Abbreviations: DCM, dilated cardiomyopathy; GEO, Gene Expression Omnibus; GO, Gene Ontology; KEGG, Kyoto Encyclopedia of Genes and Genomes; PPI, protein-protein interaction.
Introduction
Dilated cardiomyopathy (DCM) is a primary myocardial disease of unknown cause but frequently has a genetic background. The disease is characterized by left or right ventricular or bilateral ventricular enlargement with reduced ventricular systolic function, with or without congestive heart failure, and usually affects young patients with few comorbidities. Ventricular or atrial arrhythmia is more prevalent. The disease is progressive, and death can occur at any stage [1, 2]. The concept of DCM is as a dynamic disease requiring constant optimization of medical and nonpharmacological evidence-based treatments [3]. The advent of next-generation sequencing has contributed to the discovery of a large number of genes and alleles related to DCM, which makes it possible to intervene at the molecular level, especially with regard to patients in whom routine treatment is not suitable. Moreover, cascade genetic testing can identify those who are at risk or have early-stage disease, offering the opportunity for early intervention [4, 5]. Therefore, genetic testing and gene therapy in DCM will play an increasingly important role.
Although there have numerous efforts to understand the genetic mechanisms of initiation and progression of DCM, knowledge is still lacking regarding the key genes involved in both the occurrence and the progression of DCM and how to treat and control deterioration with DCM through targeted drugs. Therefore, it is important and urgent to uncover the mechanisms of DCM and develop novel therapeutic routes. Gene chip or expression profiling is a gene-level detection technique that makes it possible to detect expression of the entire genome in the same sample in a single experiment [6, 7]. A large amount of correlated genomic data from DCM patients has been deposited in public databases [8, 9]. Using the Gene Expression Omnibus (GEO; https://www.ncbi.nlm.nih.gov/geo/) public database, we identified differentially expressed genes (DEGs) of dilated cardiomyopathic heart and nonfailing heart biopsy samples and performed Gene Ontology (GO) and signaling pathway analyses.
We downloaded the GSE3586 soft formatted family file and series matrix file from the GEO. Using the RStudio-installed limma package, we identified DEGs between gene expression profiles of biopsy samples of dilated cardiomyopathic hearts, profiled at the time of transplantation, and those of biopsy samples of nonfailing hearts that were not transplanted because of palpable coronary calcifications [10, 11]. Then GO and pathway enrichment analyses were performed on the DAVID website (https://david.ncifcrf.gov) [12]. Through this work, we discovered the important biological functions and pathways in DCM, which can help to determine the hub genes and their roles in diagnosis, prognosis, and therapeutic targets.
Materials and Methods
Microarray Data
The GSE3586 microarray dataset [13] was downloaded from the GEO [14, 15]; these data are based on two genome-wide expression studies involving complementary DNA and short-oligonucleotide microarray platforms. The GSE3586 dataset contains data from 13 dilated cardiomyopathic hearts and 15 nonfailing donor hearts.
Data Preprocessing
After the GSE3586 dataset had been downloaded, we found that the expression matrix retrieved from the GEO had been standardized and probe identification numbers were transformed into gene symbols. If a gene’s symbols corresponded with multiple probe IDs, the expression level of that gene was represented by the mean of the probes.
Identification of DEGs
DEG analysis refers to identification of genes that are expressed at significantly different levels by multiple modes of analysis [16]. The txt and soft data GSE3586 files were analyzed with RStudio and the R package limma to identify the DEGs between dilated cardiomyopathic heart biopsy samples and nonfailing heart biopsy samples [16–18]. We identified the DEGs by using a threshold of the log2-fold change of 0.6 or greater and adjusted Pvalues less than 0.01 for subsequent analysis.
GO and Pathway Enrichment Analysis
To further understand the biofunctions of the DEGs, GO analysis and Kyoto Encyclopedia of Genes and Genomes (KEGG) analysis were performed through multiple online tools to annotate genes and gene products [19], identify the biological roles of the DEGs [20], and interconnect the genomic information with high-level functional information [21]. DAVID, among them, is a website with gene annotation and visualization, and provides gene attributes. P<0.05 was considered statistically significant and was used as the cutoff criterion [21, 22].
