Introduction
Cardiovascular diseases, particularly acute myocardial infarction (AMI), are the leading cause of disability and death [1]. AMI is a myocardial necrosis caused by acute and persistent coronary artery ischemia and hypoxia [2]. Atherosclerosis, the pathological basis of AMI, can be accelerated by chronic inflammation [3]. Although early treatment with reperfusion after AMI onset can improve clinical outcomes, the risk of recurrence and mortality remains high for as long as 1 year after AMI onset [4]. In addition, reperfusion therapy not only increases damage to ischemic myocardial tissue but also implicates normal myocardial tissue [5]. Reperfusion-induced injury accounts for as much as 50% of overall myocardial injury, and is often associated with serious adverse events [5]. Therefore, preventing AMI is a major priority.
Ulcerative colitis (UC) is a type of inflammatory bowel disease that causes chronic inflammation of the intestinal tract [6]. Its pathological mechanism is complex and influenced by multiple factors, such as genetic background, intestinal immune status and intestinal microbial balance. The risk of developing colon cancer is 2–3 fold greater in patients with UC than the general population. [7, 8]. The age of onset of UC is bimodal, with peaks at 2–3 years and 50–80 years [9]. UC affects not only the gastrointestinal tract but also the heart, and pericarditis and myocarditis are the most common manifestations [10]. Furthermore, patients with UC are at significantly elevated risk of both venous thromboembolism and mesenteric ischemia [10].
Although UC has been reported to be a risk factor for AMI progression [11], the relationship between these two diseases remains controversial. Osterman et al. [12] have concluded that patients with UC do not have elevated AMI incidence. However, Choi et al. [13] have reported elevated risk of myocardial infarction in patients with UC and a trend towards a younger incidence of AMI. UC causes systemic inflammatory responses and promotes hypercoagulation in the body [13]. Furthermore, disruption of the intestinal mucosal barrier leads to the transfer of lipopolysaccharides (LPS) and other endotoxins into the bloodstream, thereby inducing the secretion of pro-inflammatory cytokines and leading to endothelial disturbances, atherosclerosis and acute cardiovascular events [14]. Gut microecological dysregulation increases plaque vulnerability by affecting lipid metabolism and the inflammatory response [15]. Azimi et al. [16] have demonstrated that Clostridium difficile, Escherichia coli and Campylobacter are closely associated with the development of UC. Chronic bacterial infections may contribute to the formation of vulnerable plaques through enhanced T-cell activation and inflammatory responses [17]. Therefore, the cardio-intestinal axis may be an important pathway through which UC promotes the development of AMI. Owing to inconsistent research findings regarding the relationship between AMI and UC, no standard of medical care has been established for patients with both conditions. This study therefore explored the pathogenesis of AMI and UC, to provide new perspectives on the clinical management of patients with AMI and UC.
Matrials and Methods
Data Source
Relevant datasets in the Gene Expression Omnibus (GEO; https://www.ncbi.nlm.nih.gov/geo/) database [18] were searched with the keywords “acute myocardial infarction” and “ulcerative colitis.” The inclusion criteria were: 1) Homo sapiens (top organism); 2) expression profiling with an array (study type); 3) total number of samples in a single microarray dataset >50; and 4) single microarray dataset from the same sequencing platform. We downloaded four microarray datasets from GEO: GSE66360-GPL570 [19] and GSE62646-GPL6244 [20] for AMI, and GSE87473-GPL13158 [21] and GSE59071-GPL6244 [22] for UC. The GSE66360 and GSE87473 datasets were used for mechanistic analysis of differentially expressed genes (DEGs), and GSE62646 and GSE59071 were used for further screening of hub genes. GSE66360 contained 49 AMI and 50 normal (NL) samples; GSE62646 included 84 AMI and 14 NL samples; GSE87473 contained 106 UC-active and 21 NL samples; and GSE59071 included 74 UC-active, 23 UC-inactive and 11 NL samples. Datasets were downloaded from the GEO database via the R GEOquery package and pre-processed with Rstudio [23]. Genes were annotated with gene symbols. Because the data used in this study were obtained from public databases, no local ethics committee approval or informed consent was required.
Identification of DEGs
We analyzed the GSE66360 and GSE87473 datasets separately with the R limma package [24]. Filtering criteria (|logFC|>0.585 and adjusted P<0.05) were used to determine DEGs between AMI or UC and the corresponding NL groups. Volcano plots of DEGs were constructed with the R ggplot2 package [25, 23]. Among the DEGs, logFC>0.585 or logFC<−0.585 indicated upregulation or downregulation in the disease group, respectively [26, 27]. We used the R VennDiagram package to plot Venn diagrams of up- and downregulated genes between the datasets GSE66360 and GSE87473 to obtain co-DEGs common to both diseases [28].
Functional Enrichment Analyses of co-DEGs
Gene Ontology (GO) and Kyoto Encyclopedia of Genes and Genomes (KEGG) analyses were used to evaluate functional annotation of the key module genes. GO terms include biological process, cellular component and molecular function categories [29]. KEGG is a knowledgebase for systematic analysis of gene function, linking genomic information to higher-order functional information [30]. We performed enrichment analysis with the R clusterProfiler package [31].
PPI Network Construction and Module Analysis
The STRING (https://cn.string-db.org/) database [32] is designed to integrate all known and predicted associations among proteins. The common DEGs (co-DEGs) were input into the STRING database, “Homo sapiens” was selected, the minimum required interaction score was set to ≥0.4, and the free nodes were hidden. The downloaded results were input into Cytoscape software in tab-separated values format for further analysis [33]. The key modules were obtained with the MCODE plugin in Cytoscape with default settings. Subsequently, enrichment analysis was performed on the module genes.
Screening and Analysis of Hub Genes
The cytoHubba plugin in Cytoscape was used to filter hub genes [34]. Here, we used four algorithms (degree, closeness, EPC and MCC) and selected the genes of the first 20 intersections of these four algorithms for further analysis. The co-expression network of these hub genes was constructed via the GeneMANIA (http://genemania.org/) [35] database, with the species set to human.
Validation of Hub Gene Expression
We further validated hub gene expression in the GSE62646 and GSE59071 datasets. P-value <0.05 was considered to indicate a significant difference.
Prediction and Verification of Transcription Factors
The TRRUST (https://www.grnpedia.org/trrust) database [36] was used to predict transcriptional regulatory networks. The species was set to human, and the co-hub genes stably expressed in the datasets GSE66360, GSE87473, GSE62646 and GSE59071 were imported into the TRRUST database to obtain the associated transcription factors (TFs). TF regulatory networks were constructed in Cytoscape. Subsequently, the expression of these TFs was further validated with the datasets GSE66360 and GSE87473.
