Introduction
Despite substantial advances in drug therapy, surgery, and cardiac rehabilitation, the incidence and mortality rates of acute myocardial infarction (MI) remain high [1]. These persistently high rates might be largely attributable to modern lifestyle factors – such as smoking, obesity from consumption of high-calorie diets, and hypertension induced by high sodium consumption – which obviously elevate the risk of MI. Early identification of individuals at high risk of MI is crucial in enabling timely therapeutic interventions that can substantially decrease mortality [2]. However, although these risk factors do provide some predictive power, they alone are insufficient for early diagnosis of MI [3].
With advancements in microarray and next-generation sequencing technologies, identifying disease mechanisms has become increasingly effective and precise. Genome-wide transcriptome analyses, such as those provided by microarrays, offer gene expression profiles that are instrumental in pinpointing key genes and screening for biomarkers, thereby aiding in the development of new therapeutic targets. For instance, a thorough comprehensive molecular analysis by Salwa has indicated that hypoxia-induced miR-137, a marker of cell apoptosis, and inflammation-induced miR-106b, a marker of inflammation, have potential for clinical use as diagnostic biomarkers [4].
Furthermore, the introduction of weighted gene co-expression network analysis (WGCNA) for high-throughput data has revolutionized the study of genes associated with clinical disease features [5]. WGCNA is an R package algorithm designed to extract module information from gene chip expression data [6]. In gene co-expression networks, genes that exhibit similar expression patterns within the same sample are grouped into the same network or module, according to the correlation coefficients between their gene expression.
Immune processes are fundamental to the development of MI [7]. In this study, we identified genes characteristic of patients with MI by using advanced bioinformatics tools, including Cytoscape’s cytoHubba plugin and WGCNA. Our analysis focused on co-expression modules reflecting changes in the immune cells of patients with MI, thereby enabling examination of the relationship between MI-related gene modules and immune processes.
Furthermore, we validated the expression differences in core genes between infarcted and non-infarcted patients. We selected a key gene for preliminary exploration of its association with inflammatory responses and oxidative stress. The primary aim of this research was to identify core genes associated with MI and immune responses, to provide a scientific basis for uncovering potential regulatory targets and mechanisms. Ultimately, our findings may offer new insights into treatment strategies for MI.
Materials and Methods
Data Download and Pretreatment
The Gene Expression Omnibus (GEO) database collects and publicly shares high-throughput sequencing and microarray-based datasets. We searched the GEO database (https://www.ncbi.nlm.nih.gov/geo/) for datasets consisting of patients with or without MI, and selected three datasets with microarray data: GSE29111, GSE66360, and GSE48060. In selecting these datasets, we used the following detailed criteria to ensure the relevance and quality of the data. All three datasets contained MI samples, and the microarray platform was GPL570 ([HG-U133_Plus_2] Affymetrix Human Genome U133 Plus 2.0 Array). This consistency avoided potential technical bias due to platform differences, thereby facilitating data integration and cross-dataset comparison. These datasets are publicly available, and the data quality was preliminarily evaluated and found to meet our analytical requirements, including good baseline information and complete data annotations. GSE29111 included 52 samples (36 MI samples and 16 controls); GSE66360 contained 99 samples (49 MI samples and 50 controls), and GSE48060 contained 52 samples (31 MI samples and 21 controls), as shown in Table 1. We combined GSE29111 and GSE66360 into one dataset and used the ComBat function of the “SVA” package 3.5 [8] based on R 4.3.3 to remove batch effects; these data served as the training set. GSE48060 was used as the external validation dataset. Dataset and platform details are listed in Table 1. Probe IDs were converted to gene symbols according to the annotation file of the platform, excluding probes that did not correspond to gene symbols. For multiple probe loci corresponding to the same gene symbol, the average of all probe expression was taken, and duplicate probes were removed.
Differential Gene Expression
Before the differential gene expression analysis, we performed preprocessing. If a gene corresponded to multiple probe loci, we averaged the values of the probe loci. Probe IDs were converted to gene symbols according to the annotation file of the platform, and probes that did not correspond to gene symbols were excluded. In addition, the two datasets were merged into one dataset, and batch correction was performed with the ComBat function of the “SVA” package in R software [8]. We identified differentially expressed genes between patients with MI and controls with the “limma” package 3.58.1 [9]. The screening thresholds for differentially expressed genes were P < 0.05 and |log2 fold change| > 1.
Functional Enrichment Analysis
Gene Ontology (GO) [10] is a frequently used functional enrichment analysis that includes molecular function (MF), biological process (BP), and cellular composition (CC) categories. The Kyoto Encyclopedia of Genes and Genomes (KEGG) [11] is a widely used database that stores large amounts of pathway data on genomes, biological pathways, diseases, chemicals, and drugs. The clusterProfiler package 4.10.1 [12], ggplot2 package 3.5.1 [13], and circlize package 0.4.16 [14] were used to analyze differentially expressed genes, and to perform GO and KEGG enrichment and visualization. A threshold of P < 0.05 was considered to indicate significantly enriched gene sets.
PPI Analysis
To identify proteins that interact directly or indirectly with differentially expressed genes, we used the STRING database [15] to construct a protein-protein interaction (PPI) network. The confidence score used to construct the network was set to 0.9. Cytoscape 3.8.2 was used to visualize the network, and the CytoHubba plugin was used to identify core genes.
Immune Gene Set Enrichment Analysis
To determine the relationship between MI-related genes and immunity, we used the immunesigdb.v7.4.symbols.gmt gene set downloaded from the Molecular Signature Database [16] as the reference gene set and the R software “clusterProfiler” package of R software for enrichment analysis. The gene set was screened with |NES| > 2 and P < 0.05 as the criteria.
WGCNA
The co-expression network of infarct-related genes was constructed with the “WGCNA” package 1.72.5 in R software [6]. First, we clustered the samples and removed the outlier samples. Then we selected the best power value of the balanced fit index and average connectivity, calculated the adjacency matrix and distance matrix, constructed a hierarchical clustering tree, and used a dynamic shearing algorithm to construct the co-expression network. The minimum number of genes per module was set to 30, and the shear height was set to 0.4 for module clustering. The correlation between each module and grouping was calculated, and P < 0.05 was considered significant. Module membership (MM) and gene significance (GS) were calculated, and genes in the modules were screened for MM > 0.8 and GS > 0.5.
Diagnostic Value and Validation of Core Genes
The genes screened with the weighted gene co-expression network and the PPI network were intersected to obtain the final core genes. The receiver operating characteristic (ROC) curves were constructed with the “pROC” package 1.18.5 in R software [17], with expression data from 116 patients with MI and 87 controls, and the area under the curve for the core genes was calculated. We also used the “ggpubr” package 0.6.0 to construct violin plots of patients with MI and controls, to reflect the diagnostic efficiency of the genes and the expression differences between groups. In addition, in the GSE48060 dataset, we verified that the value of P < 0.05 was considered statistically significant.
Enrichment Analysis of the Immune Cell Gene Set
The infiltration of immune cells was evaluated with the “GSEAbase” package 1.64.0 [18] in R software, the “GSVA” package 1.48.3 [19], and TILS.txt (Gene Signatures of 28 tumor-infiltrating lys) downloaded from TISSIDB [20] (http://cis.hku.hk/TISIDB/download.php). We obtained scores associated with immune cell infiltration, and used the “pheatmap” package 1.0.12 and “vioplot” package 0.5.0 [21] to identify differences between 28 immune cell types among patients with MI and controls.
