In the rapidly developing insurance industry, China saw an addition of 55.4 billion insurance policies in 2022, representing a 13.3% year-on-year growth rate, with a payout and expenditure of RMB 1.5 trillion Yuan [1]. Identity recognition of customers, a crucial cornerstone of transactions between insurance companies and customers, refers to the obligation of institutions to use reasonable methods to understand customers and their transactional information, to prevent insurance fraud, money laundering, and other illegal activities. This process involves various business aspects, such as customer registration, policy underwriting, endorsements, and claims processing [2]. Currently, the insurance industry relies primarily on valid identification documents and biometric recognition, such as facial, fingerprint, iris, and DNA technologies, for customer identity recognition. However, these methods have limited reliability, because they are relatively simple, and are vulnerable to imitation and forgery. In recent years, the number of insurance fraud cases involving the use of another person’s heart disease history has increased. These cases have resulted in substantial financial losses, thus undermining the fairness and authority of the law, and posing severe challenges to the development of the insurance industry. Under current circumstances, traditional customer identity verification methods and technologies no longer meet the customer identity recognition needs in the insurance industry. Therefore, the insurance industry, both domestically and internationally, urgently requires effective rapid biometric identity recognition technologies for identifying living people. The electrocardiogram (ECG), a surface recording of the electrical activity of the heart, is universal, portable, and based on unique and stable biometric features. Consequently, it has found cross-over applications in the field of information technology and has been used for biometric identity recognition of living people in the insurance industry.
ECG-based identity recognition technology has been applied in China’s domestic insurance industry for nearly 20 years and has achieved notable results. In 2006, Professor Li Zhongjian and colleagues from the Institute of Electrocardiology at Zhengzhou University proposed the groundbreaking hypothesis of ECG waveform uniqueness [3]. They subsequently extended this concept to medical insurance identification cards and applied for a national invention patent for ECG biometric identity recognition cards in the same year [4]. In 2009, the research achievement of using ECG examination technology for the recognition of living people received the Third Prize of the Henan Province Scientific and Technological Progress Award [5]. In 2022, research applying ECGs to the identification of insured personnel received the First Prize of the Henan Province Scientific and Technological Achievements Award [6]. The research team has published more than 30 related articles.
To further enrich and improve biometric recognition technology and promote the application of ECG-based biometric identity recognition of living people in the insurance industry, the Institute of Electrocardiology of Zhengzhou University and the Tianjin Institute of Cardiology have jointly organized relevant experts in the field to prepare the “Expert consensus on ECG identification applied in the insurance industry.”
Common Biometric Recognition Technologies
Biometric recognition technology is a technique that combines computer technology with optics, acoustics, and biometric sensors to collect, collate, compare, and analyze an individual’s inherent physiological or behavioral characteristics for personal identification [7]. Because of their universality, portability, and basis on unique and stable biometric features, various biometric recognition technologies, such as facial recognition [8], fingerprint recognition [9], iris recognition [10], genetic recognition [11], hand shape recognition [12], voiceprint recognition [13], and gait recognition [14] systems have gradually been developed and applied in multiple fields.
Biometric recognition technologies are generally classified into three categories: lesser biometric recognition technology, high biometric recognition technology, and esoteric biometric recognition technology. Lesser biometric recognition technologies include facial, palm, palmprint, signature, and voice recognition. Because of the ease at which these biometric features can be imitated or mimicked, their reliability of identification is relatively low; therefore, these methods are generally suitable for applications with lower security requirements. High biometric recognition technologies refer to the recognition of retinal, iris, and fingerprint features. These biometric features are relatively complex and difficult to imitate. Consequently, these methods are widely recognized for their high credibility and accuracy, and are commonly applied in scenarios with relatively high security requirements, such as government departments, military institutions, and banks. Esoteric biometric recognition technologies involve primarily vascular patterns, human body odors, and DNA recognition. These biometric features are highly complex and cannot be easily imitated, and are generally considered to have absolute credibility and accuracy. However, collecting vascular patterns and human body odor characteristics is challenging, and current recognition technologies are immature. DNA recognition technology is relatively mature but has high costs. This method is used primarily in criminal investigations and paternity testing. Each biometric recognition technology has its own limitations. For example, retinal recognition has a high misrecognition rate among people with eye diseases; iris recognition is less suitable for people with darker irises or eye diseases; and fingerprint recognition may encounter fingerprint blurring difficulties due to finger injuries or scars. Additionally, fingerprints can be easily replicated with latex, thus resulting in low forgery costs.