Integration of the Protein-Protein Interaction Network and Module Analysis
Afterward, we constructed a connection diagram to show DEG-encoded proteins and protein-protein interaction (PPI) information to understand the potential functions of a certain protein (STRING, https://string-db.org) [23]. We chose a minimum required interaction score of 0.400 (medium confidence) as the significance limitation. Then the PPI network acquired from the STRING database was imported into Cytoscape with the help of the Molecular Complex Detection (MCODE) plug-in in the software to mark the pivotal modules in huge complex PPI networks [24]. Except for a K-core of 4, we used the default parameters of MCODE.
Drug-Gene Interaction and Prediction of Transcription Factors
DGIdb (the drug-gene interaction database; https://dgidb.org) is an online database that integrates several databases, including ChEMBL and Ensembl, to search for drug-gene interactions by gene symbols. We imported the nine hub genes of the pivotal modules into DGIdb [25] to get the drug-gene interaction results. Furthermore, the prediction of related transcription factors was performed with PASTAA [26] (a program for predicting transcription factors that regulate a user-defined set of genes.)
Results
Identification of DEGs
The GSE3586 dataset was analyzed with RStudio to identify the DEGs. By comparing dilated cardiomyopathic heart biopsy samples versus nonfailing heart biopsy samples, we identified a total of 172 genes, including 162 upregulated DEGs and ten downregulated DEGs (Table 1 and Figure 1), using adjusted Pvalues less than 0.01 and a modulus of the log2 fold change of 0.6 or greater as criteria.
Differentially Expressed Genes were Identified From the GSE3586 Dataset, Including 162 Upregulated Genes and Ten Downregulated Genes in Dilated Cardiomyopathic Heart Biopsy Samples Compared with Nonfailing Heart Biopsy Samples.
Status | Gene |
---|---|
Upregulated | DLAT, GJA5, CDC42EP3, SEC31L1, PAIP2, UNR, PRSS11, ZNFN1A5, YWHA, SUCLA2, ATP6V1D, ZNF83, ECHDC1, GHITM, CPT1B, Ells1, ASAH1, HNRPC, CRYM, FLJ14054, ANKMY2, PRDX3, ACO2, TTC12, FBXO8, TP53INP2, DKFZp434I1610, NFE2L2, ZNF567, DLD, HIST1H4E, VDAC3, MLYCD, EIF4A2, FH, RPL22, TOP2B, MGC2714, NDUFA5, KIF5B, FLJ13710, ZNF23, ZNF600, TAX1BP1, C2orf25, LDB3, APLP2, E2-230K, SCHIP1, EXT1, COPS8, ZNF165, SGCG, UQCRC2, DNM3, HSPA2, STK38L, PSMC6, NDUFV1, HSPCB, SSPN, TARS, UBB, NFIA, P29, CRYZ, MPP4, CASQ2, NNT, MCC, CDH2, FLJ26175, AKAP13, BCAR1, ARIH2, GJA1, ARGBP2, EIF4G2, MGC7036, SDHD, MORF4L2, SGCD, SMARCC1, KLHDC2, CANX, NIPSNAP3A, CAP2, PTPN11, MFN2, TXNIP, MATR3, SLC40A1, HBP1, HDLBP, NQO3A2, ZNF7, GNAS, DAP3, AK3, LAPTM4B, ODC1, ZNF267, HMGN2, HIST2H2BE, KIAA1618, CGI-51, STAT1, BRP44L, PPARA, MAOA, ATP5A1, NOL5A, MB, MAP2K6, KIAA0774, SH3BP2, PSME1, LUM, PPP1R14C, LMOD2, RBBP7, GUP1, FLJ22662, OCIL, ZFP106, SLC8A1, NFE2L1, ERBP, ELOVL6, INSIG1, PROS1, DNCL1, LTBP2, SLC16A1, HMGB3, PKP2, NPM1, FHOD3, DSP, FLJ21127, MGC15619, HSPA1L, AF1Q, CD36, FYCO1, HSPA8, KIF5C, NDRG4, LOC339924, FGF1, FLJ20152, EEF1A1, LOC92235, PDE4DIP, NRG1, C6orf142, TNNI3, CSDA, TNNC2, SFRP1, PAM, ACPP, ABLIM1, ZNF364, ACSL1, CA3, MYOZ2, FHL2 |
Downregulated | HDAC9, C18orf22, PAPD5, PRAME, DEPC-1, IFITM3, ARFIP1, IFITM2, C3, KCNIP2, CCL2 |
GO Enrichment Analysis
We uploaded the DEGs to the DAVID website to identify the significant GO terms and classified them into three functional categories: biological processes, molecular functions, and cell components (Figure 2). In the biological process group, the upregulated DEGs were enriched mainly in genes associated with the heart development and oxidation-reduction processes. The downregulated DEGs were enriched in genes associated with the regulation of vasculature development response to biotic stimulus and positive regulation of apoptotic cell clearance. In the cell component group, the upregulated DEGs were enriched mainly in genes associated with the actin cytoskeleton and intercalated disk, but as for the downregulated DEGs, we did not find any useful information. In the molecular function group, the upregulated DEGs were enriched mainly in genes associated with oxidoreductase activity, acting on NAD(P)H, and wide pore channel activity. For the downregulated DEGs, there were no available significant molecular functions (P<0.05) (Table 2).