Correlation Analysis between Core Genes and Immune Cells
We performed Spearman rank correlation analysis of core genes and immune cells to further explore the immune mechanisms involved in the development of MI. The immune cell enrichment score across each sample was calculated with the ssgsea method in the GSVA package, and correlation analysis was applied between the immune cell enrichment score and core gene expression. The results were visualized with the “ggplot2” package.
Preliminary Validation of Core Genes (RT-PCR)
From blood samples from patients at the Huai’an First People’s Hospital, we isolated total RNA with TRIzol reagent (Nanjing Punon Biotechnology Co., Ltd.), reverse transcribed the RNA to cDNA with a TRUE script RT kit (+gDNA Eraser) (Nanjing Punon Biotechnology Co., Ltd.), and analyzed the RNA with 2× SYBR Green qPCR Mix (with 100× ROX) (Nanjing Punoen Biotechnology Co., Ltd.) in RT-PCR with a Roche 480 fluorescence quantitative PCR instrument. GAPDH was used as an internal control, and the expression levels of genes were assessed with the 2-△△Ct method. The primers used for RT-PCR were provided by Invitrogen (Table 2).
Cell Culture
Cardiac H9c2 cells (Cell Bank of Chinese Academy of Sciences) were cultured in DMEM (Gibco) containing 10% FBS (Gibco) and 100 μg/mL penicillin, then resuspended in new culture flasks, and incubated at 37 °C and 5% CO2. The culture medium was changed regularly.
Cell Transfection
The cell culture medium was changed to Opti-MEM (Gibco), and cells were incubated for at least 12 hours before the start of transfection. The siRNA (negative control group) and Bcl6-siRNA (Santa Cruz, experimental group) were separately added to Opti-MEM containing Lipofectamine 2000 (Thermo Fisher Scientific), mixed, and allowed to stand for 20 min at room temperature. The mixture was then added into H9c2 cell culture plates, which were placed in an incubator (37 °C, 5% CO2) for 24 hours to complete transfection.
H9c2 Cell Hypoxia Model Construction
The transfected mouse cardiomyocyte cell line H9c2 was purchased from the Cell Bank of the Chinese Academy of Sciences and used for subsequent in vitro experiments. The transfected cells were incubated in a hypoxic chamber (37 °C, 95% N2, and 5% CO2) and normoxic chamber (37 °C and 5% CO2) for 24 hours, then used for subsequent experiments.
Detection of Cell Viability
H9c2 cells transfected and cultured under normoxic or hypoxic conditions were seeded in 96-well plates. MTT solution (Shanghai Hengfei Biotechnology Co., Ltd.) was added to each well and incubated for 4 hours at 37 °C. The absorbance at 570 nm was measured with a microplate reader. The cell viability of each group was calculated with respect to that of the control group, which was set as 100%.
Detection of Inflammatory Factors (RT-PCR)
The mRNA expression of the inflammatory factors TNF-a, IL-1, and IL-6 among groups was detected with RT-PCR. Total RNA from H9c2 cells transfected and cultured under hypoxic or normoxic conditions was isolated with TRIzol reagent (Sigma), and total RNA from H9c2 cells cultured in hypoxic or normoxic conditions was reverse transcribed to cDNA with a PrimeSeriptTM 1st Strand cDNA Synthesis Kit (Takara). RT-PCR was performed with Realtime PCR Master Mix kit (Toyobo) and a Roche 480 fluorescence quantitative PCR instrument. GAPDH was used as an internal control, and the expression levels of genes were assessed with the 2-△△Ct method. The primers used to detect the mRNA expression of inflammatory factors were provided by Shanghai Bioengineering Company and are shown in Table 3.
TNF-α, IL-1, IL-6, and GAPDH Primer Sequences.
Name | Primer sequences | |
---|---|---|
Forward primer (5′-3′) | Reverse primer (5′-3′) | |
TFN-α | AGCATGATCCGAGATGTGGAA | TAGACAGAAG AGCGTGGTGGC |
IL-1 | GGGATGATGACGACCTGCTAG | ACCACTTGTTGGCTTATGTTCTG |
IL-6 | GTTGCCTTCTTGGGACTGATG | ATACTGGTCTGTTGTGGGTGGT |
GAPDH | GACATGCCGCCTGGAGAAAC | AGCCCAGGATGCCCTTTAGT |
Assays of SOD Activity and MDA Content
The levels of malondialdehyde (MDA) and superoxide dismutase (SOD) were measured through ELISA with an MDA assay kit (Nanjing Jiancheng Institute of Biological Engineering) and SOD assay kit (Nanjing Jiancheng Institute of Biological Engineering). Transfected H9c2 cells under hypoxic or normoxic culture were ultrasonicated, and the cell lysate supernatants were collected by centrifugation. SOD activity and MDA level in H9c2 cells were detected and calculated according to the kit manufacturer’s instructions, and measured with an enzyme marker.
NADPH Oxidase Subunit p67 and gp91 Expression Assay (RT-PCR)
The mRNA expression of the NADPH oxidase subunits p67 and gp91 among groups was detected with RT-PCR. Total RNA from H9c2 cells transfected and cultured under hypoxic or normoxic conditions was isolated with TRIzol reagent (Sigma), and total RNA from H9c2 cells cultured under hypoxic or normoxic conditions was isolated and reverse transcribed to cDNA with a PrimeSeriptTM 1st Strand cDNA Synthesis Kit (Takara). RT-PCR was performed with a Realtime PCR Master Mix kit (Toyobo) with a Roche 480 fluorescence quantitative PCR instrument. GAPDH was used as an internal control, and gene expression levels were assessed with the 2-△△Ct method. The primers used to detect the expression of NADPH oxidase subunits p67 and gp91 mRNA were provided by Shanghai Bioengineering Company (Table 4).
Results
Screening for Differentially Expressed Genes
Three GEO datasets (GSE29111, GSE66360, and GSE48060) were included in this study. The combined dataset contained 85 patients with MI and 66 controls. After removal of batch effects, analysis was performed with the “limma” package [9] in R software. The volcano plot and heat map of differentially expressed genes were plotted with the “ggplot2” package. In the volcano plot, red represents up-regulated genes, and green represents down-regulated genes; in the heat map, red represents up-regulated genes, and blue represents down-regulated genes. A total of 91 differentially expressed genes were obtained in the merged and calibrated matrix, including 88 up-regulated genes and three down-regulated genes. Up-regulated and down-regulated genes were significantly separated in the volcano plot (Figure 1A). The expression levels of differentially expressed genes in patients with MI and controls significantly differed, as indicated by the heat map (Figure 1B).