Results
Identification of DEGs
Figure 1 shows the diagram of the study design and workflow. After normalization of the microarray data, 670 upregulated and 405 downregulated DEGs in GSE66360, and 1323 upregulated and 1138 downregulated DEGs in GSE87473, were identified (Figure 2A, B). The upregulated and downregulated DEGs were intersected separately to identify co-DEGs for AMI and UC, of which 233 co-DEGs were upregulated, and 34 were downregulated (Figure 2C, D).
Functional Enrichment Analyses of Co-DEGs
Functional enrichment analyses were performed for 267 co-DEGs (Figure 3A, B). GO enrichment analysis revealed the following biological processes: response to lipopolysaccharide, response to molecule of bacterial origin, positive regulation of cytokine production and leukocyte migration (Figure 3A). KEGG enrichment analysis suggested the importance of osteoclast differentiation, lipid and atherosclerosis, NF-kappa B, TNF and IL-17 signaling pathways (Figure 3B, Table 1). Together, these results suggested that inflammation and the immune response are common core mechanisms of UC and AMI.
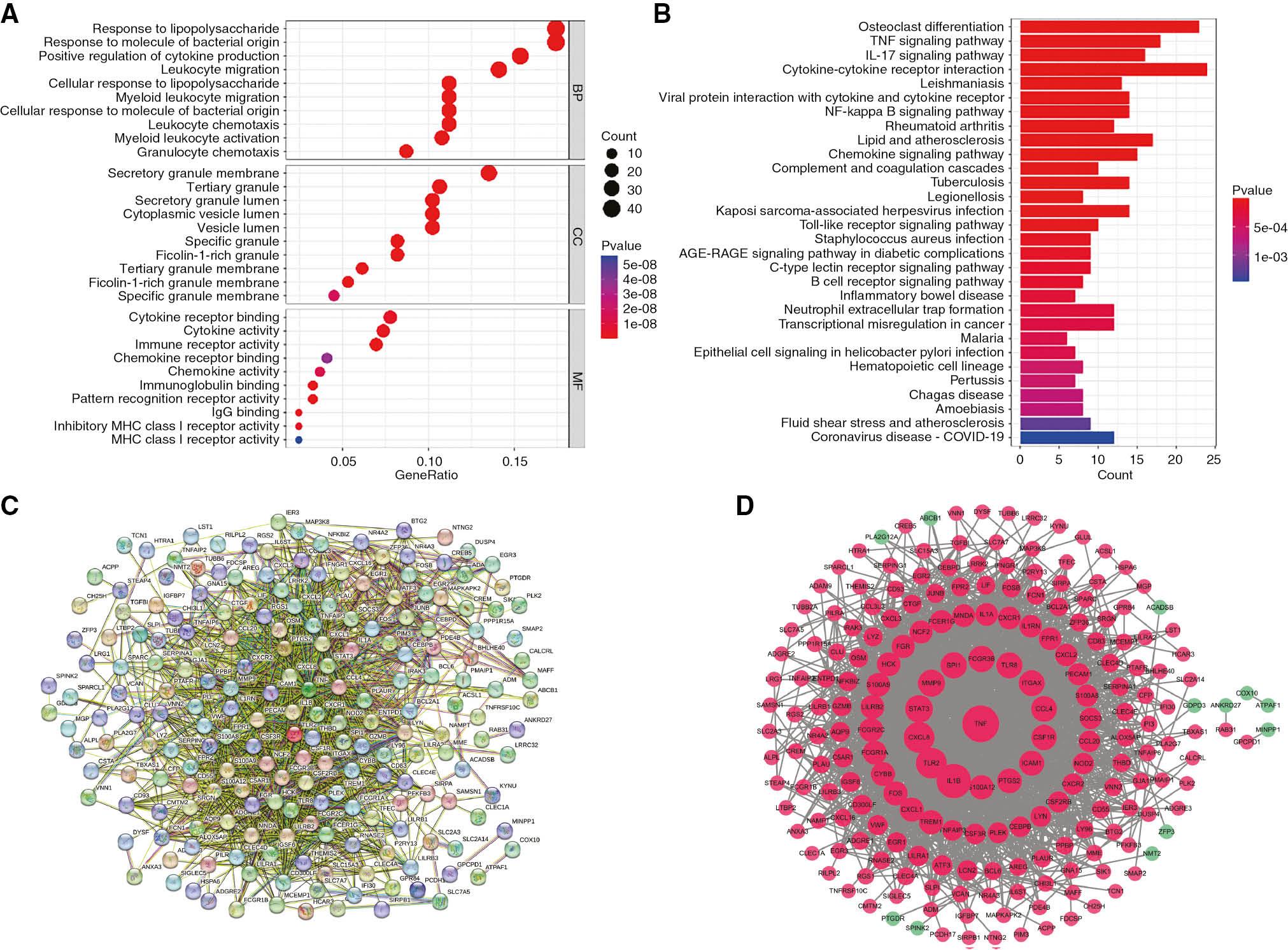
Functional Enrichment and PPI Network Analyses of Co-DEGs.
(A) GO. (B) KEGG. (C) STRING. (D) Cytoscape. Red indicates upregulated genes, and green indicates downregulated genes.
Top 30 Enriched KEGG Pathways.
