Introduction
Dilated cardiomyopathy (DCM) is a non-ischemic heart disease characterized by unexplained dilatation and systolic dysfunction of the left ventricle [1]. DCM is the second most common cause of heart failure, with a prevalence of approximately 1:2500 in the general population, and which has increased in recent years [2, 3]. Similarly, the prevalence of heart failure, a costly and severe condition, has also significantly increased [4]. Symptom-based therapies, such as angiotensin converting enzyme inhibitors/angiotensin receptor antagonists, aldosterone antagonists and β-blockers, are common treatments for symptomatic patients with DCM. For patients with end-stage heart failure with DCM, implantation of left ventricular assist devices and orthotopic heart transplantation are options for improving patient prognosis [5].
Although many studies have explored the mechanism of DCM, its exact pathological mechanism remains unclear. DCM is known to have multiple genetic or acquired causes. The most common acquired causes are inflammation, nutritive-toxic influences and metabolic disorders [6]. However, approximately 35% of patients with DCM have a positive family history, thus indicating that genetic mutations are important causes of DCM. Most affected genes associated with DCM encode cytoskeletal, sarcomere or nuclear envelope proteins, such as LMNA, MYH7, TNNT2, TTN, RBM and SCN5A [1]. These mutated genes ultimately lead to abnormal structure and function of the heart muscle in patients with DCM. Additionally, fibrosis and the renin-angiotensin-aldosterone system play important roles in the pathogenesis of DCM [7]. Cardiac fibrosis can increase cardiac rigidity, contractile impairment and the occurrence of diastolic dysfunction and malignant arrhythmias [8, 9]. However, the exact pathological mechanism of DCM remains unclear. Given the consistently high morbidity and mortality of DCM, more studies are necessary to investigate the molecular basis of DCM development, discover new biomarkers for diagnosis and identify novel molecular targets for treatment.
In recent years, bioinformatics analyses using microarray and high-throughput sequencing technologies have been widely used to explore disease-associated genes, biological processes and biomarkers for diagnosis and prognosis. Previous studies have investigated hub genes and long non-coding RNAs in patients with DCM and heart failure with bioinformatics methods; however, these studies are limited by small sample sizes [10–12]. The present study used high-throughput sequencing and microarray profiles with a larger sample size than those in prior studies to identify target genes in DCM patients with heart failure. Additionally, enrichment analysis, construction of a logistic regression model, and prediction of miRNA-circular RNA (circRNA) and small molecular compounds were performed to explore the pathogenesis of DCM. The current study provides a comprehensive understanding of the genetic etiology of DCM, as well as valuable information for the clinical diagnosis and treatment of the disease.
Results
Construction of a Weighted Gene Co-expression Network
The workflow of the analysis is shown in Figure 1. To investigate genes strongly associated with DCM with heart failure, we constructed gene co-expression networks with the GSE141910 and GSE5406 datasets in R. A soft power β=3 was selected in GSE141910 (Figure 2A) and β=11 chosen in GSE5406 (Figure 3A), to construct a scale-free weighted gene co-expression network. A total of 11 co-expression modules in GSE141910 (Figure 2B) and 12 co-expression modules in GSE5406 (Figure 3B) were identified in the present analysis.
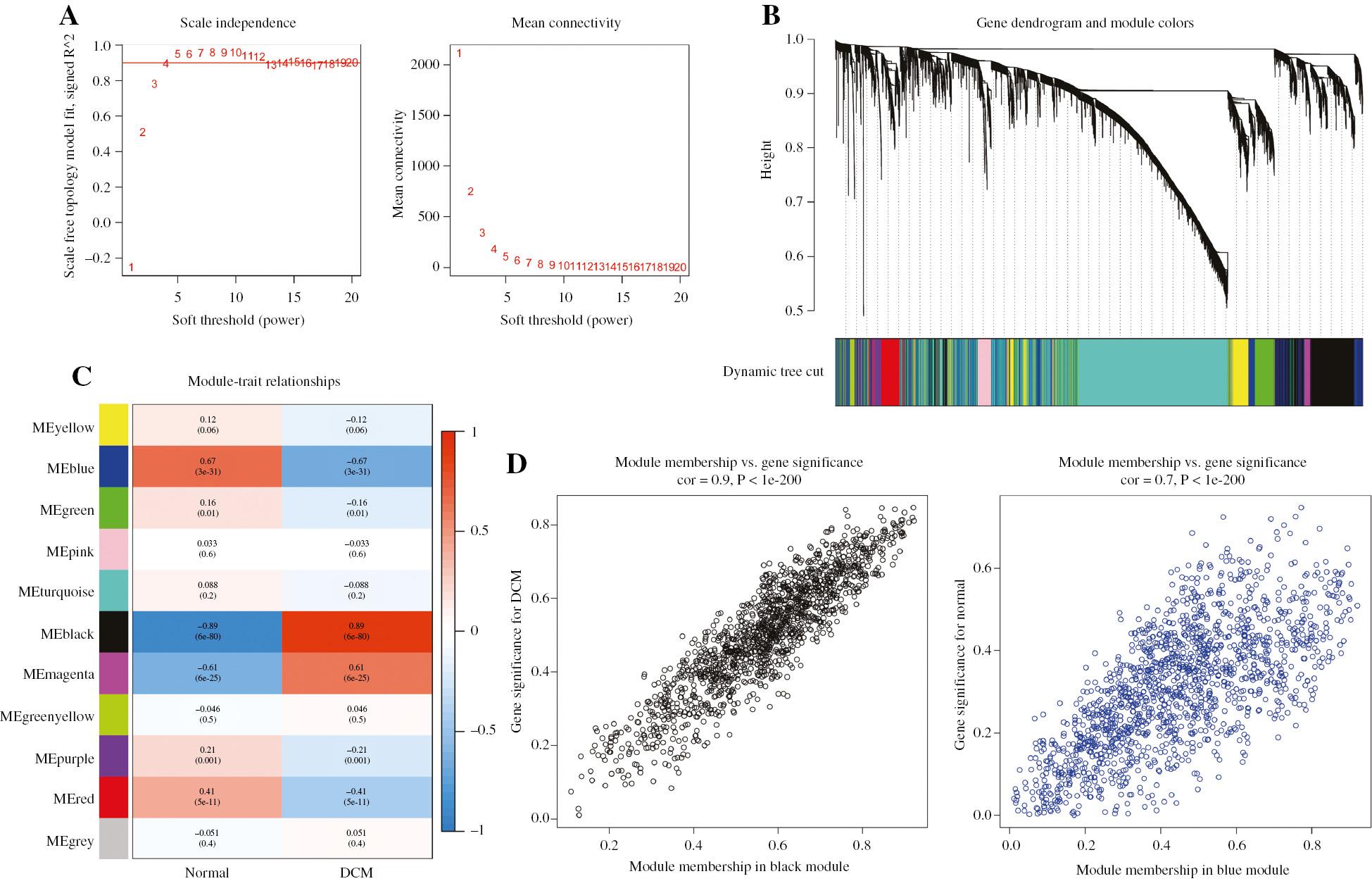
Identification of Gene Co-expression Networks by WGCNA in the GSE141910 Dataset.