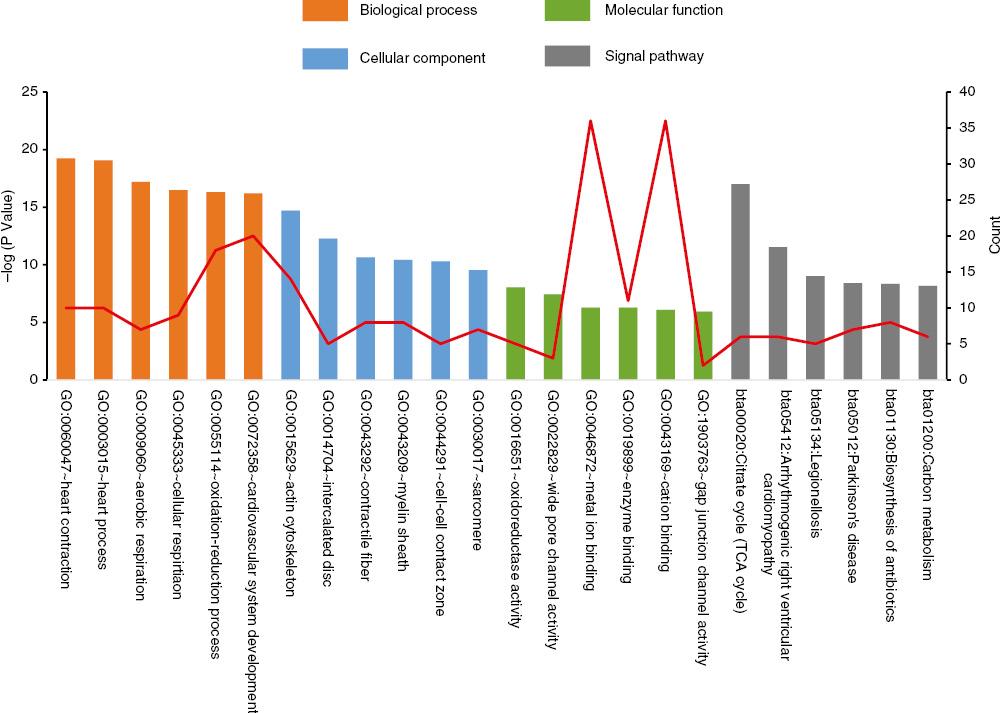
Gene Ontology Terms and Kyoto Encyclopedia of Genes and Genomes Pathways of Differentially Expressed Genes Significantly Enriched in Dilated Cardiomyopathy.
TCA, tricarboxylic acid.
Gene Ontology Analysis of Differentially Expressed Genes (DEGs) Associated with Dilated Cardiomyopathy.
