The red line represents the model based on the CT score alone, the blue line represents the model based on the clinical characteristics alone, and the green line represents the model based on clinical characteristics and CT score. DCA, decision curves analysis.
4. DISCUSSION
COVID-19 is continuing to have an impact on a worldwide scale. Severe and critical cases have created a huge medical burden to society, especially in countries with large populations or limited medical resources. An easy and feasible clinical model predicting the severity of COVID-19 infections in a timely fashion is vital for optimal management of potentially severe cases and to achieve better clinical outcomes. Our study developed a nomogram derived from the baseline clinical and laboratory findings, CT characteristics, and CT score. Five variables were identified as risk factors for severe COVID-19 infections, including the CT score, age, LDH and HBDH levels, and hypertension. These factors can be assessed easily and in a timely fashion. In addition, the correlation between the CT score and patients with severe COVID-19 infections was investigated. The nomogram described herein, which was based on a combination of clinical characteristics and the CT score, demonstrated good performance in the calibration curve and DCA. Indeed, the model showed better prognostic ability compared to the clinical characteristics or CT score model alone.
In the current study age was a significant risk factor for severe COVID-19. Previous studies [24–26] concluded that elderly patients were at increased risk to deteriorate during the course of a COVID-19 infection. A model created by age and laboratory findings showed that patients > 65 years of age had a substantially increased probability of developing a severe COVID-19 infection than patients < 65 years of age [27]. Earlier nomograms with clinical characteristics also demonstrated that age was a significant variable in severe cases [1, 17]. It was shown that age and COVID-19 mortality were associated [28, 29], and a research involving COVID-19 patients from various nations reported the average age of the majority of COVID-19 fatalities was > 60 years. [30]. Angiotensin-converting enzyme 2 (ACE2) receptor is the gateway to human cells for SARS-CoV-2, and in patients with a severe COVID-19 infection, ACE2 expression has been shown to increase with age [31]. This finding might explain why the elderly are at highest risk for a severe COVID-19 infection.
In the present study the CT score served as an independent risk indicator for severe COVID-19 infections. Analysis of the ROC curve revealed an AUC for the CT score of 0.933% (95% CI, 0.901–0.965; P=0.000) with a 6.5 cut-off value (sensitivity, 95.70%; specificity, 78%). The CT score was the most significant variable based on multivariable logistic regression analysis (P=0.009). The relationship between the CT score and a severe COVID-19 infection has been supported by numerous investigators. Francone et al. [32] used a semi-quantitative method based on CT scores (only assessing the extent of lung lesions) in a study involving 1274 patients, which indicated that the CT score was related to age, inflammatory features, and severity. Likewise, Yang et al. [33] and Hajiahmadi et al. [34] adopted a similar scoring system and reported AUCs of 0.892 (95% CI, 0.814-0.944) and 0.764 (95% CI, 0.682-0.847), respectively. The current study used a quantitative CT scoring system that assessed the extent and nature of the lung lesions. The AUC reached 0.933 (95% CI, 0.901–0.965), demonstrating a superior power of predicting severity. Additional studies [35–37] reported nomograms that combined CT and clinical characteristics. The models outperformed the model based on clinical factors or CT features alone; however, most focused on CT radiomics and deep learning techniques. Artificial intelligence is not a widely used technique among the hospitals in China, while the CT scoring method can be easily adopted clinically.
Co-morbidities have been reported to be correlated with severe COVID-19 infections [2, 38]. Our study identified hypertension as a risk factor for poor prognosis. Previous studies [18, 39] extracted hypertension as an important variable in the development of clinical models, which is consistent with our study.
Laboratory parameters, including the LDH and HBDH levels, were shown to be independent risk factors for severe COVID-19 infections based on multivariate logistic regression analysis. The LDH level has an important role in predicting severe COVID-19 infections [4, 40, 41]. Our study also showed the HBDH level to be a risk factor. Most severe and critical patients had multiple organ dysfunction syndromes, which may be attributed to the widespread distribution of ACE2 in multiple organs, including the heart [42]. This finding explains why there are COVID-19 patients developing myocarditis in Chengdu city.
Our research has a few shortcomings and restrictions. First, there was a limited number of people in the sample. Second, this research only focused on a single site and used retrospective data. Finally, the study period was short. A larger sample and multi-centered study should be conducted to confirm our model in the future.
5. CONCLUSION
CT score, age, hypertension, and LDH and HBDH levels were shown to be risk factors to predict severe COVID-19 infections. We constructed a straightforward nomogram that has excellent predictive power to forecast poor prognosis in patients with severe COVID-19 infections, which could give early advice in medical management and increase the likelihood of a positive outcome for patients in Chengdu, China.