(A) Effects of power values on the scale independence and mean connectivity of gene co-expression modules of DCM. (B) Cluster dendrogram of gene co-expression modules, with 11 different modules indicated in different colors. (C) Correlation heat map of gene modules and clinical traits, with the corresponding correlation and P-value displayed in each cell. (D) Gene significance for DCM in the black and blue modules.
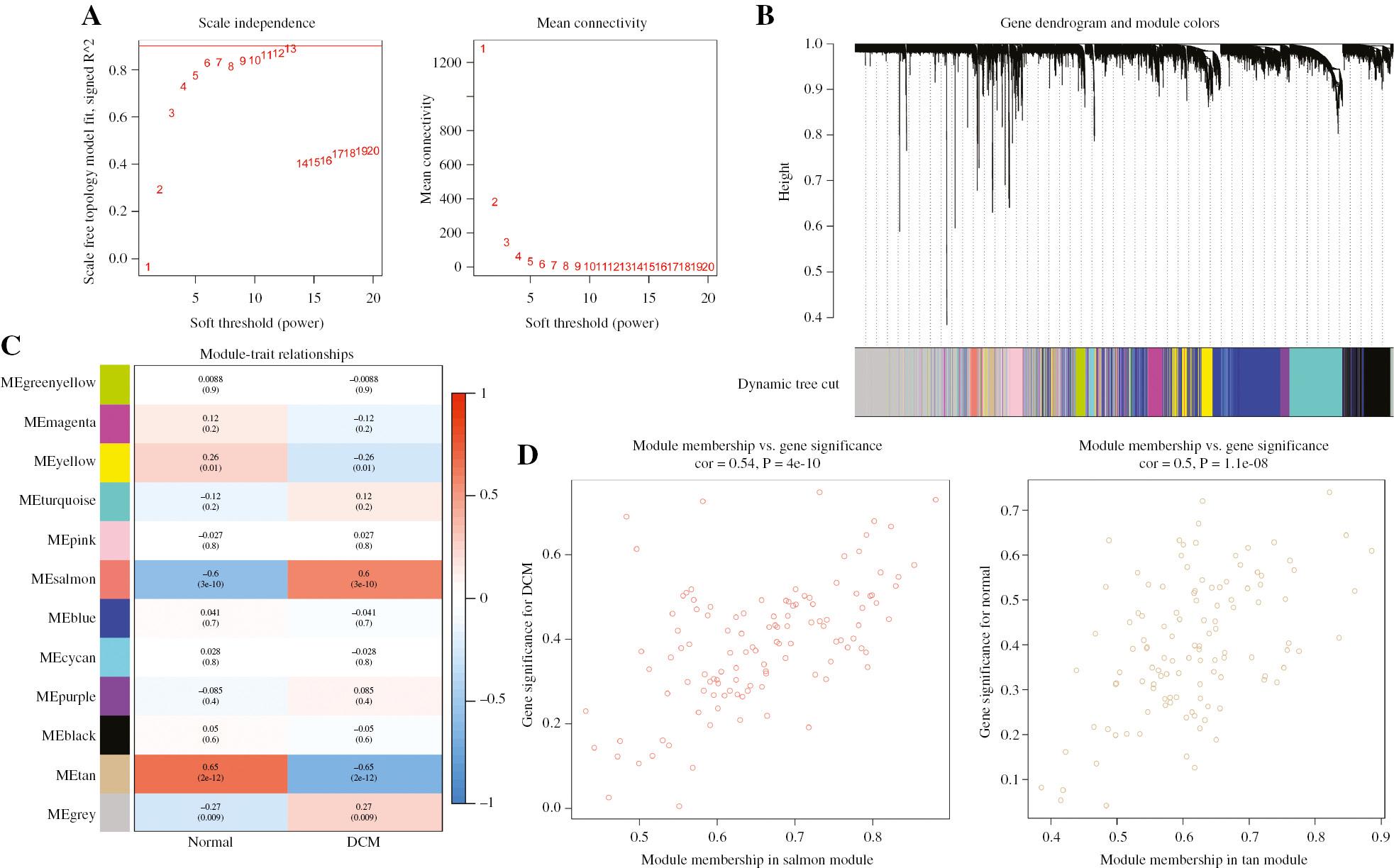
Identification of Gene Co-expression Networks by WGCNA in the GSE5406 Dataset.