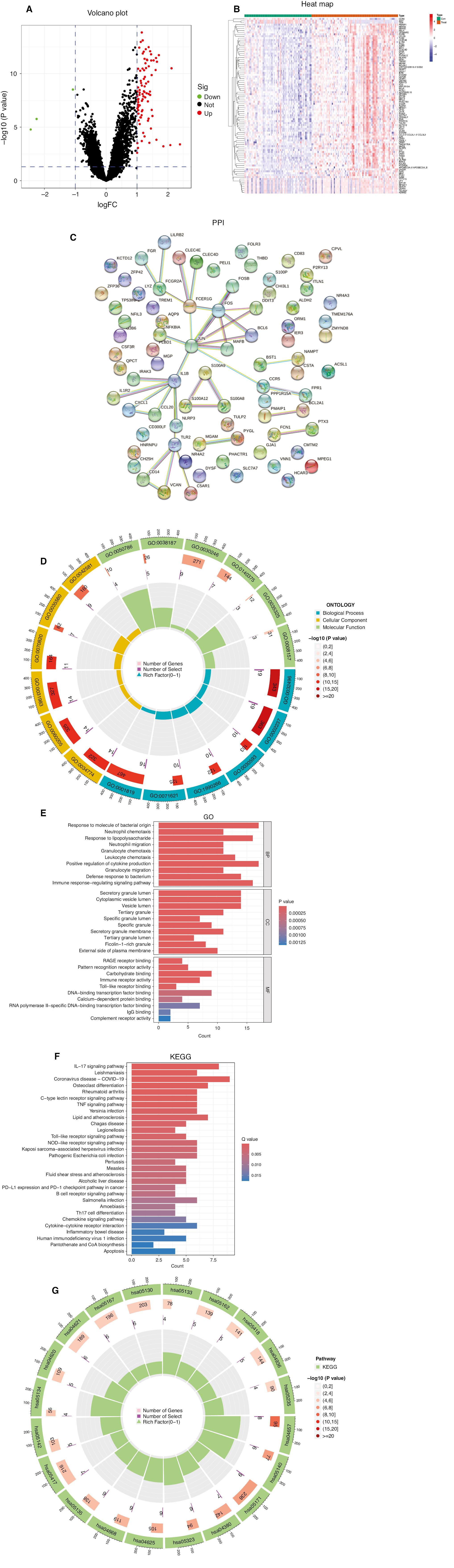
Difference Analysis, Protein-Protein Interaction Analysis, and KEGG/GO Enrichment Analysis.
(A) Difference analysis volcano plot. (B) Difference analysis heat map. (C) Protein-protein interaction network. (D) Gene Ontology (GO) term functional enrichment circle plots. The GO terms include biological process (BP), cellular component (CC), and molecular function (MF). (E) GO bar plots. (F) Kyoto Encyclopedia of Genes and Genomes (KEGG) bar plots. (G) KEGG circle plot.
Functional Enrichment
We performed GO and KEGG functional enrichment analyses to gain insight into the relevant functions of differentially expressed genes. GO contains three components: BP, CC, and MF. Figure 1D shows the top ten GO enrichment entries for each; the vertical coordinate shows the entry name, and the horizontal coordinate shows the number of enriched genes. The color represents the P-value, i.e., the degree of variation. Figure 1E shows the top six enriched entries in each section. The outermost circle is the entry number, the second circle corresponds to the total set of genes contained in the entry, and the color indicates the enrichment of differentially expressed genes in the entry. Darker color indicates more significant enrichment. The third circle indicates the number of differentially expressed genes enriched for each entry. The innermost circle indicates the proportion of differentially expressed genes enriched in that entry. The main GO biological processes included response to lipopolysaccharide, response to molecules, bacterial origin, neutrophil tropism, neutrophil migration, and granulocyte chemotaxis. The main cell composition terms were secretory granule lumen and cytoplasmic vesicle lumen. The main molecular functional processes were RAGE receptor binding, pattern recognition receptor activity, carbohydrate binding, and immunoreceptor activity. Figure 1F demonstrates the KEGG enrichment, which included primarily the IL-17 signaling pathway, C-type lectin receptor signaling pathway, and lipid and atherosclerosis-related pathways. Figure 1G shows the top entries in the KEGG pathway enrichment analysis. The outermost circle is the entry number, the second circle corresponds to the total set of genes contained in the entry, and the color indicates the enrichment of differentially expressed genes in the entry. Darker color indicates more significant enrichment.
Screening of Candidate Core Genes
We constructed a PPI network with the STRING database 12.0 [15]. The screened network is shown in Figure 4. We imported the network into Cytoscape software for visualization and used the CytoHubba plugin to identify core genes. We used five algorithms (MCC, DMNC, MNC, Clustering, and BottleNeck) to screen the candidate core genes separately, and determined the intersection of the first ten genes of each algorithm, thus yielding IL1B, S100A12, BCL6, and S100A8 (Table 5).
Screening of Candidate Core Genes.
Top ten genes obtained with various algorithms | Intersection | ||||
---|---|---|---|---|---|
MCC | DMNC | MNC | Clustering | BottleNeck | |
JUN | S100A12 | FOS | S100A12 | JUN | IL1B |
FOS | BCL6 | JUN | BCL6 | IL1B | S100A12 |
IL1B | S100A8 | S100A12 | S100A8 | FCER1G | BCL6 |
FCER1G | S100A9 | BCL6 | S100A9 | TLR2 | S100A8 |
TLR2 | TLR2 | S100A8 | CCL20 | FCGR2A | |
FCGR2A | CCL20 | S100A9 | CXCL1 | CCR5 | |
S100A12 | IL1B | TLR2 | CD14 | S100A12 | |
BCL6 | CXCL1 | CCL20 | VCAN | BCL6 | |
S100A8 | CD14 | IL1B | DDIT3 | S100A8 | |
S100A9 | VCAN | CXCL1 | FOSB | FOS |
WGCNA
The final 23,476 genes were obtained for WCGNA with the expression matrix after merging of GSE29111 with GSE66360, removal of batch effects, and pre-processing. Correlation analysis was performed on the samples, and no outlier samples were found; therefore, all samples were included in the analysis. The weighted gene co-expression network was constructed with the “WGCNA” package in R software. The best power value, as calculated with the “pickSoftThreshol” function, was 4 (Figure 2A, B). The power was used to construct a more advantageous scale-free network. The correlation matrix and adjacency matrix were subsequently calculated to construct a hierarchical clustering tree, and the dynamic shear tree algorithm was used to cluster modules. We finally obtained 12 modules (Figure 2E): the brown module (6685 genes), cyan module (4803 genes), dark-green module (90 genes), dark-grey module (67 genes), dark-orange module (49 genes), dark-red module (102 genes), dark-turquoise module (70 genes), grey60 module (644 genes), light-cyan module (2076 genes), light-yellow module (116 genes), midnight blue module (210 genes), and white module (44 genes). We also calculated the correlation of modules with the MI group and control group, and observed the highest correlation for the MEgrey60 module (Figure 2D), which showed a positive correlation of 0.52 with gene expression in patients with MI, at P < 0.05. MM and GS were calculated, and gene significance graphs were plotted for each module (Figure 2F). The highest gene significance was observed for the MEgrey60 module, in agreement with the previous results. We selected the genes in this module for subsequent analysis. The candidate core genes in the MEgrey60 module were screened with MM > 0.8 and GS > 0.5. A total of 13 genes were finally identified: ACSL1, BCL6, C5AR1, CLEC4E, CSF3R, IL1R2, IRAK3, LILRB2, NAMPT, QPCT, S100A12, S100A9, and TLR2.
