Introduction
In discussions about the locations that make up the key productive nodes of the digital economy, Africa rarely gets a mention. Autonomous vehicles, machine learning systems, next-generation search engines and recommendations systems: how many of these technologies are ‘made in Africa’? The answer is actually ‘all of them’. In this briefing we seek to show how certain technologies that range from the emergent (autonomous vehicles) to the everyday (search engines and recommendation systems) are made possible by relatively low-paid African workers. African workers are making possible key technologies that underpin, or soon will underpin, the enormous profits made by large technology companies based in the global North. In the context of hyperbolic claims about automation and robotisation and the impending technological unemployment they are predicted to bring about, this briefing shows that African workers remain very much a part of contemporary digital capitalism.
When we buy a material product like a shirt or an apple, we can usually find out where they were produced from the labelling. This provides an entry-point to follow-the-thing back, through global commodity chains to the sites of production (Cook 2004). These connected lives and entanglements are, in turn, the beginning from which not just current capitalist relations can be understood, but also the ground on which alternate relations could be envisioned. The work of organisations like the Fairtrade Foundation and Living Wage Foundation, much of the vast sums spent on corporate social responsibility, and the glossy photographs of happy coffee pickers, fishers, children etc. that adorn supermarket walls and product packaging are all premised on a simple idea: that consumers do not want to be enrolled into production networks that are exploitative or harmful for workers on the other end (Graham and Haarstad 2011).
While there have been many efforts to trace commodity chains back to nodes of production, we see a particular need to examine digital labour in Africa for four main reasons. First, the nature of a lot of work has changed, with ever more jobs involving the creation and processing of information in relatively standardised ways. The entire Business Process Outsourcing sector is dependent on standardised, commodified chunks of work (e.g. answering phones, entering data and creating content) which can be performed non-proximately from clients and consumers (Graham and Anwar 2018a). Because of the data-heavy nature of many contemporary products, there is ever more demand for information-based services to support them. And as machine learning – the science of getting computers to make specific decisions – becomes ever more important in the contemporary era, there will be a concomitant rise in the need for people to train those machine learning systems. As this briefing will show through examples, many of these new jobs require little training other than basic computer literacy on the part of the worker.
Second, while stretched-out international divisions of labour are not new (Massey 1995), for the first time there is the possibility for digital jobs to move quickly and en masse to the African continent. Only 10 years ago, there were more Internet users in France than in all of Africa put together. The African continent now has over 700 million mobile phone subscribers and 450 million Internet users, and this number is expected to rise. Furthermore, rising labour costs in traditional outsourcing destinations like India and the Philippines combined with the increased connectivity in many parts of Africa now mean that African workers are becoming competitive in the global market for digital work. That is, capital can now tap into a hitherto disconnected reserve army of labour in the least likely places on the continent (Huws 2015). In fact, according to Smit et al. (2019), in their sample of seven countries (South Africa, Kenya, Rwanda, Uganda, Tanzania, Ghana and Nigeria), there are an estimated 4.8 million workers in Africa who derive income from digital platforms. With Internet connectivity on the continent increasing rapidly, that number is only likely to grow over the next few years.
Third, arms-length outsourcing jobs are characterised by notoriously problematic production practices (Fleming and Sturdy 2011; Taylor and Bain 2005). Within the outsourcing sector in Africa, workers are required to work under strict managerial and technocratic control, and face unsocial working hours, physical and psychological duress, and various forms of labour insecurities (Anwar and Graham 2019a). Similarly, in the context of remote digital work in Africa, workers do gain some forms of autonomy at work and discretion over tasks, due to non-proximate monitoring, but their actual flexibility is constrained due to long and unsociable working hours to offset low wages (Wood et al. 2019). Also, non-payment of wages is a genuine threat facing workers given that many platforms advertise that clients do not have to pay for work they deem unsatisfactory, with many workers reporting to have not been paid by the client (Anwar and Graham 2020). For place-based work such as ride-hailing services through Uber, increased urban mobility among its users is a positive outcome (Giddy 2019). But there is a potential for wider conflict with meter taxi drivers (Adebayo 2019) and also evidence of new forms of informalisation and casualisation in the local labour markets (Carmody and Fortuin 2019). Yet, in a highly informalised local labour markets that characterised much of the continent (Meagher 2016), these digital jobs are also creating a dilemma among African states with regards to regulation. Regulate and some of these jobs could soon disappear and relocate to other parts of the world, a concern raised to us by many workers we met across the continent. Not regulating the sector and expecting market-mediated measures to solve unemployment (e.g. gig economy employment relations) can further entrench informality, on the one hand, and weaken the social and interpersonal relations that define the informal sector, on the other hand (see Callebert 2017). Put differently, many digital jobs are characterised by poor working conditions, and many jurisdictions in which those jobs are located lack either the means, motivation or desire to improve them.