Each biometric recognition technology also has unique characteristics and performs well in different situations. By combining multiple complementary biometric recognition technologies, the reliability can be further improved, thus broadening application prospects [15–19]. New methods are being researched with the aim of enriching and improving biometric recognition technology, rather than replacing one method with another. The main goal is to use simple and reliable biometric features for identification. Currently, the insurance industry uses different methods to achieve facial, fingerprint, iris, genetic, and voiceprint recognition, as well as traditional documents, such as identification cards, for customer identity recognition. In practice, except for identification cards being the main identification method, others are less commonly used.
Advantages of Bio-feature Recognition Technology Based on ECGs
Before the heart contracts, it generates an electrical impulse, recorded by an electrocardiograph machine, thus forming a dynamic curve, known as the ECG, that varies rhythmically with the cardiac cycle [20]. The normal ECG waveform generally includes the P wave, QRS complex, T wave, U wave, PR interval, QT interval, and ST segment, each of which has its own normal range in terms of morphology, voltage, and duration. ECGs, the primary method for cardiac electrophysiological examination, have been clinically applied for more than 120 years and are considered the gold standard for diagnosing cardiac arrhythmias. Moreover, ECGs meet the four basic requirements for biometric identification of living people: universality, portability, and a basis on biometric features that are both unique and stable. Hence, ECGs may serve as a new biological feature for identity recognition, thereby extending conventional ECG examination to the field of information technology.
Universality
ECGs have the advantages of being non-invasive, non-destructive, safe, ecologically friendly, and easy to obtain. They have been widely applied and promoted worldwide; have become a routine examination for outpatients and inpatients; are used in health examination centers; and serve as an essential test for patients with cardiovascular disease. Almost all medical institutions are equipped with electrocardiograph machines, which are operated by professional ECG technicians. Therefore, a living person’s ECG can be collected anytime and anywhere.
Portability
As society expands, people travel to various places for work, life, and tourism. In cases of emergency, people can have their ECGs recorded with widely available civilian ECG devices and then uploaded to a network for remote assistance in diagnosis. Alternatively, people can have their ECGs recorded at medical institutions for precise diagnosis and treatment. Therefore, the ECGs of living people are portable and can be collected at different locations worldwide.
Uniqueness
Theoretically, each person’s heart has unique electrical footprints, which are detectable on ECGs. This uniqueness includes specific bio-feature recognition points, thus making ECGs inherently individual-specific. Although the ECG waveform may undergo significant changes during an individual’s growth from birth to adulthood, it stabilizes and remains relatively consistent after adulthood. Moreover, 12-, 15-, and 18-lead ECGs provide numerous characteristic points for identification, similarly to the concept of using genetic short tandem repeat testing for identifying individuals.
Stability
The ECG of a living person may show amplitude, timing, and minor waveform changes under different conditions, such as times, temperatures, heart rates, and health conditions. However, these changes do not affect the identification of a living person. Notably, the QRS waveform remains relatively stable and unchanged, thereby demonstrating the stability of an individual’s ECG bio-features. Thus, the QRS waveform of the ECG can serve as the primary identifying characteristic and an important basis for identification.