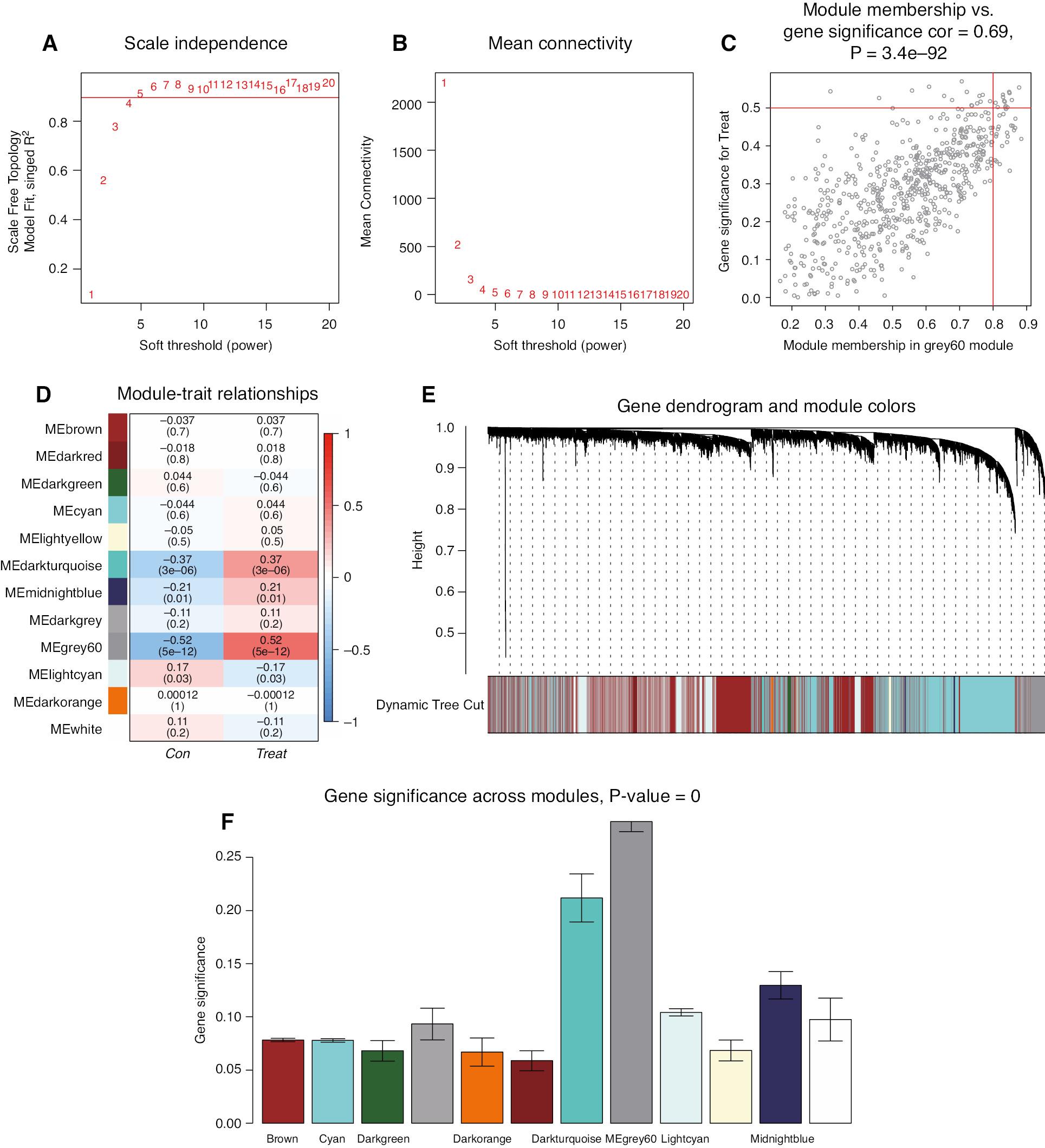
WGCNA Revealing Key Gene Modules Associated with MI.
(A, B) Calculation of the best power value. (C) Turquoise module membership and gene significance. (D) Heatmap of the correlation between module eigengenes and two groups. (E) Cluster dendrogram and color display of co-expression network modules. (F) Histogram of gene significance in various modules. The MEgrey60 module showed high gene significance across modules.
Validation of the Diagnostic Value of Core Genes
The PPI network constructed from differentially expressed genes with the Hubba plugin in Cytoscape identified a group of four candidate core genes: IL1B, S100A12, BCL6, and S100A8. The weighted gene co-expression network identified a group of 13 candidate core genes: ACSL1, BCL6, C5AR1, CLEC4E, CSF3R, IL1R2, IRAK3, LILRB2, NAMPT, QPCT, S100A12, S100A9, and TLR2. The two groups of candidate core genes were intersected to obtain the final core genes S100A12 and BCL6.
To validate the diagnostic ability of the two obtained core genes, we constructed gene expression box plots and ROC curves, and used AUC values to assess the diagnostic ability of differentiating patients with MI from controls. Figure 3A, B shows a significant difference between BCL6 and S100A12 in the MI and control groups in the training set (P < 0.001). Figure 3E, F shows AUCs of 0.809 (95% CI 0.733–0.874) and 0.837 (95% CI 0.771–0.896) for S100A12 and BCL6 in the training set. The diagnostic ability of core genes was also confirmed in the GSE48060 external validation set (Figure 3C, D, G, H), and the core genes demonstrated high diagnostic value.
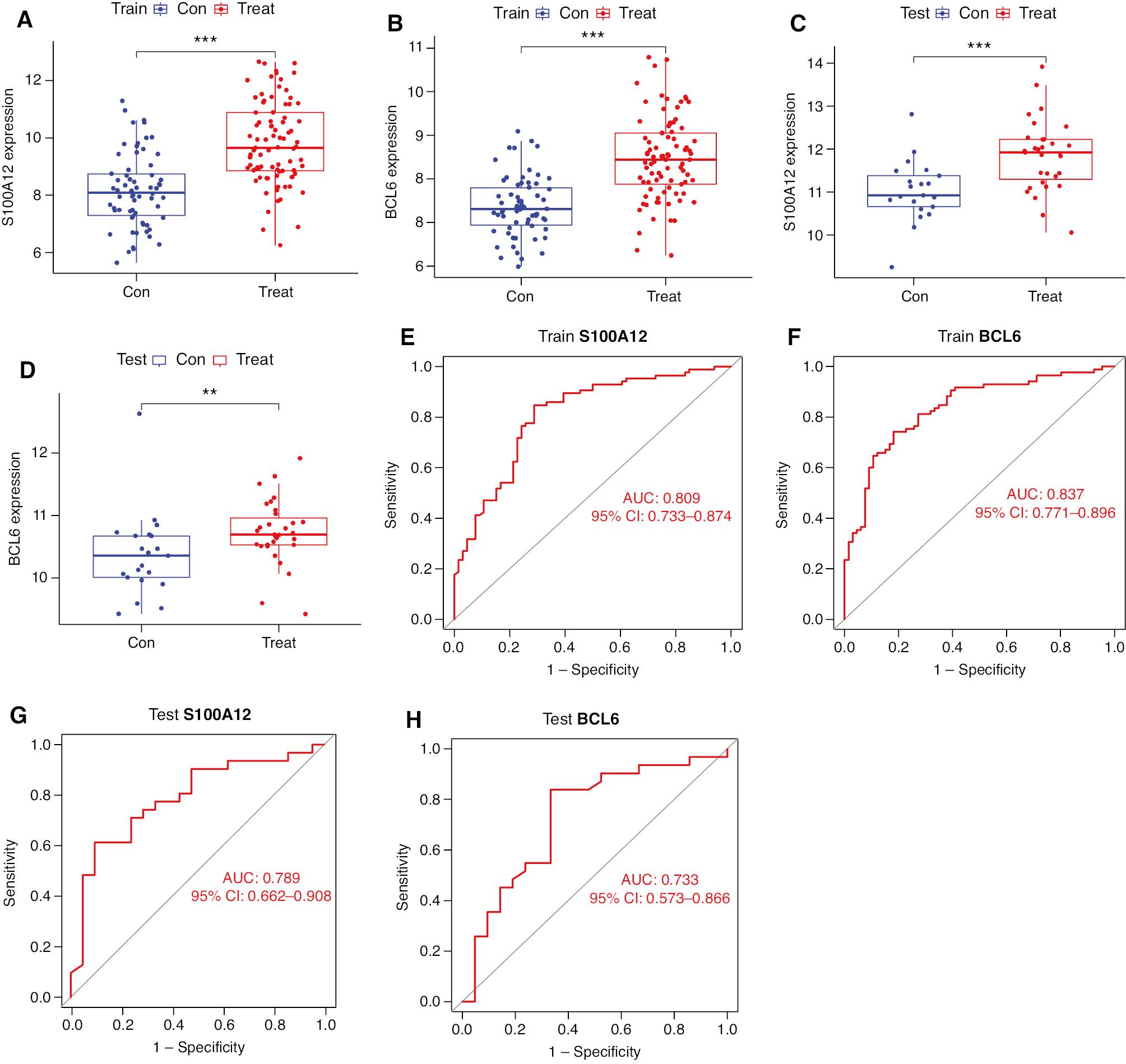
The Core Genes Validation Between Con and Treat Groups.