ID | Description | P-value | GeneID |
---|---|---|---|
hsa04380 | Osteoclast differentiation | 1.15E-16 | IL1B/SOCS3/LILRB2/FOS/FCGR2A/FOSB/JUNB/SIRPA/FCGR2C/LILRA2/LILRB1/IFNGR1/FCGR3B/SPI1/SIRPB1/LILRA3/LILRB3/TNF/CSF1R/NCF2/FCGR1A/IL1A/LILRA1 |
hsa04668 | TNF signaling pathway | 2.21E-12 | MAP3K8/IL1B/CCL20/SOCS3/CXCL2/ICAM1/FOS/JUNB/MMP9/CXCL1/TNFAIP3/CEBPB/CXCL3/TNF/LIF/PTGS2/CREB5/NOD2 |
hsa04657 | IL-17 signaling pathway | 1.60E-11 | IL1B/CCL20/S100A9/CXCL2/FOS/FOSB/MMP9/CXCL1/CXCL8/S100A8/TNFAIP3/CEBPB/CXCL3/TNF/PTGS2/LCN2 |
hsa04060 | Cytokine-cytokine receptor interaction | 1.15E-09 | IL1B/CCL20/IL1RN/CCL4/CXCL2/CCL3L3/IL6ST/CSF3R/CXCL16/CXCL1/CXCL8/OSM/IFNGR1/CXCR1/RELT/TNFRSF10C/CXCL3/TNF/CSF1R/LIF/CSF2RB/IL1A/CXCR2/PPBP |
hsa05140 | Leishmaniasis | 1.56E-09 | IL1B/TLR2/FOS/FCGR2A/FCGR2C/IFNGR1/FCGR3B/TNF/NCF2/PTGS2/FCGR1A/IL1A/CYBB |
hsa04061 | Viral protein interaction with cytokine and cytokine receptor | 4.55E-09 | CCL20/CCL4/CXCL2/CCL3L3/IL6ST/CXCL1/CXCL8/CXCR1/TNFRSF10C/CXCL3/TNF/CSF1R/CXCR2/PPBP |
hsa04064 | NF-kappa B signaling pathway | 7.67E-09 | IL1B/CCL4/CXCL2/ICAM1/BCL2A1/CXCL1/CXCL8/LY96/PLAU/LYN/TNFAIP3/CXCL3/TNF/PTGS2 |
hsa05323 | Rheumatoid arthritis | 1.53E-07 | IL1B/CCL20/TLR2/CXCL2/CCL3L3/ICAM1/FOS/CXCL1/CXCL8/CXCL3/TNF/IL1A |
hsa05417 | Lipid and atherosclerosis | 5.60E-07 | IL1B/TLR2/CXCL2/CCL3L3/ICAM1/FOS/MMP9/STAT3/CXCL1/CXCL8/LY96/LYN/CXCL3/HSPA6/TNF/NCF2/CYBB |
hsa04062 | Chemokine signaling pathway | 3.14E-06 | CCL20/CCL4/CXCL2/CCL3L3/CXCL16/STAT3/CXCL1/CXCL8/LYN/CXCR1/FGR/CXCL3/HCK/CXCR2/PPBP |
hsa04610 | Complement and coagulation cascades | 4.54E-06 | THBD/PLAUR/SERPINA1/CD55/C5AR1/ITGAX/VWF/PLAU/CLU/SERPING1 |
hsa05152 | Tuberculosis | 7.16E-06 | CLEC4E/IL1B/FCER1G/TLR2/FCGR2A/FCGR2C/ITGAX/IFNGR1/FCGR3B/CEBPB/TNF/FCGR1A/IL1A/NOD2 |
hsa05134 | Legionellosis | 1.03E-05 | IL1B/TLR2/CXCL2/CXCL1/CXCL8/CXCL3/HSPA6/TNF |
hsa05167 | Kaposi sarcoma-associated herpesvirus infection | 1.69E-05 | ZFP36/CXCL2/ICAM1/IL6ST/FOS/MAPKAPK2/STAT3/CXCL1/CXCL8/IFNGR1/LYN/CXCL3/HCK/PTGS2 |
hsa04620 | Toll-like receptor signaling pathway | 2.50E-05 | MAP3K8/IL1B/TLR2/CCL4/CCL3L3/FOS/CXCL8/LY96/TNF/TLR8 |
hsa05150 | Staphylococcus aureus infection | 7.84E-05 | C5AR1/ICAM1/FCGR2A/FCGR2C/FPR1/FPR2/PTAFR/FCGR3B/FCGR1A |
hsa04933 | AGE-RAGE signaling pathway in diabetic complications | 0.000107926 | THBD/IL1B/ICAM1/STAT3/CXCL8/TNF/EGR1/IL1A/CYBB |
hsa04625 | C-type lectin receptor signaling pathway | 0.000146178 | CLEC4E/IL1B/FCER1G/CLEC4D/MAPKAPK2/TNF/EGR2/EGR3/PTGS2 |
hsa04662 | B cell receptor signaling pathway | 0.000149294 | LILRB2/FOS/LILRA2/LILRB1/LYN/LILRA3/LILRB3/LILRA1 |
hsa05321 | Inflammatory bowel disease | 0.000210338 | IL1B/TLR2/STAT3/IFNGR1/TNF/IL1A/NOD2 |
hsa04613 | Neutrophil extracellular trap formation | 0.000245589 | TLR2/C5AR1/AQP9/FCGR2A/FPR1/FPR2/VWF/FCGR3B/TLR8/NCF2/FCGR1A/CYBB |
hsa05202 | Transcriptional misregulation in cancer | 0.000283882 | NFKBIZ/BCL6/NR4A3/BCL2A1/MMP9/CXCL8/PLAU/SPI1/CEBPB/CSF1R/GZMB/FCGR1A |
hsa05144 | Malaria | 0.000332765 | IL1B/TLR2/ICAM1/CXCL8/PECAM1/TNF |
hsa05120 | Epithelial cell signaling in Helicobacter pylori infection | 0.000334702 | CXCL2/CXCL1/CXCL8/LYN/CXCR1/CXCL3/CXCR2 |
hsa04640 | Hematopoietic cell lineage | 0.000546951 | IL1B/CD55/CSF3R/MME/TNF/CSF1R/FCGR1A/IL1A |
hsa05133 | Pertussis | 0.000555306 | IL1B/FOS/CXCL8/LY96/TNF/SERPING1/IL1A |
hsa05142 | Chagas disease | 0.00066795 | IL1B/TLR2/CCL3L3/FOS/GNA15/CXCL8/IFNGR1/TNF |
hsa05146 | Amoebiasis | 0.00066795 | IL1B/TLR2/CXCL2/GNA15/CXCL1/CXCL8/CXCL3/TNF |
hsa05418 | Fluid shear stress and atherosclerosis | 0.001243797 | THBD/IL1B/ICAM1/FOS/MMP9/PECAM1/TNF/NCF2/IL1A |
hsa05171 | Coronavirus disease, COVID-19 | 0.001456841 | IL1B/TLR2/C5AR1/IL6ST/FOS/FCGR2A/STAT3/VWF/CXCL8/TNF/TLR8/CYBB |
PPI Network Construction and Module Analysis
PPI network analysis of co-DEGs was performed with the STRING database (Figure 3C). The results were imported into Cytoscape in tab-separated values format to construct a PPI network. The PPI network comprised 205 nodes and 1782 edges (Figure 3D). The more connections between nodes and the higher the relevance, the higher the node’s ranking in the PPI network. (Figure 3C, D). In addition, five tightly linked gene modules were screened via the MCODE plugin in Cytoscape (Figure 4A–E). Furthermore, modular genes were integrated, and GO and KEGG analyses were performed (Figure 4F, G). GO enrichment analysis indicated that modular genes were enriched primarily in biological processes, such as response to molecule of bacterial origin, response to lipopolysaccharide and leukocyte migration (Figure 4F). KEGG enrichment analysis revealed that modular genes were involved mainly in osteoclast differentiation, lipid and atherosclerosis, NF-κB, TNF and IL-17 signaling pathways (Figure 4G). Overall, AMI and UC had many common pathogenic mechanisms, which might be mediated by specific hub genes.