Fourth, most of the digital jobs that are created are defined by opacity in their production networks. A core feature of contemporary globalisation is that despite fostering world-spanning economic connectivities, it has weakened our social connections with our neighbours, while failing to introduce new ones with people on the other side of the world. Workers often know little about why they are doing what they are doing, or for whom they are working. The lack of publicly available data on this topic resulting from the notorious secrecy of large technology firms, and the seeming impenetrability of their production networks for digital services, mean that it is impossible to say with any degree of certainty how prevalent any of these activities are. The nature of the digital services produced through complex global production networks (Foster and Graham 2017) means that a ‘follow the thing’ approach (e.g. Cook 2004) can’t be attempted.
These four motivations have led us to this briefing on digital labour in Africa. It is not news that capitalism ‘must nestle everywhere, settle everywhere, establish connections everywhere’ (Marx and Engels 2002, 223). But our knowledge about contemporary digital capitalism is incredibly partial. For goods and services that are created with African digital labour, it is extremely challenging to trace their origins, their networks and labour processes, and under what conditions they were produced. While recent academic research is beginning to shed light on some of these issues in Africa, many forms of digital work still remain invisible to the public eye. The aim of this briefing is therefore to make the invisible more visible. Doing so allows us to put the human back in the machine, illustrate the ways in which Africa is embedded into contemporary digital production networks and motivate future research that will shed more light on these processes. We acknowledge that what we present here cannot be topologically or geographically comprehensive. However, the examples we discuss will hopefully motivate future research on the myriad ways in which African workers are enrolled into technology production networks and in some case high-value activities. We hope that such research can help highlight the moral entanglements that we all find ourselves within, and ultimately allow us to build effective transnational alliances and solidarities.
The findings we present in this briefing are based on a five-year study (2014–19) we conducted in South Africa, Ghana, Nigeria, Uganda and Kenya, involving in-depth interviews and group discussions with over two hundred stakeholders including digital workers, managers of outsourcing firms, government officials, trade unions, employment agencies, private sector associations and industry experts. Our extended fieldwork in these countries allowed us to build a detailed picture of most outsourced work happening in East, West and Southern Africa. Here, we have sought to construct a snapshot of the key ways in which African labour has been integrated into the production networks of digital products and services that will ultimately be deployed around the world. We focus on two types of digital work: machine learning and digital decision making. It is worth noting that these activities are performed by workers employed within firms or operating as freelancers through digital work platforms (e.g. Upwork, Freelancer.com, Amazon Mechanical Turk), which act as intermediaries between employers and workers (Graham and Anwar 2018b).
Digital work tasks in Africa
Machine learning
In 2017, we visited a machine learning training centre, in a rural African town, located about a ten-hour drive from the nearest international airport.1 It is here, in a place where few families possess any information and communication technologies beyond radios and feature phones, that workers are helping to build some of the world’s most advanced technologies and services. The foreign-owned centre began in 2012 in old shipping containers with communication towers and satellites for the Internet connection (Figure 1). It was in these shipping containers that workers started training machine learning systems that were designed on other continents.