(A) Effects of power values on the scale independence and mean connectivity of gene co-expression modules of DCM. (B) Cluster dendrogram of gene co-expression modules, with 12 different modules indicated in different colors. (C) Correlation heat map of gene modules and clinical traits, with the corresponding correlation and P-value displayed in each cell. (D) Gene significance for DCM in the salmon and tan modules.
Identification of Co-expression Modules and Functional Annotations
To investigate the association between clinical traits and co-expression modules, we generated heatmaps in the present analysis. The black module was found to be most positively correlated with DCM (gene number=1466, r=0.89, P=6e-80), whereas the blue module was most negatively correlated with DCM (gene number=1476, r=0.67, P=3e-31), in GSE141910 (Figure 2C). The scatterplots of gene significance (GS) and module membership (MM) were calculated for both the black module (R=0.9, P<1e-200) and blue module (R=0.7, P<1e-200) (Figure 2D), and suggested a strong correlation between the genes in these two modules and DCM. Gene Ontology (GO) analysis revealed that genes in the black module were enriched mainly in extracellular matrix-associated biological process, such as extracellular matrix organization, extracellular structure organization and collagen-containing extracellular matrix (Supplementary Figure S1A). In contrast, genes in the blue module were enriched primarily in carboxylic acid transport, cardiac muscle contraction and ion channel complex (Supplementary Figure S1C). Kyoto Encyclopedia of Genes and Genomes (KEGG) analysis indicated that genes in the black module were enriched mainly in ECM receptor interaction and the renin angiotensin system (Supplementary Figure S1B), whereas genes in the blue module were enriched mainly in the TNF signaling pathway and NF kappa B signaling pathway (Supplementary Figure S1D).
In the analysis of GSE5406, the salmon module displayed the highest positive correlation with DCM (gene number=116, r=0.6, P=3e-10), whereas the tan module exhibited the most negative correlation with DCM (gene number=116, r=0.65, P=2e-12) (Figure 3C). Scatterplots of GS and MM were calculated in the salmon module (R=0.54, P<4e-10) and tan module (R=0.5, P=1.1e-08) (Figure 3D). Additionally, the GO analysis revealed that genes in the salmon module were enriched in biological processes associated with the extracellular matrix (Supplementary Figure S2A), whereas genes in the tan module were enriched in detoxification of copper ion, contractile actin filament bundle and G protein coupled peptide receptor activity (Supplementary Figure S2C). Moreover, KEGG analysis indicated that genes in the salmon module were enriched in focal adhesion, ECM receptor interaction and protein digestion and absorption (Supplementary Figure S2B), whereas genes in the tan module were enriched in mineral absorption and the FoxO signaling pathway (Supplementary Figure S2D).
Gene Set Enrichment Analysis
Gene set enrichment analysis (GSEA) was performed on the basis of the GO and KEGG analyses for all expressed genes in the GSE141910 and GSE5406 datasets. The results for GSE141910 indicated upregulation of molecular carrier activity and the transforming growth factor (TGF) beta signaling pathway, and downregulation of mitochondrial RNA processing, glycolysis and gluconeogenesis in DCM (Figure 4A, B). The results for GSE5406 indicated upregulation of calcium activated cation channel activity and beta alanine metabolism, and downregulation of regulation of telomerase activity and the MAPK signaling pathway in DCM (Figure 4C, D).
Identification of Differential Expression of Genes in DCM
Differentially expressed genes (DEGs) between patients with DCM and healthy donors were screened with the limma R package, with cut-off criteria of |log fold change|;0.6 and adj. P<0.05. A total of 2073 DEGs were identified in GSE141910 (Figure 5A), and 151 DEGs were identified in GSE5406 (Figure 5B). Subsequently, 26 overlapping genes among the DEGs and positively correlated modules in both datasets were obtained (Figure 5C), along with four overlapping genes among the DEGs and negatively correlated modules in both datasets (Figure 5D).