Screening and Analysis of Hub Genes
The top 20 genes were screened in the cytoHubba plugin, with the degree, closeness, EPC and MCC algorithms. (Table 2). The R ggplot2 package was used to plot a Venn diagram to identify hub genes (Figure 5A). Eleven hub genes were screened: TNF, IL1B, TLR2, CXCL8, STAT3, MMP9, ITGAX, CCL4, CSF1R, ICAM1 and CXCL1 (Table 3). The GeneMANIA database was used to structure hub gene co-expression networks [35]. Co-expression network analysis indicated complicated PPI networks with co-expression (72.67%), co-localization (14.25%), physical interactions (5.40%), pathways (2.98%), shared protein domains (2.47%), predicted interactions (1.86%) and genetic interactions (0.37%) (Figure 5B). GO analysis revealed the key associations of hub genes with biological processes such as leukocyte migration, cellular response to lipopolysaccharide, cellular response to molecule of bacterial origin, cytokine-mediated signaling pathway and cytokine receptor binding (Figure 6A). These results suggested the importance of LPS and cytokines in both AMI and UC. In addition, the KEGG results suggested that the hub genes were involved in lipid and atherosclerosis, rheumatoid arthritis (RA), AGE-RAGE signaling pathway in diabetic complications, NF-kappa B, IL-17, and Toll-like receptor signaling pathways (Figure 6B).
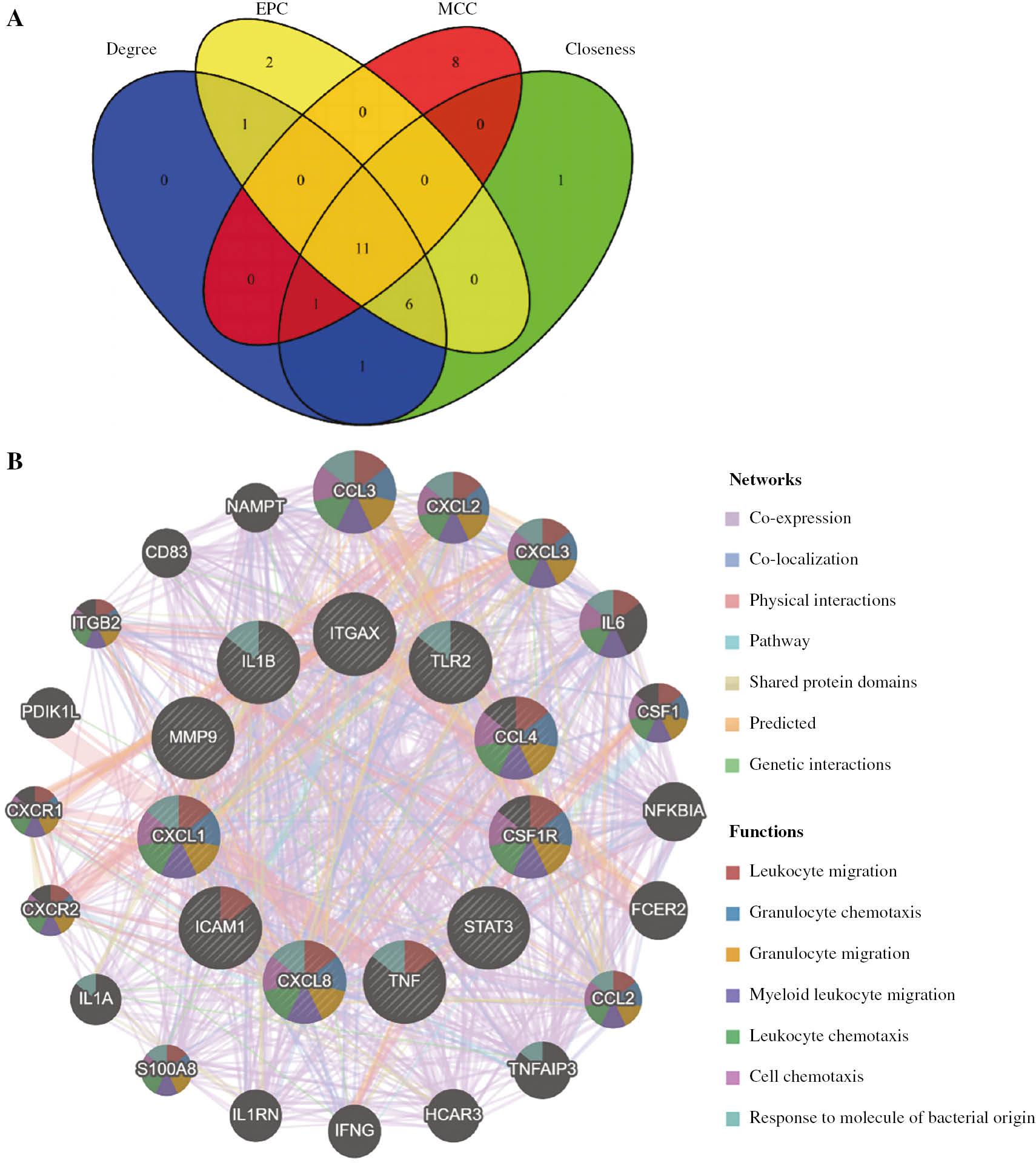
Venn Diagram and Co-Expression Network of Hub Genes.
(A) Venn diagram, showing 11 overlapping hub genes identified by four algorithms. (B) Co-expression network of hub genes.