(A–D) Boxplots of the expression of the two core genes between the MI and negative control groups in the training set and validation set. (E–H) ROC curves of the two core genes in the training and validation set. **: P < 0.01; ***: P < 0.001.
GSEA of Immune Gene Sets
To gain insight into the molecular mechanisms of genes associated with MI development, we performed gene set enrichment analysis (GSEA) between the MI group and control group with the immunesigdb.v7.4.symbols.gmt gene set downloaded from the Molecular Signature Database [16] as a reference gene set. Genes associated with the occurrence of MI regulated more than 1000 immune features within the immune system. Figure 4A, B shows the top five entries enriched in the AMI group and control group. In patients with MI (Figure 4A), the B-cell to myeloid ratio, CD4 T-cell to myeloid ratio, and CD4 cell to peripheral blood mononuclear cell ratio were diminished. In controls (Figure 4B), the CD4 T-cell to B-cell ratio, CD4 T-cell to myeloid ratio, and CD4 cell to peripheral blood mononuclear cell ratio were diminished, in agreement with the results obtained in the MI group.
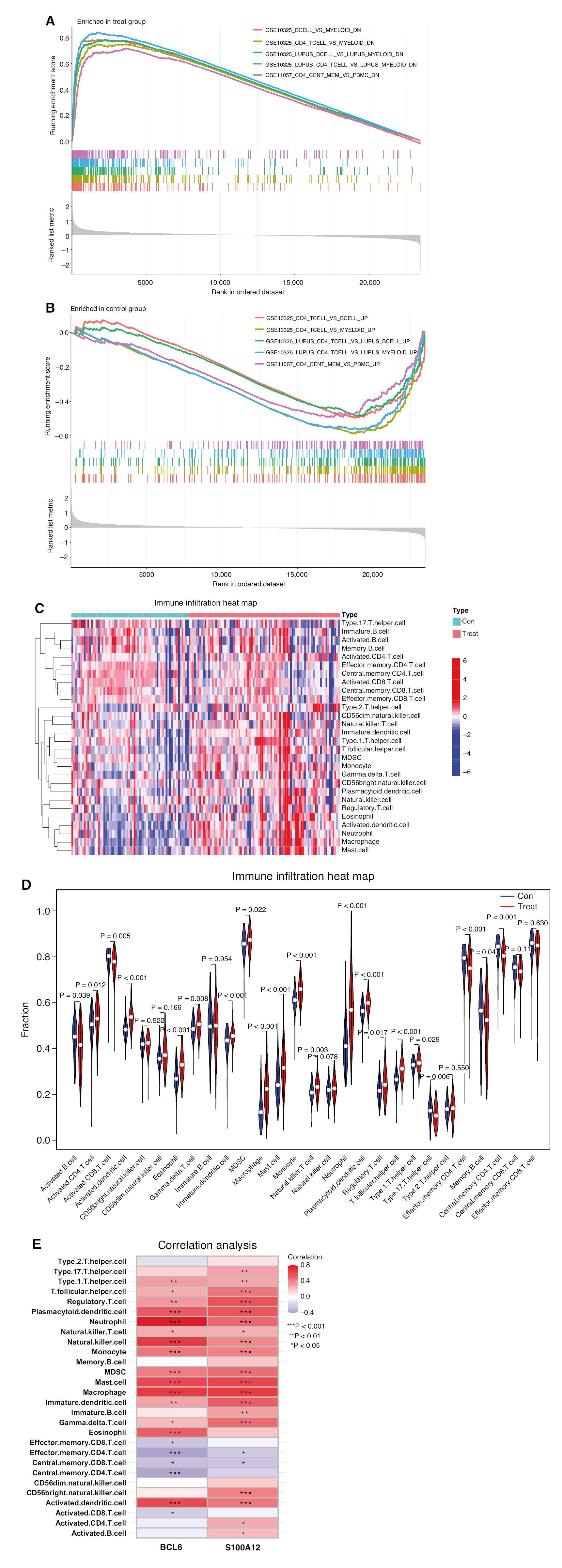
GSEA and Correlation Analysis of Infiltrating Immune Cells.
(A) GSEA of the MI and negative control groups. (B) GSEA of the negative control groups. (C) Immune cell infiltration heat map of the MI and negative control groups. (D) Differences in immune cell infiltration between the MI and negative control groups. (E) Correlation analysis between immune cells and two core genes.
Immune Cell Infiltration
To further clarify the specific immune cell differences between the MI and control groups, we also explored immune cell composition and visualized the significant difference in the levels of immune cell infiltration between the MI group and the control group (Figure 4C). We also compared the differential levels of immune cells between groups (Figure 4D) and observed that the MI group demonstrated lower levels of activated B cells (P = 0.039), activated CD8 T cells (P = 0.005), T helper 17 cells (P = 0.006), CD4 effector T cells (P < 0.01), effector memory B cells (P = 0.047), and central memory CD4 T cells (P < 0.01) than the control group. However, the MI group contained a higher proportion of activated CD4 T cells (P = 0.012), activated dendritic cells (P < 0.01), eosinophils (P < 0.01), γδ T cells (P = 0.008), immature dendritic cells (P < 0.01), myeloid-derived suppressor cells (P = 0.022), macrophages (P < 0.01), mast cells (P < 0.01), monocytes (P < 0.01), natural killer cells (P < 0.01), neutrophils (P < 0.01), plasmacytoid dendritic cells (P < 0.01), regulatory T cells (P = 0.017), and follicular helper T cells (P < 0.01).
Correlation Analysis between Core Genes and Immune Cells
We performed Spearman’s rank correlation analysis to assess the relationship between core genes and immune cells, which was considered statistically significant at P < 0.001 (Figure 4D). Bcl6 was positively correlated with plasmacytoid dendritic cells, neutrophils, natural killer cells, monocytes, myeloid-derived suppressor cells, mast cells, macrophages, eosinophils, and activated dendritic cells, and inversely correlated with effector memory CD4 T cells and central memory CD4 T cells. S100A12 was positively correlated with follicular helper T cells, regulatory T cells, plasmacytoid dendritic cells, neutrophils, natural killer cells, monocytes, myeloid-derived suppressor cells, mast cells, immature dendritic cells, γδ T cells, CD56 natural killer cells, and activated dendritic cells.
Preliminary Validation of Core Gene Expression
To initially validate our screened core genes, we detected the expression of S100A12 and BCL6 in blood samples from infarcted and non-infarcted patients by using RT-PCR. The results were consistent with those from our previous analysis: S100A12 (Figure 5A, P < 0.001) and BCL6 (Figure 5B, P < 0.05) expression was significantly higher in the AMI group than in the control group.