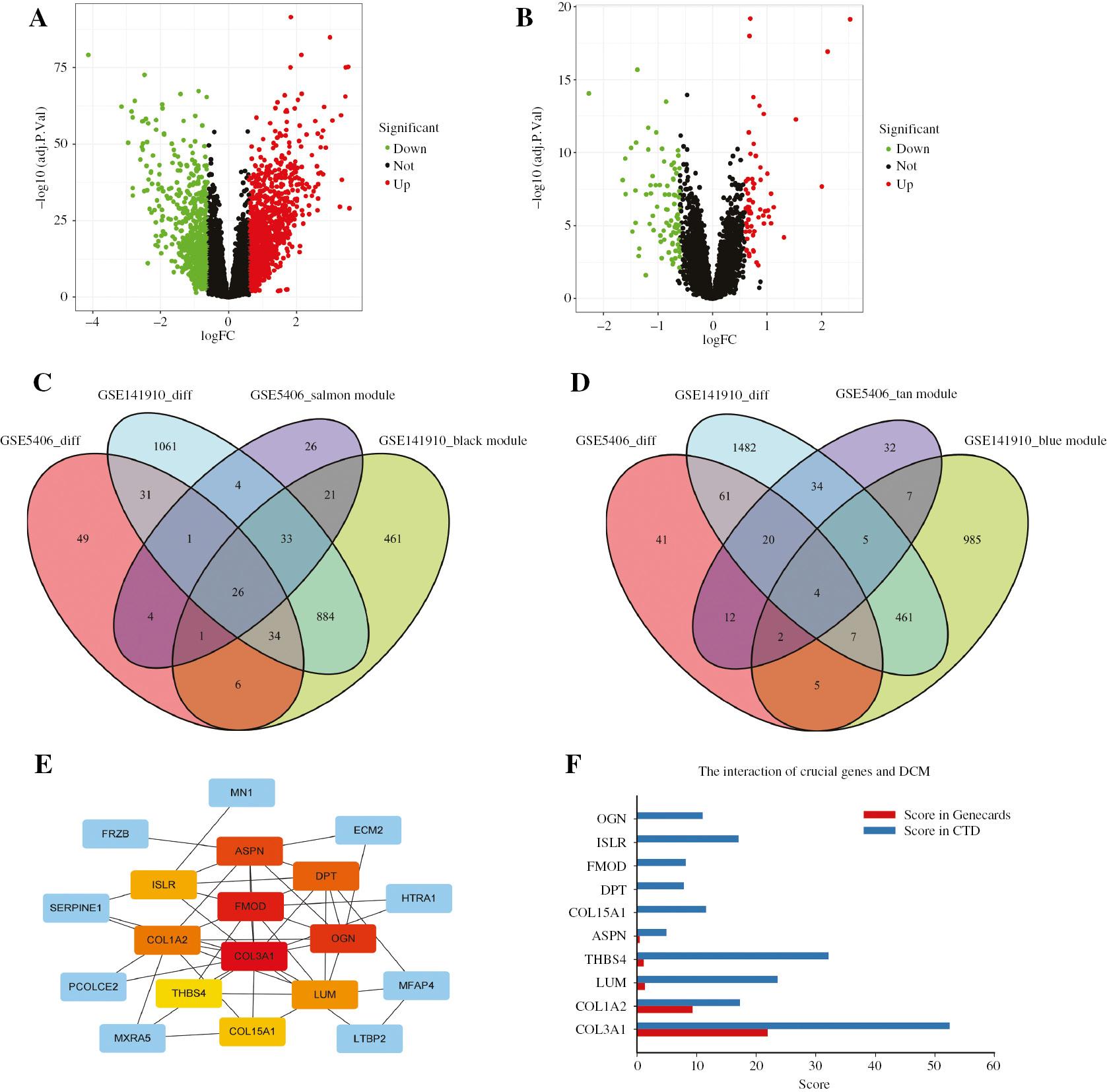
Identification of Differentially Expressed Genes (DEGs) and Hub Genes.
(A) Volcano plot of DEGs in GSE141910. (B) Volcano plot of DEGs in GSE5406. (C) Venn diagram of genes among DEG lists and positively correlated co-expression modules, with a total of 26 overlapping genes in the intersection of DEG lists and two co-expression modules. (D) Venn diagram of genes among DEG lists and negatively correlated co-expression modules, with a total of four overlapping genes in the intersection of DEG lists and two co-expression modules. (E) Identification of hub genes from the PPI network by using the maximal clique centrality (MCC) algorithm. Red represents higher MCC scores, and yellow represents lower MCC scores. (F) Bar graph of genes among the top ten hub genes with higher MCC scores and two online databases (Genecards and CTD). The x axis represents the score obtained by the hub genes in the databases (relevance score in Genecards and inference score in CTD), and the y axis represents the gene symbols.
Construction of the Protein–Protein Interaction (PPI) Network and Identification of Hub Genes
The PPI network, constructed with the STRING database with the 30 overlapping genes, consisted of 20 nodes and 48 edges (Supplementary Figure S3). The hub genes were identified with the CytoHubba plugin in Cytoscape. On the basis of the maximal clique centrality (MCC) sores, the top ten highest-scoring genes were COL15A1, OGN, ASPN, COL1A2, COL3A1, LUM, THBS4, FMOD, DPT and ISLR (Figure 5E). Subsequently, the Genecards database and comparative toxicogenomics database (CTD) were used to further screen the hub genes. From these databases, five overlapping genes were obtained: COL1A2, COL3A1, LUM, THBS4 and ASPN (Figure 5F). However, owing to the low score of ASPN in both databases, COL1A2, COL3A1, LUM and THBS4 were selected for further analysis.
Validation of Expression of Hub Genes
In the GSE141910 (Supplementary Figure S4A–D) and GSE5406 datasets (Supplementary Figure S4E–H), COL1A2, COL3A1, LUM and THBS4 showed significant upregulation in patients with DCM (all P<0.001). These four hub genes also had elevated expression in patients with DCM in another expression profile dataset, GSE116250 (Supplementary Figure S4I–L).
Identification of Enriched Biological Processes and Pathways for Hub Genes
GSEA revealed that four genes (COL1A2, COL3A1, LUM and THBS4) were significantly enriched in the ECM receptor interaction pathway, TGF beta signaling pathway, extracellular matrix and collagen biological process in GSE141910 (P<0.05) (Figure 6, Supplementary Figure S5). GSEA of the same genes in GSE5406 showed similar results, with significant enrichment in extracellular matrix biological process, and the TGF beta signaling pathway and focal adhesion pathway (P<0.05) (Figure 7, Supplementary Figure S6).