Compared with use of other biometric features, such as facial changes due to cosmetic surgery or damage, or fingerprint damage and counterfeiting, DNA identification is complex, costly, and time-consuming. This method also faces challenges in cases of genetic mutations or identical twins. ECG bio-feature recognition technology overcomes these limitations, with its advantages of low cost, convenience, tamper resistance, and non-replicability. However, it also has several limitations, such as an inability to achieve identification when a living person’s normal sinus rhythm is replaced by ventricular arrhythmias or when the heart stops beating.
Major Aspects of ECG Biometric Identification Technology
By definition, ECG biometric identification technology uses an electrocardiograph machine to record the electric activity waveform generated by the heart during each cardiac cycle from the body surface of a living person. This waveform then provides identification feature points for identity recognition and authentication.
The standardized use of electrocardiograph machines should conform to national or international standards (digital electrocardiograph machines with permanent storage of raw data are recommended). The sampling frequency should be 0.05–150 Hz for adults and 0.05–250 Hz for children [21].
During ECG recording, accurate and fixed placement of the lead electrodes and consistency of lead electrode positions should be ensured during every examination. Operators should receive standardized training to avoid lead electrode misconnections and waveform interference.
The 12-, 15-, or 18-lead ECGs of a living person collected before and after insurance enrollment or on multiple occasions should be compared.
Letters and symbols should be used to qualitatively mark the morphological characteristics of P-QRS-T-U waves in the 12-, 15-, or 18-lead ECG and compare them according to different waveforms. For example, the P wave should be upright, the QRS wave should be qRs, the ST segment should be normal, and the T wave should be upright, with a U wave.
Quantitative analysis of the morphological characteristics of P-QRS-T-U waves in the 12-, 15-, or 18-lead ECG should be performed. Starting from lead I, the voltage, duration, notches, and humps of each wave should be carefully observed, recorded, and compared. If the waveform description is the same in lead I, lead II should subsequently be observed; if the waveform description remains the same in lead II, lead III should subsequently be observed, and so on, until all leads have been analyzed. Absolute and relative methods can be used to describe the waves. For the absolute method, if the voltage of the waveform is ≥0.5 mV, uppercase letters should be used for identification; if the voltage of waveform is <0.5 mV, lowercase letters should be used. For example, if the QRS wave in a lead appears as qR, the voltage of the q wave is <0.5 mV, and the voltage of the R wave is >0.5 amV. For the relative method, in the comparison of two waveforms, the waveform with a larger amplitude should be marked with uppercase letters, and the waveform with a smaller amplitude should be marked with lowercase letters. For example, if both the voltages of the R and the S waves in a lead are >0.5 mV (or <0.5 mV), the waveform with the larger amplitude should be marked with uppercase letters, and the other should be marked with lowercase letters. A specific morphological characteristic (such as voltage, duration, double peaks, notches, or humps) should be added as a suffix to describe the wave.
Whether the examined ECGs are from the same person is determined through comparison of two or more ECGs.
Whether the examined ECGs are normal is determine through comparison of two or more ECGs.
If the waveforms of two or more ECGs differ (possibly because of different settings on the electrocardiograph machine), thus potentially affecting the recognition decision, ECG experts can be asked to perform a preliminary qualitative analysis to assess the machine’s performance indicators and a quantitative analysis within an error range. If necessary (and if possible), a new ECG may be collected to increase the accuracy of ECG waveform recognition.
Features of ECG and Features Extraction for ECG Biometric Identification
Relevant International Studies
Since 2001, research on ECG biometric identification outside China, primarily in engineering or biomedical fields, has been performed by using publicly available ECG databases. The research methods have evolved from early mathematical and signal processing comparisons to traditional machine learning models and, more recently, deep learning methods. All these studies have confirmed the feasibility and reliability of using ECGs for identity recognition. The relevant research findings are summarized in Table 1.
International Engineering or Biomedical Studies and Results.