Category | Term | Count | P |
---|---|---|---|
Upregulated DEGs | |||
GOTERM_BP_FAT | GO:0060047 heart contraction | 10 | 7.07×10−7 |
GOTERM_BP_FAT | GO:0003015 heart process | 10 | 8.08×10−7 |
GOTERM_BP_FAT | GO:0055114 oxidation-reduction process | 18 | 3.25×10−6 |
GOTERM_BP_FAT | GO:0009060 aerobic respiration | 7 | 3.79×10−6 |
GOTERM_CC_FAT | GO:0015629 actin cytoskeleton | 14 | 2.28×10−5 |
GOTERM_CC_FAT | GO:0014704 intercalated disc | 5 | 1.70×10−4 |
GOTERM_CC_FAT | GO:0043292 contractile fiber | 8 | 4.71×10−4 |
GOTERM_MF_FAT | GO:0016651 oxidoreductase activity, acting on NAD(P)H | 5 | 0.003076304 |
GOTERM_MF_FAT | GO:0022829 wide pore channel activity | 3 | 0.005179203 |
GOTERM_MF_FAT | GO:0019899 enzyme binding | 11 | 0.008728806 |
Downregulated DEGs | |||
GOTERM_BP_FAT | GO:2000427 positive regulation of apoptotic cell clearance | 2 | 3.69×10−3 |
GOTERM_BP_FAT | GO:2000425 regulation of apoptotic cell clearance | 2 | 5.89×10−3 |
GOTERM_BP_FAT | GO:0045765 regulation of angiogenesis | 3 | 6.16×10−3 |
GOTERM_BP_FAT | GO:1901342 regulation of vasculature development | 3 | 7.37×10−3 |
GOTERM_BP_FAT | GO:0009607 response to biotic stimulus | 4 | 1.07×10−2 |
GOTERM_BP_FAT | GO:0043277 apoptotic cell clearance | 2 | 1.76×10−2 |
GOTERM_BP_FAT | GO:0010574 regulation of vascular endothelial growth factor production | 2 | 1.83×10−2 |
GOTERM_BP_FAT | GO:0001525 angiogenesis | 3 | 1.98×10−2 |
GOTERM_BP_FAT | GO:0050766 positive regulation of phagocytosis | 2 | 2.27×10−2 |
GOTERM_BP_FAT | GO:0048514 blood vessel morphogenesis | 3 | 2.93×10−2 |
KEGG Pathway Analysis
The associated KEGG pathways for the most significantly enriched DEGs were identified by means of the DAVID website and are listed in Figure 2. In the KEGG pathway group, the most enriched signaling pathways for the upregulated DEGs were in the citrate cycle (also known as the tricarboxylic acid cycle) and arrhythmogenic right ventricular cardiomyopathy. The downregulated DEGs were enriched only in Chagas disease (also known as American trypanosomiasis), at a cutoff of P adjusted<0.05 (Table 3).
Pathway Enrichment Analysis of Differentially Expressed Genes (DEGs).
Pathway | Term | Count | P | Genes |
---|---|---|---|---|
Upregulated DEGs | ||||
KEGG_PATHWAY | bta01100: metabolic pathways | 18 | 0.038370751 | UQCRC2, ODC1, NDUFA5, ACO2, MAOA, DLAT, ATP6V1D, ASAH1, ACSL1, NNT, MLYCD, FH |
KEGG_PATHWAY | bta01130: biosynthesis of antibiotic | 8 | 0.002208011 | NDUFV1, SDHD, DLD, ATP5A1, SUCLA2, EXT1 |
KEGG_PATHWAY | bta05012: Parkinson’s disease | 7 | 0.002189488 | ODC1, ACO2, DLD, SDHD, AK3, DLAT, SUCLA2, FH |
KEGG_PATHWAY | bta00020: citrate cycle (TCA cycle) | 6 | 5.68×10−6 | UQCRC2, NDUFA5, NDUFV1, SDHD, UBB, ATP5A1, VDAC3 |
KEGG_PATHWAY | bta05412: arrhythmogenic right ventricular cardiomyopathy | 6 | 2.59×10−4 | ACO2, DLD, SDHD, DLAT, SUCLA2, FH |
KEGG_PATHWAY | bta01200: carbon metabolism | 6 | 0.002714139 |
SGCG, PKP2, GJA1, DSP, SGCD, CDH2, DLD
ACO2,SDHD, DLAT, SUCLA2, FH |
Downregulated DEGs | ||||
KEGG_PATHWAY | bta05142: Chagas disease | 2 | 0.044623307 | CCL2, C3 |
TCA, tricarboxylic acid.