Least Absolute Shrinkage and Selection Operator (LASSO) Model Values are a Potential Predictive Marker for DCM
A LASSO model was constructed with the expression profiles of four genes—COL1A2, COL3A1, LUM and THBS4—from the GSE141910 dataset (Figure 8A). All four genes showed non-zero regression coefficients, and the value of lambda.min was found to be 0.04013996. The model index was generated with the following formula: index=−54.4056564479581 + COL3A1×(−3.35510157525245) + COL1A2× 2.99369800089778 + LUM×2.73749080158362 + THBS4×1.16297453349407. The area under the receiver operating characteristic curve (AUC) of the training set was 0.978, and that of the test set was 0.992 (Figure 8B), thus suggesting that values obtained from the model may be used as a biomarker for diagnosing DCM. The LASSO model was also validated in two independent datasets: validation set 1 (GSE5406) and validation set 2 (GSE116250). Both datasets showed high AUC values (GSE5406: AUC=0.977; GSE116250: AUC=0.933, Figure 8C). Moreover, the model exhibited high sensitivity, specificity, positive predictive value, negative predictive value, and accuracy values (Supplementary Table S1), thereby indicating its potential for accurately distinguishing patients with DCM.
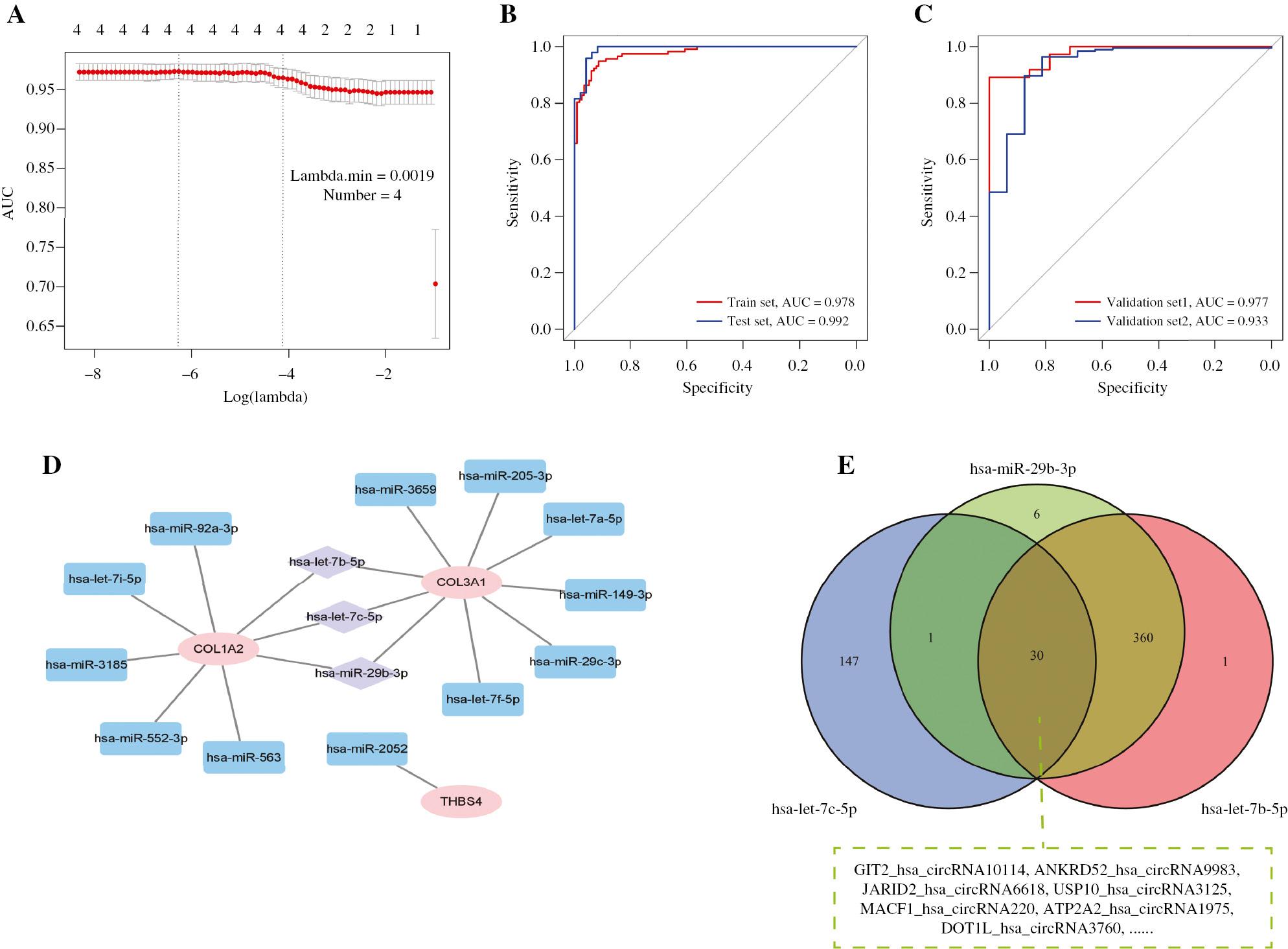
Model for Predicting DCM, and Prediction of miRNAs and circRNAs.
(A) LASSO model. (B) ROC curve analysis of the training set and test set. (C) ROC curve analysis of validation set 1 (GSE5406) and set 2 (GSE116250). (D) Interaction network between hub genes and targeted miRNAs, with genes in pink, miRNAs in blue and overlapping miRNAs in purple. (E) Venn diagram of targeted circRNAs among three overlapping miRNAs.