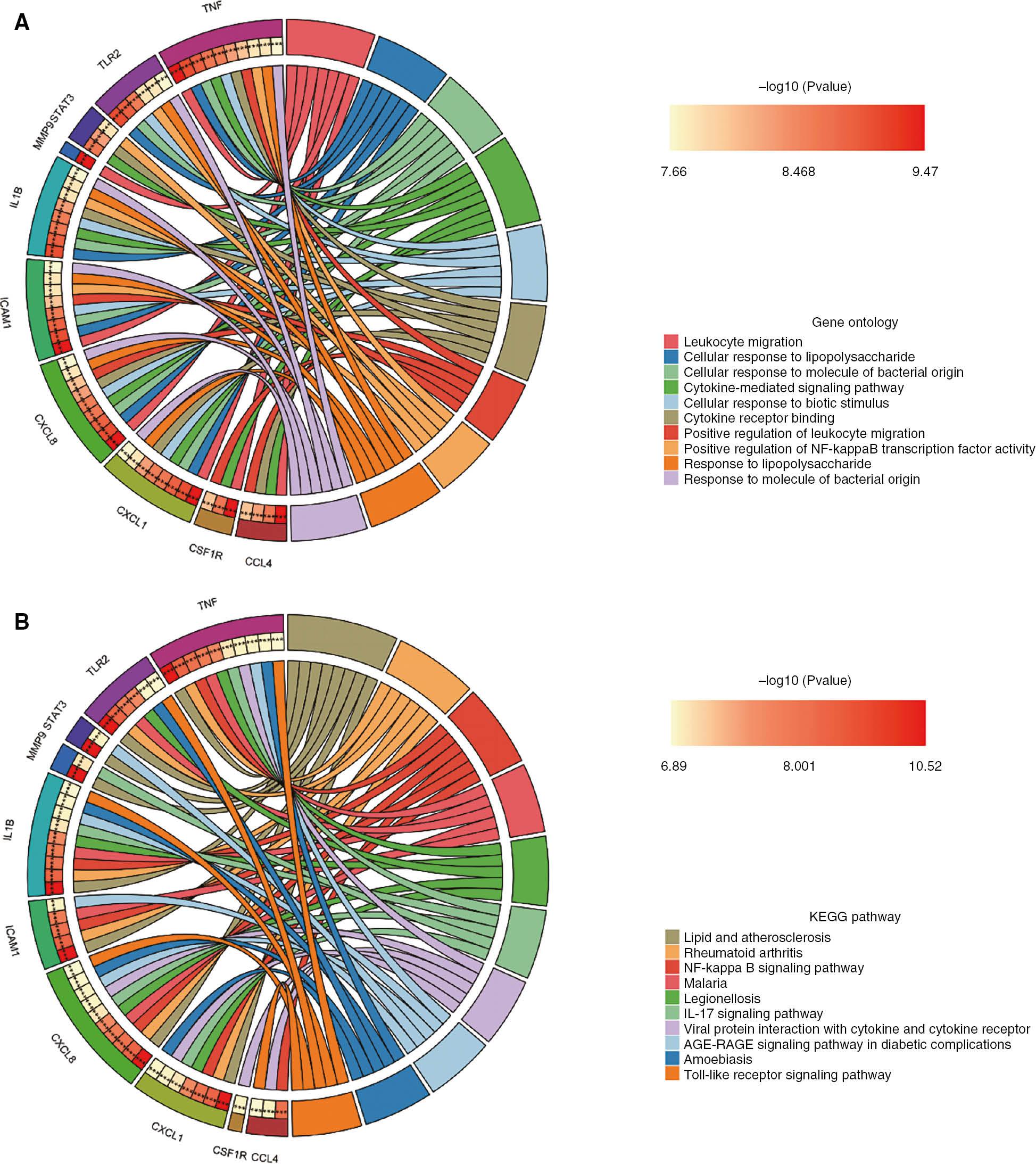
Functional Enrichment Analyses of Hub Genes.
(A) GO. (B) KEGG. The left half-circle represents hub genes significantly enriched in different terms. Different colors on the right side represent different terms. *** P<0.001.
Top 20 Genes Ranked in cytoHubba.
Rank | Degree | Closeness | EPC | MCC |
---|---|---|---|---|
1 | TNF | TNF | IL1B | TLR2 |
2 | IL1B | IL1B | TNF | TNF |
3 | TLR2 | CXCL8 | TLR2 | IL1B |
4 | CXCL8 | TLR2 | CXCL8 | CXCL8 |
5 | STAT3 | STAT3 | CCL4 | CCL4 |
6 | MMP9 | MMP9 | STAT3 | STAT3 |
7 | SPI1 | SPI1 | ITGAX | ICAM1 |
8 | FCGR3B | CCL4 | TLR8 | CXCL1 |
9 | TLR8 | FCGR3B | FCGR3B | CXCL2 |
10 | ITGAX | TLR8 | SPI1 | IL1A |
11 | CCL4 | ICAM1 | MMP9 | IL1RN |
12 | CSF1R | CSF1R | CSF1R | PTGS2 |
13 | ICAM1 | ITGAX | TREM1 | CXCL3 |
14 | PTGS2 | PTGS2 | CXCL1 | MMP9 |
15 | S100A12 | CYBB | CYBB | CCL20 |
16 | TREM1 | CXCL1 | S100A12 | CXCR2 |
17 | CYBB | FOS | ICAM1 | TNFAIP3 |
18 | FOS | S100A12 | S100A9 | SOCS3 |
19 | CXCL1 | TREM1 | LILRB2 | ITGAX |
20 | FCGR2C | FCGR1A | FCGR2C | CSF1R |
Information on the 11 Hub Genes.
Entry | Gene symbol | Description |
---|---|---|
P01375 | TNF | Tumor necrosis factor |
P01584 | IL1B | Interleukin-1 beta |
O60603 | TLR2 | Toll-like receptor 2 |
P10145 | CXCL8 | C-X-C motif chemokine 8 |
P40763 | STAT3 | Signal transducer and activator of transcription 3 |
P14780 | MMP9 | Matrix metalloproteinase-9 |
P20702 | ITGAX | Integrin alpha-X |
P13236 | CCL4 | C-C motif chemokine 4 |
P07333 | CSF1R | Macrophage colony-stimulating factor 1 receptor |
P05362 | ICAM1 | Intercellular adhesion molecule 1 |
P09341 | CXCL1 | C-X-C motif chemokine 1 |
Validation of Hub Gene Expression
We additionally selected two datasets including AMI and UC and validated the reliability of the 11 hub genes. In the dataset GSE62646, seven hub genes were significantly differentially expressed in the AMI versus NL group: TNF, STAT3, CCL1, ITGAX, CSF1R, ICAM1 and CCL4 (Figure 7). Interestingly, CCL4 expression was downregulated in AMI (GSE62646), contrary to the previous trend of upregulated expression in AMI (GSE66360), possibly because of insufficient sample size, the sequencing technology used, sample variation, etc. The remaining six hub genes with significant differences were all significantly upregulated in the AMI group, in agreement with the previous expression trend. In the GSE59071 dataset, all 11 hub genes were significantly upregulated in the UC-active group compared with the NL group (Figure 8). We defined the six hub genes that were stably upregulated in all datasets as co-hub genes; these genes were TNF, STAT3, ITGAX, CSF1R, ICAM1 and CCL1.