Today the company operates out of a large open-plan office made out of multiple shipping containers. In it, three hundred workers sit over computer screens to train machine learning systems in three eight-hour shifts.2 These workers, who are paid approximately US$300 a month (a decent salary for the region), undertake tasks like tagging images, identifying objects in photographs (usually of suburban America) and matching names to photographs (usually of celebrities that they’ve never heard of). What the myriad tasks have in common is that they are jobs that computers are unable to do as effectively as humans – namely, structuring unstructured information.
Why is this work being done in Africa, specifically? One of the firm’s officials explained:
Primarily we do data services. Which means that we do not do voice services – as most call centres or Business Process Outsourcing firms do. That allows us to penetrate into regions with not very good infrastructure at all, because with voice you need a higher threshold. You need a higher benchmark in terms of infrastructure, security, and all those things.
A sales team in San Francisco liaises with large technology firms that are building products and services that involve machine learning approaches (for instance, autonomous cars that can’t yet be pre-programmed to react appropriately into every possible context they will encounter). These approaches typically require extremely large training datasets. In the case of autonomous vehicles, this is primarily accomplished through two different tasks: image annotation and video annotation. In order for driverless cars to drive safely on the roads, the cameras mounted on the cars must be able to identify objects, road markings, people, trees and traffic lights with great accuracy. To help cameras identify these things, millions of images of American cities need to be tagged so that computers can learn the difference between a pavement, a road, a tree and a person. Autonomous vehicles will eventually make fewer and fewer identification mistakes as more data is fed into the system. Indeed, the level of detail needed from the workers who build the training dataset is astonishing. Workers annotate not just large features on the road, but also wires, leaves, the outlines of door numbers and – in one case that we saw – a bird sitting atop a pole (Figure 2). Each image takes a worker anywhere from one to five hours to identify and tag all its contents. Workers have a target of seven images in their shift. A supervisor then checks the images for quality control before they are sent back to the client.
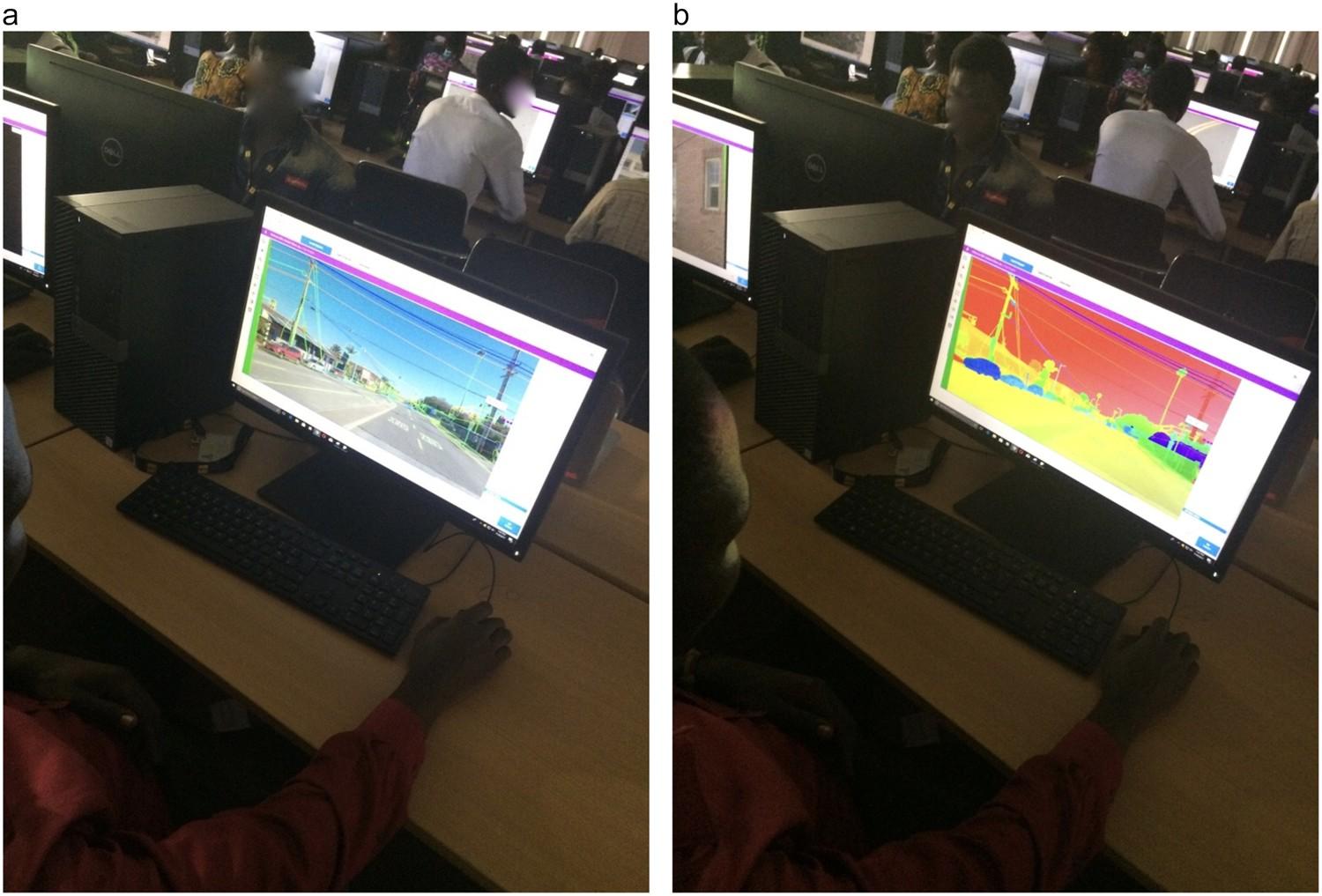
Image annotation for a machine learning system that could be used by autonomous vehicles. (a) image annotation in progress, and (b) a completed image.