Research | Year | Study Population/Database | Comparison Items | Main Techniques or Methods | Results |
---|---|---|---|---|---|
Biel [22] | 2001 | Healthy volunteers/– | Extracted features related to time and amplitude of lead I | Soft independent modeling of class analogy (SIMCA) methods | Accuracy rate of 90%–100% |
Shen [23] | 2005 | Healthy volunteers/– | Extracted features, such as time and amplitude, from the QRS complex | Decision-Based Neural Networks (DBNN) | Seventeen features screened out could be used in identity recognition |
Israel [24] | 2003 | Healthy volunteers/– | Combination of facial and ECG features | Principal Component Analysis (PCA) and multimodality fusion | ECG alone correctly classified 55 ± 11% Combined ECG and face attributes identified 94 ± 6% |
Wübbeler [25] | 2007 | Healthy volunteers/PTB ECG Database | 100 ms interval centered on the R peak | Distance comparison between two ECG vectors | Equal error rate <3% |
Agrafioti [26] | 2008 | Healthy volunteers/– | Fiducial-based feature extracted | Linear Discriminant Analysis (LDA) | ECG signals can be used for identity recognition |
Wang [27] | 2008 | Healthy volunteers/– | Time and amplitude based multi-feature points and single feature point | Autocorrelation coefficients and the discrete cosine transform (DCT) | Identity can be recognized without detecting the baseline features in the ECG signal |
Deng [28] | 2018 | Healthy volunteers/Fuwai and PTB ECG Database | Extracted cardiac dynamics along the periodic ECG state trajectories | Radial basis function (RBF) neural networks | A dynamic ECG recognition framework is established |
Biran [29] | 2020 | Healthy volunteers /ECG ID Database | Non-fiducial-based feature extracted: Short Time Fourier Transform (STFT) | Fréchet distance | Average recognition accuracy of 96.45% |
Belo [30] | 2020 | Healthy volunteers /Fantasia, MIT-BIH, CYBHi ECG Database | Features extracted by non-fiducial-based method | Recurrent neural network (RNN) and time convolutional neural networks (TCNN) | Almost 100%, 96%, and 90% accuracy for identification, and 0.0%, 0.1%, and 2.2% equal error rate |
Pereira [31] | 2022 | Healthy volunteers /CYBHi Database | Features extracted by non-fiducial-based method | Traditional machine learning methods with Convolutional Neural Networks (CNN) | Accuracy rates of 79.37% and 69.84% |
Camara [32] | 2023 | Healthy volunteers (participants engaged in four specific activities)/MIT-BIH, ECG-GUDB Database | Gramian Angular Field (GAF) images transformed from ECG | CNN | Accuracy rate of 91% |
Relevant Domestic Studies
Domestic Engineering Studies
Since 2005, domestic engineering researchers have conducted studies on ECG biometric identification. They have mainly used various algorithms to extract feature points from ECG signals, thus effectively increasing the accuracy of ECG signal identity recognition. The relevant research findings are summarized in Table 2.
Domestic Engineering Studies and Results.
First Author | Year | Identity Recognition Method |
---|---|---|
Wang [33] | 2005 | The improved Radial Basis Function (RBF) Network was used to develop a biometric identification system. |
Zhuang [34] | 2005 | Feature points were extracted with the discrete wavelet transform, and the Euclidean minimum distance method was then used for identification. |
Chen [35] | 2006 | Dynamic model parameters, adjusted with a Genetic Algorithm (GA) and Particle Swarm Optimization, were input into a neural network for identity recognition. |
Lin [36] | 2007 | Autocorrelation Coefficient and Linear Discriminant Analysis (LDA) were used for recognizing a person’s identity. |