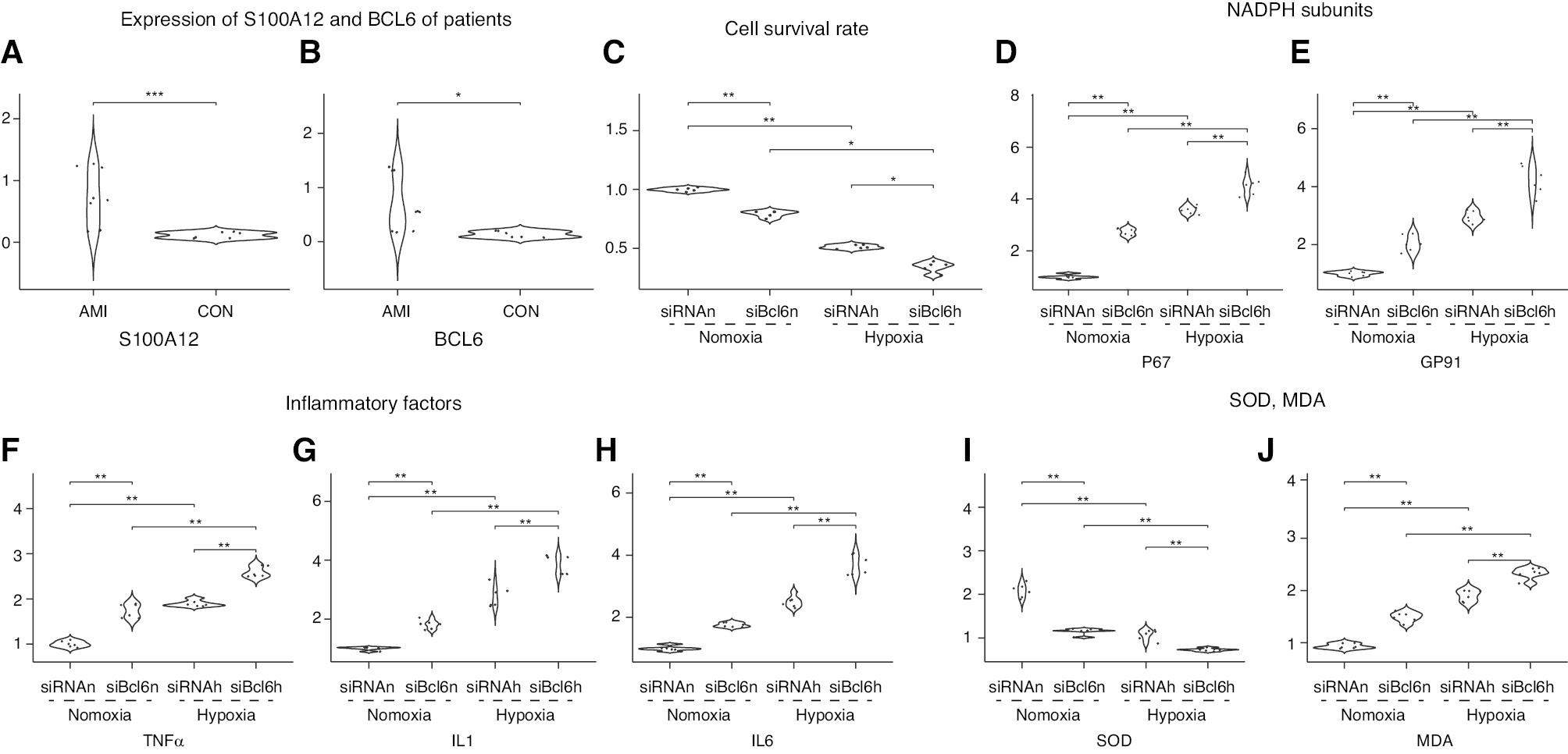
Differences among Groups.
(A, B) Expression differences in two core genes between the MI group and control group. (C) Differences in cell survival rates among four groups (siRNAn, siBcl6n, siRNAh, and siBcl6h). (D, E) Differences in the NADPH subunits P67 and GP91 among four groups (siRNAn, siBcl6n, siRNAh, and siBcl6h). (F-H) Differences in inflammatory factors among four groups (siRNAn, siBcl6n, siRNAh, and siBcl6h). (I, J) Differences in SOD activity and MDA production among four groups (siRNAn, siBcl6n, siRNAh, and siBcl6h). *: P < 0.05; **: P < 0.01; ***: P < 0.001; siBCl6: BCl6 knokdown; siRNA: negative control; n: nomoxia; h: hypoxia.
Bcl6 Knockdown and Hypoxia-Induced Myocardial Injury
We incubated the transfected siRNA negative control group and Bcl6-siRNA experimental group in normoxic or hypoxic chambers for 24 hours for subsequent experiments. Cells were divided into four groups: siRNA-normoxia, siRNA-hypoxia, bcl6-siRNA-normoxia, and bcl6-siRNA-hypoxia. A cell model of MI was established with hypoxia. Cell survival was determined with the MTT method, and the survival rate of the control group was set to 100%. Hypoxia decreased cell survival in both the siRNA-negative control group and the Bcl6-siRNA group (normoxia: P < 0.01; hypoxia: P < 0.05) (Figure 5C). The knockdown of bcl6 decreased cell survival under both normoxia and hypoxia (normoxia: P < 0.01; hypoxia: P < 0.05).
Bcl6 Knockdown Exacerbates the Inflammatory Response in H9c2 Cardiomyocytes
We used RT-PCR to detect the expression of the inflammatory factors TNF-α, IL-1, and IL-6 (Figure 5F–H). Hypoxia resulted in elevated expression of inflammatory factors in the siRNA negative control group and Bcl6-siRNA group (P < 0.01). Under both normoxia and hypoxia, bcl6 knockdown resulted in elevated inflammatory factor expression (P < 0.01).
Bcl6 Knockdown Exacerbates Oxidative Stress in H9c2 Cardiomyocytes
To assess oxidative stress levels, we examined NADPH oxidase subunits p67 and gp91, SOD activity, and MDA content in treated H9c2 cardiomyocytes in each group. Figure 5D and E show the group differences in NADPH oxidase subunit p67 and gp91 expression. Bcl6 knockdown resulted in increased expression under both normoxic and hypoxic conditions (P < 0.01). Figure 5I and J show SOD activity and MDA levels. Knockdown of Bcl6 decreased SOD activity and increased MDA levels under both normoxia and hypoxia (P < 0.01). These findings indicated that Bcl6 knockdown aggravates oxidative stress.
Discussion
High-throughput transcriptomics technology is a potential strategy to explore immune related genes in MI. In this study, we used the GSE29111 and GSE66360 datasets downloaded from the GEO database, and merged them as the training set to remove batch effects, whereas the GSE48060 dataset served as the validation set. We performed differential analysis in the merged dataset. Bias might potentially arise from the use of microarray data for differential gene expression analysis [10]; therefore, we used the limma software package for differential expression analysis, which takes into account the potential correlations between samples and array-specific noise when processing microarray data, thus further enhancing analytic accuracy and the credibility of the results [9]. Finally we filtered 91 differentially expressed genes with |log2 fold change| > 1 and P < 0.5, and visualized significant gene expression differences between the MI group and control group.