Additionally, the calibration plot showed that this model was statistically indistinguishable from the ideal model (Supplementary Figure S7A). Decision curve analysis (DCA) revealed that this gene-based model provided more benefit than both the treating-none curve and treating-all curve at any threshold probability, thus suggesting the efficacy of this model (Supplementary Figure S7B). Finally, a visual nomogram was constructed to help clinicians use this model (Supplementary Figure S8).
miRNA and circRNA Prediction
The target miRNAs of four hub genes were identified with miRWalk 2.0. A total of 15 miRNAs were detected, and three miRNAs (hsa-let-7b-5p, hsa-let-7c-5p and hsa-miR-29b-3p) were found to target both COL3A1 and COL1A2 (Figure 8D). Corresponding circRNAs of the three overlapping miRNAs were predicted with StarBase 2.0, and 30 circRNAs targeting the three key miRNAs were identified (Figure 8E). The complete list of 30 circRNAs is provided in Supplementary Figure S9.
Drug Prediction
COL1A2, COL3A1, LUM and THBS4 were uploaded into DGIdb to identify potential drugs. A total of three drugs (ocriplasmin, collagenase clostridum histolyticum and vasopressin) were identified after the analysis (Supplementary Table S2).
Discussion
Through bioinformatic analysis, the present study identified four candidate genes (COL3A1, COL1A2, LUM and THBS4) associated with DCM. Enrichment analysis indicated that these genes were all enriched in extracellular matrix, and the TGF beta signaling, focal adhesion, and collagen related pathways. A LASSO model constructed with expression profiles of these four genes indicated that they may serve as biomarkers for DCM. The miRNA-mRNA network analysis revealed that hsa-let-7b-5p, hsa-let-7c-5p and hsa-miR-29b-3p may play crucial roles in the pathogenesis of DCM. The circRNA prediction suggested that 30 potential circRNAs, including GIT2_hsa_circRNA10114, ANKRD52_hsa_circRNA9983 and JARID2_hsa_circRNA6618, may be involved in DCM. Additionally, three potential small molecule compounds were identified with the DGIdb database in this study.
DCM is one of the most common causes of heart failure in the general population, yet its molecular basis and pathological mechanism remain poorly understood. However, advances in bioinformatics have provided researchers with an optimal approach to explore disease-associated genes and potential medication targets, on the basis of extensive genetic data [13]. Although several studies have investigated specific disease-associated molecular targets of DCM through bioinformatics in recent years, these studies had small sample sizes, and their bioinformatic analyses were not comprehensive and rigorous. In the present study, the datasets with large sample sizes were used for the analysis (a total of 252 patients with DCM and 182 healthy donors were included), and differential expression analysis and weighted gene co-expression network analysis (WGCNA) were applied to identify the hub genes of DCM. These genes were validated in another dataset with a large sample size (37 patients with DCM and 14 healthy donors). Clinically, the LASSO model and visual nomogram constructed in this study may support prediction of DCM, and possibly aid in its early detection and treatment. Moreover, the prediction of related miRNAs and circRNAs may facilitate the discovery of novel DCM targets for future research. Therefore, the present bioinformatic analysis reports a thorough analysis of DCM, providing crucial information for further understanding the disease’s molecular basis and pathological mechanism.
Differential expression and WGCNA analyses were used to identify overlapping genes between the GSE141910 and GSE5406 datasets, thus resulting in a total of 30 genes, including ASPN, PENK, LTBP2, COL1A2, COL3A1, COL15A1, LUM, THBS4, MYOT, SLC19A2, SERPINE1 and SERPINE2. These findings were consistent with those from other studies of DCM. For instance, in Zhao et al. [11], the GSE3585 and GSE42955 datasets were subjected to differential expression analysis, which led to the identification of 89 DEGs, including COL1A2, THBS2, THBS4 and CTGF. In another study by Zhang et al. [14], ASPN was identified as a potential biomarker for patients with DCM with heart failure. Furthermore, LTBP2 inhibition has been suggested to decrease myocardial oxidative stress injury, myocardial fibrosis and myocardial remodeling in DCM model rats through the NF-κB signaling pathway [15].
GSEA of whole expression profiles of GSE141910 and GSE5406 indicated that several pathways, including the TGF beta signaling pathway, mitochondrial RNA processing, calcium activated cation channel activity and MAPK signaling pathway, may play critical roles in the pathogenesis of DCM. TGF β signaling, the primary contributor to fibrosis development, has also been reported to be involved in the progression of DCM and to be activated by angiotensin II in cardiac remodeling [16]. Inhibition of TGF beta may have therapeutic effects in various fibrotic heart conditions, including DCM. Calcium channel dysfunction has also been associated with DCM. A previous study has indicated that the expression of CACNA1C is downregulated in DCM cardiomyocytes, and the L-type calcium channel currents are diminished [17]. In another study, Orai channel deficient mice have been found to develop rapid dilated cardiomyopathy with the loss of channel function [18], thereby indicating that Orai channels, which are an essential part of cellular calcium signaling, have major roles in DCM pathogenesis. In agreement with the GSEA results, GO and KEGG analyses of WGCNA key modules also revealed the critical role of TGF beta signaling and fibrosis in patients with DCM, as well as the dysfunction of cardiac muscle contraction and ion channels.
Four hub genes, COL1A2, COL3A1, LUM and THBS4, were identified through PPI network analysis and related online databases. These genes were associated with fibrosis and myocardium remodeling, thus highlighting the major role of cardiac fibrosis in the progression of DCM. Cardiac fibrosis can impair the contractile function of the myocardium, thereby leading to poor prognosis in patients with DCM. In a study by Verdonschot et al. [19], COL1A2 and COL3A1, along with COL1A1, COL5A1 and TGFβ1, had significantly higher expression in patients with DCM who did not respond to cardiac resynchronization therapy (CRT) than DCM patients who responded to CRT. Furthermore, higher expression of COL1A1, COL3A1 and matrix metalloproteinase-9 (MMP9) was observed in a DCM mouse model than in control mice [20]. Recent studies have indicated that LUM and THBS4 are associated with heart failure [21, 22]. In addition, another study has indicated that Thbs4(-/-) mice exhibit higher heart weight because of increased extracellular matrix deposition, thus leading to impaired channel function and decreased vessel density [23]. However, limited studies have explored the relationship between DCM and these two genes (LUM and THBS4). The present analysis provides the first evidence that LUM and THBS4 may play crucial roles in the pathogenesis of DCM. Nonetheless, further validated experiments are required to confirm these findings.