The centre also did other kinds of digital work such as data entry, which involved matching names to photographs of minor celebrities the workers had never heard of, or correcting information about a hotel on travel sites. Some of the video annotation work involves workers being given a series of images extracted from a video (Figure 3), where a person (probably a worker) is sitting in a studio in front of a camera blinking their eyes for a few seconds. We asked one of the workers to describe video annotation work. He said that the client is ‘doing an algorithm that is detecting like the width of the eye when someone is driving and how it closes. So, we tag when an eye is open and when an eye is closed.’ Another worker, when asked to describe their job, said ‘I am tagging images.’ We then pressed for more detail: ‘But, why? What is somebody doing with these tagged images?’ The response then was just that: ‘They don’t tell me; they just want lots of tagged images.’
Like much outsourced machine learning work, here workers can only speculate about the utility of the jobs that they do. What is most noteworthy about the centre is that workers themselves are never told what the purpose of their work is. Workers know that they need to tell the computer what the difference is between a house and a road sign, but they are told nothing about the end-client, or what products are being built with all this information. Labour could not be more alienated: workers selling their labour power to far-off unknown firms, building products that they are unlikely to ever see or use.
Digital decision-making
Here we seek to describe not a single job-type, but rather a suite of activities that have a broad, but common purpose. Namely, to influence the decision-making processes of end-users of digital services. Contemporary search engines, recommendation systems and comparison websites all seek to narrow down what is usually an overwhelming amount of choices into a ranked and/or rated list for users. Google, for instance, deploys complex and often opaque processes to choose what information to make visible or invisible in response to any particular search terms. These tools are far from neutral reflections of underlying realities, rather they offer biased and unrepresentative representations (e.g. Ballatore, Graham, and Sen 2017).
But the opaque processes under the hood of most decision-making tools do not mean that they are not manipulatable. Indeed, the search engine optimisation (SEO) industry is approaching a worth of US$80 billion in the United States alone (McCue 2018). This industry is concerned with increasing the ranking (and thus visibility) of websites, images, news and even academic articles within ‘organic’ (i.e. unpaid) search results on both desktop and mobile interfaces. Workers in the SEO sector deploy either ‘white hat’ (strategies recommended by search engine companies) or ‘black hat’ (strategies they do not endorse) techniques. The SEO industry provides a good example of how the labour of African workers can impact on everyday technology used by billions of Internet users worldwide (see Figure 4 for an example of a job listing).