PPI Analysis and Gene Module Analysis
With the aid of the STRING online database and the Cytoscape software platform, we formed a complex PPI network for genes, consisting of 85 nodes and 162 edges (Figure 3A) and accounting for 49.42% of the DEGs. According to the filtering of node degree 8 criteria, the top ten genes were UBB, ATP5A1, FH, UQCRC2, DLD, DLAT, SDHD, NDUFV1, PRDX3, and HSP90AB1. Then we identified the key module (nine nodes and 34 edges, Figure 3B) from the PPI network with use of the MCODE plug-in. The functional annotation of the hub genes is listed in Table 4 and visualized in Figure 4. Our results show the functions of nine hub genes and their probable role in DCM via use of DAVID.
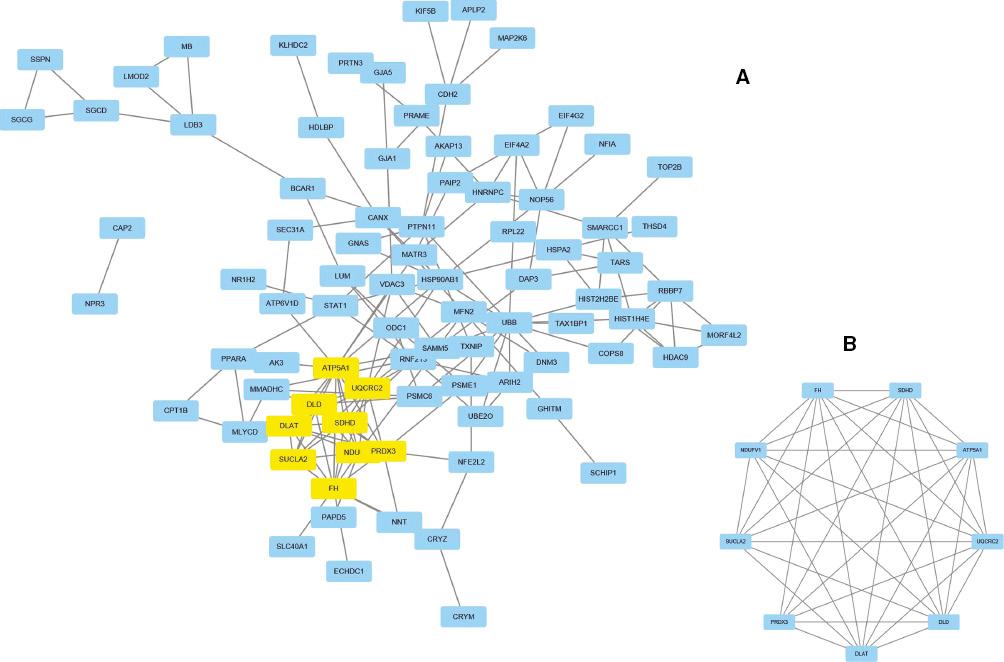
Protein-Protein Interaction (PPI) Networks of Differentially Expressed Genes (DEGs).
(A) By means of the STRING online database, 172 genes/nodes were filtered into a DEG-PPI network. (B) The key module from the PPI network.