Prediction and Verification of TFs
Using the TRRUST database, we identified 11 TFs that might regulate the expression of the co-hub genes (Figure 9 and Table 4). The reliability of the 11 TFs was verified in the GSE66360 and GSE87473 datasets. In GSE66360, eight TFs significantly differed between the AMI and NL groups (Figure 10). Six TFs (SPI1, RELA, NFKB1, JUN, CEBPD and CEBPA) were upregulated, and two TFs (HDAC1 and STAT1) were downregulated in the AMI group compared with the NL group. In GSE87473, six TFs were significantly differentially expressed between the UC-active and NL groups (Figure 11). Five TFs (STAT1, SPI1, RELA, NFKB1 and CEBPD) were upregulated, and one TF (CEBPA) was downregulated in the UC-active group compared with the NL group. Notably, CEBPA and STAT1 showed opposite expression trends in the AMI and UC groups, perhaps because of factors such as disease progression, cell cycle, tissue and sample differences. SPI1, RELA, NFKB1 and CEBPD were stably upregulated in both the AMI and UC-active groups. These TFs may be involved in disease progression through the regulation of TNF, STAT3, ITGAX, ICAM1 and CCL1.
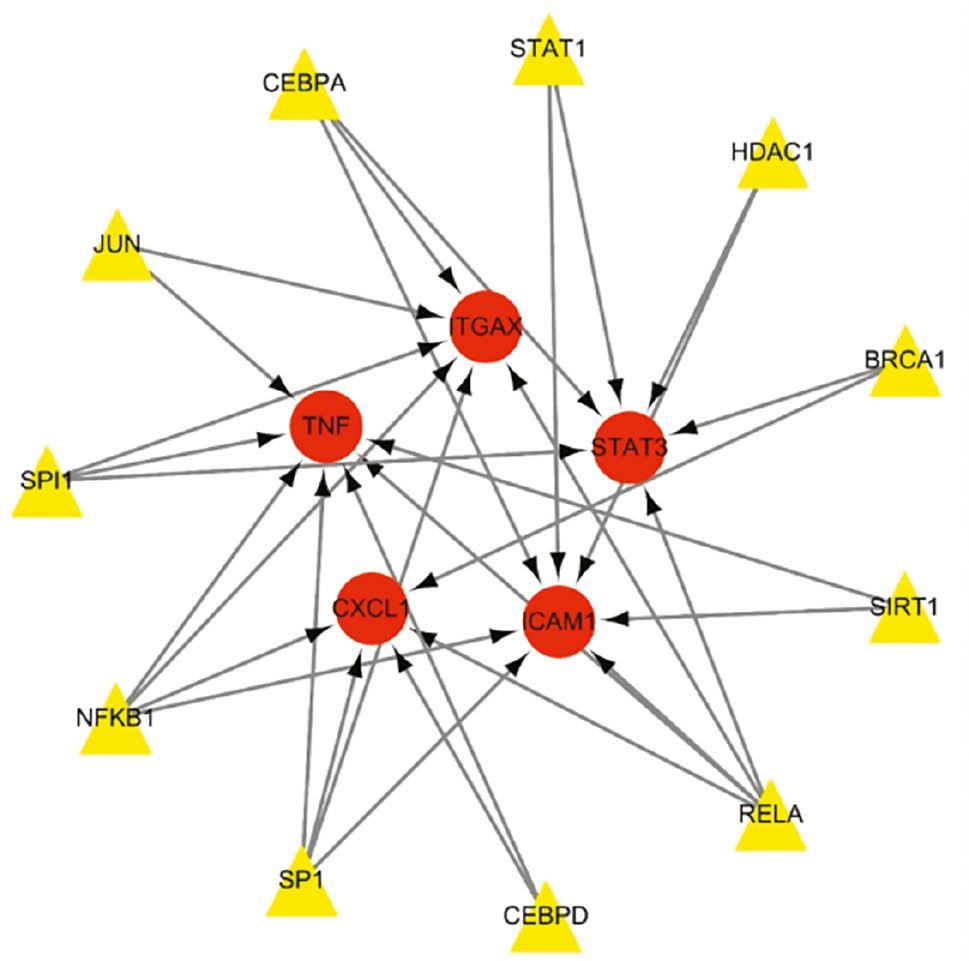
Transcriptional Regulatory Network.
Yellow represents transcription factors, and red represents genes.
Key Transcriptional Factors of Co-Hub Genes.
Key TF | Description | P-value | Genes |
---|---|---|---|
RELA | v-rel reticuloendotheliosis viral oncogene homolog A (avian) | 5.91E-09 | ICAM1, STAT3, ITGAX, TNF, CXCL1 |
CEBPA | CCAAT/enhancer binding protein (C/EBP), alpha | 3.70E-07 | ICAM1, ITGAX, STAT3 |
SPI1 | Spleen focus forming virus (SFFV) proviral integration oncogene spi1 | 6.71E-07 | STAT3, TNF, ITGAX |
NFKB1 | Nuclear factor of kappa light polypeptide gene enhancer in B-cells 1 | 9.53E-07 | ITGAX, ICAM1, TNF, CXCL1 |
SP1 | Sp1 transcription factor | 5.57E-06 | ICAM1, TNF, ITGAX, CXCL1 |
CEBPD | CCAAT/enhancer binding protein (C/EBP), delta | 1.14E-05 | TNF, CXCL1 |
SIRT1 | Sirtuin 1 | 9.44E-05 | ICAM1, TNF |
BRCA1 | Breast cancer 1, early onset | 0.000133 | CXCL1, STAT3 |
HDAC1 | Histone deacetylase 1 | 0.000207 | STAT3, ICAM1 |
STAT1 | Signal transducer and activator of transcription 1, 91 kDa | 0.00029 | ICAM1, STAT3 |
JUN | Jun proto-oncogene | 0.00091 | ITGAX, TNF |
Discussion
UC, a type of inflammatory bowel disease, is an immune-associated chronic inflammatory disease affecting the intestines [6]. Notably, active UC not only aggravates the systemic inflammatory response but also contributes to the hypercoagulable state of the body [13]. AMI is a myocardial necrosis caused by acute and persistent coronary artery ischemia and hypoxia [2]. Atherosclerotic plaque rupture is the most common cause of AMI [37]. In addition to accelerating the progression of atherosclerosis, UC can lead to the formation of vulnerable plaques by affecting intestinal microecology [17]. Patients with UC have significantly higher thrombotic events and cardiovascular mortality [38]. Kristensen et al. [39] have similarly concluded that UC contributes to an increased risk of AMI, stroke and cardiovascular death, particularly when UC is active, thus further increasing the incidence of these adverse events. In addition, Ha et al. [40] have suggested that female patients with UC are more likely to develop AMI than their male counterparts. However, Sinh et al. [41] have reported that UC does not increase mortality in patients with AMI. Currently, no consensus exists, and the relationship between AMI and UC remains controversial. The present study explored the pathogenesis of AMI and UC, to provide new perspectives for the clinical management of patients with AMI and UC, and novel ideas for further research on the molecular mechanisms of AMI combined with UC.