The single-gene GSEA of the four hub genes revealed enrichment in biological processes and pathways associated with fibrosis, including extracellular matrix, collagen binding, the TGF beta signaling pathway, focal adhesion and smooth muscle contraction. Furthermore, the expression levels of these four genes in GSE116250 were found to be significantly higher in patients with DCM than controls.
A LASSO model was generated by using the expression profiles of COL1A2, COL3A1, LUM and THBS4. Receiver operating characteristic (ROC) curve analysis indicated that the model had a high AUC value in both the training and test sets, thereby suggesting that these four genes may serve as potential biomarkers of DCM. A calibration plot was constructed, and DCA further validated the model’s effectiveness. Notably, the model displayed high specificity, negative predictive value, sensitivity and positive predictive value, thus indicating its strong ability to distinguish patients with DCM from healthy donors. Additionally, the model was validated in two independent datasets, GSE5406 and GSE116250, both of which showed high AUC values. These findings further supported that increased expression of COL1A2, COL3A1, LUM and THBS4 may contribute to the pathogenesis of DCM.
miRNAs are endogenous non-coding RNA molecules that target the 3‘UTR regions of genes, thereby regulating gene expression through suppressing target gene translation [24]. Recent studies have suggested important roles of miRNAs in the progression of DCM. In ADAR2-/- mice, downregulation of miR-29b, miR-405 and miR-19 ais associated with cardiomyopathy and cardiac fibrosis [25]. To further explore the molecular basis of DCM, we performed miRNA prediction on COL1A2, COL3A1, LUM and THBS4.
Our analysis identified three overlapping miRNAs (hsa-let-7b-5p, hsa-let-7c-5p and hsa-miR-29b-3p) that may play important roles in DCM. These findings were consistent with those from a study by Onrat et al. [26] indicating significantly higher let-7b-5p and let-7c-5p in DCM than in ischemic cardiomyopathy. However, Wang et al. [27] have reported downregulation of the miR29 (miR29a, miR29b and miR29c) and miR133 (miR-133a and miR-133b) families in patients with DCM. Therefore, further experimental validation of the effect of hsa-miR-29b-3p on DCM is needed. Recent studies have investigated circRNAs—miRNA sponges that inhibit miRNA function [28]—and their potential role in cardiovascular diseases [29]. In myocardial infarction, upregulation of circRNA CDR1as has been found to increase cardiac infarct size [30]. Another study has indicated differential expression of circRNAs originating from the TTN gene in neonatal rat hearts compared with adult rat hearts, thereby suggesting a critical role of circRNAs in heart development [31]. In a study by Siede et al. [32], compared to control patients with non-failing hearts, patients with DCM showed a decrease in circDNAJ6C and an increase in circSLC8A1, circ CHD7 and circATXN10. However, the roles of circRNAs in DCM remain poorly understood. In the present study, the prediction of circRNAs based on the three overlapping miRNAs resulted in the identification of 30 circRNAs that may have high potential value as novel biomarkers for DCM.
Finally, three potential drugs, ocriplasmin, collagenase, clostridum histolyticum and vasopressin, were identified on the basis of the four hub genes. Studies have shown that arginine vasopressin levels are elevated in dogs and patients with DCM [33, 34]. Therefore, the therapeutic value of vasopressin inhibitors in treating DCM may be helpful but requires further investigation. These three drugs could be validated through in vitro experiments to provide a reference for clinical practice.
Limitation
A common limitation of bioinformatic analysis is poor reproducibility, because of the variability of results generated with different methods and parameters. Therefore, this study used large sample sizes and multiple analysis methods to screen the hub genes and ensure validation. The results showed good overall reproducibility. However, because this study investigated the molecular basis for DCM through only bioinformatics, the findings require experimental validation.
Conclusion
In summary, this study used datasets with large sample sizes and multiple analysis methods to screen and validate the hub genes in DCM. The hub genes, including COL3A1, COL1A2, LUM and THBS4, were identified as potential biomarkers of DCM. Furthermore, the present analysis identified several hub gene-associated molecules, such as miRNAs (hsa-let-7b-5p, hsa-let-7c-5p and hsa-miR-29b-3p) and circRNAs (GIT2_hsa_circRNA10114, ANKRD52_hsa_circRNA9983, JARID2_hsa_circRNA6618, etc.) that may contribute to the development of DCM. These findings may help improve diagnosis and the development of new treatment strategies for preventing the progression of DCM, and may serve as a foundation for further studies.