We performed GO and KEGG enrichment analyses of 91 differentially expressed genes. The main GO biological processes included response to lipopolysaccharide, response to molecules, bacterial origin, neutrophil tropism, neutrophil migration, and granulocyte chemotaxis. Reactions to lipopolysaccharides, reactions to molecules, bacterial origin, and other processes suggested inflammatory reactions after MI, particularly inflammation associated with bacterial infection or endotoxin exposure. Lipopolysaccharides are a component of bacterial cell walls that induce inflammatory reactions by activating pattern recognition receptors such as TLR4 in the immune system, thereby aggravating tissue damage after MI [22–24]. The main cell composition terms were secretory granule lumen and cytoplasmic vesicle lumen, thus indicating increased activity of neutrophils and macrophages after MI. These immune cells effectively remove waste in heart tissue and secrete inflammatory factors through their internal secretory granular cavity and cytoplasmic vesicle cavity, thus participating in cardiac inflammation and repair [25, 26]. The main molecular functional processes were receptor for advanced glycation end products (RAGE) receptor binding, pattern recognition receptor activity, carbohydrate binding, and immune receptor activity. By interacting with RAGE, the activation of immune receptors promotes the recruitment of neutrophils and macrophages, thereby initiating an inflammatory response and exacerbating heart damage [27, 28]. Pattern recognition receptors directly recognize specific molecular structures on the surfaces of pathogens in MI, trigger target cell signal transmission, activate the immune response, and regulate inflammatory responses in MI; therefore, immune responses participate in MI [29, 30]. The main KEGG pathway enrichment terms were the IL-17 signaling pathway, C-type lectin receptor signaling pathway, and lipid and atherosclerosis related pathways. The enrichment in the IL-17 signaling pathway and the C-type lectin receptor signaling pathway suggested the importance of regulating the immune response and pro-inflammatory effect after MI. IL-17, a pro-inflammatory cytokine produced by Th17 cells, is essential in recruiting neutrophils and other immune cells to inflammatory sites, and participates in the inflammatory response after MI [31]. After MI, damaged heart tissue triggers a series of immune responses, and the role of IL-17 in this process is particularly important. IL-17 promotes the recruitment of inflammatory cells, particularly neutrophils, to the damaged area. These neutrophils help remove dead myocardial cells by releasing enzymes, reactive oxygen species, and other pro-inflammatory mediators [32], but may also exacerbate local tissue destruction and inflammatory reactions [33]. The C-type lectin receptor signaling pathway is also an important immune regulation mechanism, which plays a crucial role in the inflammatory response after MI [34]. These receptors activate macroimmune cells such as macrophages and dendritic cells by recognizing dead cells and other dangerous signals in the tissue after injury [35]. After activation, these cells recruit neutrophils and other immune cells to inflammatory sites by releasing cytokines and chemofactors, thus promoting the inflammatory response and subsequent repair process [25, 36, 37]. The activation of this signaling pathway helps remove necrotic and apoptotic cells in the MI area; however, if improperly regulated, it may also aggravate damage to heart tissue [25].
Subsequently, we imported 91 differentially expressed genes into the STRING database and performed PPI analysis with an evidence level set to 0.9. The obtained data were imported into Cytoscape, and five algorithms (MCC, DMNC, MNC, Clustering, and BottleNeck) of the CytoHubba plug-in were used to identify the core genes and determine their intersection. We finally performed WGCNA on the training set and obtained 12 modules, and also calculated MM and GS. The MMgrey60 module had the highest correlation, of 0.52, at P < 0.05, and its GS was also highest. The genes in this module were screened with MM > 0.8, GS > 0.5 as candidate core genes. The results identified ACSL1, BCL6, C5AR1, CLEC4E, CSF3R, IL1R2, IRAK3, LILRB2, NAMPT, QPCT, S100A12, S100A9, and TLR2. The 13 candidate genes identified through WGCNA were intersected with the four previously identified candidate genes (IL1B, S100A12, BCL6, and S100A8) using the CytoHubba plug-in. The final results identified S100A12 and BCL6. We subsequently validated the diagnostic ability of these two final core genes. The expression of S100A12 and BCL6 significantly differed between the MI group and control group in the training set (P < 0.01). The AUCs were 0.809 (95% CI 0.733–0.874) and 0.837 (95% CI 0.771–0.896) for the two groups. The diagnostic ability in the validation set was equally favorable, with AUCs of 0.789 (95% CI 0.662–0.908) and 0.733 (95% CI 0.573–0.866).
Many factors affect the occurrence and development of MI. Askin has investigated the role of serum apelin levels in ST-elevation MI [38]. In addition, immune processes play a key role in the development of MI [7]. After MI, cardiac tissue damage triggers an acute inflammatory response including the activation and aggregation of immune cells, such as neutrophils and monocytes, in affected areas. These cells release inflammatory factors that help clear dead and necrotic cardiac cells [39, 40]. Together, these immune cells play essential roles in the cardiac restitution process. We performed an enrichment analysis of the immune gene set for MI related genes and found that patients with MI showed diminished B-cell to myeloid, CD4 T-cell to myeloid, and CD4 cell to peripheral blood mononuclear cell ratios. In contrast, controls showed elevated CD4 T-cell to B-cell, CD4 T-cell to myeloid, and CD4 cell to peripheral blood mononuclear cell ratios. Subsequently, to further clarify specific immune cell differences between the MI and control groups, we assessed immune cell infiltration according to the genetic profiles of 28 infiltrating lymphocytes downloaded from TISIDB. The MI group, compared with the control group, demonstrated fewer activated B cells (P = 0.039), CD4 effector T cells (P < 0.01), effector memory B cells (P = 0.047), and central memory CD4 T cells (P < 0.01), in agreement with previous immune gene set enrichment results. In addition, the MI group contained higher proportions of macrophages (P < 0.01), mast cells (P < 0.01), monocytes (P < 0.01), and neutrophils (P < 0.01) than the control group. Zhou similarly observed a trend of upregulation of neutrophils and macrophages in patients with MI [41]. Zhang has reported a trend of upregulation of neutrophils and macrophages in an MI model established by ligating the anterior descending branch of the left coronary artery in Syrian hamsters; the number of mast cells was much higher in the MI group than in the control group [42]. Kupreishvili, in patient necropsies of acute MI (up to 5 days; n = 27) and chronic MI (5–14 days; n = 18) as well as controls without heart disease (n = 10), has observed significantly higher coronary intima and mesocardial mast cell content in patients with acute and chronic MI than in controls [43]. Together, these findings suggest the involvement of mast cells in the development of MI. Dai et al. [44] has assessed the monocyte to lymphocyte ratio (MLR) in 109 patients with cardiac rupture and 327 hospitalized patients without cardiac rupture (OR = 3.57, 95% CI: 1.28–9.97, P = 0.015). Wang et al. [45] similarly found that the (neutrophil+ monocyte)/lymphocyte ratio (NMLR) is associated with mortality after hospital admission: the KM survival curve was significantly lower in the high NMLR group than the low NMLR group (P < 0.001), and the former group showed greater risk of in-hospital mortality (hazard ratio, 1.452; 95% confidence interval, 1.132–1.862; P < 0.05). We explored the relationship between core genes and immune cells through Spearman rank correlation analysis. Using P < 0.001 as a screening condition, we found that core genes were significantly positively associated with immune cells (monocytes, neutrophils, natural killer cells, and activated dendritic cells) that were upregulated in MI. These findings together suggested an inextricable relationship between immune processes and MI development and prognosis.
Our previous analysis indicated that S100A12 and BCL6 are highly correlated with the development of MI. The expression of these two genes was significantly higher in the MI group than the control group (P < 0.001). We determined the expression levels of S100A12 and BCL6 with RT-PCR in whole blood specimens from infarcted and non-infarcted hospitalized patients, to verify the accuracy of our previous results. The findings were consistent with our previous results, and indicated higher expression of S100A12 and BCL6 in the MI group than the control group (P < 0.05). Additionally, these two genes are associated with immune function. S100A12 plays a crucial role in modulating the immune response, particularly by influencing the activation of inflammatory pathways [46]. In addition, S100A12 facilitates the recruitment of neutrophils and macrophages by interacting with specific receptors, such as RAGE and possibly G-protein coupled receptors (GPCRs) [47, 48], thereby promoting the release of cytokines and chemokines that mediate the immune response, and leading to attraction of these cells to the inflamed tissues. These roles are also consistent with findings from our enrichment analysis, which identified molecular functional processes associated with RAGE receptor binding. The immune related gene BCL6, a transcriptional repressor, is integral to the immune regulation of B cells, and controls the expression of various genes essential for the activation and function of these cells. By repressing genes that trigger excessive immune responses, BCL6 ensures that the immune system remains balanced and does not overreact and potentially cause damage to the body [49]. This mechanism is crucial in preventing autoimmune disorders, wherein the immune system mistakenly attacks the body’s own tissues. This also aligns with our previous finding, where BCL6 gene expression has a negative correlation with activated B cells abundance.