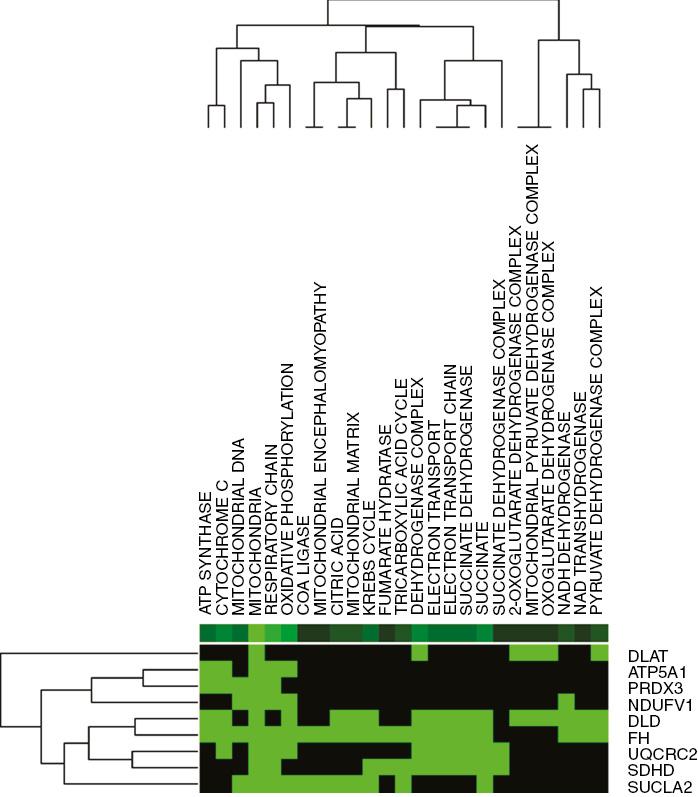
Functional Enrichment Analysis of Nine Candidate Hepatocellular Dilated Cardiomyopathy–Related Genes (green indicates that the gene is enriched in the item, black indicates that the gene is not enriched in the item).
Color images are available online. TCA, tricarboxylic acid.
Functional and Pathway Enrichment of Nine Hub Genes.
Category | Term | Genes |
---|---|---|
GOTERM_BP_FAT | GO:0055114 oxidation-reduction process | UQCRC2, NDUFV1, DLD, PRDX3, FH |
GOTERM_BP_FAT | GO:0045333 cellular respiration | UQCRC2, NDUFV1, DLD, FH |
GOTERM_BP_FAT | GO:0015980 energy derivation by oxidation of organic compounds | UQCRC2, NDUFV1, DLD, FH |
GOTERM_CC_FAT | GO:0005739 mitochondrion | UQCRC2, NDUFV1, DLD, SDHD, PRDX3, DLAT, ATP5A1, SUCLA2, FH |
GOTERM_CC_FAT | GO:1990204 oxidoreductase complex | UQCRC2, NDUFV1, DLD, DLAT |
GOTERM_MF_FAT | GO:0030523 dihydrolipoamide S-acyltransferase activity | DLAT |
GOTERM_MF_FAT | GO:0016651 oxidoreductase activity, acting on NAD(P)H | NDUFV1, DLD |
KEGG_PATHWAY | mcc00020: citrate cycle (TCA cycle) | DLD, SDHD, DLAT, SUCLA2, FH |
KEGG_PATHWAY | mcc01200: carbon metabolism | DLD, SDHD, DLAT, SUCLA2, FH |
KEGG_PATHWAY | mcc01100: metabolic pathways | UQCRC2, NDUFV1, DLD, SDHD, DLAT, ATP5A1, SUCLA2, FH |
TCA, tricarboxylic acid.
Drug-Gene Interactions and Prediction of Transcription Factors
The drugs with the highest scores for each gene were identified with use of DGIdb. Among them, the SDHD and NDUFV1 genes correspond to the drugs succinic acid and CHEMBL1161866, respectively. However, no interactions were found for PRDX3 (Table 5). Meanwhile the top 20 transcription factors predicted by hub gene expression are listed in Table 6, and on the basis of the Pvalue and association score that were used to evaluate the correlation between the disease and transcription factors, we identified the transcription factor–binding site of the highest-scoring transcription factor (Figure 5). Neurofibromin1 could be a potential target for gene therapy.
Gene-Drug Interactions.
Gene | Drug | Interaction types | Sources | Score |
---|---|---|---|---|
DLD | CHEMBL1161866 | NA | DrugBank | 4 |
UQCRC2 | Coenzyme Q2 | NA | DrugBank | 2 |
DLAT | CHEMBL1161866 | NA | DrugBank | 4 |
SUCLA2 | Succinic acid | NA | DrugBank | 4 |
ATP5A1 | Quercetin | NA | DrugBank | 2 |
FH | Ditiocarb | NA | NCI | 2 |
SDHD | Succinic acid | NA | DrugBank | 6 |
NDUFV1 | CHEMBL1161866 | NA | DrugBank | 6 |
PRDX3 | No interactions found |
NA, not available; NCI, National Cancer Institute.