Hao [37] | 2010 | Through computer processing of ECG images to obtain digital information and conducting non-repeated experiments with bivariate analysis of data, identity recognition based on ECG was verified to be feasible. |
Shi [38] | 2011 | The Dynamic Time Warping (DTW) algorithm was used to determine the optimal matching distance between heartbeat test data and various heartbeat templates. |
Wang [39] | 2012 | Identification recognition was conducted based on single-lead ECG frequency band characteristics. |
Chen [40] | 2014 | By measuring the preprocessing results through the signal-to-noise ratio and correlation coefficient, various classification and recognition methods were tested, and the most suitable method for ECG recognition was confirmed to be support vector machine (SVM) with small samples. |
Huang [41] | 2020 | The fusion feature ECG signal recognition algorithm based on Hilbert vibration decomposition and Convolutional Neural Network has efficient recognition performance and good application prospects. |
Dou [42] | 2022 | A quick connection design and extraction of feature points was achieved by increasing the number of convolutional layers and kernels. |
Domestic Medical Studies
Since 1988, Professor Li Zhongjian at the Institute of Electrocardiology of Zhengzhou University has conducted research on ECG biometric identification and was the first to apply related research results to the insurance industry. Before that time, no international reports had described using ECG examination technology for identifying living people, thus making Professor Li the world’s first expert in using ECG examination technology for identifying living people [43]. In 2005 and 2006, Professor Li trained two master’s students in ECG identity recognition and proposed the following academic viewpoints: (1) The “ECG waveform uniqueness” hypothesis [3] was proposed and then extended to the application of medical insurance cards [4, 44]. (2) Each person’s 12-lead ECG P-QRS-T-U wave contains unique ECG waveform features [45] (Table 3 [4]). (3) Each living person’s ECG waveform is different, and has slightly different waveforms in qualitative and quantitative analysis [46]. (4) Under the influence of different environments, seasons, heart rates, times, medications, and other factors, the QRS waveform of the same living person remains relatively stable and essentially unchanged [47]. (5) Different regions (areas) have non-repeating ECG waveforms in adults [48]. (6) The QRS waveform of the ECG is tall, with minimal changes and variations, and therefore is suitable as an indicator for rapid artificial intelligence (AI)-based identity recognition [49]. Table 4 lists the relevant research findings from Professor Li Zhongjian’s team.
Each Segment of a Single Cardiac Cycle in a 12-Lead Electrocardiogram (ECG).
ECG Segment | Direction | Duration | Number of Feature Points* | ||||
---|---|---|---|---|---|---|---|
Upright | Inverted | Bidirectional | Non-Deviation | Absence | |||
P wave | √ | √ | √ | √ | √ | 5 | |
QRS complex | |||||||
Q wave | √ | √ | √ | 3 | |||
R wave | √ | √ | √ | 3 | |||
S wave | √ | √ | √ | 3 | |||
T wave | √ | √ | √ | √ | √ | 5 | |
U wave | √ | √ | √ | √ | √ | 5 | |
P-R segment | √ | 1 | |||||
ST segment | √ | √ | √ | √ | 4 | ||
Q-T interval | √ | 1 | |||||
| |||||||
Sum in lead I | 5 | 6 | 3 | 1 | 6 | 9 | 30 |
Total in 12 leads | 60 | 72 | 36 | 12 | 72 | 108 | 360 |
*A single cardiac cycle in one lead can have 30 feature points. With 12 leads, there are 30 × 12 = 360 feature points used for identity recognition [4].
Relevant Domestic Medical Research Results in Electrocardiogram (ECG) Identity Recognition.