We identified 267 co-DEGs shared by the two diseases through bioinformatic methods. Finally, six co-hub genes were found to be stably upregulated in both AMI and UC: TNF, STAT3, CCL1, ITGAX, CSF1R and ICAM1. The results of enrichment analysis indicated that UC may promote AMI through inflammatory and immune responses. GO enrichment analysis highlighted the importance of leukocyte migration, cytokine-mediated signaling pathway, cellular response to lipopolysaccharide and positive regulation of NF-kappa B TF activity. Leukocyte migration is an early event in vascular inflammation progression and is closely associated with atherosclerosis [42]. In addition, chemokines and cytokines have been associated with chronic inflammation [43]. Atherosclerosis is a pathological disease characterized by fibroproliferation, chronic inflammation, lipid accumulation and immune disorders of the vessel wall [44]. As atherosclerotic plaques progress to advanced stages, vulnerable plaques rupture, thereby leading to the development of AMI. UC is characterized by a massive accumulation of immune cells, myeloid cells and lymphocytes in the diseased intestines [45]. The continued activation of these cells, together with the production of inflammatory mediators, promotes UC recurrence, thus making complete disease cure difficult [45]. Therefore, blocking the migration of leukocytes to the intestines is the main strategy used to control UC and relieve symptoms [45]. LPS is a potent inducer of inflammation. Intestinal LPS binds LPS-binding protein and celiac particles, and enters the body’s circulation through the lymphatics, thus enhancing the inflammatory process [46]. In addition, disruption of the intestinal mucosal barrier in UC leads to the transfer of LPS and other endotoxins into the bloodstream, thereby inducing a pro-inflammatory cytokine response that leads to endothelial dysfunction, atherosclerosis and acute cardiovascular events [14]. KEGG enrichment analysis highlighted the importance of lipid and atherosclerosis, RA, NF-kappa B, IL-17 and Toll-like receptor signaling pathways. Atherosclerosis is an inflammatory disease characterized by lipid accumulation in the arterial wall [47]. Lipids and atherosclerosis are the pathological basis for the development of AMI. The pathogenesis of RA is associated with chronic inflammatory and immune system disorders [48]. Numerous studies have reported a significantly elevated risk of AMI and UC in patients with RA [49, 50]. NF-κB is involved in immunity, inflammation, cell proliferation and apoptosis [51]. In particular, overactivation of the NF-κB signaling pathway is closely associated with various inflammatory diseases [51].
IL-17 is expressed by various leukocyte subsets, such as gamma-delta (γδ) T cells, natural killer (NK) cells, NK T cells and neutrophils [52]. IL-17 exacerbates the inflammatory response of plaque tissues, and promotes thrombosis and vulnerable plaque formation [52]. TLRs are clonal transmembrane signaling receptors that link intrinsic and specific immunity [53]. They are found primarily in macrophages, dendritic cells, NK cells and lymphocytes [54]. TLRs are activated by binding damage-associated molecular patterns, microbial-associated molecular patterns and pathogen-associated molecular patterns, thereby regulating inflammation and the immune response [55].
Eleven TFs that may regulate co-hub gene expression were identified with the TRRUST database. However, colony-stimulating factor 1 receptor (CSF1R) was found to be outside the transcriptional regulatory network. This finding might have been due to a lack of data in the TRRUST database. CSF1R is a type I single-transmembrane protein that is abundantly enriched in myeloid cells. CSF1R binds its endogenous ligands (CSF1 and IL-34) and activates downstream signaling pathways, including PI3K/AKT, JAK/STATs and MAPK, thereby regulating the proliferation, differentiation, migration and activation of target immune cells [56]. Xiang et al.[56] have suggested that CSF-1R is a potential target for regulating inflammatory diseases. Furthermore, through further validation, four TFs (SPI1, RELA, NFKB1 and CEBPD) were found to be stably upregulated in AMI compared with UC. These TFs synergistically regulate co-hub genes (TNF, STAT3, ITGAX, ICAM1 and CCL1).
TNF acts as a pleiotropic cytokine, which directly induces the expression of inflammation-associated genes, induces cell death, and indirectly drives inflammation and immune responses [57]. Luo et al. [58] have proposed TNF-α as a novel biomarker for predicting plaque rupture in patients with ST-elevation myocardial infarction. Thus, inhibition of TNF-α may decrease the inflammatory load of the body and stabilize plaques, particularly in people with multiple inflammatory diseases. TNF-α antagonists are important drugs in the treatment of UC [59]. However, long-term inhibition of TNF-α increases the risk of opportunistic infections and skin cancer [60]. To overcome such limitations, inhibition of TNF-α downstream inflammation-associated pathways may serve as an alternative. TNF activates caspase protease, JNK and NF-κB signaling pathways, thus regulating apoptosis, inflammation and immune processes [61]. Diminished DNA methylation in diseased tissue during AMI induces SPI1 overexpression and overactivates the TNF-α/NF-κB signaling pathway, thus exacerbating myocardial tissue inflammation [62]. The NF-κB family has five members: NFKB1, NFKB2, RELA, c-REL and RELB [63]. These proteins dimerize and form functional NF-κB. Among them, the NFKB1 gene promoter-94 insertion/deletion ATTG polymorphism is associated with the risk and severity of acute coronary syndromes [64]. Chen et al. [65] have found that inhibition of the inflammatory pathway associated with v-rel avian reticuloendotheliosis viral oncogene homolog A (RELA) is an effective target for the treatment of UC. In addition, inhibition of the RELA/TNF-α signaling pathway in AMI protects cardiac function through anti-inflammatory effects [66].