Top 20 transcription Factors Predicted by Hub Gene Expression.
Rank | Matrix | Transcription factor | Full name | Association score | P |
---|---|---|---|---|---|
1 | NF1_Q6 | Nf-1 | Nuclear factor 1 | 4.48 | 2.19×10−4 |
2 | NF1_Q6_01 | Ctf-1, Ctf-2 | Cardiotrophin factors 1, Cardiotrophin factors 2 | 4.48 | 2.19×10−4 |
3 | HES1_Q2 | Hes-1 | Hairy-related factors 1 | 3.255 | 2.52×10−3 |
4 | IK3_01 | N/A | N/A | 3.252 | 2.52×10−3 |
5 | ZIC2_01 | Zic2, Zic2 | Zinc finger factors 2 | 2.792 | 6.74×10−3 |
6 | MAX_01 | Max1 | MYC associated factor X 1 | 2.577 | 1.07×10−2 |
7 | HFH4_01 | Foxf1, Foxj1 | Forkhead box (FOX) factors f1, Forkhead box (FOX) factors j1 | 2.521 | 1.33×10−2 |
8 | MYCMAX_03 | Max, C-myc | MYC associated factor X, MYC proto-oncogene | 2.414 | 1.70×10−2 |
9 | CMYB_01 | N/A | N/A | 2.372 | 1.80×10−2 |
10 | MYCMAX_02 | Max1, C-myc | MYC associated factor X 1, MYC proto-oncogene, | 2.346 | 1.83×10−2 |
11 | ZBRK1_01 | N/A | N/A | 2.34 | 1.83×10−2 |
12 | ARNT_02 | Arnt | Aryl hydrocarbon receptor nuclear translocator | 2.332 | 1.85×10−2 |
13 | USF_01 | Usf1 | upstream transcription factor 1 | 2.332 | 1.85×10−2 |
14 | USF_02 | Usf1 | upstream transcription factor 1 | 2.332 | 1.85×10−2 |
15 | USF_Q6_01 | Usf-1, Usf1 | upstream transcription factor 1 | 2.332 | 1.85×10−2 |
16 | USF_Q6 | Usf1, Usf2a | upstream transcription factor 1, upstream transcription factor 2a | 2.331 | 1.85×10−2 |
17 | COUP_01 | Coup-tf1, Hnf-4alpha1 | Coup-Transcription factor 1, hepatocyte nuclear factor 4 alpha1 | 2.221 | 2.49×10−2 |
18 | HFH1_01 | Hfh-1 | forkhead box Q1 | 2.22 | 2.49×10−2 |
19 | STRA13_01 | Stra13 | stimulated by retinoic acid 13 | 2.196 | 2.49×10−2 |
20 | P300_01 | P300 | zinc finger protein 300 | 2.125 | 3.12×10−2 |
NA, not available.
Discussion
DCM is a heart muscle disease characterized by left ventricular or biventricular dilatation and systolic dysfunction [27]. The implementation of optimal pharmacological and nonpharmacological treatment has dramatically improved the prognosis of DCM [28], but despite this therapeutic success, emerging evidence suggests that some patients remain vulnerable to sudden cardiac death and refractory heart failure requiring heart transplantation or mechanical circulatory support [29]. Moreover, genetic screening suggests that up to 40% of DCM is genetically determined [29]. Familial screening should be systematically performed to obtain an early diagnosis in relatives as this facilitates prompt prophylactic therapy in early or preclinical disease [30] but gene diagnosis and therapy of DCM are not currently sufficiently advanced in clinical practice.
In this study, we screened potentially related pathogenic genes by comparing dilated cardiomyopathic heart biopsy samples with nonfailing heart biopsy samples during transplantation. Among 162 upregulated DEGs and ten downregulated DEGs identified, we selected nine key candidate genes, DLD, UQCRC2, DLAT, SUCLA2, ATP5A1, PRDX3, FH, SDHD, and NDUFV1, for characterization and found connections with these lay in the oxidation-reduction process, cellular respiration, the citrate cycle, and carbon metabolism of metabolic pathways by using GO and KEGG annotation and PPI network analysis.