First Author | Year | Method | Results | Conclusion |
---|---|---|---|---|
Li [3] | 2006 | Selected 100 synchronous 12-lead ECGs for descriptive analysis and comparison of similarities and differences | Of 100 cases, no two ECG waveforms were exactly the same in all 12 leads. | The “ECG waveform uniqueness” hypothesis was proposed. |
Li [45] | 2008 | Randomly selected 3000 12-lead ECGs from adults, extracted feature points from ECG waveforms, and conducted statistical analysis | A 12-lead ECG waveform can have 3432 feature points in one cardiac cycle. | Each person’s P-QRS-T-U waves in the 12-lead ECG is unique to the individual. |
Li [46] | 2008 | Selected 6000 adult people living in Zhengzhou for qualitative and quantitative analysis of their 12-lead ECG waveforms | No completely identical waveforms were found among 6000 12-lead ECGs. | The uniqueness of ECG waveforms in living people was confirmed. |
Li [47] | 2008 | Examined ECGs of 5500 living adults with different environments, seasons, heart rates, times, medications, etc. | Despite various influencing factors, the QRS waveforms of the same living person remained relatively stable. | ECG waveforms show stability. |
Li [48] | 2009 | Conducted qualitative and quantitative analysis of 12-lead ECG waveforms in 3586 adults in Zhengzhou | ECG waveforms of adults in Zhengzhou were not duplicated, thus confirming the uniqueness of ECG waveforms in living people. | The uniqueness of ECG waveforms in living people was further validated. |
Li [49] | 2009 | Studied the application value of QRS waveforms in 12-lead ECG for computer-based identity recognition | The QRS waves have a tall appearance, few influencing factors, small waveform variations, and low variability. | QRS waves are suitable as indicators for rapid computer-based identity recognition |
Technical Aspects of Using ECG for Identity Recognition in the Insurance Industry
The technical identification process of using ECG identity recognition in the insurance industry is shown in Figure 1. In identifying the customer’s identity for insurance purposes, the applicant’s questioned sample must be compared with the applicant’s known sample. The applicant’s known sample refers to the 12-, 15-, or 18-lead ECG recorded before application for insurance, which can be extracted from an ECG database. The applicant’s questioned sample refers to the disease ECG recorded by the applicant when applying for a claim (single or multiple records).
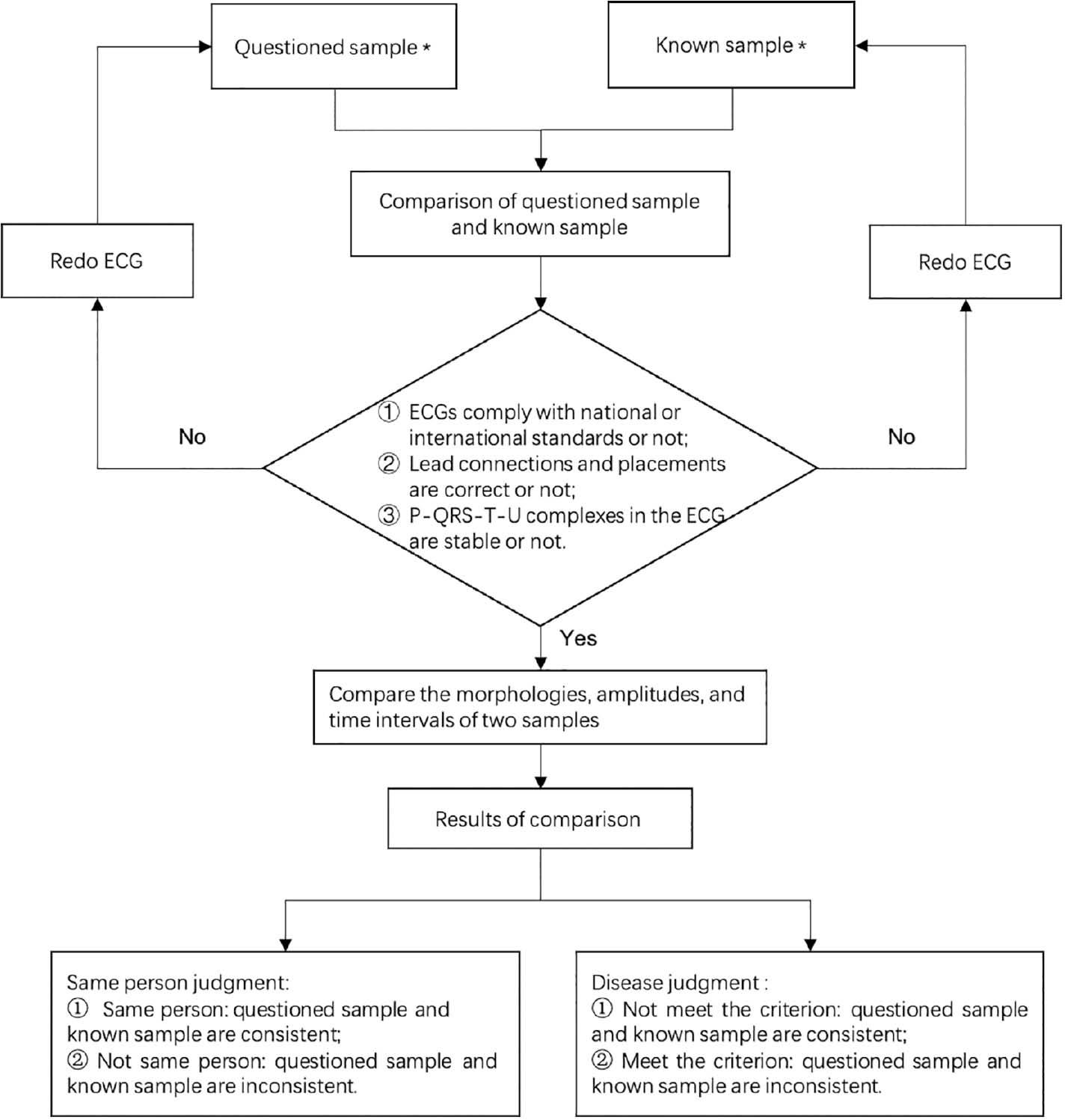
Technical Aspects of Using ECG for Identity Recognition in the Insurance Industry.
*12-, 15-, or 18-lead electrocardiogram (ECG).
Comparing the applicant’s questioned sample with the known sample includes primarily the following steps:
Observe whether the questioned sample and known sample ECGs comply with national or international standards, with sampling frequencies of 0.05–150 Hz for adults and 0.05–250 Hz for children.