On one Internet platform (Upwork.com) alone, there are 2171 African workers registered as specialising in SEO on 17 September 2019. We encountered many of those workers in our interviews throughout the continent. One of them, a Nigerian who had been working in this area for four years, described his work:
I do SEO, which is link building, SEO copywriting, and pure SEO article writing. Though I do link building most of the time, comments link building, you know, write comments on blogs. You do research about blogs that are related to a particular industry and make comments based on the article, the topic of the article. The purpose of the comments is to build links to your client’s site. For example, I do research about a topic related to my clients’ business. I go to Forbes.com. Forbes has a blog and after reading through the contents and the comments on the blog, I put links back to my client’s site on those blogs and those links will boost my client’s website on the search engines. The client makes money through the products that he is promoting on his sites.
African digital labour and the future of work
The purpose of this briefing is not to offer a comprehensive overview of all digital labour happening in Africa, nor can it detail the myriad livelihoods impacted by these jobs. We have covered this elsewhere (see Anwar and Graham 2019b) and other studies have also highlighted deteriorating job quality in digital work in Africa (Graham, Hjorth, and Lehdonvirta 2017). Instead we aim to demonstrate that key emergent and everyday digital technologies have much they hide behind their sleek automated surfaces. Furthermore, we want to show that African workers play an important role in building and maintaining these technologies: acting as ‘data janitors’ (Irani 2015). Through processes of what Ekbia and Nardi (2017) refer to as heteromation, real people are still needed to structure, classify and tag an enormous amount of unstructured information for the companies using machine learning algorithms in their products. At a time when many scholars are predicting that machines will replace humans in the production process, thus increasing unemployment around the world (Frey and Osborne 2013), it is important to point to the fact that automation is not always what it seems. Technological advancements and the use of machines in production can destroy jobs in one location (primarily richer regions), but it can also open up many lower-income work possibilities for workers in poorer countries (see Fröbel, Heinrichs, and Kreye 1981).
Once we acknowledge that many contemporary digital technologies rely on a lot of human labour to drive their interfaces, we can begin to piece together what the new global division of labour for digital work looks like. We need detailed empirical studies of where value is created and captured in these production networks that are opaque by design. Research can start to make the invisible nodes of these chains more visible and highlight the pay and working conditions of the workers who make everything possible.
This is not to say that many of these digital workers are poorly paid by local standards, or that they are ungrateful for their jobs. But high unemployment and a large informal sector mean these digital jobs receive overly positive reviews, and the risks are sidelined (see Kuek et al. 2015). And it remains that digital workers in Africa are earning a tiny fraction of the profits generated from their labour. Importantly, African Labour Studies has a rich history of scholarship on labour movements and union organisation (Beckman, Buhlungu, and Sachikonye 2010; Lambert and Webster 2001). This body of work would greatly benefit from sustained research in this area such as examining political economy protests in digital labour (see Rizzo 2019 on Uber protest in South Africa), understanding new forms of labour movements (see Bramble and Barchiesi 2018) and examining unique ways in which agency is exercised in digital labour (Anwar and Graham 2019b). We need to acknowledge that companies are using recent advances in connectivity to tap into low-cost workforces at some of the world’s economic margins, and – as researchers, workers, activists and campaigners – hence, we need to devise ways of making some of those value chains more transparent.
In summary, we hope that this briefing has demonstrated that the contemporary digital economy offers jobs and opportunities to African workers, but even more of an opportunity to the international corporations that seek to profit from their labour. There is no easy means for firms and individuals based in the world’s economic margins to move up global value chains (Weber 2017), but this does not mean that we should throw up our hands and accept the status quo. As digital connectivity spreads to the last corners of the world, we hope this briefing will help build a greater socio-political response to the relatively labour-intensive nature of the contemporary digital economy in which African workers play a significant role in value creation. There is still much needed to be done in order to better understand African digital labour, challenge labour processes and employment relations, improve the quality of work and identify the common interests of workers, and the ways that their labour connects distant sites of production and consumption.