We selected one of these two genes, BCL6, for further investigation. BCL6 plays a crucial role in regulating immune responses, particularly in controlling the development and function of B cells. After MI, an excessive or inappropriate immune response can lead to persistent cardiac inflammation, thus accelerating the progression of heart disease. B-cell-mediated immune responses are closely associated with cardiac inflammation, repair, and subsequent fibrosis. By suppressing excessive immune activation, BCL6 helps maintain immune balance, and can be beneficial in the cardiac repair process [50, 51]. Therefore, immune regulation plays a crucial role in the cardiac repair process, and the regulatory function of BCL6 helps alleviate inflammation and decrease the occurrence of cardiac lesions. Thus, studying the regulatory mechanisms of BCL6 and its role in MI might provide a foundation for developing new treatment strategies for heart attacks [52]. We incubated siBCL6-transfected or control siRNA-transfected H9c2 cardiomyocytes under normoxic or hypoxic conditions to construct hypoxic cell models. The expression of the inflammatory factors TNF-α, IL-1, and IL-6 was measured to assess the level of inflammation. The NADPH oxidase subunits p67 and gp91, SOD activity, and MDA content were measured to assess oxidative stress. The expression of inflammatory factors, MDA content, and SOD activity were significantly higher in cells under hypoxic than normoxic conditions, thus indicating that hypoxia led to an inflammatory response and oxidative stress in cells. Regardless of the presence of normoxic or hypoxic conditions, the content of inflammatory factors and MDA was further elevated, and the SOD activity was further diminished, in the BCL6 knockdown group, thus indicating that BCL6 knockdown further aggravated the inflammatory response and oxidative stress caused by hypoxia. These findings suggested that BCL6 might protect against inflammatory responses and oxidative stress. Chen has suggested that BCL6 binds and has a negative regulatory effect on nucleotide-binding oligomerization domain-like receptor family pyrin domain 3 (NLRP3), thus attenuating inflammation in human renal tubular epithelial cells and in vivo [53]. Zhang has found that BCL6 is a target of mir-205-5p, whereas mir-205-5p knockdown decreases the inflammatory response in allergic rhinitis by targeting BCL6 [54]. Jager has found that Bcl6 has an important regulatory function in macrophages, by limiting TLR-induced inflammatory responses [55]. In addition, BCL6 is transiently induced in macrophages and directly inhibits CCL2 (MCP-1) expression; therefore, BCL6 suppresses acute inflammatory responses [56, 57]. Lin has demonstrated that BCL6 increases miR-34a promoter H3K27me3 levels through EZH2 recruitment and CTRP9 upregulation, thus inhibiting cardiomyocyte apoptosis, and revealing the therapeutic value of BCL-6 for AMI [58]. The results of these studies are all consistent with our findings suggesting that BCL6 negatively regulates the inflammatory response and inhibits apoptosis of cardiomyocytes through various mechanisms. BCL6 overexpression inhibits ROS production in B-cell lymphoma cells, thereby suppressing increased levels of reactive oxygen species and apoptosis, and enhancing the antioxidant defense system [59]. Chen has similarly found that BCL6 overexpression decreases Ang II-induced NAD (P)H oxidase activation and ROS production, and BCL6 knockdown exacerbates Ang II-induced oxidative stress and proliferation of human vascular smooth muscle cells [60]. These observations are consistent with our findings that BCL6 knockdown exacerbates hypoxia-induced oxidative stress and cardiomyocyte injury. However, Wei has found that inhibition of BCL6 attenuates oxidative stress-induced neuronal injury, potentially by targeting the miR-31/PKD1 axis [61]. This finding is contrary to our conclusion; therefore, further studies remain necessary to explore the reasons for this discrepancy.
We found a compelling case for the clinical relevance of S100A12 and BCL6 in MI, given their combined properties. S100A12, a calcium-binding protein closely associated with inflammatory processes, has been extensively studied for its role in cardiovascular diseases. Our analysis indicated that S100A12 expression was upregulated post-MI, thus suggesting its involvement in the acute inflammatory response after infarction. This early expression change in S100A12 might potentially signal the onset of the pathological process, thereby aiding in the early diagnosis of MI. Similarly, BCL6 functions as a transcription inhibitor and plays a critical role in regulating immune responses and inflammation. We observed that the expression patterns of BCL6 were similar to those of S100A12, and showed significant increases post-MI. This expression shift is likely to represent a defensive response by myocardial cells to ischemia and ensuing inflammation. Monitoring BCL6 expression might not only facilitate early MI diagnosis but also offer insights into the regulatory mechanisms of inflammation post-MI.
Although this study did not directly analyze the interactions between the core genes S100A12 and BCL6 and traditional MI risk factors such as age, smoking, hypertension, and diabetes, the existing literature suggests that these factors might influence the expression of these genes through effects on inflammatory pathways and oxidative stress responses. For instance, age, an independent risk factor for cardiovascular diseases, is associated with prolonged low-grade inflammation, which in turn might lead to increased expression of inflammation-related genes such as S100A12 and BCL6, and potentially exacerbate the inflammatory response after MI. Similarly, smoking accelerates the progression of cardiovascular diseases by inducing oxidative stress and chronic inflammation, which in turn might not only increase the risk of MI but also enhance the expression of BCL6 through regulated signaling pathways. Likewise, hypertension contributes to myocardial injury by promoting the production of inflammatory mediators through continuous vascular pressure and consequent structural damage to blood vessels, thereby increasing the expression of both S100A12 and BCL6. Patients with diabetes might experience aberrant regulation of these genes, as a result of high blood sugar-induced oxidative stress and the accumulation of advanced glycation end products, which can intensify myocardial ischemia and subsequent inflammatory responses. These insights suggest that including common cardiovascular risk factors as covariates in future research might enable more accurate assessment of the potential of these genes to serve as biomarkers. A deeper understanding of the interactions between these genes and traditional risk factors might reveal the complex biological mechanisms of MI and foster the development of more targeted treatment strategies, thus offering new perspectives in the prevention and treatment of this condition.
We believe that S100A12 and BCL6, given their high potential as biomarkers, might markedly influence the management and treatment of MI, if detected early. Future research will focus on validating these markers in larger patient groups and exploring their clinical application value.
Limitations
The study has several limitations. The analyzed data came from microarrays, which were unable to identify novel genes. Although we combined the first two datasets as the training set, the sample size remained small, and some genes might potentially have been missed. Although core genes were identified, and PPI and co-expression network analyses were performed, the hierarchical processes between these core genes remain to be fully elucidated. The core immune-related genes still require further experiments in larger sample sizes to elucidate their biological functions in MI. We selected only the BCL6 gene for validation and explored it in an in vitro hypoxic cell model. Although this method provided the convenience of controlled experimental conditions to rapidly verify our hypotheses, it has limitations, particularly in replicating the complex in-body environment of MI. This method lacks the complexity of the extracellular microenvironment and organizational-level response; consequently, further animal experiments remain necessary to elucidate the mechanism of action.
In conclusion, this study provides a research basis for the prediction of MI-related genes and the discovery of new potential biomarkers. The core genes S100A12 and BCL6 might be considered as biomarkers for early detection of MI, and have promise as new therapeutic targets.