Dihydrolipoamide dehydrogenase (encoded by DLD) is essential for energy metabolism across eukaryotes. The enzyme modulates production of reactive oxygen [31], and also affects branched-chain amino acid metabolism, resulting in maple syrup urine disease [32]. Mitochondrial complex III deficiency caused by UQCRC2 mutation can lead to neonatal-onset recurrent hepatocellular insufficiency, lactic acidosis, hypoglycemia, ketosis, and hyperammonemia [33, 34], and alterations in gene-related mitochondrial proteins and their quantities have been confirmed to affect prognosis and progression in many cancers [35, 36]. The DLAT gene encodes component E2 of the multienzyme pyruvate dehydrogenase complex, and E2 is the main mitochondrial autoantigen that plays a vital role of the innate immune recognition system in the pathogenesis of primary biliary cirrhosis [37]. SUCLA2 encodes a subunit of the enzyme succinate-CoA ligase and is at the crossroads of several biochemical pathways encompassing the citrate cycle, heme synthesis, propionyl-CoA metabolism, and ketone body utilization, to name a few [38]. ATP5A1 encodes a subunit of mitochondrial ATP synthase and plays a critical role in catalyzing ATP synthesis. Not only is it highly expressed in glioblastoma tumor cells and endothelial cells of microvascular proliferation [39], it is also involved in progression of clear cell carcinoma and mitochondrial encephalopathy, which could cause death in neonates [40, 41]. PRDX3 encodes a mitochondrial protein that has antioxidant functions and has physiological and pathological significance, with the initiation and progression of various cancer types, such as ovarian cancer, endometrial cancer, and prostate cancer [42–45]. FH encodes a homotetrameric mitochondrial protein that is part of the citrate cycle [46]. Current data show that recessive mutations in FH cause severe encephalopathies and early death, whereas dominant FH mutations predispose to tumor formation [47], but fumarate hydratase (which is encoded by this gene) can be used as a multifaceted tumor suppressor in cancer [48]. SDHD encodes one of four subunits of the citrate cycle enzyme succinate dehydrogenase (SDH), which is responsible for the oxidation of succinate [49]. The most important SDH-related mutation is in the SDHD gene, which has a key function both in familial and nonfamilial hereditary paraganglioma/pheochromocytoma syndrome [50]. NDUFV1 encodes a 51 kDa subunit of NADH:ubiquinone oxidoreductase complex I. Complex I deficiency is one of the most common mitochondrial respiratory chain defects. Mutations in NDUFV1 are involved in autosomal recessive mitochondrial complex I deficiency, and account for a constellation of clinical features, including encephalopathies, myopathies, and Leigh syndrome [51, 52].
Among the predicted transcription factors that modulate gene expression in DCM, we chose the NFI/CTF family of mammalian transcription factors. The NFI/CTF family is a family of transcription-replication factors comprising polypeptides encoded by four paralogous genes located on different chromosomes in mammals (NFIA, NFIB, NFIC, and NFIX). The NFI family of proteins displays the unusual property of not only regulating the initiation of transcription but also mediating DNA replication. NFI recognition sequences are found in the promoter sequences of many cellular genes, where they may act as an activator or a repressor of transcription. Recently, it was proposed that NFI transcription-replication factors may be involved in long-range regulation of gene expression through the formation of chromatin barriers and by blocking the propagation of a heterochromatic structure [53, 54].
By the preceding identification of hub genes from a GO, KEGG, and transcription factor perspective, we know that proteins encoded by these genes are involved in mitochondrial activity. Mitochondria play a key role in providing ATP for the energy-consuming cardiac tissues. Mitochondrial cardiomyopathy is a myocardial condition characterized by abnormal heart structure and/or function secondary to genetic defects involving the mitochondrial respiratory chain. Mitochondrial DNA deletion mutations are common in a variety of heart lesions, such as DCM and hypertrophic cardiomyopathy [55, 56]. In conclusion, our study provides greater understanding of the pathogenesis and underlying molecular mechanisms in DCM. This contributes to the exploration of potential gene therapy targets. However, further molecular biological experiments are required to confirm the function of these identified genes in DCM.