Check whether the lead-electrode connections and placements are correct, to avoid misconnections and interference during ECG recording.
Observe whether the P-QRS-T-U complexes in the ECG are stable, clear, and distinguishable.
Compare the morphologies, amplitudes, and time intervals of the applicant’s 12-, 15-, or 18-lead ECG collected as the questioned sample and the known sample, and perform qualitative and quantitative analyses. Qualitatively mark the morphological features of the P-QRS-T-U complexes in the 12-, 15-, or 18-lead ECG with letters or symbols, and compare them according to the different waveform orders. Then perform quantitative analysis of the qualitatively described morphological features of the P-QRS-T-U complexes. Starting from lead I, carefully observe and record the voltages, durations, notches, humps, and other characteristics for comparing the applicant’s questioned sample with the known sample. If the waveform description in lead I is the same, proceed to observe lead II. If the waveform description in lead II remains the same, continue to observe lead III, and so on, until all leads are analyzed. Use uppercase and lowercase letters to indicate the size of each waveform, followed by specific morphological features (e.g., voltage, durations, double peak, notches, or humps) in the description.
On the basis of the comparison results between the applicant’s questioned sample and the known sample, whether the ECGs from multiple collections are from the same person, and whether the disease ECGs belong to the same person, can be determined, thus confirming whether the known sample meets the insurance company’s claim disease criteria.
Future Outlook
Previous research results have fully demonstrated the stability of the ECG, particularly the morphology of the QRS complex, which is an important cornerstone of ECG identity recognition. Simultaneously, with the rapid development of AI and machine learning based on large-scale ECG databases, this characteristic of ECG is used. Computer-based personal identity recognition software systems are expected to be developed by using digital signal processing, hierarchical classification, pattern recognition, and other technologies to achieve rapid computer-based personal identity recognition. This future trend in the development of ECG biometric recognition technology is expected. Moreover, the future may witness transitions from recognition by experts to ordinary people, and from human eye recognition to AI recognition. Furthermore, ECG biometric recognition technology, after being widely applied in the insurance industry, will ideally continually expand to other fields, such as medical insurance, banks, judiciary, public security, military, government, and enterprise areas, to better promote social stability and national security.