Materials and Methods
Data Processing
The high-throughput sequencing expression profile GSE141910 dataset, based on the GPL16791 Illumina HiSeq 2500 (Homo sapiens) platform, and the microarray expression profile GSE5406 dataset, based on the GPL96 [HG-U133A] Affymetrix Human Genome U133A Array platform, were downloaded from the Gene Expression Omnibus (GEO) database (http://www.ncbi.nlm.nih.gov/geo/) [35] for further analysis. The GSE141910 dataset includes heart tissue samples from 166 patients with DCM with heart failure and 166 healthy donors without heat failure. The GSE5406 dataset includes heart tissue samples from 86 patients with DCM with heart failure and 16 healthy donors without heart failure. In addition, the validation expression profile GSE116250 dataset, based on the GPL16791 Illumina HiSeq 2500 (Homo sapiens) platform, includes heart tissue samples from 50 patients with DCM with heart failure and 14 healthy donors without heart failure. All data from the three expression files were displayed in fragments per kilobase of transcripts per million mapped reads and normalized by log2 conversion or robust multi-array analysis. Probes without gene symbols were removed, and genes with more than one probe were averaged in R software.
Weighted Gene Co-expression Network Construction
The WGCNA package in R was used to process the data from GSE141910 and GSE5406 to construct gene co-expression networks [36]. WGCNA can be used to identify candidate biomarkers and therapeutic targets by indicating modules with highly related genes among samples. Soft powers β=3 in GSE141910 and β=11 in GSE5406 were selected with the function pickSoftThreshold to build a scale-free network. The topological overlap matrix (TOM) and the corresponding dissimilarity (1-TOM) were calculated from the adjacency matrix. Subsequently, the 1-TOM matrix was clustered with hierarchical clustering to classify similar gene expression into different modules. The module size was set to 50–10,000, merge cut height was set to 0.25, and verbose was set to 3. To further identify module-trait relationships and functional modules in the network, we calculated MM and GS values for all modules. MM represents the correlation between gene expression values and module eigengenes [37], whereas GS represents the correlation between genes and samples [38]. Modules with high correlation coefficients were selected for further analyses.
Identification of Differentially Expressed Genes
The limma package in R was used to screen the DEGs between patients with DCM with heart failure and healthy donors [39]. The criteria for differential analysis were an adjusted P value less than 0.05 and |log2-fold change| greater than 0.6 in both GSE141910 and GSE5406. The adjusted P-value was calculated with the Benjamini and Hochberg false discovery rate (<0.05). Subsequently, the overlapping genes between DEGs and co-expressed genes from the most positively and negatively correlated modules were visualized with the R package VennDiagram.
Enrichment Analysis
GSEA is a powerful analytical method that allows for sequencing of genes on the basis of differential expression between two groups. It can be used to investigate whether preset gene sets are enriched at the top or bottom of the sequencing table [40]. The GSEA software was used to explore differential biological functions and pathways between patients with DCM and healthy donors. The gene set c5.go.v7.4.symbols.gmt [Gene ontology] and c2.cp.kegg.v7.4.symbols.gmt [Curated] were downloaded for analysis. P<0.05 was considered statistically significant.
The clusterProfiler package in R was used to conduct GO analysis and KEGG pathway enrichment analysis [41]. An adjusted P value <0.05 was selected as the criterion for analysis. GO annotation included biological process, cellular component and molecular function.
PPI Network Analysis
To predict the PPI network, the STRING database (version 11.0, https://string-db.org/) was used [42]. Genes with a score of 0.4 or higher were chosen for PPI network construction. Subsequently, the PPI network was visualized and analyzed with Cytoscape software [43].
Hub Gene Screening and Validation
CytoHubba, a Cytoscape plugin, was used to calculate the MCC of each node and screen the top ten hub genes in the PPI network [44]. The search term “dilated cardiomyopathy” was used to identify genes associated with DCM in the Genecards (https://www.genecards.org/) database and the CTD (http://ctdbase.org/) [45, 46]. Four overlapping genes (COL3A1, COL1A2, LUM and THBS4) between hub genes and databases were further analyzed. The differential expression of these four genes in patients with DCM compared with healthy donors was investigated with Student’s t-test in the validation dataset GSE116250.
Construction and Validation of the LASSO Model
LASSO was used to select the best features for high-dimensional data [47]. The expression profiles of the identified hub genes were extracted to construct the LASSO model with the glmnet R package to estimate the predictive value of hub genes for DCM. The genes were considered biomarkers for DCM when their regression coefficients were non-zero in the LASSO model. An index for the model was created with the following formula: index=ExpGene1×Coef1 + ExpGene2×Coef2 + ExpGene3×Coef3 +…, where Exp represents the expression value of genes, and Coef represents the regression coefficient of genes. GSE141910 was randomly assigned to a training set (70%) and test set (30%). In addition, GSE5406 and GSE116250 were used as validation sets to evaluate the reliability of the model.
The ability of the LASSO model to identify DCM was assessed by calculation of the AUC with the pROC R package [48]. An AUC greater than 0.7 indicates a well-constructed model. The calibration performance and DCA were conducted with the rms package and rmda package in R, respectively [49, 50].
miRNA and circRNA Prediction
The hub genes were analyzed with miRWalk 2.0 (http://mirwalk.umm.uni-heidelberg.de/) to predict targeted miRNAs [51]. Five datasets (TargetScan, miRanda, miRDB, miRWalk and RNA22) were used for analysis with selection conditions set at P<0.05 and a minimum seed sequence length of a heptamer. The predicted miRNAs were visualized with Cytoscape. Overlapping miRNAs were selected to predict upstream molecules of circRNAs with the StarBase v2.0 tool (http://starbase.sysu.edu.cn/starbase2/index.php) [52], with a selection condition of the highest reliability (very high stringency ≥ 5). The overlapping circRNAs were visualized with the R package VennDiagram.
Drug Screening
The drug prediction database DGIdb (https://www.dgidb.org) was used to obtain gene-drug interactions and potential drug candidates for the identified hub genes [53]. The hub genes were input into DGIdb to retrieve all possible gene-drug interactions.