CCAAT enhancer binding protein delta (CEBPD), a member of the CEBP family, is a TF that regulates many biological processes, particularly inflammation and immune responses [67, 68]. CEBPD is activated by inflammatory factors such as IL-6, IFN-α, IFN-γ and IL1B, and it participates in inflammation and immune regulation [67]. CEBPD is considered an inflammatory enhancer in aortic endothelial cells, and it exacerbates tissue damage in concert with TNF-α [69]. CEBPD aggravates tissue damage by inducing inflammatory gene expression in liver tissue, lung tissue and brain glial cells [70]. Moreover, CEBPD promotes macrophage polarization toward M1 and exacerbates tissue inflammation [71]. CEBPD and TNF have been found to be stably upregulated in patients with AMI and UC. Thus, the CEBPD/TNF axis may be a potential mechanism for the treatment of AMI and UC. However, this possibility must be further verified in in vivo and in vitro models.
STAT3 is an important regulator of cell proliferation, differentiation, apoptosis, angiogenesis, inflammation and the immune response [72]. STAT proteins are activated by a variety of protein kinases, including Janus kinase, growth factor receptor, non-receptor tyrosine kinase and G protein-coupled receptor [73]. Among them, STAT3 is involved in atherosclerosis through the regulation of endothelial cell function, macrophage polarization, inflammation and the immune response [74]. STAT3 has four isoforms with different functions: STAT3-α, STAT3-β, STAT3-γ and STAT3-δ [74]. STAT3-α-mediated activation of the IL-6/JAK2/STAT3 signaling pathway is predominantly pro-inflammatory [74]. Jiang et al. [75] have constructed a UC model in rats and have reported anti-inflammatory protective effects via inhibition of the JAK2/STAT3 signaling pathway, thereby decreasing IL-1β, IL-6 and TNF-α expression. However, STAT3-β promotes the expression of certain anti-inflammatory genes while inhibiting the synthesis of inflammatory factors [74]. These predominant anti-inflammatory effects may be associated with IL-10-mediated activation of STAT3 [76]. Li et al. [77] have found that targeting the activation of the IL-10/STAT3 axis exerts cardioprotective effects by modulating the inflammatory response, myocardial fibrosis and apoptosis. In addition, activation of the IL-10/STAT3 signaling pathway promotes macrophage polarization to the M2 type [78]. STAT3 and SPI1 are overexpressed in patients with ankylosing spondylitis and are closely associated with immune system disorders [79]. Similarly, we found that STAT3 and SPI1 were overexpressed in patients with both AMI and UC, thus suggesting that SPI1/STAT3 may be an important pathway leading to AMI and UC; however, further experimental verification is warranted. Overactivation or inactivation of STAT3 can lead to human disease [80]. Therefore, how to balance the activation of STAT3 must be further explored.
Integrin alpha-X (ITGAX) is member of the integrin family [81]. ITGAX is considered a potential therapeutic target for various inflammatory and immune-associated diseases, such as periodontitis, atherosclerosis, primary dry syndrome and IgA nephropathy [82–84]. Overexpression of ITGAX has been shown to activate the PI3k/Akt axis and promote angiogenesis in ovarian tumor tissues. However, no relevant animal experiments have been reported for ITGAX in AMI or UC.
ICAM-1 is a transmembrane glycoprotein expressed at low basal levels in immune cells, endothelial cells and epithelial cells [85]. ICAM-1 expression is induced by multiple inflammatory cytokines and shows tissue-specific differences. ICAM-1 in endothelial cells is induced primarily by TNFα or IL-1β, whereas in intestinal epithelial cells, it is induced by IFNγ [86]. Freitas et al. [87] have demonstrated that circulating ICAM-1 levels are positively associated with the risk of coronary artery disease and are a potential marker of cardiovascular disease. The ICAM-1 gene polymorphism is closely associated with the development of UC [88]. ICAM-1 plays a fundamental role in neutrophil crossing of the endothelial cell layer [88]. Yu et al. [89] have found that ICAM-1 accelerates atherosclerosis by promoting leukocyte infiltration. Moreover, upregulation of ICAM-1 is associated with excessive activation of the RELA/NLRP3 axis, and leads to dysfunction of the human umbilical vein endothelium by regulating inflammatory responses and oxidative stress [90]. Similarly, we found that both RELA and ICAM-1 were stably upregulated in the disease group.
C-C motif chemokine ligand 1 (CCL1), a CC chemokine family member, mediates the migration of monocytes to regions of inflammation [91]. Harpel et al. [92] have suggested that CCL1 and its receptor CCR8 are closely associated with inflammation and atherosclerosis. CCL1 is upregulated in several immune diseases, such as rheumatic heart disease, atopic dermatitis and psoriasis [93, 94]. CCR8 is activated by CCL1 and mediates the recruitment of T helper 2 cells to sites of inflammation [95]. Notably, CCL1 has no significant chemotactic effect on neutrophils [96]. Olsen et al. [95] have demonstrated that elevated circulating CCL1 is strongly associated with prognosis and mortality in colorectal cancer. Current studies on CCL1 in AMI or UC are inadequate. In conclusion, our data indicated that TNF, STAT3, ITGAX, ICAM1 and CCL1 are important targets for the treatment of patients with both UC and AMI.
Although previous studies have explored the hub genes associated with AMI and UC separately [97, 98], most current animal studies have been limited to a single disease, thus failing to address the clinical coexistence of multiple diseases. Moreover, few studies have explored the common molecular mechanisms between these diseases through advanced bioinformatics methods. We sought to elucidate the molecular mechanisms underlying AMI and UC, to provide novel insight into the clinical management of patients with these comorbidities. Decreasing mortality and improving quality of life in patients with AMI combined with UC may be achieved by further standardizing clinical management strategies. However, this study has several limitations. First, only significant DEGs between disease and normal tissues were analyzed; consequently, certain genes that showed no significant changes in expression but played an important regulatory role were ignored. In addition, animal experiments are necessary to further reveal the causal relationship between these hub genes and the pathogenesis of the two diseases.
Conclusions
We used a bioinformatics approach to study patients with AMI combined with UC. We discovered many pathogenic mechanisms shared by AMI and UC and possibly mediated by the specified hub genes. Our research offers novel ideas to further investigate the molecular mechanisms underlying AMI combined with UC.